LncRNA RP11-10E18.7 cooperates with lncRNA RP11-481C4.2 to affect the overall survival of breast cancer patients: a TCGA-based retrospective study
Highlight box
Key findings
• Our study identified RP11-10E18.7×RP11-481C4.2 and FOXA1×U2SURP interactions as potential biomarkers for breast cancer (BC) prognosis.
What is known and what is new?
• Long non-coding RNAs (lncRNAs) have emerged as crucial players in cancer, with specific lncRNAs identified as potential biomarkers for prognosis and therapeutic targets in various cancer types.
• This is the first study of the relationship between lncRNA-lncRNA interaction and the overall survival of BC.
What is the implication, and what should change now?
• This study was based on the data from the Cancer Genome Atlas (TCGA), therefore, additional available public databases and further researches are warranted.
Introduction
Breast cancer (BC) is one of the leading causes of cancer-related mortality, with approximately 2.3 million new cases (11.7%) per year (1). Despite advances in surgery, chemotherapy, radiotherapy, and neoadjuvant therapy, the mortality rate of BC remains a global challenge (2). In addition, BC also shows a high recurrence rate (3). Due to the pathogenic complexity of BC, many biomarkers have been found, such as estrogen receptor (ER), progesterone receptor (PR), and human epidermal growth factor 2 (HER2) (4,5). However, the prognosis is still a complex problem, indicating the possible existence of new prognosis-influencing molecular mechanisms (6).
Long non-coding RNAs (lncRNAs) are a large class of transcripts from non-protein-coding regions and a group of non-coding RNAs (ncRNAs) of size >200 nucleotides in length (7). LncRNAs are involved in diverse biological processes, such as cell cycle, cell growth, proliferation, migration, invasion, and apoptosis (8). In recent years, lncRNAs have emerged as crucial players in cancer, with specific lncRNAs identified as potential biomarkers for prognosis and therapeutic targets in various cancer types, such as liver, lung, and ovarian cancers (9-12). Further, some lncRNAs may serve as prognosis biomarkers in BC (13-15).
Over the years, mounting studies have revealed that the interactive effects of lncRNA-microRNA (miRNA), lncRNA-messenger RNA (mRNA), and lncRNA-miRNA-mRNA are prognostic signatures in predicting the survival of BC patients (16-18). However, a gap in our understanding pertains to the statistical correlation between interactions among lncRNAs themselves and their impact on the overall survival (OS) of BC. To address this gap, we conducted an analysis using data from the Cancer Genome Atlas (TCGA) data to explore the potential clinical significance of interactive effects between two lncRNAs in the context of BC prognosis. We present this article in accordance with the TRIPOD reporting checklist (available at https://tcr.amegroups.com/article/view/10.21037/tcr-23-1941/rc).
Methods
Study populations
The fragments per kilobase per million (FPKM) of lncRNA expression data and corresponding clinical information of BC were derived from the TCGA database (https://portal.gdc.cancer.gov), which includes different types of BC, such as infiltrating ductal carcinomas and infiltrating lobular carcinomas. LncRNA annotation information came from gencode.v22 (https://www.gencodegenes.org/). The study sample was collected between May 2021 and October 2022. The requirement for approval from our Ethics Committee was waived since the study data was acquired from TCGA. The study was conducted in accordance with the Declaration of Helsinki (as revised in 2013).
Quality control (QC) procedures for lncRNA expression data
FPKM data and gencode.v22 annotations were matched to obtain the expression of 15,900 lncRNAs. In order to obtain reliable biomarkers in lncRNA expression data, we performed QC, after which we performed statistical association analysis. The exclusion criteria of lncRNAs were when all gene expression values equaled to 0 or the proportion of missing values was greater than 10%. Further, samples with any missing clinical variables and male patients were also removed. Finally, 890 female samples with 4,636 lncRNAs remained in the subsequent statistical association analysis. LncRNA was logarithmically transformed before analysis. The average age was 57.86 years for patients (Table 1). The validation sample was enrolled by the same method, including 157 female samples with 817 lncRNAs.
