A novel prognostic and therapeutic target biomarker based on complement-related gene signature in gastric cancer
Highlight box
Key findings
• The novel complement-related gene (CRG) signature may act as a reliable, efficient tool for prognostic prediction and treatment guidance of gastric cancer (GC) patients in future clinical practice.
What is known and what is new?
• GC is one of the most prevalent cancer types that reduce human life expectancy. The current TNM staging system is inadequate in identifying higher or lower risk of GC patients because of tumor heterogeneity. Research shows that complement plays a dual role in the tumor development and progression of GC.
• We performed bioinformatics analysis to construct a CRG risk model, and potential associations of the tumor immune microenvironment, immunotherapy response and drug resistance with CRG risk score were comprehensively explored.
What is the implication, and what should change now?
• The novel CRG signature can be used for prognostic prediction and treatment guidance of GC patients in future clinical practice.
Introduction
Gastric cancer (GC) is one of the most prevalent cancer types that reduce human life expectancy. According to the latest data on cancer statistics, GC ranks as the fifth most commonly diagnosed cancer and the fourth leading cause of cancer-related deaths globally (1). Early-stage GC is associated with few signs or symptoms, and the majority of GC patients are diagnosed at advanced stage and have less than 30% 5-year survival rate (2). Current treatment methods for GC include surgery, chemotherapy, radiation, targeted therapy and immunotherapy (3,4). The American Joint Committee on Cancer (AJCC) tumor-node-metastasis (TNM) staging criterion remains the most commonly used system to predict prognosis and guide treatment decision-making in GC patients in clinical practice. However, patients with GC at the same clinical stage would show different therapeutic efficacy due to GC’s considerable heterogeneity (5-7). Therefore, TNM staging may be unable to distinguish between higher and lower risk patients. New strategies are urgently needed to improve survival prediction and further guide individualized cancer treatment.
The complement-related genes (CRGs) have attracted growing attention in recent years in the field of oncology. The complement system, composed of more than 50 proteins, is an important part of the innate immune system. It is traditionally believed that the complement plays a critical role in the immune surveillance and eradication of tumor cells. The activation of the complement pathway can occur via the classical, lectin or alternative pathways. This process can help antigen-presenting cells (APCs) recognize tumor cells, and form membrane attack complex (MAC), leading to tumor cell lysis (8,9). The tumor microenvironment (TME) consists of tumour cells, stromal cells, immune cells and extracellular matrix, which plays a crucial role in tumor development and progression (10). Evidence has recognized the close relationship between complement factors and TME (11). The complement can recruit and activate immunosuppressive cells in TME, which contributes to the formation of an immunosuppressive microenvironment and promotes tumor progression (12,13). The low expression of Claudin-18 was closely related to nerve invasion in GC, which indicated the poor clinical prognosis of GC patients (14). A recent study showed that C3 overexpression could activate the JAK2/STAT3 pathway, and then induce GC progression (15). Chen et al. (16) believed that the C5a/C5aR complement pathway inhibits p21/p-p21 expression by activating the PI3K/AKT signaling pathway, thereby promoting GC progression. These findings show that complement plays an important role in cancer. Whether these CRGs are related to the prognosis of GC needs further study.
In the present study, we collected the sequences of ribonucleic acid (RNA-seq) data and the corresponding clinical data from public databases. We developed a CRG signature for predicting the prognosis of GC and explored the potential associations of the TME, immunotherapy response and drug resistance with CRG risk score. We present this article in accordance with the TRIPOD reporting checklist (available at https://tcr.amegroups.com/article/view/10.21037/tcr-23-628/rc).
Methods
Data acquisition
The RNA-seq data and corresponding clinicopathological information of GC samples were downloaded from The Cancer Genome Atlas (TCGA) database (https://cancergenome.nih.gov). GSE84437 dataset was acquired from Gene Expression Omnibus (GEO) database (http://www.ncbi.nlm.nih.gov/gds). Additionally, a list of 200 CRGs was obtained from the Molecular Signature Database (MSigDB). Samples without complete survival information were excluded. The TCGA dataset and GSE84437 dataset were used as the training cohort and the external validation cohort, respectively. The study was conducted in accordance with the Declaration of Helsinki (as revised in 2013).
Identification of differentially expressed complement-related genes (DECRGs)
Differentially expressed genes (DEGs) between GC tissues and normal gastric tissue were selected from the gene matrix acquired from the TCGA database using the R software “DESeq2” package. The screening criteria were set as adjusted P-value <0.05 and |logFC| ≥1. Then, the intersection of CRGs and DEGs was identified as DECRGs.
