Novel cuproptosis-related lncRNAs can predict the prognosis of patients with multiple myeloma
Highlight box
Key findings
• A prognostic model consisting of twelve cuproptosis-related long-stranded non-coding RNAs (lncRNAs) was a strong predictor of multiple myeloma (MM).
What is known and what is new?
• Cuproptosis-related lncRNAs play a key role in MM development and metastasis.
• Cuproptosis-related lncRNAs were used to construct a prognostic model, and the new model was found to be associated with the prognosis of MM.
What is the implication, and what should change now?
• This study highlights the importance of cuproptosis-related lncRNAs in predicting prognosis in MM.
Introduction
Multiple myeloma (MM) is a hematological malignant tumor caused by abnormal proliferation of plasma cells in the bone marrow. The most common manifestations of MM include hypercalcemia (1,2), renal failure (3,4), anemia (5,6), and lytic bone lesions (7,8), which mainly affect the elderly population (9). The high-dose therapy (HDT), autologous stem cell transplantation (ASCT), and the emergence of new drugs (such as bortezomib and thalidomide) have improved the survival rate of MM patients (10). However, almost all MM patients would suffer a relapse (11-13).
As a basic nutrient, copper can exert both beneficial and toxic effects on cells due to its redox properties (14,15). Intracellular copper concentration is maintained at a very low level through an active steady-state working mechanism across concentration gradients (16). Different from the known programmed cell death (such as ferroptosis and apoptosis), copper ionophore-induced cell death is closely related to mitochondrial respiration and protein lipoylation (17-19). In this process, copper binds directly to the lipoylated components of the tricarboxylic acid (TCA) cycle, which leads to the accumulation of lipoylated proteins and the subsequent loss of iron-sulfur cluster proteins. This induces protein toxic stress and eventually leads to cell death (20). In addition, it was demonstrated in a recent study that high anti-MM efficacy was showed in treatment-resistant cellular models of MM patients, when using copper ionophores (21).
Long-stranded non-coding RNAs (lncRNAs) is a group of RNA molecules with a transcriptional length more than 200 nT. They are initially considered to be false transcriptional noise caused by low RNA polymerase fidelity (22). The promoter region of lncRNAs is generally more conservative than that of messenger RNAs (mRNAs). Different from mRNAs, lncRNAs do not encode proteins. However, lncRNAs can regulate gene expression in the form of RNA at multiple epigenetic, transcriptional and post-transcriptional levels (23). LncRNAs can induce many important cancer phenotypes by interacting with other cellular macromolecules, DNAs, proteins, and RNAs (24). Some lncRNAs have been shown to regulate apoptosis (25,26), ferroptosis (27-29), and cuproptosis (30,31) of cancer cells. Furthermore, the expression of lncRNAs usually changes and is related to the prognosis of various cancer patients. Mounting evidence supports the independent prognostic value of lncRNAs in patients with MM, leading to the development of multiple prognostic lncRNA signatures (32,33).
LncRNAs participate in tumor progression by regulating cuproptosis genes. Numerous studies have focused on molecular classification and survival prediction using lncRNAs. One study (34) constructed a cuproptosis-related lncRNAs prognosis prediction model, which can reliably and accurately predict the prognosis, drug sensitivity, and clinical recurrence of acute myeloid leukaemia. However, there is no research on the relationship between the prognosis and score in MM based on cuproptosis-related lncRNAs, and whether it can be used as an independent prognostic factor is not clear. Therefore, we analyzed the data with a view to exploring cuproptosis-related lncRNAs biomarkers to predict the prognosis of MM patients.
In this study, a bioinformatics analysis method was used to construct a prognostic risk model for MM patients based on the cuproptosis-related lncRNA score. Moreover, the differential risk analysis and functional enrichment analysis were also conducted to identify the potential signaling pathways in the risk group. These findings are expected to provide some theoretical support for exploring the regulation of cuproptosis-related lncRNAs in MM. The flow chart of this research is sketched in Figure 1. We present this article in accordance with the TRIPOD reporting checklist (available at https://tcr.amegroups.com/article/view/10.21037/tcr-23-960/rc).
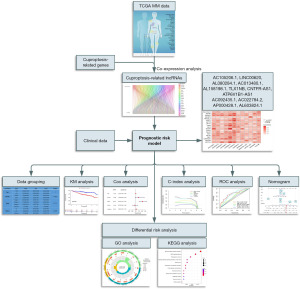
Methods
Data acquisition and preprocessing
In order to identify cuproptosis-related lncRNAs that can be employed to predict the prognosis of MM patients, this study was conducted based on the following four points.
- The expression profile was obtained by array or high-throughput sequencing.
- Data samples were collected from MM patients diagnosed for the first time.
- More than 300 cases were included to constitute a meaningful sample size.
- The data were the count files obtained by RNA sequencing (RNA-Seq) in gene expression quantification.
