Machine-learning methods based on the texture and non-texture features of MRI for the preoperative prediction of sentinel lymph node metastasis in breast cancer
Highlight box
Key findings
• A non-invasive and efficient prediction model to predict sentinel lymph node (SLN) metastasis based on the image features of magnetic resonance imaging in breast cancer patients was constructed.
What is known and what is new?
• The accurate prediction model can be established using the method of radiomics.
• The prediction model with combination of minimum redundancy maximum relevance and random forest could facilitate clinical prediction of SLN metastasis for patients with breast cancer.
What is the implication, and what should change now?
• More collection of magnetic field inhomogeneity of diffusion-weighted images and more complex machine learning methods should be included in future research.
Introduction
Breast cancer is the most frequently diagnosed cancer and the leading type of cancer among females worldwide, accounting for 24.5% of newly diagnosed female cancers in 2020 (1). An early diagnosis is key to the successful treatment of breast cancer (1,2). The axillary lymph node (ALN) status, a crucial prognostic factor for breast cancer, guides decision-making regarding treatment modalities (3); thus, the determination of the ALN status is important to the correct staging of breast cancer patients (4,5). The sentinel lymph node (SLN) is the first lymph node that receives lymphatic drainage from the tumor and it can predict the ALN status accurately (6). Therefore, SLN biopsy (SLNB) has been introduced as an alternative method to screening the ALN for metastasis, especially for early-stage breast cancer (7-10). Nevertheless, some work presented the morbidity associated with the invasive SLNB and highlighted the fact that the procedure inevitably has complications (11,12). The accurate non-invasive detection method of SLN metastasis is meaningful. Several studies have reported that clinical and histopathological data provides predictive information for SLN metastasis; but some predictive information is just obtained after operation, thereby failing to guide SLN detection (13-16). Therefore, a new preoperative method for SLN detection has been proposed based on contrast-enhanced ultrasonography with sonazoid in breast cancer; however, this method has only been applied to a small cohort, and its accuracy (ACC) and practicality remains controversial (17,18).
Medical images, changing rapidly from being primarily a diagnostic tool to playing a key role in precision medicine, providing a feasible non-invasive way to decode tumor pathophysiology by high-throughput extraction of quantitative features that transform visual images into quantifiable information (19). Typically, some studies have reported that the quantitative features derived from medical images are associated with clinical features, including histology, grades or stages of cancer, patient survival, and metastases (20-23). Several papers have explored the potential association between gene expression pattern and quantitative features (24,25). Three steps are involved in this type of analysis: region of interest (ROI) segmentation, feature extraction, and classifier modeling. The segmentation of ROIs is usually performed manually by radiologists, and the auto segmentation is still a challenge due to the indistinct borders of many tumors. The high-throughput extraction of quantitative features is imperative, and image processing technologies have provided a series of feature extraction algorithms for quantizing tumor heterogeneity (26). A high-performance classifier is then required to help making clinical decision. In general, to achieve better classification results, the feature selection approach is employed to reduce the dimension of the features space. Consequently, as a research hotspot, machine learning offers numerous feature selection operators; it is divided into three main categories, namely, filter, wrapper, and embedded methods (27,28). The performance of feature selection is entwined with classification method (29,30).
In this study, we aimed to provide a non-invasive and efficient way to predict SLN metastasis in breast cancer patients. We built a prediction model, which consisted of feature extraction, feature selection and classification modules. Figure 1 shows the framework of our study. To achieve the optimal combination of feature selection and classification methods, we extensively evaluated different combination of 24 feature selection and 11 classification methods in terms of their average performance and stability. Moreover, to explore predictive performance of feature selection and classification method separately, we independently compared feature selection and classification methods. Feature selection and classification methods were independently compared. We present this article in accordance with the TRIPOD reporting checklist (available at https://tcr.amegroups.com/article/view/10.21037/tcr-22-2534/rc).
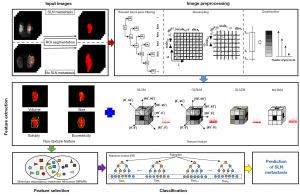
Methods
Patients
This study does not need Institutional Review Board (IRB) approval due to the purpose of retrospective study and was conducted in accordance with the Declaration of Helsinki (as revised in 2013). The individual consent for this retrospective analysis was waived. A total of 172 patients were retrospectively reviewed from March 2014 to June 2016. SLN metastasis (n=74) and non-SLN metastasis (n=98) in breast cancer patients had been histologically confirmed. All enrolled patients underwent diffusion-weighted imaging (DWI) magnetic resonance imaging (MRI) scan. The baseline characteristics of enrolled patients are listed in Table 1. The ROIs were defined as the whole single breast tumor. To reduce the perturbance brought by random dataset partition, motivated by the approach proposed by Haury et al. (30), 172 patients were enrolled in total in this work. In order to investigate the stability of feature selection-classification combinations and eliminate the influence of data division, the 172 patients were randomly divided into 50 subsets with 138 patients (80% of the enrolled patients). For each subset, 10-fold cross-validation was used to evaluate the model. Specifically, each subset (138 patients) was randomly divided into 10 folds, where nine folds (124 patients) were used to develop prediction model and the rest one (14 patients) was used to evaluate the model in sequence. The patients with SLN metastasis and non-SLN metastasis were labeled as 1 and 0, respectively. The ratio of SLN metastasis to non-SLN metastasis was the same in all subsets.