Establishment and verification of the prognostic CRG signature
A univariate Cox regression analysis was applied to screen genes related to prognosis from DECRGs. Based on a P value of less than 0.05, we selected the top 10 genes with higher absolute hazard ratio (HR) values as the significant prognostic differences. To further narrow the range of genes and create a more simplified prognostic model, least absolute shrinkage and selection operator (LASSO) Cox regression analysis was adopted using the “glmnet” package for R software. Finally, a prognostic CRG signature was developed to calculate individual risk scores. The formula was as follows: risk score = ∑xi × coef i, represents the gene coefficient, and X reflects the gene expression level. Patients with GC were classified into a high- and low-risk group with the median risk score as the cutoff point. Kaplan-Meier (K-M) survival analysis was performed to demonstrate the prognostic significance of the two risk subgroups. Time-dependent receiver operating characteristic (ROC) curves were used to evaluate the performance of the CRG signature. To verify the reliability of the model, we applied the same procedures in GSE84437 data. The R package “survminer” was used to visualize K-M survival curve, and the R package “survival ROC” was used to draw ROC curve.
Stratification analysis of the CRG signature
Stratified analysis was employed to test the predictive power of signature in various subgroups of patients. According to their clinicopathological characteristics, patients were divided into different subgroups, including age (≤65 and >65 years), sex (male and female), tumor stage (I–II and III–IV), T stage (T1–2 and T3–4), N stage (N0 and N1–3), grade (G1–2, G3). Overall survival (OS) analysis was performed to compare survival differences in different subgroups. Similarly, we also conducted a stratified analysis in the GSE84437 dataset.
Development of a prognostic nomogram
To assess the potential clinical utility of the prognostic model, we designed a nomogram integrating the risk score and common clinical factors to estimate the 1-, 2- and 3-year survival probability. Moreover, calibration curves for 1-, 2-, and 3-year OS were plotted to evaluate the goodness-of-fit and consistency of the model. The nomogram and calibration curves were drawn using “rms” and “survival” packages for R.
Gene set enrichment analysis (GSEA)
To further investigate the molecular mechanism of risk signature, the R package “Limma” was used to carry out GSEA in high- and low-risk groups. KEGG gene sets were obtained from the GSEA online database (Gsea-msigdb.org).
Comprehensive analysis of immune characteristics and gene mutations in different risk groups
The CIBERSORT algorithm was employed to estimate the relative proportions of tumor-infiltrating 22 types of immune cells. The single-sample GSEA (ssGSEA) method was performed by its R package “gsva” to investigate the differences in immune cell function between different risk subgroups. The ESTIMATE algorithm was applied to compare the immune and stromal scores between high- and low-risk groups. In addition, we also explored the correlations between the expression of six prognostic CRGs and immune cells. To further investigate the genetic variation between different risk subgroups, we obtained information on genetic alterations from the cBioPortal database, and used R package “Maftools” to analyze the differences of gene mutations in two risk subgroups.
Immunotherapy response prediction and drug susceptibility analysis
To evaluate whether CRG signature can predict response to immunotherapy, we analysed the associations of risk score with immune checkpoint gene expression and human leukocyte antigen (HLA) gene expression. To explore the application value of CRG signature in clinical drug selection, we assessed drug susceptibility in the two risk groups and calculated the half-maximal inhibitory concentration (IC50) values of commonly used chemotherapy or targeted therapeutic drugs by using R package “pRRophetic”.
Statistical analyses
Statistical analyses were performed using R statistical software (version 4.1.1). Differences between groups were analyzed using the Student’s t-test or the Wilcoxon test for continuous variables, and χ2 test or Fisher’s exact test for categorical variables. To measure the correlation between two variables, the Spearman correlation coefficient was calculated. All statistical P values were two-sided, and P<0.05 was considered statistically significant (*, P<0.05; **, P<0.01; ***, P<0.001 and ****, P<0.0001).
Results
Clinicopathologic features of patients with GC
Figure 1 depicts the design of the current study. This study included 353 GC samples from the TCGA database and 433 GC patients from the GSE84437 dataset. The detailed clinicopathological information of the two cohorts is summarized in the Table 1.
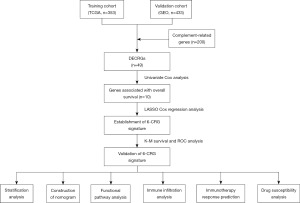
Table 1
Variables | TCGA cohort (n=353) | GEO cohort (n=433) |
---|---|---|
Age (years) | 65.51±10.62 | 60.06±11.58 |
≤65 | 158 (44.8) | 283 (65.4) |
>65 | 192 (54.4) | 150 (34.6) |
Missing data | 3 (0.8) | – |
Gender | ||
Female | 125 (35.4) | 137 (31.6) |
Male | 228 (64.6) | 296 (68.4) |
Grade | ||
G1–2 | 137 (38.8) | – |
G3 | 207 (58.6) | – |
Missing data | 9 (2.6) | – |
Stage | ||
I | 48 (13.6) | – |
II | 109 (30.9) | – |
III | 146 (41.4) | – |
IV | 35 (9.9) | – |
Missing data | 15 (4.2) | – |
Data are presented as mean ± SD or n (%). TCGA, The Cancer Genome Atlas; GEO, Gene Expression Omnibus; SD, standard deviation.