Table 1
Variable | Overall (N=890) | Alive (N=770) | Dead (N=120) | Validation (N=157) |
---|---|---|---|---|
Age (years), mean ± SD | 57.86±13.04 | 57.33±12.68 | 61.27±14.78 | 60.06±11.52 |
Race, n (%) | ||||
Asian | 56 (6.29) | 53 (6.88) | 3 (2.50) | 34 (21.66) |
Black | 169 (18.99) | 143 (18.57) | 26 (21.67) | 56 (35.67) |
White | 665 (74.72) | 574 (74.55) | 91 (75.83) | 67 (42.68) |
Neoadjuvant therapy, n (%) | ||||
No | 882 (99.10) | 765 (99.35) | 117 (97.50) | 154 (98.09) |
Yes | 8 (0.90) | 5 (0.65) | 3 (2.50) | 3 (0.19) |
Clinical stage, n (%) | ||||
I | 164 (18.43) | 149 (19.35) | 15 (12.50) | 25 (15.92) |
II | 509 (57.19) | 455 (59.09) | 54 (45.00) | 80 (50.96) |
III | 204 (22.92) | 164 (21.30) | 40 (33.33) | 48 (30.57) |
IV | 13 (1.46) | 2 (0.26) | 11 (9.17) | 4 (2.55) |
Histology, n (%) | ||||
Infiltrating ductal carcinoma | 625 (70.23) | 540 (70.13) | 85 (70.83) | 118 (75.16) |
Infiltrating lobular carcinoma | 175 (19.66) | 157 (20.39) | 18 (15.00) | 18 (11.46) |
Other | 90 (10.11) | 73 (9.48) | 17 (14.17) | 21 (13.38) |
ER, n (%) | ||||
Negative | 211 (23.71) | 175 (22.73) | 36 (30.00) | 44 (28.03) |
Positive | 679 (76.29) | 595 (77.27) | 84 (70.00) | 113 (71.97) |
PR, n (%) | ||||
Negative | 299 (33.60) | 252 (32.73) | 47 (39.17) | 67 (42.68) |
Positive | 591 (66.40) | 518 (67.27) | 73 (60.83) | 90 (57.32) |
HER2, n (%) | ||||
Negative | 470 (78.86) | 421 (79.73) | 49 (72.06) | 117 (74.52) |
Positive | 126 (21.14) | 107 (20.27) | 19 (27.94) | 31 (19.75) |
Unknown | 294 (33.03) | 242 (43.12) | 52 (43.33) | 9 (5.73) |
Subtype, n (%) | ||||
Basal-like (ER− & PR−, HER2−) | 102 (17.11) | 87 (16.48) | 15 (22.06) | 28 (17.83) |
HER2+ (ER− & PR−, HER2+) | 32 (5.38) | 26 (4.92) | 6 (8.82) | 11 (7.01) |
Luminal A (ER+/PR+, HER2−) | 368 (61.74) | 334 (63.26) | 34 (50.00) | 89 (56.69) |
Luminal B (ER+/PR+, HER2+) | 94 (15.77) | 81 (15.34) | 13 (19.12) | 20 (12.74) |
Unknown | 294 (33.03) | 242 (43.12) | 52 (43.33) | 9 (5.73) |
Survival year | ||||
Median (95% CI) | 2.41 (2.09–2.75) | 2.52 (2.18–2.92) | ||
Censoring rate, n (%) | 120 (13.48) | 20 (12.74) |
SD, standard deviation; ER, estrogen receptor; PR, progesterone receptor; HER2, human epidermal growth factor receptor 2; CI, confidence interval.
mRNA expression data
Expression and mRNA sequencing data for all 890 patients were also downloaded from TCGA. Gene expression was measured by RNA sequencing (RNA-seq). Data processing and QC were completed by the TCGA workgroup. RNA-Seq expectation maximization (RSEM) was adopted to normalize raw counts. We downloaded the Level-3 (gene-level) gene quantification data from TCGA and further checked the data quality. Gene expression data were extracted and transformed on log2 scale before statistical association analysis.
