Establishment and validation of a prognostic immune-related lncRNA risk model for acute myeloid leukemia
Highlight box
Key findings
• This study provided innovative ideas for studying acute myeloid leukemia (AML) pathogenesis and improved the risk stratification of AML.
What is known and what is new?
• The current mechanism of prognosis evaluation for AML is relatively simple and it does not include the evaluation of immune status.
• Immune-related long non-coding RNAs could provide prognostic information and treatment guidance for AML patients.
What is the implication, and what should change now?
• This study conducted an assessment of immune status within the prognosis evaluation of AML by screening for immune predictors to improve the existing evaluation mechanism and allow enhanced treatment methods to be developed.
Introduction
Acute myeloid leukemia (AML) is a cancer arising from myeloid hematopoietic stem or progenitor cells (1). AML incidence increases with age and ranks the first in all adult acute leukemia types. In its pathogenesis, leukemia cells proliferate clonally and hematopoietic stem cells are prevented from differentiating, resulting in the disorder of normal hematopoiesis (2). Currently, the risk stratification of AML is mainly based on molecular biology and cytogenetic analyses adopted by the 2017 European LeukemiaNet (ELN) AML recommendations. According to this risk stratification, patients are treated with either chemotherapy alone, or chemotherapy combined with stem cell transplantation. Despite these treatment methods, the 5-year survival rates and median survival times AML patients are low and their prognosis is poor. Recent studies have found that AML cells interact with immune cells and cytokines in the immune microenvironment of the bone marrow, allowing leukemic cells to escape immune surveillance (3) and ultimately leading to immune drug resistance. With the development of single-cell RNA sequencing (scRNA-seq) technology, it was found that immune cells play a vital role in anti-tumor effects (4). The findings have suggested the close relation between the immune status and prognosis of patients with AML. However, the current mechanism of prognosis evaluation for AML is relatively simple and it does not involve the evaluation of the immune status. Therefore, this study assessed immune status within the prognosis evaluation of AML by screening for immune predictors to improve the existing evaluation mechanism and allow enhanced treatment methods to be developed.
A long non-coding RNA (lncRNA) does not code for a protein (5); thus, lncRNA was believed to be unrelated to gene expression. However, in the increasingly high-throughput sequencing era, more lncRNAs have been annotated, and some studies have reported that the lncRNA promoter sequences are even more conserved than protein-coding genes, indicating that lncRNA plays an important role in gene expression (6). Notably, a study (7) showed that lncRNAs were expressed in an aberrant manner and were found to be involved in gene transcription, RNA ligation, protein transport, and other processes in many neoplastic diseases (8). In AML, lncRNAs, such as HOTAIRMI, NEAT1, PVT1, CASC15, and UCA1, were found to regulate leukemic cell proliferation, differentiation, and apoptosis (9-14). Hence, the immune regulation of lncRNAs in cancer has become a research hotspot. Importantly, immune-related lncRNAs could provide prognostic information and treatment guidance for cancer patients (15).
In this study, three types of data, namely, transcriptome, high-throughput sequencing chip, and clinical data, were obtained from The Cancer Genome Atlas (TCGA) and Gene Expression Omnibus (GEO) databases and used to conduct a comprehensive analysis of AML immune-related lncRNAs. Based on the analysis results, a prognostic risk model was constructed to provide guidance for the prognostic assessment of patients as well as a theoretical basis for researching new therapeutic targets for AML. We present this article in accordance with the TRIPOD reporting checklist (available at https://tcr.amegroups.com/article/view/10.21037/tcr-23-429/rc).
Methods
Data collection and organization
The RNA-seq and clinical data of AML patients from the TCGA database were obtained using the UCSC Xena database (https://xena.ucsc.edu/). The microarray and clinical data were downloaded from the GEO repository; the GPL96 annotation platform had the largest sample size in the GPL96-GSE37642 dataset; thus, the GPL96-GSE37642 dataset was selected for analysis. Samples with a survival time of <30 days were excluded when screening for clinical data and the TCGA and GPL96-GSE37642 datasets were annotated using the “AnnoProbe” and “tinyarray” packages to distinguish messenger RNAs (mRNAs) and lncRNAs. A set of immune genes was obtained from ImmPort for analysis, the “limma” package was used to calculate the correlation between lncRNA and immune-related genes by co-expression analysis. Immune-related lncRNAs were obtained using the filtering coefficients corFilter =0.4 and pvalueFilter =0.001. The study was conducted in accordance with the Declaration of Helsinki (as revised in 2013).