Identification of DECRGs
A total of 4,406 DEGs were screened from TCGA database and 200 CRGs were obtained from the MSigDB (Table S1). As presented in Figure 2A, 49 DECRGs were identified through Venn diagram analyses.
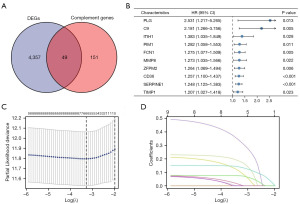
Development of CRG signature
Sixteen genes associated with OS were screened from DECRGs using univariate Cox regression analysis. The top ten genes with the higher absolute HR values were presented in Figure 2B. Subsequently, a LASSO Cox regression model was constructed (Figure 2C,2D), and six CRGs were finally identified, including PLG, C9, ITIH1, ZFPM2, CD36, and SERPINE1. The signature formula was as follows: risk score = (0.1804 × expression of PLG) + (0.3302 × expression of C9) + (0.1029 × expression of ITIH1) + (0.0078 × expression of ZFPM2) + (0.0740 × expression of CD36) + (0.1385 × expression of SERPINE1). According to the median risk score, 350 GC cases were divided into high- and low-risk groups. The risk curves showed the death sample survival time decreased with an increase in the risk score. Most deaths occurred in the high-risk group (Figure 3A). Heatmap depicted the expression of six genes in the prognostic model, and it was higher in the high-risk group (Figure 3A). Moreover, we conducted further survival analysis on the basis of the risk score, and found that the overall prognosis of patients with low-risk score was better than that of patients with high-risk score (Figure 3B). To further evaluate the model performance, we plotted ROC curves. The area under the curve (AUC) values for 1, 3, and 5 years were 0.616, 0.686, and 0.771, respectively (Figure 3C).
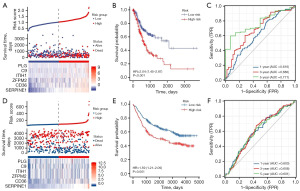
Validation of CRG signature
We used the GSE84437 dataset for external validation. The same risk model was applied to calculate individual risk scores. As shown in Figure 3D, the high-risk group had less survival time and more deaths. Heatmap also showed that the six genes were highly expressed in the high-risk group (Figure 3D). The K-M survival curves showed that the survival probability in the high-risk group was significantly shorter than that in the low-risk group (Figure 3E). The AUC values for 1, 3 and 5 years were 0.603, 0.608 and 0.601, respectively (Figure 3F). These results indicate that this model has a moderate accuracy in predicting the prognosis of GC patients.
Stratification analysis of the CRG signature
In different clinicopathological subgroups, such as age, sex, tumor stage, T stage, N stage and grade, the OS had a significant difference between high- and low-risk patients. High-risk GC patients had worse OS than low-risk patients (Figure 4A-4L). The same conclusion can be reached in GSE84437 (Figure 5A-5H).
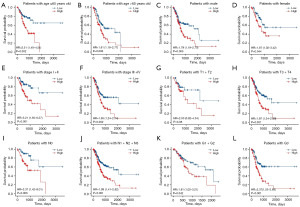
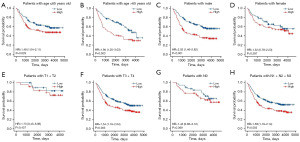
Construction and validation of nomogram based on CRG risk score
The CRG risk score and common clinicopathological factors, including age, gender, clinical stage and pathological grade were analyzed using univariate and multivariate Cox regression analyses. It revealed that CRG risk score was an independent prognostic factor for GC patients in the TCGA cohort (Table 2). To further explore the prognostic value of the risk signature, we constructed the nomogram with combined risk score and factors showed significance in the multivariate analysis (Figure 6A). The 1-, 2-, and 3-year calibration curves demonstrated the accuracy of the nomogram (Figure 6B-6D).
Table 2
Characteristics | Univariate analysis | Multivariate analysis | |||
---|---|---|---|---|---|
HR (95% CI) | P value | HR (95% CI) | P value | ||
Age | 1.022 (1.005–1.038) | 0.010* | 1.030 (1.012–1.049) | 0.001* | |
Sex | |||||
Male | Reference | ||||
Female | 0.771 (0.541–1.101) | 0.152 | |||
Grade | |||||
G2 | Reference | ||||
G3 | 1.305 (0.918–1.856) | 0.138 | |||
G1 | 0.607 (0.147–2.500) | 0.490 | |||
Stage | |||||
I | Reference | ||||
II | 2.385 (1.258–4.522) | 0.008* | 2.437 (1.283–4.630) | 0.007* | |
III | 1.553 (0.783–3.082) | 0.208 | 1.678 (0.845–3.334) | 0.140 | |
IV | 3.827 (1.855–7.898) | <0.001* | 5.518 (2.628–11.586) | <0.001* | |
Risk score | 4.220 (2.507–7.102) | <0.001* | 4.672 (2.631–8.294) | <0.001* |
*, P<0.05; HR, hazard ratio; 95% CI: 95% confidence interval.