Genome-wide lncRNA-lncRNA interaction analysis
The analysis workflow is displayed in Figure 1. We applied a multivariate Cox proportional hazards model adjusted for age, race, clinical stage, neoadjuvant therapy, ER, and PR to test lncRNA-lncRNA interaction items, by using the R package survival (https://cran.r-project.org/web/packages/survival/index.html). The effect size was measure by hazard ratio (HR) and its 95% confidence interval (CI) according to per 5% expression increment. The significance level accounting for multiple comparison was set based on the Bonferroni method, where the significance level was defined as 0.05 divided by the number of tests. Therefore, we controlled the overall type I error at the 0.05 level. The significance level of lncRNA-lncRNA interaction study was set to be 4.65×10−9=0.05/(4,636×4,635/2).
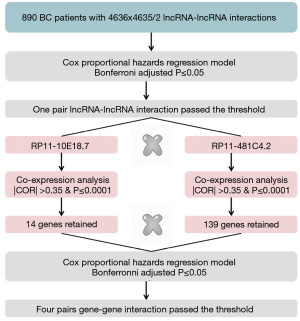
Gene-gene interaction analysis
For a better exploration of the function of the significant lncRNA-lncRNA items, the related mRNAs were identified by co-expression methods based on the Pearson correlation, respectively. The related mRNAs were screened according to |COR| >0.35, Bonferroni adjusted P<0.0001. For the significant co-expression genes, we also applied a multivariate Cox proportional hazards model adjusted for the aforementioned covariates to test gene-gene interactions.
Statistical analysis
Continuous variables were described as mean ± standard deviation and compared by Student’s t-test using R package t-test (19), and these categorized variables were summarized as frequency (n) and proportion (%) and compared by chi-square test. All statistical analyses were performed using R version 3.6.1 (The R Foundation for Statistical Computing, Vienna, Austria), unless otherwise specified. P values less than 0.5 were considered as statistically significance.
Results
For the lncRNA-lncRNA interaction analysis, only RP11-10E18.7×RP11-481C4.2 (HRinteraction =1.04, 95% CI: 1.03–1.06, P=3.35×10−9) was significantly associated with BC OS. As presented in Figure 2A, with the increased expression level of RP11-481C4.2, there was the elevated risk for RP11-10E18.7 on BC OS. Therefore, RP11-481C4.2 was a modifier of the association between RP11-10E18.7 and BC survival. To illustrate the modification effect, patients were categorized into low and high groups based on the intersection (0.69) of RP11-481C4.2 expression in Figure 2A. The effect of RP11-10E18.7 varied across patients with different RP11-481C4.2 expressions. For patients with a low level of RP11-481C4.2, high expression of RP11-10E18.7 had significantly better OS (HRhighvs. low =0.54, 95% CI: 0.34–0.84, P=7.13×10−3) (Figure 2B,2C). Conversely, high expression of RP11-10E18.7 had poor effect on patients with high level of RP11-481C4.2 (HRhighvs. low =2.36, 95% CI: 1.06–5.25, P=3.37×10−3) (Figure 2B,2D). The results showed that the effect direction of RP11-10E18.7 on BC OS was opposite at the low and high levels of RP11-481C4.2. To further evaluate our findings regarding RP11-10E18.7 and RP11-481C4.2, we repeated the interaction analysis in the validation dataset of 817 gene expression samples, and the results were similar (HRinteraction =1.02, 95% CI: 1.01–1.05, P=3.01×10−9).