Table 1
Characteristics | Non-SLN metastasis group (n=98) | SLN metastasis group (n=74) |
---|---|---|
Age (years) | 47.10±11.0 | 48.0±10.2 |
Histological grade | ||
I | 14 (14.3) | 3 (4.1) |
II | 35 (35.7) | 27 (36.5) |
III | 42 (42.9) | 25 (33.8) |
Other | 7 (7.1) | 19 (25.7) |
ER status | ||
Negative | 23 (23.5) | 5 (6.8) |
1+ | 14 (14.3) | 8 (10.8) |
2+ | 12 (12.2) | 10 (13.5) |
3+ | 42 (42.9) | 32 (43.2) |
Other | 7 (7.1) | 19 (25.7) |
PR status | ||
Negative | 19 (19.4) | 5 (6.8) |
1+ | 24 (24.5) | 16 (21.6) |
2+ | 9 (9.2) | 8 (10.8) |
3+ | 39 (39.8) | 26 (35.1) |
Other | 7 (7.1) | 19 (25.7) |
cerbB-2 | ||
Negative | 22 (22.4) | 10 (13.5) |
1+ | 19 (19.4) | 13 (17.6) |
2+ | 28 (28.6) | 21 (28.4) |
3+ | 22 (22.4) | 11 (14.9) |
Other | 7 (7.1) | 19 (25.7) |
HER2 status | ||
Positive | 26 (26.5) | 16 (21.6) |
Negative | 65 (66.3) | 39 (52.7) |
Other | 7 (7.1) | 19 (25.7) |
Ki-67 (%) | 34.0±25.0 | 28.1±19.7 |
ADC value | 0.88±0.25 | 0.83±0.20 |
Data are presented as mean ± SD or n (%). Other means that information absence or other types. SLN, sentinel lymph node; ER, estrogen receptor; PR, progesterone receptor; HER2, human epidermal growth factor receptor 2; ADC, apparent diffusion coefficient; SD, standard deviation.
Imaging data acquisition
MRI was performed by using a 1.5-T MR imager (Achieva 1.5 T, Philips Healthcare, Best, Netherlands) equipped with a 4-channel SENSE breast coil. The diffusion-weighted (DW) images were acquired by single-shot spin-echo echo-planar imaging (EPI) and recorded by the picture archiving and communication system (PACS). The data acquisition parameters were as follows: resolution, 200 pixels × 196 pixels; field of volume, 300×300 mm2; time of repetition/time of echo (TR/TE), 5,065/66 ms; slice thickness, 5 mm; slice gap, 1 mm; b values, 0 and 1,000 s/mm2.
ROI segmentation
Segmentation of ROIs is required before quantitative feature extraction. The DWI Digital Imaging and Communications in Medicine (DICOM) images that had been archived in the PACS were transmitted to the radiologists without any pathological information and preprocessing. ITKSNAP 3.6 (ITK-SNAP 3.xTeam) was used by the radiologists for three-dimensional (3D) manual segmentation. Specifically, the radiologists first delineated the margin of tumor at each transverse plane, covered the whole tumor gradually, and repeated the above-mention procedure slice by slice. An example of segmentation is shown in the upper left of Figure 1. All manual segmentation was performed by a radiologist with 15 years of experience and was validated by a senior radiologist with 20 years of experience. A total of two radiologists involved in this work. And a radiologist with 15 years of experience performed manual segmentation, followed by a senior radiologist with 20 years of experience to validate and fine-tune the segmented results.
Image preprocessing and feature extraction
The preprocessing of ROIs is necessary, because the DWI images of different patients have different scan parameters (31,32). In the current study, a series of preprocessing methods were preformed, including: (I) wavelet bandpass filtering, aiming to denoise the noise in ROIs and focus on different bandwidth information, and the operator was performed by setting different weights to the bandpass or sub-bands of the ROIs in the wavelet domain; (II) isotropic resampling, aiming to keep rotation invariance and normalize the pixel and thickness, and the operator was carried out by cube interpolation to an appropriate resolution; and (III) quantization of gray level, aiming to normalize the different gray level of images affecting feature extraction, and the operator was performed by using equal-probability and Lloyd-Max quantization algorithms (33).
A series of texture and non-texture features were extracted from ROIs. Texture feature extraction was based on statistical distributions, including: (I) global features [dimension (D) =3], describe the histogram distribution of the ROIs intensity; (II) gray-level co-occurrence matrix (GLCM, D =9), depicting the statistical interrelationships between voxels of ROIs; (III) gray-level run-length matrix (GLRLM, D =13) and gray-level size zone matrix (GLSZM, D =13), computing the statistical interrelationship of neighboring voxels along a longitudinal run and the statistical distribution of similar and dissimilar regions; and (IV) neighborhood gray-tone difference matrix (NGTDM, D =5), quantifying the spatial interrelationship of neighboring voxels between adjacent image planes (34,35). Notably, the same preprocessing procedure with different parameters resulted in different features. As shown in Table 2, different parameters were used in the same procedure to enrich the texture features. Finally, (3+9+13+13+5)×(5×6×2×4)=10,320 enhanced texture features were obtained.
Table 2
Parameters name | Values | Number of parameters |
---|---|---|
Wavelet band-pass filtering | Weight = [1/2, 2/3, 1, 3/2, 2] | 5 |
Isotropic voxel size | Scale = {in-pR, 1, 2, 3, 4, 5} | 6 |
Quantization algorithm | Quan algorithm = {Equal, Lloyd} | 2 |
Number of gray levels | Ng† = [8, 16, 32, 64] | 4 |
†, Ng denotes gray levels respectively. in-pR, initial in-plane resolution.