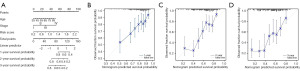
GSEA between different risk subgroups
GSEA was conducted to identify KEGG enrichment differences in different risk subgroups. We observed that the pathways such as cytosolic DNA sensing, olfactory transduction, regulation of autophagy, and receptor signaling were enriched in the high-risk group, while the gene sets in the low-risk group were enriched in RIG-I like receptors, base excision repair, DNA replication, and nucleotide excision repair pathways (Figure 7A,7B).
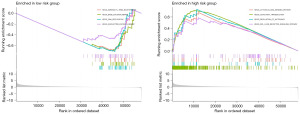
Immune characteristics and mutation analysis in the high- and low-risk groups
CIBERSORT algorithm results indicated positive associations between the prognostic CRG risk score and eosinophils, activated mast cells and neutrophils, and negative correlations with activated natural killer (NK) cells (Figure 8A-8D). We further examined the relationship between 6 prognostic CRGs and 22 human immune-related cells. It could be found that the majority of immune cells were significantly positively regulated with CD36, ITIH1, SERPINE1, ZFPM2, while negatively regulated with C9 and PLG (Figure 8E). The relative proportion of immune infiltration was also visualized (Figure 8F). In addition, ssGSEA analysis showed that the high-risk score was positively associated with most immune cells and immune-related pathways (Figure 8G). Next, the ESTIMATE algorithm was applied to explore the relationship between risk score and TME. The results showed that the immune score, stromal score, and estimate score of patients in the high-risk group were higher than those in the low-risk group. It suggested that high-risk group had more stromal component in the TME (Figure 8H). Furthermore, mutation analysis in TCGA samples revealed that 5%, 9%, 5%, 10%, 4%, and 5% of patients had mutations in PLG, C9, ITIH1, ZFPM2, CD36 and SERPINE1, respectively (Figure 9A). The distribution of somatic mutations was roughly the same between two risk subgroups. Missense variations, nonsense variations, and frameshift deletions were the common mutation types. The mutation rates of TTN, TP53 and MUC16 were more than 30% in the two subgroups (Figure 9B,9C).
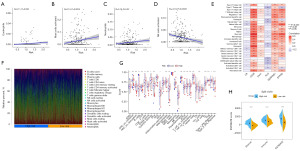
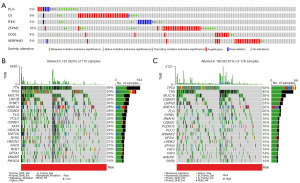
Immunotherapy response prediction and drug susceptibility analysis
To evaluate the predictive ability of the model for immunotherapy response, we analyzed the relationship between CRG risk score with immune checkpoint genes and HLA gene expression. Notably, the results indicated that most immune checkpoint genes and HLA genes were highly expressed in the high-risk groups (Figure 10A,10B). In addition, the differences in drug susceptibility between the high- and low-risk groups were analyzed to detect suitable chemotherapy or targeted drugs for GC patients. Interestingly, patients in the high-risk group had lower IC50 values for axitinib, dasatinib, pazopanib, saracatinib, sunitinib and temsirolimus (Figure 11A-11F), while patients in the low-risk group had significantly lower IC50 values for 5-fluorouracil, epothilone, mitomycin, and pyrimethamine (Figure 11G-11J). Taken together, these data support the feasibility and potential utility of CRG signature in the prediction of drug sensitivity and selection of appropriate treatment drugs for GC patients.
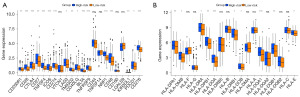
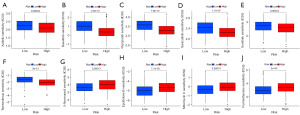
Discussion
GC is one of the most common malignant tumors worldwide, with a high incidence rate, poor prognosis and high mortality (17). Due to the limitations of the traditional TNM staging system, it is still a great challenge to accurately predict the prognosis and risk stratification of patients with GC. The complement system is an ancient part of the immune system that bridges innate and adaptive immunity (18). This system is responsible for clearing immune complexes, invading bacteria, and eliminating abnormal somatic cells, including tumor cells (19). Research evidence has showed that complement plays a dual role in cancer and can regulate the fate of tumors in both directions. Meanwhile, the expression of complement genes is associated with the survival of various tumors, including GC (19,20). At present, there are few studies on CRG-associated biomarkers for predicting prognosis of GC patients. In view of the potential impact of complement system on the occurrence and development of GC, the identification of novel CRG-associated biomarkers may facilitate prognosis assessment and therapeutic guidance for patients with GC.