The expression profile data and clinical data of MM (MMRF-COMMPASS) were downloaded from the Cancer Genome Atlas (TCGA) database (https://portal.gdc.cancer.gov/), and then lncRNAs were identified according to the gene annotation of TCGA. The study was conducted in accordance with the Declaration of Helsinki (as revised in 2013).
Cuproptosis-related genes were collected from a series of recent reports (35-38), including NFE2L2, NLRP3, ATP7A, ATP7B, SLC31A1, FDX1, LIAS, LIPT1, LIPT2, DLD, DLAT, PDHA1, PDHB, MTF1, GLS, CDKN2A, DBT, GCSH, and DLST. Combined with the standardized gene expression matrix, the cuproptosis-related gene expression matrix was obtained. Finally, the potential cuproptosis-related lncRNAs were identified based on the co-expression analysis of the cuproptosis-related genes expression matrix and the lncRNA expression matrix with the aid of the limma package in R software, with a correlation coefficient larger than 0.3 and P value less than 0.001 as screening conditions.
Construction of a prognostic risk model by the least absolute shrinkage and selection operator (LASSO) regression
First of all, the sample data of MM patients were randomly divided into the training group and the testing group. Then, cuproptosis-related lncRNAs were screened out by the univariate LASSO regression in the glmnet package in R software (P<0.05). After redundant genes were removed, the remaining cuproptosis-related lncRNAs were used to construct a prognostic risk model. The risk score of each patient was identified according to the expression of the 12 cuproptosis-related lncRNAs and the risk coefficient. The risk score was calculated as follows: (0.370160265859127 × AC105206.1) + (0.430732849678822 × LINC00620) + (0.932090945670286 × AL080284.1) + (0.735909366869273 × AC013480.1) + (0.639619158470217 × AL158198.1) + (0.40164431674857 × TLX1NB) + (−2.18823271531587 × CNTFR-AS1) + (−0.2783376598488 × ATP6V1B1-AS1) + (0.398459050292138 × AC092435.1) + (0.313821766142159 × AC022784.2) + (0.317809597431966 × AP000428.1) + (0.395740225449718 × AL603824.1).
Subsequently, a multivariate Cox model was constructed with these selected cuproptosis-related lncRNAs related for the prognosis and survival prediction. Besides, the risk scores of all MM patients were calculated. These patients were divided into the low- and high-risk groups according to the median value of risk scores for prognosis assessment. Furthermore, the Kaplan-Meier survival curves of MM patients in the low- and high-risk groups of the training group and testing group were plotted.
Independent prognostic analysis of the prognostic risk model
The univariate and multivariate Cox analyses were performed to evaluate the effects of risk score, age, gender, International Staging System (ISS) stage, and family tumor history on the prognosis of MM patients. And the variable in the Cox model has respected the hazards proportionality. Then a forest map was plotted. In order to verify the accuracy of this model in predicting the prognosis and survival of MM patients, the survival and timeROC packages in R software were used to calculate the factors of the risk score model and the 1-, 3-, and 5-year area under the curve (AUC). Besides, the C-index curve was constructed.
Construction of nomogram of clinical subgroups
The survival, regplot and rms packages in R software were used to construct a nomogram of clinical subgroups to identify the 1-, 3-, and 5-year survival rates of these MM patients. The nomogram of clinical subgroups was constructed based on the risk score, age, gender, ISS stage, and family history of these MM patients.
Differential risk analysis
With the aid of the limma package in R software, the differentially expressed risk-related genes (DERGs) in the samples of MM patients in the low- and high-risk groups were screened under the condition of |log2FC| >1 and P<0.05.
Functional enrichment analysis
The functional enrichment analysis of DERGs was carried out by GO and KEGG through the clusterProfiler package in R software. The results were screened according to corrected P (adj.P)<0.01 to identify the potential signaling pathways in the risk group.
Statistical analysis
The R software is used for data analysis and graphics rendering. The survival curve was drawn using R software package “survival”, and the difference in prognosis between groups was analyzed using survival function in the R software package. Receiver operating characteristic (ROC) analysis was performed using the R software package “timeROC” to obtain the AUC. Nomograms were established using the R the software package “rms” to assess the prognostic significance of some of the features in the samples. P<0.05 indicated that the difference was statistically significant.
Results
Cuproptosis-related lncRNA data acquisition
The bone marrow tissue transcriptome data of 575 MM patients and the clinical data of 516 MM patients were downloaded from the TCGA database (https://portal.gdc.cancer.gov/). Based on the Gencode Database, 16,901 lncRNAs were selected from the TCGA Multiple Myeloma Database. Then, the matrix expression of lncRNAs and cuproptosis-related genes was analyzed under the screening conditions of a correlation coefficient larger than 0.3 and P value less than 0.001. Finally, a total of 294 cuproptosis-related lncRNAs were screened out. The co-expression relationship between cuproptosis-related genes and cuproptosis-related lncRNAs was visualized by the Sankey diagram (Figure 2).