In addition, non-texture features were extracted to depict intuitional and simple image information, including: (I) volume, computed by the number of voxels in the ROIs multiplied by the dimension of voxels; (II) size, obtained by measuring the longest diameter of ROI; (III) solidity, the ratio of the number of voxels in the ROIs to the number of voxels in the 3D convex hull of the ROIs; and (IV) eccentricity, obtained by measuring the eccentricity of the ellipsoid that best fits the ROIs. All used features in our work are listed in Table 3. Totally, 10,320 texture features and four non-texture features were extracted from ROIs. Afterward, a linear normalization operator minimum–maximum method was used to eliminate the magnitude of features and negative effects of large magnitude difference.
Table 3
Type of texture | Parameters |
---|---|
First order | |
Global (D† =3) | Variance (No. 1), skewness (No. 2), kurtosis (No. 3) |
Second order | |
GLCM (D =9) | Energy (No. 4), contrast (No. 5), correlation (No. 6), homogeneity (No. 7), variance (No. 8), sum average (No. 9), entropy (No. 10), dissimilarity (No. 11), auto-correlation (No. 12) |
High order | |
GLRLM (D =13) | Short run emphasis (No. 13), long run emphasis (No. 14), gray-level nonuniformity (No. 15), run-length nonuniformity (No. 16), run percentage (No. 17), low gray-level run emphasis (No. 18), high gray-level run emphasis (No. 19), short run low gray-level emphasis (No. 20), short run high gray-level emphasis (No. 21), long run low gray-level emphasis (No. 22), long run high gray-level emphasis (No. 23), gray-level variance (No. 24), run-length variance (No. 25) |
GLZSM (D =13) | Small zone emphasis (No. 26), large zone emphasis (No. 27), gray-level nonuniformity (No. 28), zone-size nonuniformity (No. 29) zone percentage (No.30), low gray-level zone emphasis (No. 31), high gray-level zone emphasis (No. 32), small zone low gray-level emphasis (No. 33), small zone high gray-level emphasis (No. 34), large zone low gray-level emphasis (No. 35), large zone high gray-level emphasis (No. 36), gray-level variance (No. 37), zone-size variance (No. 38) |
NGTDM (D =5) | Coarseness (No. 39), contrast (No. 40), busyness (No. 41), complexity (No. 42), strength (No. 43) |
†, D denotes dimension of feature space. The number inside parenthesis denotes the feature number. GLCM, gray-level co-occurrence matrix; GLRLM, gray-level run-length matrix; GLZSM, gray-level size zone matrix; NGTDM, neighborhood gray-tone difference matrix.
Establishment of the optimal predictive model for SLN metastasis
As shown in Figure 1, the predictive model for SLN metastasis also included feature selection and classification modeling.
Feature selection methods
Feature selection can efficiently improve the performance of classification by eliminating redundant and irrelevant features. In general, feature selection methods are classified into three categories, namely, filter, wrapper, and embedded methods (36,37). Filter methods rank all the features in terms of their relevance scores based on their correlations with the class label, and choose an appropriate feature subset. Wrapper methods directly search for the feature subset with the optimal predictive performance for a given classification method. Embedded methods perform feature selection during classifier training to select stable and sparse features based on some strategies such as bootstrap and regularization. To compare different feature selection methods, 24 representative methods [including Las Vegas wrapper (LVW), sequential forward floating selection (SFFS), minimum redundancy maximum relevance (MRMR), and so on] were selected from the three categories. The abbreviations of all feature selection methods and their category are listed in Table 4.
Table 4
Feature selection method acronym | Feature selection method name | Classification method acronym | Classification method name |
---|---|---|---|
LVW† | Las Vegas wrapper | BAG | Bagging |
SFFS† | Sequential forward floating selection | BAYES | Naive bayes |
SFS† | Sequential forward selection | BST | Boosting |
RF‡ | Random forest | L-DA | Linear discriminant analysis |
RFE‡ | Recursive feature elimination | DT | Decision tree |
L1-SVM‡ | L1 regularization based on SVM | GLM | Generalized linear model |
L2-SVM‡ | L2 regularization based on SVM | K-NN | k-nearest neighbor |
CHSQ§ | Chi-square score | SVM | Support vector machine |
CIFE§ | Conditional infomax feature extraction | MARS | Multi-adaptive regression splines |
CMIM§ | Conditional mutual information maximization | PLSR | Partial least squares regression |
DISR§ | Double input symmetric relevance | RF | Random forest |
DC§ | Distance correlation | ||
FSCR§ | Fisher score | ||
GINI§ | Gini index | ||
ICAP§ | Interaction capping | ||
ILFS§ | Infinite latent feature selection | ||
JMI§ | Joint mutual information | ||
LS§ | Laplacian score | ||
MIFS§ | Mutual information feature selection | ||
MIM§ | Mutual information maximization | ||
MRMR§ | Minimum redundancy maximum relevance | ||
RELF§ | Relieff | ||
SIS§ | Sure independence screening | ||
TSCR§ | T-score |
Classification methods
Different classification methods with various complexity affect the performance of model directly. Therefore, 11 classifications methods [including boosting (BST), decision tree (DT), random forest (RF), etc.] were extensively investigated. The abbreviations of all classification methods are also listed in Table 4. Most parameters in the classification methods were selected by 10-fold cross-validation in each training set, whereas others were chosen based on fine-tuning default parameters in all methods.