In our study, we first intersected DEGs acquired from TCGA cohorts with CRGs obtained from MSigDB. A total of 49 DECRGs between GC tissues and normal gastric tissue were identified. After discovering the survival-related CRGs using univariate regression analysis, the LASSO Cox regression analysis was applied, and a 6-gene signature was generated to predict individual survival among patients with GC. Risk curve analysis, survival analysis and ROC curve analysis were conducted in both the training set and validation set. The results demonstrated that our signature was robust enough to predict the prognosis of GC patients. In addition, we created the nomogram integrated independent prognostic factors including risk score, age and stage for predicting 1-, 2-, and 3-year OS. This nomogram might offer convenient and reliable prognosis prediction information of patients with GC for clinicians.
To further understand the mechanism underlying our risk signature, GSEA was conducted to determine the differences in enrichment pathways between high- and low-risk group. We found that the enrichment pathways in the low-risk group were mostly related to genetic repair. The activation of DNA damage repair in tumor such as breast cancer serves to restore genetic integrity and impede tumor progression (21). Functional genetic variants of DNA repair genes may change the host DNA repair ability and thus affect tumor prognosis (22).
In recent years, the rapid development of immunotherapy has revolutionized tumor therapy. However, GC is a highly heterogeneous malignant tumor, and the proportion of patients who benefit from these treatments clinically remains small (23,24). Considering the close connection between the complement system and the immune system, we examined whether our signature could also play a role in predicting immunotherapy response. The infiltration of TME immune cells is considered one of the most important factors to predict the immunotherapeutic response of many tumors in clinic (25,26). Therefore, we further compared the difference of TME between two groups. By using the CIBERSORT algorithm, we observed that the risk score was positively correlated with eosinophils, activated mast cells and neutrophils. This suggests that infiltration of these cells may contribute to formation of TME and higher the risk of fatality. And the risk score was negatively correlated with activated NK cells. High neutrophil infiltration of immune cells in pancreatic ductal adenocarcinoma and hepatocellular carcinoma indicated a higher malignancy and a worse prognosis (27,28). Infiltration of neutrophils could also promote GC cell migration and invasion via EMT pathway (29). It has been reported that NK cells are positively correlated with T-cell infiltration, and associated with favorable prognosis in patients with neuroblastoma (30). The ssGSEA analysis based on 29 immune signatures demonstrated a higher enrichment score in the high-risk group. These results showed that patients in the high-risk group may benefit from immunotherapy. Attractively, our research showed that the high-risk score was characterized by high stromal score, which indicated that patients in the high-risk group had a larger ratio of stroma component but poorer prognosis. We speculated that it is due that the TME of the high-risk group belonged to the immune-excluded subtype. Although there are a large number of infiltrating immune cells in TME, they cannot recognize and eliminate cancer cells because of the obstruction of abundant stromal elements (31). In breast cancer, the presence of stromal cells in the TME has been confirmed to be related to epithelial-mesenchymal transition (32).
The ability of immune system to kill tumor cells depends on the efficient antigen presentation of HLA molecules. There is increasing evidence that HLA is a useful predictor of immunotherapy efficacy (33). Patients with higher expression of HLA-related genes might have a better response to immune checkpoint blockade (34,35). In our present study, the expression of most HLA-related genes and immune checkpoint genes was higher in the high-risk group. The results indicated that patients in the high-risk group may have a better response to immunotherapy, and this conclusion is consistent with a recent study (36). In addition, analysis of resistance and susceptibility to chemotherapy or targeted therapeutic agents verified the potential of CRG signature to predict the therapeutic effect. In a drug sensitivity analysis, high-risk group were more sensitive to targeted agents such as axitinib, dasatinib, pazopanib, saracatinib, sunitinib and temsirolimus, low-risk group were more sensitive to chemotherapy agents such as 5-fluorouracil, epothilone, mitomycin, and pyrimethamine. Therefore, our signature provides guidance for the selection drugs for advanced GC.