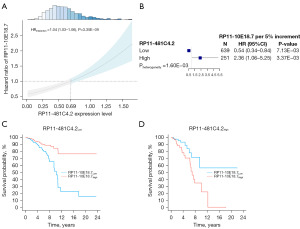
Based on the BC-related mRNA expression data from TCGA, co-expression analysis was performed on RP11-10E18.7 and RP11-481C4.2 respectively. The results showed that 14 and 139 genes were closely related to the 2 lncRNAs, respectively, that were then used for gene-gene interaction analysis (Figure 3). Finally, 4 pairs of genes were identified with Bonferroni adjusted P≤0.05 (Table 2). However, as shown in Figure 4, there were high correlations among the expression of these genes, and the subsequent analysis focused on the FOXA1×U2SURP that had the lowest P value (HRinteraction =1.49, 95% CI: 1.28–1.73, P=2.16×10−7). The associations between RP11-10E18.7 and FOXA1 (r=−0.37, P=3.70×10−30), RP11-481C4.2 and U2SURP (r=0.38, P=1.37×10−32) were all significant (Figure S1).
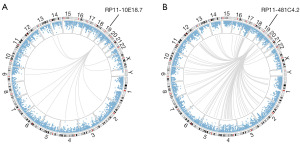
Table 2
Interaction | HR (95% CI) | Z | P value | P adjust |
---|---|---|---|---|
FOXA1×U2SURP | 1.49 (1.28–1.73) | 5.185 | 2.16×10−7 | 4.20×10−4 |
FOXA1×TMEM194A | 1.35 (1.19–1.52) | 4.850 | 1.24×10−6 | 2.40×10−3 |
GATA3×U2SURP | 1.41 (1.21–1.64) | 4.410 | 1.04×10−5 | 0.020 |
ESR1×U2SURP | 1.45 (1.23–1.72) | 4.368 | 1.25×10−5 | 0.024 |
LncRNA, long non-coding RNA; HR, hazard ratio; CI, confidence interval.
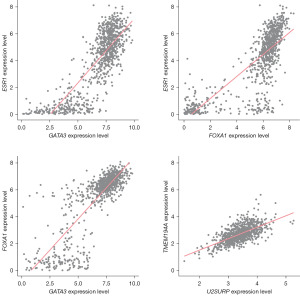
The similar interactive pattern was exhibited between FOXA1 and U2SURP (Figure 5). FOXA1 had an elevated risk on BC OS with the increased expression level of U2SURP (Figure 5A). Again, after classifying the patients by intersection (3.47), we observed that the effect of FOXA1 opposite in patients with different U2SURP expression level (HRhighvs. low =0.58, 95% CI: 0.34–0.99, P=0.046 in low expression of U2SURP; HRhighvs. low =1.56, 95% CI: 1.18–2.87, P=0.029 in high expression of U2SURP) (Figure 5B-5D).
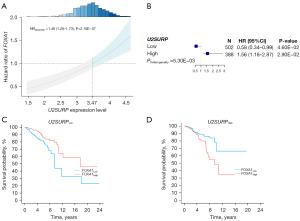
Discussion
In this study, we used transcriptional data from TCGA to identify RP11-10E18.7×RP11-481C4.2 interaction that had an impact on BC OS. Then, through co-expression analysis, 4 pairs of gene-gene interactions, including FOXA1×U2SURP, were also found to be associated with BC OS.
Approximately 93% of DNA can be transcribed as RNA in the human genome, 98% of which is known as ncRNAs (20). Among them, lncRNAs are RNAs longer than 200 nucleotides in length that have been demonstrated to play important roles in epigenetic control and the regulation of transcription and translation (21). As a new type of gene regulator, lncRNA is related to the occurrence, development, and prognosis of human diseases, especially cancer (22,23). In recent years, lncRNAs have been demonstrated to be engaged in BC development, progression, invasion, and metastasis (24-27).