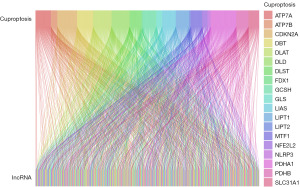
Construction of prognostic risk model for MM patients
Firstly, due to lack of related data such as the initial treatment regimen, treatment response, and status of stem cell transplantation, the clinical data of 516 MM patients were randomly divided into the training group and the testing group (Table 1). Besides, 294 cuproptosis-related lncRNAs were screened by the univariate Cox analysis (P<0.05), and a total of 76 cuproptosis-related lncRNAs were found to be associated with the survival of MM patients. Additionally, 12 independent prognostic cuproptosis-related lncRNAs were screened according to multivariate Cox analysis.
Table 1
Covariates | Type | Total | Test | Train | P value |
---|---|---|---|---|---|
Age (years) | ≤65 | 264 (51.16) | 132 (51.16) | 132 (51.16) | >0.99 |
>65 | 252 (48.84) | 126 (48.84) | 126 (48.84) | ||
Gender | Female | 201 (38.95) | 97 (37.6) | 104 (40.31) | 0.5881 |
Male | 315 (61.05) | 161 (62.4) | 154 (59.69) | ||
Stage | Stage I | 165 (31.98) | 87 (33.72) | 78 (30.23) | 0.1751 |
Stage II | 175 (33.91) | 77 (29.84) | 98 (37.98) | ||
Stage III | 159 (30.81) | 87 (33.72) | 72 (27.91) | ||
Unknown | 17 (3.29) | 7 (2.71) | 10 (3.88) | ||
History | No | 177 (34.3) | 85 (32.95) | 92 (35.66) | 0.5685 |
Yes | 242 (46.9) | 127 (49.22) | 115 (44.57) | ||
Unknown | 97 (18.8) | 46 (17.83) | 51 (19.77) |
Data are presented in n (%).
The relationship between cuproptosis-related genes and lncRNAs was presented in a heatmap (Figure 3). According to the median risk score, patients with the risk score lower than the median were classified as the low-risk group, and those with the risk score higher than the median were classified as the high-risk group. It was found that in the training group, the testing group, and all groups, the overall survival (OS) of patients in the high-risk group was shorter than that in the low-risk group (Figure 4A-4C).
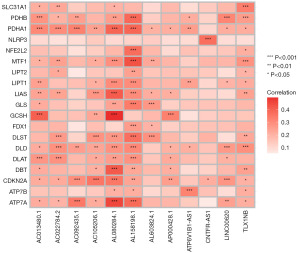
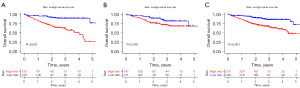
Independent prognostic analysis of factors and evaluation of the accuracy of the prognostic model
The univariate and multivariate Cox regression analyses can be employed to identify whether the risk score calculated can be used as an independent prognostic factor. Due to the lack of related data of the serum lactate dehydrogenase (LDH) level and high-risk cytogenetics, we constructed the univariate and multivariate Cox regression analysis with age, gender, ISS stage, tumor family history and risk score. The univariate Cox analysis results showed that the age [hazard ratio (HR) =1.039, 95% CI: 1.017–1.062, P<0.001], stage (HR =2.092, 95% CI: 1.587–2.757, P<0.001), and risk score (HR =1.073, 95% CI: 1.038–1.110, P<0.001) of MM patients were correlated with the OS of MM patients (Figure 5A). The multivariate Cox analysis results showed that the age (HR =1.027, 95% CI: 1.005–1.049, P=0.018), stage (HR =2.053, 95% CI: 1.541–2.735, P<0.001), and risk score (HR =1.098, 95% CI: 1.059–1.139, P<0.001) were independently correlated with the OS of these patients (Figure 5B). This finding suggested that these prognostic factors are independent in MM patients. Then, the ROC curve was employed to evaluate the prediction accuracy of the risk score. On the one hand, the AUC of risk score was 0.732, which was larger than that of age (0.571), gender (0.582), stage (0.703), and tumor family history (0.535) (Figure 6A). On the other hand, the AUC of 1-, 3-, and 5-year OS was 0.732, 0.705, and 0.778, respectively, which confirmed the favorable diagnostic significance of this prognostic model (Figure 6B). In addition, a C-index curve was also constructed to compare the consistency index of risk score with other clinical features (age, gender, stage, and tumor family history). It was revealed that the C-index value of the risk score was larger than that of other clinical features (Figure 6C), which verified the high prediction accuracy of this model.