Among the six genes in the risk signature, SERPINE1, a fibrinolytic inhibitor, is exactly a tissue plasminogen activator and urokinase inhibitor. Yang et al. (37) discovered that SERPINE1 was elevated in the GC tissues, and it could promote migration and invasion by regulating EMT. CD36, a cell surface receptor, has been found to promote the occurrence and development of multiple types of cancers (38,39). Chen et al. (40) reported that elevated expression of CD36 in GC tissues correlated with poor prognosis. A study (41) has shown that CD36 mediates palmitate acid-induced metastasis of GC via AKT/GSK-3β/β-catenin pathway. It has been reported that C9 is a potentially useful biomarker for the detection of GC (42). Elevated levels of C9 have been found in serum samples from patients with acute leukemia and sarcoma (43). ZFPM2 is one important member of the FOG family. In the study of hepatocellular carcinoma (44), the higher expression level of ZFPM2 gene was associated with a more favorable prognosis. As reported, plasminogen (Plg) is involved in extracellular matrix degradation, cell migration, angiogenesis, tumorigenesis and metastasis (45,46). Fang et al. demonstrated that plasminogen kringle 5 suppressed the growth of GC by inhibiting angiogenesis and apoptosis (47). ITIH1 is one of five members of the inter-α-trypsin inhibitor (ITI) family. Studies have shown that all members of the ITI family play an important role in cell malignant processes and tumor growth (48,49). The previous pan-cancer study has reported that ITIHs were mostly down-regulated in cancers (48). Therefore, the role of ZFPM2, PLG, and ITIH1in the progression of GC needs further exploration.
The innovative aspect of this study is that analyze the prognosis of GC by CRGs, and to establish a prognosis model associated with OS by fewer genes. However, it should be noted that there are some limitations to the current study. First, the survival prediction model in this study was constructed and validated with retrospective data from public databases. In addition, this study was mainly analyzed by bioinformatics methods. Hence, future multicenter prospective clinical studies are necessary, and further experimental research is required to explore the underlying mechanisms for the association between the identified CRGs and the prognosis of GC patients.
Conclusions
We successfully developed and validated a novel GC prognostic model, which may act as a reliable, efficient tool for prognostic prediction and treatment guidance in future clinical practice.
Acknowledgments
Funding: This work was supported by
Footnote
Reporting Checklist: The authors have completed the TRIPOD reporting checklist. Available at https://tcr.amegroups.com/article/view/10.21037/tcr-23-628/rc
Peer Review File: Available at https://tcr.amegroups.com/article/view/10.21037/tcr-23-628/prf
Conflicts of Interest: All authors have completed the ICMJE uniform disclosure form (available at https://tcr.amegroups.com/article/view/10.21037/tcr-23-628/coif). The authors have no conflicts of interest to declare.
Ethical Statement: The authors are accountable for all aspects of the work in ensuring that questions related to the accuracy or integrity of any part of the work are appropriately investigated and resolved. The study was conducted in accordance with the Declaration of Helsinki (as revised in 2013).
Open Access Statement: This is an Open Access article distributed in accordance with the Creative Commons Attribution-NonCommercial-NoDerivs 4.0 International License (CC BY-NC-ND 4.0), which permits the non-commercial replication and distribution of the article with the strict proviso that no changes or edits are made and the original work is properly cited (including links to both the formal publication through the relevant DOI and the license). See: https://creativecommons.org/licenses/by-nc-nd/4.0/.
References
- Sung H, Ferlay J, Siegel RL, et al. Global Cancer Statistics 2020: GLOBOCAN Estimates of Incidence and Mortality Worldwide for 36 Cancers in 185 Countries. CA Cancer J Clin 2021;71:209-49. [Crossref] [PubMed]
- Li Z, Gao X, Peng X, et al. Multi-omics characterization of molecular features of gastric cancer correlated with response to neoadjuvant chemotherapy. Sci Adv 2020;6:eaay4211. [Crossref] [PubMed]
- Song Z, Wu Y, Yang J, et al. Progress in the treatment of advanced gastric cancer. Tumour Biol 2017;39:1010428317714626. [Crossref] [PubMed]