Several studies have demonstrated that the abnormal expression of some lncRNAs may be associated with the poor prognosis of BC by testing their main effects (28,29). To the best of our knowledge, this may be the first attempt to explore the relationship between lncRNA-lncRNA interactions and BC OS at the population level. Interactions have been shown to provide important clues to the biologic mechanisms of complex diseases (30). Besides this, interactions could increase the power to detect associations and then be leveraged for the identification of new biomarkers (31). Previous studies have identified some gene-gene interactions related to BC survival (32,33). Our results found that biomarkers with RP11-10E18.7×RP11-481C4.2 interaction and FOXA1×U2SURP interaction significantly affected the prognosis of BC.
LncRNA RP11-10E18.7 was found to be associated with miRNA hsa-miR-181a-5p that regulated gene SRPK2 in blood (34). Forkhead box A1 (FOXA1) is a forkhead box transcription factor expressed in mammary luminal epithelial cells (LECs) (35). FOXA1 mutations are a hallmark of ER+ BC and have been widely regarded as a determinant of breast tumor response to endocrine therapy as well as a marker for favorable patient prognosis (36). Previous study has indicated that elevated FOXA1 expression level is associated with better outcome in BC (37). However, our results showed that high level of FOXA1 expression had protective effect on BC survival only when U2SURP expression was low, meaning that U2SURP modified the relationship between FOXA1 and BC OS. Meanwhile, U2SURP was also found to be significantly associated with BC survival (38).
Our study has several advantages. First, to our knowledge, this is the first study of the relationship between lncRNA-lncRNA interaction and the OS of BC. The RP11-10E18.7×RP11-481C4.2 interaction provides potential evidence that complex disease is driven by intricate association patterns. Second, our study used co-expression analysis to find gene-gene interactions related to lncRNAs and impacting BC OS, which was a comprehensive evaluation of lncRNAs. Third, for the lncRNA-lncRNA interaction analysis, we applied the most conservative Bonferroni correction to control for false positives.
The study has some limitations. First, our study lacked independent validation. Additional available public databases and further researches are warranted. Second, biological evidence requires further functional experiments of lncRNAs, not just our statistical evidence. This association should be interpreted with caution.
Conclusions
Our study identified RP11-10E18.7×RP11-481C4.2 and FOXA1×U2SURP interactions as potential biomarkers for BC prognosis. Our findings elucidated potential molecular mechanisms of BC progression under complex association patterns and provided potential dynamic and reversible therapeutic targets for BC patients.
Acknowledgments
Funding: This study was funded by Outstanding Young Medical Talents Program of Pudong Health Bureau of Shanghai (No. PWRq2020-40).
Footnote
Reporting Checklist: The authors have completed the TRIPOD reporting checklist. Available at https://tcr.amegroups.com/article/view/10.21037/tcr-23-1941/rc
Peer Review File: Available at https://tcr.amegroups.com/article/view/10.21037/tcr-23-1941/prf
Conflicts of Interest: All authors have completed the ICMJE uniform disclosure form (available at https://tcr.amegroups.com/article/view/10.21037/tcr-23-1941/coif). The authors have no conflicts of interest to declare.
Ethical Statement: The authors are accountable for all aspects of the work in ensuring that questions related to the accuracy or integrity of any part of the work are appropriately investigated and resolved. The study was conducted in accordance with the Declaration of Helsinki (as revised in 2013).
Open Access Statement: This is an Open Access article distributed in accordance with the Creative Commons Attribution-NonCommercial-NoDerivs 4.0 International License (CC BY-NC-ND 4.0), which permits the non-commercial replication and distribution of the article with the strict proviso that no changes or edits are made and the original work is properly cited (including links to both the formal publication through the relevant DOI and the license). See: https://creativecommons.org/licenses/by-nc-nd/4.0/.