Optimal feature selection—classification combination analysis
The optimal combination of feature selection and classification methods in terms of their predictive performance and stability were first identified. The feature selection and classification were then compared independently to explore predictive performance of feature selection and classification method separately.
Predictive performances of feature selection-classification combinations
In this paper, the predictive performances of the feature selection and classification methods were compared by cross-combination. The features selected by each feature selection method were subsequently transferred to the classification method. The predictive performance of each combination for each subset was evaluated using area under the curve (AUC) and ACC. The aforementioned process was repeated to the 50 subsets. The final performance of any combination was assessed in terms of average values of AUC and ACC over 50 subsets.
Stability of feature selection-classification combinations
The stability of combination of feature selection and classification method is mainly caused by the perturbance of random dataset partition. In addition to perturbance of dataset, choice of feature selection and classification method is also an important factor for stability of feature selection and classification category. To quantify stability, the relative standard deviation (RSD) was used to assess the feature selection and classification methods. RSD is the absolute value of the ratio of standard deviation (SD) to average, and it is defined as:
where σAUC and µAUC are the SD and average of AUC, respectively. It indicates that a lower value means more stability. For each combination of feature selection and classification method, AUCsubset was first obtained over 10-fold at each subset, and then σAUC and µAUC were computed based on AUCsubset over the 50 subsets.
Obviously, combination of feature selection and classification methods with the highest prediction performance and the best stability is the optimal model for prediction of SLN metastasis in breast cancer.
Performance analysis based on feature selection and classification method respectively
The predictive performance of feature selection and classification methods were further compared separately. For each feature selection method, the average AUC and ACC were computed over the 11 classification methods. Meanwhile, the stability of feature selection methods and classification methods were investigated separately. The RSD of each feature selection method and classification method was also calculated. As to each feature selection method, σAUC and µAUC were yielded over the 11 classification methods. Correspondingly, to each classification method, σAUC and µAUC were yielded over the 24 feature selection methods.
Statistical analysis
All the analyses were implemented using R software 3.4.2 (R Core Team, Vienna, Austria) and MATLAB 2016b (MathWorks, Natick, MA, USA).
Results
Predictive performances of feature selection-classification combinations
AUC and ACC were used to quantify the predictive performances of the cross-combinations of 24 feature selection and 11 classification methods. In total, 264 different combinations were assessed in this study. Figures 2,3 showed the specific AUC and ACC values. The results show that MRMR + RF exhibited the optimal predictive performance [(AUC: 0.97±0.03; range: 0.95–1.00) and (ACC: 0.89±0.05; range: 0.86–0.93)], followed by chi-square score (CHSQ) + RF [(AUC: 0.95±0.04; range: 0.94–0.98) and (ACC: 0.89±0.05; range: 0.86–0.93)] and L2-support vector machine (L2-SVM) + RF [(AUC: 0.94±0.05; range: 0.91–0.98) and (ACC: 0.87±0.05; range: 0.86–0.93)]. The confusion matrix of three feature selection-classification combinations with good model performance (i.e., MRMR + RF, CHSQ + RF, and L2-SVM + RF) are shown in Figure 4, which contained the testing results in all subsets. The three methods could correctly predict most of the positive and negative classes, and the positive samples were relatively easier to classify compared with the negative ones.
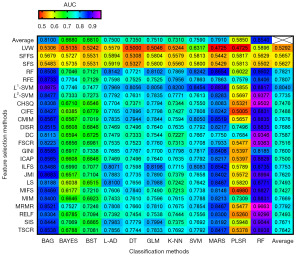
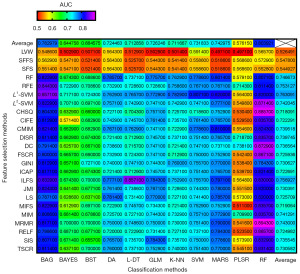
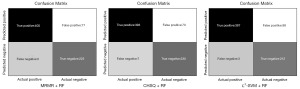
Stability of the feature selection-classification combinations
The RSD was computed to assess the stability of the different combination models. As Figure 5 shown, MRMR + RF exhibited the best stability (stability: 2.94), followed by DC + RF (stability: 3.96) and CHSQ + RF (stability: 3.97).
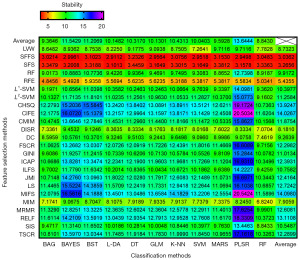
Performance analysis based on feature selection and classification method respectively
For the average performance of prediction made by feature selection and classification method separately, as the top and the far right of Figures 2,3 shown, L1-SVM selection methods [(AUC: 0.80±0.08; range: 0.59–0.90) and (ACC: 0.76±0.07; range: 0.56–0.86)] and RF classifier [(AUC: 0.85±0.11; range: 0.55–0.97) and (ACC: 0.80±0.09; range: 0.55–0.89)] showed the best predictive performance among their respective categories. As to the average stability made by feature selection methods and classification methods separately, it can be observed from the top and the far right of Figure 5 that SFFS (stability: 3.04) and RF (stability: 8.84) showed the optimal stability among their respective categories. Overall, in addition to the RF classifier, bootstrap aggregating (BAG) was also a well-performed ensemble method [(AUC: 0.81±0.10; range: 0.53–0.89), (ACC: 0.78±0.09; range: 0.54–0.85), and (stability: 9.36)], followed by multi-adaptive regression splines (MARS) [(AUC: 0.79±0.10; range: 0.47–0.88), (ACC: 0.74±0.09; range: 0.49–0.82), and (stability: 9.59)], while SVM, generalize linear model (GLM), linear discriminant analysis (L-DA), k-nearest neighbor (KNN), and DT [(average AUC range: 0.73–0.76), (average ACC range: 0.71–0.73), and (stability range: 10.04–10.43)] obtained similar model performance. In contrast, binary search tree (BST), naive bayes (BAYES), and partial least squares regression (PLSR) [(average AUC range: 0.59–0.68), (average ACC range: 0.58–0.66), and (stability range: 11.21–13.64)] had relatively inferior results.