Immune-related lncRNA risk model construction
The TCGA and GPL96-GSE37642 datasets were used in the training cohort and validation cohort, respectively. The batch effect between platforms was eliminated using the Combat method so that all data were at the same quantitative level. In the training cohort, using the “survival” package in R, univariate Cox regression analysis was used to detect immune-related lncRNAs involved in prognosis, using variables of P<0.05 as threshold. Further screening was performed using Cox regression analysis and the least absolute shrinkage and selection operator (LASSO) was used to reduce overfitting of the data, and the prognostic regression model was obtained to generate a risk score for each patient. In this study, the TCGA training cohort and GPL96-GSE37642 validation cohort were further classified into high- and low-risk groups as per the median risk score.
Statistical analysis
In the training cohort, Kaplan-Meier survival curves and the log-rank test were used for comparing survival differences between the high- and low-risk groups. The accuracy of the model was evaluated using the time-dependent receiver operating characteristic (timeROC) curves, risk heatmap, risk score distribution, and survival status, and validated using the validation cohort. Data from the training cohort were analyzed using principal component analysis (PCA) and linearly downscaled to demonstrate the model’s accuracy. Univariate and multivariate Cox regression analyses were used to assess the connection between risk score and clinical factors with the survival and prognosis of AML, and a forest plot was drawn to verify the model. Analysis of the correlation between lncRNAs of the training TCGA cohort and cytogenetic and clinico-pathological criteria was also performed. Gene set enrichment analysis (GSEA) was used to enrich the signaling pathways of the high- and low-risk groups of the training cohort. Statistical analyses were performed using R software (version 4.2; https://cran.r-project.org/), with P<0.05 considered a statistically significance.
Results
General information
Patients with clinical and prognostic information in the TCGA (n=130) were included as the training cohort, and those in the GPL96-GSE37642 (n=374) were included as the validation cohort. Data on the TCGA training cohort are shown in Table 1.
Table 1
Clinical features | Overall (n=130) |
---|---|
Age (years), median [range] | 55 [21–88] |
Male, n (%) | 69 (53.1) |
Cytogenetics, n (%) | |
Poor | 27 (20.8) |
Intermediate/normal | 73 (56.2) |
Favorable | 30 (23.1) |
FAB subtype, n (%) | |
M0 | 12 (9.2) |
M1 | 31 (23.8) |
M2 | 32 (24.6) |
M3 | 13 (10.0) |
M4 | 27 (20.8) |
M5 | 12 (9.2) |
M6 | 2 (1.5) |
M7 | 1 (0.8) |
FAB, French-American-British classification systems.
Screening of prognostic genes in TCGA
We performed co-expression analysis between the 4,241 lncRNAs obtained from TCGA and known immune gene sets downloaded from the ImmPort database (https://www.immport.org/shared/home) and obtained 1,991 immune-related lncRNAs. After intersecting these lncRNAs with the GPL96-GSE37642 dataset, we obtained 88 immune-related lncRNAs, with TCGA as the training cohort and GPL96-GSE37642 as the validation cohort. Subsequently, the “survival” package screened out 21 prognosis-related genes from the TCGA training cohort using Cox regression analysis (Figure 1A).
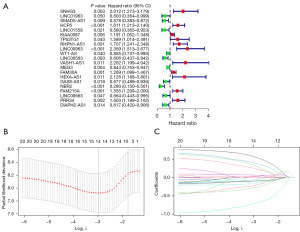
Establishment of the immune-related lncRNA risk model
We then performed LASSO regression on the 21 prognostic genes of the TCGA training cohort (Figure 1B,1C) and used the cross-validation method to output the optimal λ value. Based on this, we finally screened out 14 lncRNAs to build the risk model, generated the LASSO regression coefficient map, and output the risk score.
Risk score = SNHG3 × 0.570 − LINC01963 × 0.214 − SMAD5-AS1 × 0.099 + HCP5 × 0.399 + KIAA0087 × 0.065 + PHPN1-AS1 × 0.252 − WT1-AS × 0.055 − LINC00563 × 0.156 − MEG3 × 0.096 + FAM30A × 0.020 + HEXA-AS1 × 0.333 − NBR2 × 0.715 + FAM215A × 0.025 − DIAPH2-AS1 × 0.281.