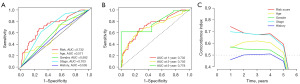
Construction of nomogram of clinical subgroups
Moreover, a nomogram was constructed based on the age, gender, ISS stage, risk score, and tumor family history from the signature (Figure 7) to predict the OS of patients. The total score calculation results demonstrated that the nomogram was effective in predicting the 1-, 3- and 5-year OS of MM patients. As the example shown in the Figure 7, the patient is a male and the age is 74 with a tumor family history. The risk score is low and the ISS stage is level 3. The total score calculation is 259, and the probability of living more than 1 year is 0.914. The probability of living more than 3 years is 0.726, while the probability of living more than 5 years is 0.475.
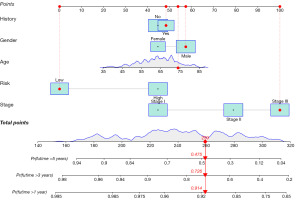
Differential risk analysis and functional enrichment analysis
The DERGs were extracted from the samples of the high- and low-risk groups by the limma package in R software. The results demonstrated that there were significant differences in the expression of 581 genes in MM patients between the high- and low-risk groups (|log2FC| >1, P<0.05). The functional enrichment analysis was also performed on 581 DERGs. The GO analysis results showed that these DERGs were mainly concentrated on such ontology annotations as extracellular structure organization, collagen-containing extracellular matrix, glycosaminoglycan binding, and sulfur compound binding (Figure 8, Table 2). The KEGG analysis showed that the DERGs may be related to neuroactive ligand-receptor interaction and mitogen-activated protein kinase (MAPK) signaling pathway. This finding indicated that these DERGs may be involved in the progression of tumors (Figure 9).
Table 2
Ontology | ID | Description | P.adjust | q value | Count |
---|---|---|---|---|---|
BP | GO: 0030198 | Extracellular matrix organization | 0.001479447 | 0.001330466 | 19 |
GO: 0043062 | Extracellular structure organization | 0.001479447 | 0.001330466 | 19 | |
GO: 0045229 | External encapsulating structure organization | 0.001479447 | 0.001330466 | 19 | |
GO: 0003007 | Heart morphogenesis | 0.000714112 | 0.000642201 | 18 | |
GO: 0003206 | Cardiac chamber morphogenesis | 0.000714112 | 0.000642201 | 13 | |
GO: 0003205 | Cardiac chamber development | 0.003280404 | 0.002950066 | 13 | |
CC | GO: 0062023 | Collagen-containing extracellular matrix | 6.91E–05 | 6.09E–05 | 25 |
GO: 0005911 | Cell-cell junction | 0.009584801 | 0.008453167 | 22 | |
GO: 0098982 | GABA-ergic synapse | 0.005135118 | 0.004528838 | 8 | |
MF | GO: 0005539 | Glycosaminoglycan binding | 6.63E–05 | 5.76E–05 | 18 |
GO: 1901681 | Sulfur compound binding | 0.000203826 | 0.000177183 | 18 | |
GO: 0008201 | Heparin binding | 6.63E–05 | 5.76E–05 | 15 | |
GO: 0003774 | Cytoskeletal motor activity | 0.003421737 | 0.002974461 | 10 | |
GO: 0098960 | Postsynaptic neurotransmitter receptor activity | 0.00879237 | 0.007643065 | 7 | |
GO: 0016917 | GABA receptor activity | 0.003421737 | 0.002974461 | 5 |
BP, biological process; GO, Gene Ontology; CC, cellular component; MF, molecular function; GABA, gamma-aminobutyric acid.
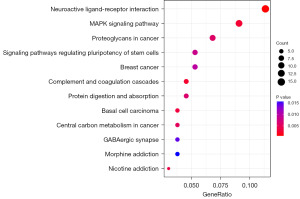
Discussion
MM is the second most common hematological malignant tumor in adults, and its incidence is on the rise worldwide (39,40). Despite the advancement in the treatment of MM with the emergence of several new drugs (carfilzomib, pomalidomide, daratumumab, elotuzumab, panobinostat, ixazomib, and Selinexor), this cancer is still incurable in most patients (41). There is an urgent demand for identifying reliable prognostic biomarkers and new therapeutic targets for MM. In recent years, the contribution of lncRNAs to cancer progression has been widely recognized (42,43). Li (44) found that cuproptosis played an important role in hematological tumors. However, there are few studies on the co-regulatory role of cuproptosis and lncRNAs in MM. Hence, exploring the biomarkers related to the prognosis of MM may have a favorable prospect in clinical application. In this study, a prognostic risk model was established for MM patients with cuproptosis-related lncRNAs.