Contributing features based on feature selection methods
The top 5 contributing features obtained by each feature selection method are shown in Table 5. All the best performed contributing features were texture features, probably because the non-texture features did not contain as much abundant information as the texture ones. The five most common features among all 24 feature selection methods were small zone emphasis (No. 26), large zone low gray-level emphasis (No. 35), small zone high gray-level emphasis (No. 34), zone percentage (No. 30), and zone-size nonuniformity (No. 29).
Table 5
Feature selection method acronym | Feature 1 | Feature 2 | Feature 3 | Feature 4 | Feature 5 |
---|---|---|---|---|---|
LVW† | No. 24 [209] | No. 30 [15] | No. 14 [52] | No. 37 [237] | No. 43 [36] |
SFFS† | No. 26 [39] | No. 26 [63] | No. 26 [85] | No. 32 [110] | No. 26 [157] |
SFS† | No. 26 [154] | No. 26 [14] | No. 26 [10] | No. 32 [5] | No. 26 [49] |
RF‡ | No. 43 [73] | No. 34 [138] | No. 31 [142] | No. 27 [12] | No. 30 [14] |
RFE‡ | No. 34 [89] | No. 28 [17] | No. 31 [165] | No. 34 [173] | No. 28 [225] |
L1-SVM‡ | No. 1 [241] | No. 38 [1] | No. 34 [173] | No. 33 [105] | No. 33 [14] |
L2-SVM‡ | No. 29 [229] | No. 34 [89] | No. 31 [165] | No. 26 [229] | No. 28 [17] |
CHSQ§ | No. 36 [142] | No. 36 [71] | No. 36 [23] | No. 26 [98] | No. 29 [146] |
CIFE§ | No. 35 [8] | No. 35 [47] | No. 35 [24] | No. 35 [95] | No. 35 [96] |
CMIM§ | No. 12 [126] | No. 43 [169] | No. 22 [19] | No. 20 [24] | No. 41 [27] |
DISR§ | No. 12 [126] | No. 10 [150] | No. 34 [104] | No. 16 [200] | No. 13 [200] |
DC§ | No. 38 [14] | No. 38 [62] | No. 25 [105] | No. 38 [110] | No. 28 [177] |
FSCR§ | No. 26 [105] | No. 26 [159] | No. 26 [14] | No. 29 [111] | No. 29 [159] |
GINI§ | No. 12 [1] | No. 10 [3] | No. 34 [2] | No. 16 [1] | No. 13 [4] |
ICAP§ | No. 15 [126] | No. 15 [150] | No. 9 [104] | No. 28 [200] | No. 19 [200] |
ILFS§ | No. 28 [5] | No. 29 [5] | No. 30 [5] | No. 31 [5] | No. 32 [5] |
JMI§ | No. 23 [143] | No. 32 [56] | No. 32 [72] | No. 9 [143] | No. 23 [144] |
LS§ | No. 30 [128] | No. 30 [31] | No. 30 [72] | No. 30 [120] | No. 30 [135] |
MIFS§ | No. 35 [8] | No. 35 [24] | No. 35 [32] | No. 35 [40] | No. 35 [47] |
MIM§ | No. 12 [126] | No. 10 [150] | No. 34 [104] | No. 16 [200] | No. 13 [200] |
MRMR§ | No. 26 [77] | No. 38 [110] | No. 26 [207] | No. 27 [210] | No. 29 [54] |
RELF§ | No. 34 [61] | No. 19 [34] | No. 32 [42] | No. 34 [10] | No. 29 [206] |
SIS§ | No. 26 [159] | No. 29 [159] | No. 26 [105] | No. 26 [14] | No. 26 [63] |
TSCR§ | No. 35 [196] | No. 35 [204] | No. 35 [212] | No. 35 [228] | No. 35 [236] |
Total | No. 26 | No. 35 | No. 34 | No. 30 | No. 29 |
†, the feature selection method from wrapper category; ‡, the feature selection method from embedded category; §, the feature selection method from filter category. The bottom row shows five most common features among all feature selection methods. The number out of square bracket denotes the feature number in Table 3. The number inside square bracket denotes combination of parameters in Table 2. The abbreviations of feature selection methods were defined in Table 4.
Discussion
Key findings
Recent studies have proven that SLNB is an alternative method for ALN metastasis detection (7,8,10). However, biopsy can bring discomfort and injury to patients, such as pain, bleeding, infection, etc. (11,12). Therefore, it is of great importance to develop a non-invasive SLN metastasis detection method. So far, machine-learning based approaches have been applied in medical image-based auxiliary diagnostic studies for varied cancers (20,23,34), histology (36,37), survival prediction (24,38), and so on. In this study, a machine learning framework for preoperative prediction of SLN metastasis in breast cancer was proposed with assessments of different selection and classification model. The findings suggested an optimal model of combination of MRMR feature selection and RF classification methods and showed a relatively high efficiency for prediction of SLN metastasis in breast cancer.