All clinical cases were divided into the high- and low-risk groups as per the median risk score, and the predicted value was further verified using Kaplan-Meier and timeROC curves.
Assessment and validation of immune-related lncRNA risk models
The Kaplan-Meier curves demonstrated that the overall survival (OS) rate of the high-risk group was significantly lower than that of low-risk group, in both the TCGA training (P<0.05) and GPL96-GSE37642 validation cohorts (P<0.05) (Figure 2A,2B). According to the results of the survival status, distribution of the risk score, and risk heat map, we found that the patient mortality rate gradually increased with increase in the risk score. Importantly, we observed that both the difference in mortality rate and expression of genes between the high- and low-risk groups were statistically significant (P<0.05) (Figure 2C,2D). The area under the curve (AUC) values for the timeROC curves of the 1-, 3-, and 5-year survival of patients in the TCGA training cohort were 0.817, 0.859, and 0.909, respectively, indicating the effectiveness of the model in predicting patient survival time (Figure 2E). The AUC values of the GPL96-GSE37642 validation cohort were 0.603, 0.652, and 0.624 for 1-, 3-, and 5-year survival time, respectively, further supporting the good predictability of the model (Figure 2F).
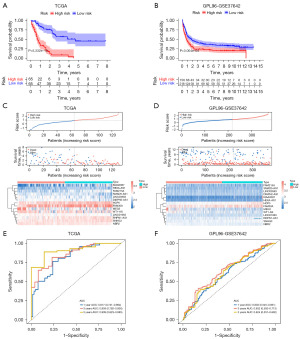
PCA
In performing PCA on the TCGA training cohort, we identified that 14 related lncRNAs effectively distinguished the low- from the high-risk group (Figure 3A). We also observed high overlaps of immune-related lncRNAs (Figure 3B) and immune genes (Figure 3C). In addition, we revealed that all genes in the low- and high-risk groups exhibited high overlap (Figure 3D).
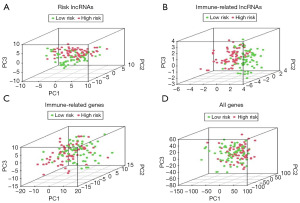
Multivariate and univariate analysis
In the TCGA training cohort, we subjected the risk score and cytogenetic stratification of patients, and clinico-pathological criteria, such as age, bone marrow blast cell number, leukocyte number, platelet number, and hemoglobin level, to univariate and multivariate independent prognostic analyses. Specifically, univariate Cox regression analyses revealed that age, risk score, and cytogenetic stratification were independent indicators for prognosis [hazard ratio (HR) =4.363; 95% confidence interval (CI): 3.024–6.296; P<0.001), whereas multivariate Cox regression revealed that the risk score was directly related to the prognosis of AML (HR =3.705; 95% CI: 2.488–5.518; P<0.001) regardless of other factors (Figure 4A,4B).
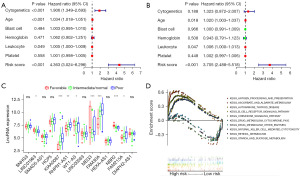
Clinical correlation analysis
In the TCGA training cohort, according to the 2017 ELN AML recommendations using cytogenetic indicators, patients were divided into three groups of prognosis: favorable, intermediate/normal, and poor. We analyzed the correlation between cytogenetic prognosis stratification and immune-related lncRNAs in this study. We detected that the genes KIAA0087, MEG3, FAM30A, NBR2, and RHPN1-AS1 were significantly correlated with cytogenetic indicators (P<0.001). Notably, the genes LINC01963, WT1-AS, and FAM215A were associated with prognosis (P<0.05), whereas the remaining lncRNAs were not associated with cytogenetic indicators (Figure 4C).
Enrichment analysis
In the TCGA training cohort, we identified that pathways related to immunity, including autoimmune diseases, processing and presentation of antigens, chemokines, B-cell receptor signaling pathways, and cytotoxicity mediated by natural killer (NK) cells, were primarily enriched in high-risk patients. Conversely, in low-risk patients, we mainly detected the enrichment of pathways such as drug metabolism (Figure 4D).