With the assistance of bioinformatics analysis, the bone marrow tissue transcriptome data of 575 MM patients and clinical data of 516 MM patients were screened from the TCGA database. After a series of cuproptosis-related genes were obtained from recent reports, cuproptosis-related lncRNAs were selected by the co-expression analysis of cuproptosis-related genes and lncRNAs. Then, 12 cuproptosis-related lncRNAs were screened by the LASSO Cox regression analysis, including AC105206, LINC00620, AL080284.1, AC013480.1, AL158198.1, TLX1NB, CNTFR-AS1, ATP6V1B1-AS, AC092435.1, AC022784.2, AP000428, and AL603824.1. Subsequently, a prognostic risk model was constructed for MM patients. The multivariate Cox regression analysis results showed that age, stage, and risk score were independently correlated with the OS of MM patients and hence could be used as independent prognostic biomarkers. Besides, the clinical data of MM patients were randomly divided into the training group and the testing group, and these patients were divided into the low- and high-risk groups based on the median risk score. The Kaplan-Meler survival analysis results showed that there was a significant difference in the survival rate between the high- and low-risk groups (P<0.001). In addition, the ROC curve and C-index curve analysis results proved the effectiveness of risk score as a prognostic biomarker. Finally, the difference risk analysis and function enrichment analysis were carried out among risk groups.
Among the 12 cuproptosis-related lncRNA genes associated with the prognosis of MM, TLX1NB and CNTFR-AS1 were verified to play a role in this cancer. TLX1NB is an overexpressed oncogene, and it is related to the tumor progression of lung cancer, colorectal cancer, and glioma. Duan et al. (45) found the low expression and hypermethylation of TLX1NB in patients with low-grade gliomas, and TLX1NB had a certain impact on the prognosis of low-grade gliomas. Further, they concluded that TLX1NB may be an early biomarker for the recurrence of low-grade gliomas. Chen et al. (46) found that the expression of TLX1NB was up-regulated in colon cancer tissue. They confirmed that TLX1NB can promote the invasion, migration, and metastasis of colon cancer cells by promoting the phosphorylation of STAT5A and it also played an important role in regulating cancer. Dastjerdi et al. (47) revealed that TLX1NB was overexpressed in colon cancer. It had potential carcinogenic characteristics and can be used as a diagnostic factor to detect tumors in normal samples. In a study of Li et al. (48), it was suggested that TLX1NB regulated CRISP1 through hsa-miR-148b-3p and can be considered a potential therapeutic target for lung adenocarcinoma. In addition, Li et al. (49) also found that the high expression of CNTFR-AS1 was related to the low survival rate of triple negative breast cancer, and it may be used as a potential biomarker for the treatment and prognostic classification of different breast cancer subtypes.
In order to clarify the potential regulatory mechanism among different risk groups, the functional enrichment analysis was performed on 581 DERGs. According to the GO analysis results, risk factors seemed to be closely related to the extracellular matrix, which was a key component in the tumor microenvironment for the regulation of cell growth and development and contributed to the transmission of cell signals. Therefore, it can be speculated that cuproptosis-related lncRNAs may play a key role in the tumor microenvironment of MM. The KEGG analysis results indicated that differentially expressed cuproptosis-related lncRNAs were highly enriched in the MAPK signaling pathway. There are five MAPK signaling pathways in mammals, which play an important role in tumor proliferation, apoptosis, invasion, and metastasis and participate in the occurrence and development of many kinds of tumors, including MM (50). It was demonstrated in a previous study that the activation of the MAPK pathway mediated the proliferation, survival, and migration of MM cells (51). Up to 50% of patients newly diagnosed with MM are affected by the abnormal MAPK pathway. Zhang et al. (52) found that inhibiting the MAPK pathway can reduce the proliferation and migration of colon cancer cells. In this study, the KEGG analysis results demonstrated that the differentially expressed cuproptosis-related lncRNAs were highly enriched in the signaling pathway of neuroactive ligand-receptor interaction. This indicated that cuproptosis-related lncRNAs may be involved in the signaling pathway of neuroactive ligand-receptor interaction. Neuroactive ligands have been verified to affect neuronal functions by binding intracellular receptors and can bind transcription factors and regulate gene expression (53,54).
To sum up, some bioinformatics methods combined with the TCGA database were adopted in this study to screen cuproptosis-related lncRNAs. The results demonstrated that TLX1NB and CNTFR-AS1 could regulate the prognosis of MM patients. Nevertheless, there are some limitations in this study. The related research on cuproptosis-related genes is still in the early stage, this paper only applied the cuproptosis-related genes reported at present, and more cuproptosis-related genes may be found in the future. There was a significant difference in gene expression between the high- and low-risk group. GO analysis showed that risk characteristics were closely related to the extracellular matrix. The KEGG analysis results showed that cuproptosis-related lncRNAs were highly enriched in the MAPK pathway and neuroactive ligand-receptor interaction signaling pathway. In this study, however, relevant analyses were only performed from the perspective of statistics, and basic experiments were not conducted. The findings of this study provided an important basis and direction for follow-up basic experimental and clinical studies.