Strengths and limitations
The one limitation of our work is that our proposed model is lack of large and independent external validation. Therefore, we used 10-fold cross-validation to assess the performance of model, which is an efficient way to decrease the variance of performance due to random dataset partition, especially for the limited number of patients. On the one hand, multicenter and prospective patient collection should be proceeded; on the other hand, a more proper way to solve the limited data problem deserves further investigation. Another possible limitation of our study is that the effect of magnetic field inhomogeneity is not considered. And the DW image is sensitive to magnetic field inhomogeneity which might result in bias for the results.
Comparison with similar researches
The promising prediction performance partly benefited from the feature selection method that decreased the dimension of feature space by identifying a set of the most contributing features. During the feature selection process, the redundancy between features and the risk of model overfitting partly decreased. In addition, classification methods play a leading role based on the given feature set after feature selection, and classification methods from different families have their own operational mechanisms with discrepant performances (39).
Explanations of findings
The results of our experiments showed that the ensemble methods such as RF classifier and BAG could obtained outstanding performance, followed by MARS, while SVM, GLM, L-DA, KNN, and DT achieved general results. Meanwhile, BST, BAYES, and PLSR got inferior performance compared with others. The possible confounding factors leading to inferior performance might be that BST, BAYES, and KNN are relatively sensitive to noise and data distribution (40-42), PLSR and GLM relied on linear assumption and could not handle nonlinear problem, MARS, L-DA and DT were likely to suffer from overfitting (43,44), SVM was easily impacted by the selection of kernel function (45). Although RF and BAG might also be affected by noise interference and required more computational power, they combined multiple uncorrelated base models and made decision based on the majority of votes that helped improve the ACC (46), thus these two models were more suitable for the current study. It is worth noting that, from the machine learning point of view, different combinations of feature selection and classification methods would lead to differences in performance, particularly for a high-dimensional dataset. The current study widely studied and compared the performances and stability of cross-combinations of 24 typical feature selection and 11 classification methods from different categories. On the basis of predictive performance and stability for preoperative prediction of SLN metastasis in breast cancer, our study offered evidence that the combination MRMR + RF is the optimal model than other combinations. MRMR is a filter method based on mutual information. It selects a feature set with minimum redundancy and maximum relevance (47). The results suggested that the enhanced features might have more redundancy. RF classifier is a supervised learning classification method (39). This classifier consists of an ensemble of tree predictors, with each tree depending on the values of a random vector sampled independently. RF has ability to expose the hidden linear or nonlinear relationship between the feature and target. Its favorable performance has also been confirmed in several previous studies (20,34,48).
Performance for the categories of feature selection and classification method was also explored separately in this study. The results showed that L1-SVM and SFFS had the best average predictive performance and the stability among all feature selection methods, respectively. RF and SVM showed the best average predictive performance and the stability among all classification methods (49), respectively. These results indicated that L1-SVM and SFFS has better robustness for feature selection in general, and RF and SVM are better selection of classifiers compared with other approaches. In general, for similar tasks, such as metastasis of other lymph node, priority can be given to the above feature selection methods and classifiers.
Implications and actions needed
Therefore, more collection of magnetic field inhomogeneity of DW images should be included in future research. In addition, our work only identified the combination of traditional methods with a relatively high predictive and stable performance for preoperative prediction of SLN metastasis in breast cancer. In the future, we will study the influences of different parameters in image acquisition and feature extraction and use more complex machine learning methods, including deep learning methods based on patch strategy (34).
Conclusions
In conclusion, an optimal machine-learning model for preoperative prediction of SLN metastasis in breast cancer was established based on the image features of MRI. The combination of MRMR and RF suggested the best predictive efficiency. It could facilitate clinical prediction of SLN metastasis for patients with breast cancer.
Acknowledgments
Funding: This work was supported by
Footnote
Reporting Checklist: The authors have completed the TRIPOD reporting checklist. Available at https://tcr.amegroups.com/article/view/10.21037/tcr-22-2534/rc
Data Sharing Statement: Available at https://tcr.amegroups.com/article/view/10.21037/tcr-22-2534/dss
Conflicts of Interest: All authors have completed the ICMJE uniform disclosure form (available at https://tcr.amegroups.com/article/view/10.21037/tcr-22-2534/coif). The authors have no conflicts of interest to declare.
Ethical Statement: The authors are accountable for all aspects of the work in ensuring that questions related to the accuracy or integrity of any part of the work are appropriately investigated and resolved. This study does not need Institutional Review Board (IRB) approval due to the purpose of retrospective study and was conducted in accordance with the Declaration of Helsinki (as revised in 2013). The individual consent for this retrospective analysis was waived.
Open Access Statement: This is an Open Access article distributed in accordance with the Creative Commons Attribution-NonCommercial-NoDerivs 4.0 International License (CC BY-NC-ND 4.0), which permits the non-commercial replication and distribution of the article with the strict proviso that no changes or edits are made and the original work is properly cited (including links to both the formal publication through the relevant DOI and the license). See: https://creativecommons.org/licenses/by-nc-nd/4.0/.