Discussion
In AML, myeloid precursors multiply clonally, making it an aggressive hematological malignancy (16). Interestingly, the heterogeneity of AML is also manifested in the immune microenvironment. It is particularly important to note that the effect of AML cells on the bone marrow immune microenvironment leads to drug resistance, relapses, and progression of disease (17). Currently, there is a lack of assessment of the AML immune status for predicting disease prognosis. Therefore, using the TCGA training cohort, we constructed the 14-immune-related-lncRNA model to predict AML prognosis. Patients with AML were divided into high- and low-risk groups as per the median risk score, and the Kaplan-Meier survival analysis, risk score maps, survival status maps, risk heat maps, and timeROC showed that the low-risk group had a significantly greater survival rate than the high-risk group, demonstrating the prognostic value of the risk score. A similar trend was observed among the GPL96-GSE37642 validation cohort risk group, indicating the performance of the model as stable and reliable. The risk heat map indicated that the model included the genes HCP5, FAM30A, PHPN1-AS1, KIAA0087, SNHG3, HEXA-AS1, and FAM215A as high-risk immune-related lncRNAs, whereas the genes SMAD5-AS1, LINC00566, MEG3, WT1-AS, LINC01963, NBR2, and DIAPH2-AS1 were identified as low-risk immune-related lncRNAs.
In the current study, we found that some lncRNAs used for constructing the model have rarely been reported in previous studies. Here, we identified HCP5, which has been linked to a number of cancers, and has been reported to exhibit elevated expression in AML, cholangiocarcinoma, esophageal cancer, and pancreatic cancer, potentially promoting cancer cell growth and metastasis (18). Similarly, SNHG3 has been mostly identified in solid tumors, which indicated poor prognosis (19). SMAD5-AS1, is a lncRNA involved in the regulation of B-lymphocytes (20), and its expression was downregulated in large B-cell lymphoma, promoting proliferation (21). WT1-AS binds to WT1 mRNA, regulating the expression of the WT1 protein through RNA-RNA interactions (22). Of note, the expression of WT1 was high in newly diagnosed patients with AML, whereas it decreased in patients after remission (23). MEG3 plays both oncogenic and tumor suppressor roles in AML, as it has been reported to be upregulated in acute promyelocytic leukemia, and often functions as a tumor suppressor in other myeloid leukemia cells (24). High LINC01963 expression levels negatively regulate miR-641 to prevent the progression of pancreatic cancer (25). Moreover, NBR2 was reported to inhibit tumorigenesis by controlling autophagy. Low expression of NBR2 in hepatocellular carcinomas has been found to significantly decrease OS (26,27); but this is unclear in AML. In conclusion, current studies of AML lack information on immune-related lncRNAs, which could provide new clues for exploring the pathogenesis of AML and could serve as potential targets for AML research.
In this study, the univariate and multifactorial Cox analyses were performed to study the relationship between risk scores, cytogenetic stratification, and clinico-pathological criteria, such as age, bone marrow blasts, leukocyte number, platelet number, and hemoglobin levels, for patients with AML. Interestingly, we determined that risk score could predict the prognosis of AML, further demonstrating that our model was capable of predicting AML prognosis. Moreover, the superiority of the model was demonstrated in three-dimensional space by PCA plots. Currently, AML is prognostically stratified using cytogenetics. Thus, we correlated the lncRNAs identified in this model with cytogenetics and found that the expression of WT1-AS, MEG3, and NBR2 was increased in patients with good prognosis, whereas that of KIAA0087, RHPN1-AS1, FAM30A, and FAM215A was increased in patients with poor prognosis, further supporting the good prognostic assessment performance of the model and demonstrating that it can serve as a good predictor for different subgroups, making the current prognostic evaluation system more comprehensive.
Finally, using GSEA, we were able to identify multiple pathways, mainly immune-related, in high-risk patients, including antigen processing, autoimmune disease, NK cell-mediated cytotoxicity, and chemokines. It is known that NK cells are mainly related to immune monitoring of tumor pathogenesis, and there were studies revealing that they mediated antibody-dependent cellular cytotoxicity (ADCC) and could resist leukemia (28,29). In addition, chemokines regulate immune cell migration and adhesion in the tumor immune microenvironment and promote the progression of AML (30).