- Kawazoe A, Shitara K, Boku N, et al. Current status of immunotherapy for advanced gastric cancer. Jpn J Clin Oncol 2021;51:20-7. [Crossref] [PubMed]
- Sasako M, Inoue M, Lin JT, et al. Gastric Cancer Working Group report. Jpn J Clin Oncol 2010;40:i28-37. [Crossref] [PubMed]
- Zhao E, Zhou C, Chen S. Prognostic nomogram based on log odds of positive lymph nodes for gastric carcinoma patients after surgical resection. Future Oncol 2019;15:4207-22. [Crossref] [PubMed]
- Nakamura Y, Yamanaka T, Chin K, et al. Survival Outcomes of Two Phase 2 Studies of Adjuvant Chemotherapy with S-1 Plus Oxaliplatin or Capecitabine Plus Oxaliplatin for Patients with Gastric Cancer After D2 Gastrectomy. Ann Surg Oncol 2019;26:465-72. [Crossref] [PubMed]
- Riihilä P, Nissinen L, Knuutila J, et al. Complement System in Cutaneous Squamous Cell Carcinoma. Int J Mol Sci 2019;20:3550. [Crossref] [PubMed]
- Geller A, Yan J. The Role of Membrane Bound Complement Regulatory Proteins in Tumor Development and Cancer Immunotherapy. Front Immunol 2019;10:1074. [Crossref] [PubMed]
- Li C, Liu T, Liu Y, et al. Prognostic value of tumour microenvironment-related genes by TCGA database in rectal cancer. J Cell Mol Med 2021;25:5811-22. [Crossref] [PubMed]
- Pio R, Ajona D, Ortiz-Espinosa S, et al. Complementing the Cancer-Immunity Cycle. Front Immunol 2019;10:774. [Crossref] [PubMed]
- Chen SMY, Krinsky AL, Woolaver RA, et al. Tumor immune microenvironment in head and neck cancers. Mol Carcinog 2020;59:766-74. [Crossref] [PubMed]
- Wei F, Wang D, Wei J, et al. Metabolic crosstalk in the tumor microenvironment regulates antitumor immunosuppression and immunotherapy resisitance. Cell Mol Life Sci 2021;78:173-93. [Crossref] [PubMed]
- Lu Y, Wu T, Sheng Y, et al. Correlation between Claudin-18 expression and clinicopathological features and prognosis in patients with gastric cancer. J Gastrointest Oncol 2020;11:1253-60. [Crossref] [PubMed]
- Yuan K, Ye J, Liu Z, et al. Complement C3 overexpression activates JAK2/STAT3 pathway and correlates with gastric cancer progression. J Exp Clin Cancer Res 2020;39:9. [Crossref] [PubMed]
- Chen J, Li GQ, Zhang L, et al. Complement C5a/C5aR pathway potentiates the pathogenesis of gastric cancer by down-regulating p21 expression. Cancer Lett 2018;412:30-6. [Crossref] [PubMed]
- Usui G, Matsusaka K, Mano Y, et al. DNA Methylation and Genetic Aberrations in Gastric Cancer. Digestion 2021;102:25-32. [Crossref] [PubMed]
- Cohen JI, Roychowdhury S, McMullen MR, et al. Complement and alcoholic liver disease: role of C1q in the pathogenesis of ethanol-induced liver injury in mice. Gastroenterology 2010;139:664-74, 674.e1.
- Ricklin D, Hajishengallis G, Yang K, et al. Complement: a key system for immune surveillance and homeostasis. Nat Immunol 2010;11:785-97. [Crossref] [PubMed]
- Roumenina LT, Daugan MV, Petitprez F, et al. Context-dependent roles of complement in cancer. Nat Rev Cancer 2019;19:698-715. [Crossref] [PubMed]
- Jiang H, Wang B, Zhang F, et al. The Expression and Clinical Outcome of pCHK2-Thr68 and pCDC25C-Ser216 in Breast Cancer. Int J Mol Sci 2016;17:1803. [Crossref] [PubMed]
- Wang XQ, Terry PD, Li Y, et al. Association of XPG rs2094258 polymorphism with gastric cancer prognosis. World J Gastroenterol 2019;25:5152-61. [Crossref] [PubMed]
- Fuchs CS, Doi T, Jang RW, et al. Safety and Efficacy of Pembrolizumab Monotherapy in Patients With Previously Treated Advanced Gastric and Gastroesophageal Junction Cancer: Phase 2 Clinical KEYNOTE-059 Trial. JAMA Oncol 2018;4:e180013. [Crossref] [PubMed]
- Kang YK, Boku N, Satoh T, et al. Nivolumab in patients with advanced gastric or gastro-oesophageal junction cancer refractory to, or intolerant of, at least two previous chemotherapy regimens (ONO-4538-12, ATTRACTION-2): a randomised, double-blind, placebo-controlled, phase 3 trial. Lancet 2017;390:2461-71. [Crossref] [PubMed]
- Lee JM, Lee MH, Garon E, et al. Phase I Trial of Intratumoral Injection of CCL21 Gene-Modified Dendritic Cells in Lung Cancer Elicits Tumor-Specific Immune Responses and CD8(+) T-cell Infiltration. Clin Cancer Res 2017;23:4556-68. [Crossref] [PubMed]
- Hegde PS, Karanikas V, Evers S. The Where, the When, and the How of Immune Monitoring for Cancer Immunotherapies in the Era of Checkpoint Inhibition. Clin Cancer Res 2016;22:1865-74. [Crossref] [PubMed]