References
- Sung H, Ferlay J, Siegel RL, et al. Global Cancer Statistics 2020: GLOBOCAN Estimates of Incidence and Mortality Worldwide for 36 Cancers in 185 Countries. CA Cancer J Clin 2021;71:209-49. [Crossref] [PubMed]
- Valachis A, Nearchou AD, Lind P. Surgical management of breast cancer in BRCA-mutation carriers: a systematic review and meta-analysis. Breast Cancer Res Treat 2014;144:443-55. [Crossref] [PubMed]
- Yang B, Chou J, Tao Y, et al. An assessment of prognostic immunity markers in breast cancer. NPJ Breast Cancer 2018;4:35. [Crossref] [PubMed]
- Senkus E, Kyriakides S, Ohno S, et al. Primary breast cancer: ESMO Clinical Practice Guidelines for diagnosis, treatment and follow-up. Ann Oncol 2015;26:v8-30. [Crossref] [PubMed]
- Zhu A, Yun Z, You M, et al. Surgical reduction in chest wall disease to prolong survival in breast cancer patients: a retrospective study. Gland Surg 2022;11:1015-25. [Crossref] [PubMed]
- Arnedos M, Vicier C, Loi S, et al. Precision medicine for metastatic breast cancer--limitations and solutions. Nat Rev Clin Oncol 2015;12:693-704. [Crossref] [PubMed]
- Matsui M, Corey DR. Non-coding RNAs as drug targets. Nat Rev Drug Discov 2017;16:167-79. [Crossref] [PubMed]
- Bhan A, Soleimani M, Mandal SS. Long Noncoding RNA and Cancer: A New Paradigm. Cancer Res 2017;77:3965-81. [Crossref] [PubMed]
- Lin C, Yang L. Long Noncoding RNA in Cancer: Wiring Signaling Circuitry. Trends Cell Biol 2018;28:287-301. [Crossref] [PubMed]
- Parasramka MA, Maji S, Matsuda A, et al. Long non-coding RNAs as novel targets for therapy in hepatocellular carcinoma. Pharmacol Ther 2016;161:67-78. [Crossref] [PubMed]
- Pan J, Fang S, Tian H, et al. lncRNA JPX/miR-33a-5p/Twist1 axis regulates tumorigenesis and metastasis of lung cancer by activating Wnt/β-catenin signaling. Mol Cancer 2020;19:9. [Crossref] [PubMed]
- Xu D, Guo Y, Lei S, et al. Identification and Characterization of TF-lncRNA Regulatory Networks Involved in the Tumorigenesis and Development of Adamantinomatous Craniopharyngioma. Front Oncol 2022;11:739714. [Crossref] [PubMed]
- Crudele F, Bianchi N, Reali E, et al. The network of non-coding RNAs and their molecular targets in breast cancer. Mol Cancer 2020;19:61. [Crossref] [PubMed]
- Tomar D, Yadav AS, Kumar D, et al. Non-coding RNAs as potential therapeutic targets in breast cancer. Biochim Biophys Acta Gene Regul Mech 2020;1863:194378. [Crossref] [PubMed]
- Chen J, Ling C. Construction of a predictive model for breast cancer metastasis based on lncRNAs. Transl Cancer Res 2023;12:387-397. [Crossref] [PubMed]
- Müller V, Oliveira-Ferrer L, Steinbach B, et al. Interplay of lncRNA H19/miR-675 and lncRNA NEAT1/miR-204 in breast cancer. Mol Oncol 2019;13:1137-49. [Crossref] [PubMed]
- Jiang YZ, Liu YR, Xu XE, et al. Transcriptome Analysis of Triple-Negative Breast Cancer Reveals an Integrated mRNA-lncRNA Signature with Predictive and Prognostic Value. Cancer Res 2016;76:2105-14. [Crossref] [PubMed]
- Fan CN, Ma L, Liu N. Systematic analysis of lncRNA-miRNA-mRNA competing endogenous RNA network identifies four-lncRNA signature as a prognostic biomarker for breast cancer. J Transl Med 2018;16:264. [Crossref] [PubMed]
- Ramsden JD. Angiogenesis in the thyroid gland. J Endocrinol 2000;166:475-80. [Crossref] [PubMed]