References
- Sung H, Ferlay J, Siegel RL, et al. Global Cancer Statistics 2020: GLOBOCAN Estimates of Incidence and Mortality Worldwide for 36 Cancers in 185 Countries. CA Cancer J Clin 2021;71:209-49. [Crossref] [PubMed]
- Chlebowski RT, Manson JE, Anderson GL, et al. Estrogen plus progestin and breast cancer incidence and mortality in the Women's Health Initiative Observational Study. J Natl Cancer Inst 2013;105:526-35. [Crossref] [PubMed]
- Qiu PF, Liu JJ, Wang YS, et al. Risk factors for sentinel lymph node metastasis and validation study of the MSKCC nomogram in breast cancer patients. Jpn J Clin Oncol 2012;42:1002-7. [Crossref] [PubMed]
- Pinheiro DJ, Elias S, Nazário AC. Axillary lymph nodes in breast cancer patients: sonographic evaluation. Radiol Bras 2014;47:240-4. [Crossref] [PubMed]
- Xu Z, Ding Y, Zhao K, et al. MRI characteristics of breast edema for assessing axillary lymph node burden in early-stage breast cancer: a retrospective bicentric study. Eur Radiol 2022;32:8213-25. [Crossref] [PubMed]
- Kim T, Giuliano AE, Lyman GH. Lymphatic mapping and sentinel lymph node biopsy in early-stage breast carcinoma: a metaanalysis. Cancer 2006;106:4-16. [Crossref] [PubMed]
- Lyman GH, Giuliano AE, Somerfield MR, et al. American Society of Clinical Oncology guideline recommendations for sentinel lymph node biopsy in early-stage breast cancer. J Clin Oncol 2005;23:7703-20. [Crossref] [PubMed]
- Veronesi U, Paganelli G, Viale G, et al. A randomized comparison of sentinel-node biopsy with routine axillary dissection in breast cancer. N Engl J Med 2003;349:546-53. [Crossref] [PubMed]
- Liu P, Tan J, Song Y, et al. The Application of Magnetic Nanoparticles for Sentinel Lymph Node Detection in Clinically Node-Negative Breast Cancer Patients: A Systemic Review and Meta-Analysis. Cancers (Basel) 2022;14:5034. [Crossref] [PubMed]
- Kimura F, Yoshimura M, Koizumi K, et al. Radiation exposure during sentinel lymph node biopsy for breast cancer: effect on pregnant female physicians. Breast Cancer 2015;22:469-74. [Crossref] [PubMed]
- Wilke LG, McCall LM, Posther KE, et al. Surgical complications associated with sentinel lymph node biopsy: results from a prospective international cooperative group trial. Ann Surg Oncol 2006;13:491-500. [Crossref] [PubMed]
- Sugden EM, Rezvani M, Harrison JM, et al. Shoulder movement after the treatment of early stage breast cancer. Clin Oncol (R Coll Radiol) 1998;10:173-81. [Crossref] [PubMed]
- Chen JY, Chen JJ, Yang BL, et al. Predicting sentinel lymph node metastasis in a Chinese breast cancer population: assessment of an existing nomogram and a new predictive nomogram. Breast Cancer Res Treat 2012;135:839-48. [Crossref] [PubMed]
- Nottegar A, Veronese N, Senthil M, et al. Extra-nodal extension of sentinel lymph node metastasis is a marker of poor prognosis in breast cancer patients: A systematic review and an exploratory meta-analysis. Eur J Surg Oncol 2016;42:919-25. [Crossref] [PubMed]
- La Verde N, Biagioli E, Gerardi C, et al. Role of patient and tumor characteristics in sentinel lymph node metastasis in patients with luminal early breast cancer: an observational study. Springerplus 2016;5:114. [Crossref] [PubMed]
- Viale G, Zurrida S, Maiorano E, et al. Predicting the status of axillary sentinel lymph nodes in 4351 patients with invasive breast carcinoma treated in a single institution. Cancer 2005;103:492-500. [Crossref] [PubMed]
- Ozemir IA, Orhun K, Eren T, et al. Factors affecting sentinel lymph node metastasis in Turkish breast cancer patients: Predictive value of Ki-67 and the size of lymph node. Bratisl Lek Listy 2016;117:436-41. [Crossref] [PubMed]
- Omoto K, Matsunaga H, Take N, et al. Sentinel node detection method using contrast-enhanced ultrasonography with sonazoid in breast cancer: preliminary clinical study. Ultrasound Med Biol 2009;35:1249-56. [Crossref] [PubMed]
- Lambin P, Leijenaar RTH, Deist TM, et al. Radiomics: the bridge between medical imaging and personalized medicine. Nat Rev Clin Oncol 2017;14:749-62. [Crossref] [PubMed]
- Zhang B, He X, Ouyang F, et al. Radiomic machine-learning classifiers for prognostic biomarkers of advanced nasopharyngeal carcinoma. Cancer Lett 2017;403:21-7. [Crossref] [PubMed]
- Chen T, Ning Z, Xu L, et al. Radiomics nomogram for predicting the malignant potential of gastrointestinal stromal tumours preoperatively. Eur Radiol 2019;29:1074-82. [Crossref] [PubMed]
- Cunha GM, Fowler KJ. Automated Liver Segmentation for Quantitative MRI Analysis. Radiology 2022;302:355-6. [Crossref] [PubMed]