Conclusions
In this study, a novel cuproptosis-related lncRNA prognostic model was constructed for MM patients. TLX1NB and CNTFR-AS1 may be cuproptosis-related lncRNAs associated with the prognosis of MM patients. Besides, the differentially expressed risk genes between the high- and low-risk groups were also analyzed, and the GO and KEGG analyses were also carried out. These findings are anticipated to contribute to the improvement of clinical outcomes. However, further verification is still needed.
Acknowledgments
Funding: This work was supported by the Health Commission of Chongqing & Science and Technology Bureau of Chongqing (No. 2021MSXM220).
Footnote
Reporting Checklist: The authors have completed the TRIPOD reporting checklist. Available at https://tcr.amegroups.com/article/view/10.21037/tcr-23-960/rc
Peer Review File: Available at https://tcr.amegroups.com/article/view/10.21037/tcr-23-960/prf
Conflicts of Interest: All authors have completed the ICMJE uniform disclosure form (available at https://tcr.amegroups.com/article/view/10.21037/tcr-23-960/coif). The authors have no conflicts of interest to declare.
Ethical Statement: The authors are accountable for all aspects of the work in ensuring that questions related to the accuracy or integrity of any part of the work are appropriately investigated and resolved. The study was conducted in accordance with the Declaration of Helsinki (as revised in 2013).
Open Access Statement: This is an Open Access article distributed in accordance with the Creative Commons Attribution-NonCommercial-NoDerivs 4.0 International License (CC BY-NC-ND 4.0), which permits the non-commercial replication and distribution of the article with the strict proviso that no changes or edits are made and the original work is properly cited (including links to both the formal publication through the relevant DOI and the license). See: https://creativecommons.org/licenses/by-nc-nd/4.0/.
References
- Samart P, Luanpitpong S, Rojanasakul Y, et al. O-GlcNAcylation homeostasis controlled by calcium influx channels regulates multiple myeloma dissemination. J Exp Clin Cancer Res 2021;40:100. [Crossref] [PubMed]
- Bansal R, Rakshit S, Kumar S. Extramedullary disease in multiple myeloma. Blood Cancer J 2021;11:161. [Crossref] [PubMed]
- Dimopoulos MA, Leleu X, Moreau P, et al. Isatuximab plus pomalidomide and dexamethasone in relapsed/refractory multiple myeloma patients with renal impairment: ICARIA-MM subgroup analysis. Leukemia 2021;35:562-72. [Crossref] [PubMed]
- Capra M, Martin T, Moreau P, et al. Isatuximab plus carfilzomib and dexamethasone versus carfilzomib and dexamethasone in relapsed multiple myeloma patients with renal impairment: IKEMA subgroup analysis. Haematologica 2022;107:1397-409. [Crossref] [PubMed]
- Zhong W, Zhang X, Zhao M, et al. Advancements in nanotechnology for the diagnosis and treatment of multiple myeloma. Biomater Sci 2020;8:4692-711. [Crossref] [PubMed]
- Kahale LA, Matar CF, Tsolakian I, et al. Antithrombotic therapy for ambulatory patients with multiple myeloma receiving immunomodulatory agents. Cochrane Database Syst Rev 2021;9:CD014739. [PubMed]
- Diaz-delCastillo M, Chantry AD, Lawson MA, et al. Multiple myeloma-A painful disease of the bone marrow. Semin Cell Dev Biol 2021;112:49-58. [Crossref] [PubMed]
- Terpos E, Ntanasis-Stathopoulos I, Christoulas D, et al. Semaphorin 4D correlates with increased bone resorption, hypercalcemia, and disease stage in newly diagnosed patients with multiple myeloma. Blood Cancer J 2018;8:42. [Crossref] [PubMed]
- Hu J, Hu WX. Targeting signaling pathways in multiple myeloma: Pathogenesis and implication for treatments. Cancer Lett 2018;414:214-21. [Crossref] [PubMed]
- Rajkumar SV, Kumar S. Multiple myeloma current treatment algorithms. Blood Cancer J 2020;10:94. [Crossref] [PubMed]
- Munshi NC, Anderson LD Jr, Shah N, et al. Idecabtagene Vicleucel in Relapsed and Refractory Multiple Myeloma. N Engl J Med 2021;384:705-16. [Crossref] [PubMed]
- Rodriguez-Otero P, Paiva B, San-Miguel JF. Roadmap to cure multiple myeloma. Cancer Treat Rev 2021;100:102284. [Crossref] [PubMed]