- Mattick JS. Non-coding RNAs: the architects of eukaryotic complexity. EMBO Rep 2001;2:986-91. [Crossref] [PubMed]
- Carrieri C, Cimatti L, Biagioli M, et al. Long non-coding antisense RNA controls Uchl1 translation through an embedded SINEB2 repeat. Nature 2012;491:454-7. [Crossref] [PubMed]
- Liang C, Qi Z, Ge H, et al. Long non-coding RNA PCAT-1 in human cancers: A meta-analysis. Clin Chim Acta 2018;480:47-55. [Crossref] [PubMed]
- Chen Y, Li Z, Chen X, et al. Long non-coding RNAs: From disease code to drug role. Acta Pharm Sin B 2021;11:340-54. [Crossref] [PubMed]
- Huang QY, Liu GF, Qian XL, et al. Long Non-Coding RNA: Dual Effects on Breast Cancer Metastasis and Clinical Applications. Cancers (Basel) 2019;11:1802. [Crossref] [PubMed]
- Zhang Y, Huang X, Liu J, et al. New insight into long non-coding RNAs associated with bone metastasis of breast cancer based on an integrated analysis. Cancer Cell Int 2021;21:372. [Crossref] [PubMed]
- Sha R, Wu Z, Xu Y, et al. Predictive value of lncRNA LOC100505851 in breast cancer in the neoadjuvant setting. Gland Surg 2021;10:1899-909. [Crossref] [PubMed]
- Zhang Y, He W, Zhang S. Seeking for Correlative Genes annd Signaling Pathways with Bone Metastasis from Breast Cancer by Integrated Analysis. Front Oncol 2019;9:138. [Crossref] [PubMed]
- Yang J, Zhang X, Ye Y, et al. Postmastectomy radiation therapy can improve survival for breast cancer patients with 1–3 positive axillary lymph nodes: a retrospective cohort study using the SEER database. Transl Cancer Res 2021;10:1984-2001. [Crossref] [PubMed]
- Li W, Jia G, Qu Y, et al. Long Non-Coding RNA (LncRNA) HOXA11-AS Promotes Breast Cancer Invasion and Metastasis by Regulating Epithelial-Mesenchymal Transition. Med Sci Monit 2017;23:3393-403. [Crossref] [PubMed]
- Trerotola M, Relli V, Simeone P, et al. Epigenetic inheritance and the missing heritability. Hum Genomics 2015;9:17. [Crossref] [PubMed]
- Cordell HJ. Detecting gene-gene interactions that underlie human diseases. Nat Rev Genet 2009;10:392-404. [Crossref] [PubMed]
- Chen X, Theobard R, Zhang J, et al. Genetic interactions between INPP4B and RAD50 is prognostic of breast cancer survival. Biosci Rep 2020;40:BSR20192546. [Crossref] [PubMed]
- Dai X, Fagerholm R, Khan S, et al. INPP4B and RAD50 have an interactive effect on survival after breast cancer. Breast Cancer Res Treat 2015;149:363-71. [Crossref] [PubMed]
- Su L, Wang C, Zheng C, et al. A meta-analysis of public microarray data identifies biological regulatory networks in Parkinson's disease. BMC Med Genomics 2018;11:40. [Crossref] [PubMed]
- Carroll JS, Liu XS, Brodsky AS, et al. Chromosome-wide mapping of estrogen receptor binding reveals long-range regulation requiring the forkhead protein FoxA1. Cell 2005;122:33-43. [Crossref] [PubMed]
- Hurtado A, Holmes KA, Ross-Innes CS, et al. FOXA1 is a key determinant of estrogen receptor function and endocrine response. Nat Genet 2011;43:27-33. [Crossref] [PubMed]
- Shou J, Lai Y, Xu J, et al. Prognostic value of FOXA1 in breast cancer: A systematic review and meta-analysis. Breast 2016;27:35-43. [Crossref] [PubMed]
- An J, Luo Z, An W, et al. Identification of spliceosome components pivotal to breast cancer survival. RNA Biol 2021;18:833-42. [Crossref] [PubMed]