- Li H, Zhu Y, Burnside ES, et al. Quantitative MRI radiomics in the prediction of molecular classifications of breast cancer subtypes in the TCGA/TCIA data set. NPJ Breast Cancer 2016;2:16012. [Crossref] [PubMed]
- Zhou M, Leung A, Echegaray S, et al. Non-Small Cell Lung Cancer Radiogenomics Map Identifies Relationships between Molecular and Imaging Phenotypes with Prognostic Implications. Radiology 2018;286:307-15. [Crossref] [PubMed]
- Kuo MD, Jamshidi N. Behind the numbers: Decoding molecular phenotypes with radiogenomics--guiding principles and technical considerations. Radiology 2014;270:320-5. [Crossref] [PubMed]
- Gillies RJ, Kinahan PE, Hricak H. Radiomics: Images Are More than Pictures, They Are Data. Radiology 2016;278:563-77. [Crossref] [PubMed]
- Bolón-Canedo V, Sánchez-Maroño N, Alonso-Betanzos A. A review of feature selection methods on synthetic data. Knowl Inf Syst 2013;34:483-519. [Crossref]
- Bolón-Canedo V, Sánchez-Marono N, Alonso-Betanzos A, et al. A review of microarray datasets and applied feature selection methods. Information Sciences 2014;282:111-35. [Crossref]
- Tan KM, London P, Mohan K, et al. Learning Graphical Models With Hubs. J Mach Learn Res 2014;15:3297-331. [PubMed]
- Haury AC, Gestraud P, Vert JP. The influence of feature selection methods on accuracy, stability and interpretability of molecular signatures. PLoS One 2011;6:e28210. [Crossref] [PubMed]
- Ganeshan B, Goh V, Mandeville HC, et al. Non-small cell lung cancer: histopathologic correlates for texture parameters at CT. Radiology 2013;266:326-36. [Crossref] [PubMed]
- Lv W, Yuan Q, Wang Q, et al. Robustness versus disease differentiation when varying parameter settings in radiomics features: application to nasopharyngeal PET/CT. Eur Radiol 2018;28:3245-54. [Crossref] [PubMed]
- Max J. Quantizing for minimum distortion. IRE Trans Inf Theory 1960;6:7-12. [Crossref]
- Ning Z, Luo J, Li Y, et al. Pattern Classification for Gastrointestinal Stromal Tumors by Integration of Radiomics and Deep Convolutional Features. IEEE J Biomed Health Inform 2019;23:1181-91. [Crossref] [PubMed]
- Vallières M, Freeman CR, Skamene SR, et al. A radiomics model from joint FDG-PET and MRI texture features for the prediction of lung metastases in soft-tissue sarcomas of the extremities. Phys Med Biol 2015;60:5471-96. [Crossref] [PubMed]
- Spanhol FA, Oliveira LS, Petitjean C, et al. A Dataset for Breast Cancer Histopathological Image Classification. IEEE Trans Biomed Eng 2016;63:1455-62. [Crossref] [PubMed]
- Hamidinekoo A, Denton E, Rampun A, et al. Deep learning in mammography and breast histology, an overview and future trends. Med Image Anal 2018;47:45-67. [Crossref] [PubMed]
- Cheng J, Zhang J, Han Y, et al. Integrative Analysis of Histopathological Images and Genomic Data Predicts Clear Cell Renal Cell Carcinoma Prognosis. Cancer Res 2017;77:e91-e100. [Crossref] [PubMed]
- Mohan K, London P, Fazel M, et al. Node-Based Learning of Multiple Gaussian Graphical Models. J Mach Learn Res 2014;15:445-88. [PubMed]
- Bootkrajang J, Kabán A. Boosting in the presence of label noise. arXiv:1309.6818 [Preprint]. 2013. Available online: https://arxiv.org/abs/1309.6818
- Jadhav SD, Channe HP. Comparative study of K-NN, naive Bayes and decision tree classification techniques. International Journal of Science and Research 2016;5:1842-5. (IJSR).
- Zhang S. Cost-sensitive KNN classification. Neurocomputing 2020;391:234-42. [Crossref]
- Bateni SM, Vosoughifar HR, Truce B, et al. Estimation of clear-water local scour at pile groups using genetic expression programming and multivariate adaptive regression splines. J Waterw Port Coast Ocean Eng 2019;145:04018029. [Crossref]
- Luo D, Ding C, Huang H. Linear discriminant analysis: New formulations and overfit analysis. Proceedings of the AAAI Conference on Artificial Intelligence. 2011;25:417-22. [Crossref]
- Hussain M, Wajid SK, Elzaart A, et al. A comparison of SVM kernel functions for breast cancer detection. In: 2011 Eighth International Conference Computer Graphics, Imaging and Visualization. IEEE; 2011:145-50.
- Fawagreh K, Gaber MM, Elyan E. Random forests: from early developments to recent advancements. Systems Science & Control Engineering: An Open Access Journal 2014;2:602-9. [Crossref]
- Peng H, Long F, Ding C. Feature selection based on mutual information: criteria of max-dependency, max-relevance, and min-redundancy. IEEE Trans Pattern Anal Mach Intell 2005;27:1226-38. [Crossref] [PubMed]
- Parmar C, Grossmann P, Bussink J, et al. Machine Learning methods for Quantitative Radiomic Biomarkers. Sci Rep 2015;5:13087. [Crossref] [PubMed]
- Fan J, Samworth R, Wu Y. Ultrahigh dimensional feature selection: beyond the linear model. J Mach Learn Res 2009;10:2013-38. [PubMed]