- Chim CS, Kumar SK, Orlowski RZ, et al. Management of relapsed and refractory multiple myeloma: novel agents, antibodies, immunotherapies and beyond. Leukemia 2018;32:252-62. [Crossref] [PubMed]
- Liu X, Cheng W, Li H, et al. Identification and validation of cuproptosis-related LncRNA signatures as a novel prognostic model for head and neck squamous cell cancer. Cancer Cell Int 2022;22:345. [Crossref] [PubMed]
- Jiang Z, Sha G, Zhang W, et al. The huge potential of targeting copper status in the treatment of colorectal cancer. Clin Transl Oncol 2023;25:1977-90. [Crossref] [PubMed]
- Lin Z, Song J, Gao Y, et al. Hypoxia-induced HIF-1α/lncRNA-PMAN inhibits ferroptosis by promoting the cytoplasmic translocation of ELAVL1 in peritoneal dissemination from gastric cancer. Redox Biol 2022;52:102312. [Crossref] [PubMed]
- Cui G, Liu J, Wang C, et al. Comprehensive analysis of the prognostic signature and tumor microenvironment infiltration characteristics of cuproptosis-related lncRNAs for patients with colon adenocarcinoma. Front Oncol 2022;12:1007918. [Crossref] [PubMed]
- Tsvetkov P, Coy S, Petrova B, et al. Copper induces cell death by targeting lipoylated TCA cycle proteins. Science 2022;375:1254-61. [Crossref] [PubMed]
- Ge EJ, Bush AI, Casini A, et al. Connecting copper and cancer: from transition metal signalling to metalloplasia. Nat Rev Cancer 2022;22:102-13. [Crossref] [PubMed]
- Yang M, Zheng H, Xu K, et al. A novel signature to guide osteosarcoma prognosis and immune microenvironment: Cuproptosis-related lncRNA. Front Immunol 2022;13:919231. [Crossref] [PubMed]
- Chroma K, Skrott Z, Gursky J, et al. A drug repurposing strategy for overcoming human multiple myeloma resistance to standard-of-care treatment. Cell Death Dis 2022;13:203. [Crossref] [PubMed]
- Mercer TR, Dinger ME, Mattick JS. Long non-coding RNAs: insights into functions. Nat Rev Genet 2009;10:155-9. [Crossref] [PubMed]
- Schmitt AM, Chang HY. Long Noncoding RNAs in Cancer Pathways. Cancer Cell 2016;29:452-63. [Crossref] [PubMed]
- Carninci P, Kasukawa T, Katayama S, et al. The transcriptional landscape of the mammalian genome. Science 2005;309:1559-63. [Crossref] [PubMed]
- Yang G, Li Z, Dong L, et al. lncRNA ADAMTS9-AS1 promotes bladder cancer cell invasion, migration, and inhibits apoptosis and autophagy through PI3K/AKT/mTOR signaling pathway. Int J Biochem Cell Biol 2021;140:106069. [Crossref] [PubMed]
- Zhao W, Geng D, Li S, et al. LncRNA HOTAIR influences cell growth, migration, invasion, and apoptosis via the miR-20a-5p/HMGA2 axis in breast cancer. Cancer Med 2018;7:842-55. [Crossref] [PubMed]
- Mao C, Wang X, Liu Y, et al. A G3BP1-Interacting lncRNA Promotes Ferroptosis and Apoptosis in Cancer via Nuclear Sequestration of p53. Cancer Res 2018;78:3484-96. [Crossref] [PubMed]
- Huang G, Xiang Z, Wu H, et al. The lncRNA BDNF-AS/WDR5/FBXW7 axis mediates ferroptosis in gastric cancer peritoneal metastasis by regulating VDAC3 ubiquitination. Int J Biol Sci 2022;18:1415-33. [Crossref] [PubMed]
- Yang H, Hu Y, Weng M, et al. Hypoxia inducible lncRNA-CBSLR modulates ferroptosis through m6A-YTHDF2-dependent modulation of CBS in gastric cancer. J Adv Res 2021;37:91-106. [Crossref] [PubMed]
- Feng A, He L, Chen T, et al. A novel cuproptosis-related lncRNA nomogram to improve the prognosis prediction of gastric cancer. Front Oncol 2022;12:957966. [Crossref] [PubMed]
- Zhou L, Cheng Q, Hu Y, et al. Cuproptosis-related LncRNAs are potential prognostic and immune response markers for patients with HNSCC via the integration of bioinformatics analysis and experimental validation. Front Oncol 2022;12:1030802. [Crossref] [PubMed]
- Long X, Wen F, Li J, Huang X. LncRNA FEZF1-AS1 accelerates multiple myeloma progression by regulating IGF2BP1/BZW2 signaling. Hematol Oncol 2023;41:694-703. [Crossref] [PubMed]
- Yang C, Liang Y, Shu J, et al. Long non-coding RNAs in multiple myeloma Int J Oncol 2023;62:69. (Review). [Crossref] [PubMed]