Conclusions
We performed an immune-related lncRNA expression profile analysis using the TCGA and GPL96-GSE37642 datasets, with TCGA as the training cohort and GPL96-GSE37642 as the validation cohort and found that the immune-related lncRNA prognostic risk model of the training cohort effectively predicted the prognosis and performed the risk stratification of patients with AML, and the immune-related lncRNA prognostic risk model can thus be a biomarker of AML prognosis. The pathogenesis of AML is complex, and multi-target combination therapy may be required in the future. Overall, this study provides an innovative approach for determining AML pathogenesis with an improved risk stratification. However, the study has some limitations, including the small number of patients in the test cohort and the lack of a multi-center database validation. This study also lacks clinical samples experiments to verify the reliability of the risk model, and we will add clinical samples experiments in the further study. Therefore, elucidation of the mechanisms and pathways of immune-related lncRNAs in the current model require further investigation.
Acknowledgments
Funding: This study was supported by
Footnote
Reporting Checklist: The authors have completed the TRIPOD reporting checklist. Available at https://tcr.amegroups.com/article/view/10.21037/tcr-23-429/rc
Peer Review File: Available at https://tcr.amegroups.com/article/view/10.21037/tcr-23-429/prf
Conflicts of Interest: All authors have completed the ICMJE uniform disclosure form (available at https://tcr.amegroups.com/article/view/10.21037/tcr-23-429/coif). The authors have no conflicts of interest to declare.
Ethical Statement: The authors are accountable for all aspects of the work in ensuring that questions related to the accuracy or integrity of any part of the work are appropriately investigated and resolved. This study is a bioinformatics study based on an existing database and does not involve medical ethical review. The study was conducted in accordance with the Declaration of Helsinki (as revised in 2013).
Open Access Statement: This is an Open Access article distributed in accordance with the Creative Commons Attribution-NonCommercial-NoDerivs 4.0 International License (CC BY-NC-ND 4.0), which permits the non-commercial replication and distribution of the article with the strict proviso that no changes or edits are made and the original work is properly cited (including links to both the formal publication through the relevant DOI and the license). See: https://creativecommons.org/licenses/by-nc-nd/4.0/.
References
- Creutzig U, Kutny MA, Barr R, et al. Acute myelogenous leukemia in adolescents and young adults. Pediatr Blood Cancer 2018;65:e27089. [Crossref] [PubMed]
- Cai SF, Levine RL. Genetic and epigenetic determinants of AML pathogenesis. Semin Hematol 2019;56:84-9. [Crossref] [PubMed]
- Epperly R, Gottschalk S, Velasquez MP. A Bump in the Road: How the Hostile AML Microenvironment Affects CAR T Cell Therapy. Front Oncol 2020;10:262. [Crossref] [PubMed]
- Guo R, Lü M, Cao F, et al. Single-cell map of diverse immune phenotypes in the acute myeloid leukemia microenvironment. Biomark Res 2021;9:15. [Crossref] [PubMed]
- Mendell JT, Sharifi NA, Meyers JL, et al. Nonsense surveillance regulates expression of diverse classes of mammalian transcripts and mutes genomic noise. Nat Genet 2004;36:1073-8. [Crossref] [PubMed]
- Mumtaz PT, Bhat SA, Ahmad SM, et al. LncRNAs and immunity: watchdogs for host pathogen interactions. Biol Proced Online 2017;19:3. [Crossref] [PubMed]
- Zhu H, Lv Z, An C, et al. Onco-lncRNA HOTAIR and its functional genetic variants in papillary thyroid carcinoma. Sci Rep 2016;6:31969. [Crossref] [PubMed]
- Mercer TR, Dinger ME, Mattick JS. Long non-coding RNAs: insights into functions. Nat Rev Genet 2009;10:155-9. [Crossref] [PubMed]