- Wang Y, Fang T, Huang L, et al. Neutrophils infiltrating pancreatic ductal adenocarcinoma indicate higher malignancy and worse prognosis. Biochem Biophys Res Commun 2018;501:313-9. [Crossref] [PubMed]
- Margetts J, Ogle LF, Chan SL, et al. Neutrophils: driving progression and poor prognosis in hepatocellular carcinoma? Br J Cancer 2018;118:248-57. [Crossref] [PubMed]
- Li S, Cong X, Gao H, et al. Tumor-associated neutrophils induce EMT by IL-17a to promote migration and invasion in gastric cancer cells. J Exp Clin Cancer Res 2019;38:6. [Crossref] [PubMed]
- Melaiu O, Chierici M, Lucarini V, et al. Cellular and gene signatures of tumor-infiltrating dendritic cells and natural-killer cells predict prognosis of neuroblastoma. Nat Commun 2020;11:5992. [Crossref] [PubMed]
- Zhang B, Wu Q, Li B, et al. m(6)A regulator-mediated methylation modification patterns and tumor microenvironment infiltration characterization in gastric cancer. Mol Cancer 2020;19:53. [Crossref] [PubMed]
- Bezdenezhnykh N, Semesiuk N, Lykhova O, et al. Impact of stromal cell components of tumor microenvironment on epithelial-mesenchymal transition in breast cancer cells. Exp Oncol 2014;36:72-8. [PubMed]
- Chowell D, Morris LGT, Grigg CM, et al. Patient HLA class I genotype influences cancer response to checkpoint blockade immunotherapy. Science 2018;359:582-7. [Crossref] [PubMed]
- Schaafsma E, Fugle CM, Wang X, et al. Pan-cancer association of HLA gene expression with cancer prognosis and immunotherapy efficacy. Br J Cancer 2021;125:422-32. [Crossref] [PubMed]
- Barrios DM, Do MH, Phillips GS, et al. Immune checkpoint inhibitors to treat cutaneous malignancies. J Am Acad Dermatol 2020;83:1239-53. [Crossref] [PubMed]
- Tong X, Yang X, Tong X, et al. Complement system-related genes in stomach adenocarcinoma: Prognostic signature, immune landscape, and drug resistance. Front Genet 2022;13:903421. [Crossref] [PubMed]
- Yang JD, Ma L, Zhu Z. SERPINE1 as a cancer-promoting gene in gastric adenocarcinoma: facilitates tumour cell proliferation, migration, and invasion by regulating EMT. J Chemother 2019;31:408-18. [Crossref] [PubMed]
- Kuemmerle NB, Rysman E, Lombardo PS, et al. Lipoprotein lipase links dietary fat to solid tumor cell proliferation. Mol Cancer Ther 2011;10:427-36. [Crossref] [PubMed]
- Pascual G, Avgustinova A, Mejetta S, et al. Targeting metastasis-initiating cells through the fatty acid receptor CD36. Nature 2017;541:41-5. [Crossref] [PubMed]
- Chen CN, Lin JJ, Chen JJ, et al. Gene expression profile predicts patient survival of gastric cancer after surgical resection. J Clin Oncol 2005;23:7286-95. [Crossref] [PubMed]
- Pan J, Fan Z, Wang Z, et al. CD36 mediates palmitate acid-induced metastasis of gastric cancer via AKT/GSK-3β/β-catenin pathway. J Exp Clin Cancer Res 2019;38:52. [Crossref] [PubMed]
- Chong PK, Lee H, Loh MC, et al. Upregulation of plasma C9 protein in gastric cancer patients. Proteomics 2010;10:3210-21. [Crossref] [PubMed]
- Lichtenfeld JL, Wiernik PH, Mardiney MR Jr, et al. Abnormalities of complement and its components in patients with acute leukemia, Hodgkin's disease, and sarcoma. Cancer Res 1976;36:3678-80. [PubMed]
- Luo Y, Wang X, Ma L, et al. Bioinformatics analyses and biological function of lncRNA ZFPM2-AS1 and ZFPM2 gene in hepatocellular carcinoma. Oncol Lett 2020;19:3677-86. [Crossref] [PubMed]
- Law RH, Abu-Ssaydeh D, Whisstock JC. New insights into the structure and function of the plasminogen/plasmin system. Curr Opin Struct Biol 2013;23:836-41. [Crossref] [PubMed]
- Bharadwaj AG, Holloway RW, Miller VA, et al. Plasmin and Plasminogen System in the Tumor Microenvironment: Implications for Cancer Diagnosis, Prognosis, and Therapy. Cancers (Basel) 2021;13:1838. [Crossref] [PubMed]
- Fang S, Hong H, Li L, et al. Plasminogen kringle 5 suppresses gastric cancer via regulating HIF-1α and GRP78. Cell Death Dis 2017;8:e3144. [Crossref] [PubMed]
- Hamm A, Veeck J, Bektas N, et al. Frequent expression loss of Inter-alpha-trypsin inhibitor heavy chain (ITIH) genes in multiple human solid tumors: a systematic expression analysis. BMC Cancer 2008;8:25. [Crossref] [PubMed]
- Ohyama K, Yoshimi H, Aibara N, et al. Immune complexome analysis reveals the specific and frequent presence of immune complex antigens in lung cancer patients: A pilot study. Int J Cancer 2017;140:370-80. [Crossref] [PubMed]