- Wang Q. Five cuprotosis-related lncRNA signatures for prognosis prediction in acute myeloid leukaemia. Hematology 2023;28:2231737. [Crossref] [PubMed]
- Xu M, Mu J, Wang J, et al. Construction and validation of a cuproptosis-related lncRNA signature as a novel and robust prognostic model for colon adenocarcinoma. Front Oncol 2022;12:961213. [Crossref] [PubMed]
- Wang F, Lin H, Su Q, et al. Cuproptosis-related lncRNA predict prognosis and immune response of lung adenocarcinoma. World J Surg Oncol 2022;20:275. [Crossref] [PubMed]
- Bai Y, Zhang Q, Liu F, et al. A novel cuproptosis-related lncRNA signature predicts the prognosis and immune landscape in bladder cancer. Front Immunol 2022;13:1027449. [Crossref] [PubMed]
- Chen S, Liu P, Zhao L, et al. A novel cuproptosis-related prognostic lncRNA signature for predicting immune and drug therapy response in hepatocellular carcinoma. Front Immunol 2022;13:954653. [Crossref] [PubMed]
- Weißbach S, Heredia-Guerrero SC, Barnsteiner S, et al. Exon-4 Mutations in KRAS Affect MEK/ERK and PI3K/AKT Signaling in Human Multiple Myeloma Cell Lines. Cancers (Basel) 2020;12:455. [Crossref] [PubMed]
- Huang X, Cao W, Yao S, et al. NEDD4L binds the proteasome and promotes autophagy and bortezomib sensitivity in multiple myeloma. Cell Death Dis 2022;13:197. [Crossref] [PubMed]
- Kastritis E, Terpos E, Dimopoulos MA. How I treat relapsed multiple myeloma. Blood 2022;139:2904-17. [Crossref] [PubMed]
- Liu B, Pang K, Feng C, et al. Comprehensive analysis of a novel cuproptosis-related lncRNA signature associated with prognosis and tumor matrix features to predict immunotherapy in soft tissue carcinoma. Front Genet 2022;13:1063057. [Crossref] [PubMed]
- Zhang G, Sun J, Zhang X. A novel Cuproptosis-related LncRNA signature to predict prognosis in hepatocellular carcinoma. Sci Rep 2022;12:11325. [Crossref] [PubMed]
- Li P, Li J, Wen F, et al. A novel cuproptosis-related LncRNA signature: Prognostic and therapeutic value for acute myeloid leukemia. Front Oncol 2022;12:966920. [Crossref] [PubMed]
- Duan H, Yang Z, Li C, et al. Dysregulated Expression and Methylation Analysis Identified TLX1NB as a Novel Recurrence Marker in Low-Grade Gliomas. Int J Genomics 2020;2020:5069204. [Crossref] [PubMed]
- Chen G, Lian D, Zhao L, et al. The long non-coding RNA T cell leukemia homeobox 1 neighbor enhances signal transducer and activator of transcription 5A phosphorylation to promote colon cancer cell invasion, migration, and metastasis. Bioengineered 2022;13:11083-95. [Crossref] [PubMed]
- Dastjerdi S, Valizadeh M, Nemati R, et al. Highly expressed TLX1NB and NPSR1-AS1 lncRNAs could serve as diagnostic tools in colorectal cancer. Hum Cell 2021;34:1765-74. [Crossref] [PubMed]
- Li X, Li B, Ran P, et al. Identification of ceRNA network based on a RNA-seq shows prognostic lncRNA biomarkers in human lung adenocarcinoma. Oncol Lett 2018;16:5697-708. [Crossref] [PubMed]
- Li XX, Wang LJ, Hou J, et al. Identification of long non-coding RNAs as predictors of survival in triple-negative breast cancer based on network analysis. Biomed Res Int 2020;2020:8970340. [PubMed]
- Qu J, Hou Y, Chen Q, et al. RNA demethylase ALKBH5 promotes tumorigenesis in multiple myeloma via TRAF1-mediated activation of NF-κB and MAPK signaling pathways. Oncogene 2022;41:400-13. [Crossref] [PubMed]
- Vendramini E, Bomben R, Pozzo F, et al. KRAS and RAS-MAPK Pathway Deregulation in Mature B Cell Lymphoproliferative Disorders. Cancers (Basel) 2022;14:666. [Crossref] [PubMed]
- Zhang H, Liu J, Dang Q, et al. Ribosomal protein RPL5 regulates colon cancer cell proliferation and migration through MAPK/ERK signaling pathway. BMC Mol Cell Biol 2022;23:48. [Crossref] [PubMed]
- Wei J, Liu J, Liang S, et al. Low-Dose Exposure of Silica Nanoparticles Induces Neurotoxicity via Neuroactive Ligand-Receptor Interaction Signaling Pathway in Zebrafish Embryos. Int J Nanomedicine 2020;15:4407-15. [Crossref] [PubMed]
- Xu H, Sun Y, Ma Z, et al. LOC134466 methylation promotes oncogenesis of endometrial carcinoma through LOC134466/hsa-miR-196a-5p/TAC1 axis. Aging (Albany NY) 2018;10:3353-70. [Crossref] [PubMed]