- Zhang X, Weissman SM, Newburger PE. Long intergenic non-coding RNA HOTAIRM1 regulates cell cycle progression during myeloid maturation in NB4 human promyelocytic leukemia cells. RNA Biol 2014;11:777-87. [Crossref] [PubMed]
- Zeng C, Xu Y, Xu L, et al. Inhibition of long non-coding RNA NEAT1 impairs myeloid differentiation in acute promyelocytic leukemia cells. BMC Cancer 2014;14:693. [Crossref] [PubMed]
- Tseng YY, Moriarity BS, Gong W, et al. PVT1 dependence in cancer with MYC copy-number increase. Nature 2014;512:82-6. [Crossref] [PubMed]
- Salehi M, Sharifi M. Induction of apoptosis and necrosis in human acute erythroleukemia cells by inhibition of long non-coding RNA PVT1. Mol Biol Res Commun 2018;7:89-96. [PubMed]
- Fernando TR, Contreras JR, Zampini M, et al. The lncRNA CASC15 regulates SOX4 expression in RUNX1-rearranged acute leukemia. Mol Cancer 2017;16:126. [Crossref] [PubMed]
- Hughes JM, Legnini I, Salvatori B, et al. C/EBPα-p30 protein induces expression of the oncogenic long non-coding RNA UCA1 in acute myeloid leukemia. Oncotarget 2015;6:18534-44. [Crossref] [PubMed]
- Li Y, Jiang T, Zhou W, et al. Pan-cancer characterization of immune-related lncRNAs identifies potential oncogenic biomarkers. Nat Commun 2020;11:1000. [Crossref] [PubMed]
- Döhner H, Weisdorf DJ, Bloomfield CD. Acute Myeloid Leukemia. N Engl J Med 2015;373:1136-52. [Crossref] [PubMed]
- Binnewies M, Roberts EW, Kersten K, et al. Understanding the tumor immune microenvironment (TIME) for effective therapy. Nat Med 2018;24:541-50. [Crossref] [PubMed]
- Hu SP, Ge MX, Gao L, et al. LncRNA HCP5 as a potential therapeutic target and prognostic biomarker for various cancers: a meta-analysis and bioinformatics analysis. Cancer Cell Int 2021;21:686. [Crossref] [PubMed]
- Shan DD, Zheng QX, Wang J, et al. Small nucleolar RNA host gene 3 functions as a novel biomarker in liver cancer and other tumour progression. World J Gastroenterol 2022;28:1641-55. [Crossref] [PubMed]
- Ghafouri-Fard S, Khoshbakht T, Hussen BM, et al. The emerging role non-coding RNAs in B cell-related disorders. Cancer Cell Int 2022;22:91. [Crossref] [PubMed]
- Zhao CC, Jiao Y, Zhang YY, et al. Lnc SMAD5-AS1 as ceRNA inhibit proliferation of diffuse large B cell lymphoma via Wnt/β-catenin pathway by sponging miR-135b-5p to elevate expression of APC. Cell Death Dis 2019;10:252. [Crossref] [PubMed]
- Zhang Y, Fan LJ, Zhang Y, et al. Long Non-coding Wilms Tumor 1 Antisense RNA in the Development and Progression of Malignant Tumors. Front Oncol 2020;10:35. [Crossref] [PubMed]
- Østergaard M, Olesen LH, Hasle H, et al. WT1 gene expression: an excellent tool for monitoring minimal residual disease in 70% of acute myeloid leukaemia patients - results from a single-centre study. Br J Haematol 2004;125:590-600. [Crossref] [PubMed]
- Zimta AA, Tomuleasa C, Sahnoune I, et al. Long Non-coding RNAs in Myeloid Malignancies. Front Oncol 2019;9:1048. [Crossref] [PubMed]
- Ghafouri-Fard S, Fathi M, Zhai T, et al. LncRNAs: Novel Biomarkers for Pancreatic Cancer. Biomolecules 2021;11:1665. [Crossref] [PubMed]
- Wang T, Li Z, Yan L, et al. Long Non-Coding RNA Neighbor of BRCA1 Gene 2: A Crucial Regulator in Cancer Biology. Front Oncol 2021;11:783526. [Crossref] [PubMed]
- Sheng JQ, Wang MR, Fang D, et al. LncRNA NBR2 inhibits tumorigenesis by regulating autophagy in hepatocellular carcinoma. Biomed Pharmacother 2021;133:111023. [Crossref] [PubMed]
- Xu J, Niu T. Natural killer cell-based immunotherapy for acute myeloid leukemia. J Hematol Oncol 2020;13:167. [Crossref] [PubMed]
- Mastaglio S, Wong E, Perera T, et al. Natural killer receptor ligand expression on acute myeloid leukemia impacts survival and relapse after chemotherapy. Blood Adv 2018;2:335-46. [Crossref] [PubMed]
- Hino C, Pham B, Park D, et al. Targeting the Tumor Microenvironment in Acute Myeloid Leukemia: The Future of Immunotherapy and Natural Products. Biomedicines 2022;10:1410. [Crossref] [PubMed]