A model for predicting clinical prognosis based on brain metastasis-related genes in patients with breast cancer
Highlight box
Key findings
• This study developed a risk score model based on 12 brain metastasis (BM)-related genes in breast cancer (BRCA). Low-risk patients showed more favorable immune cell infiltration and higher expression of immune checkpoint genes, suggesting a robust immune response. Additionally, the study constructed a predictive model for the survival of BRCA patients by incorporating risk scores, clinical stage, and age, yielding a high degree of predictive accuracy.
What is known and what is new?
• BM is a clinically relevant cause of death in patients with BRCA.
• A predictive clinical model for BRCA patients was successfully established in this study, providing a valuable tool that may be particularly helpful for the assessment of patients facing a risk of BM development.
What is the implication, and what should change now?
• It is necessary to evaluate the prognosis of BRCA patients as early as possible.
Introduction
Brain metastases (BMs) are among the most frequently detected forms of intracranial tumor (1), most often arising from primary tumor types including breast cancer (BRCA), melanoma, and lung cancer (2). Therapeutic advances have prolonged patient survival, resulting in a consequent increase in BM-related morbidity. Once patients develop BMs, they face a very poor prognosis as these metastases do not respond well to treatment (3). BRCA is the most common cause of cancer among women and the second most common primary tumor type associated with BMs, which develop in 10–30% of patients with metastatic BRCA (4). In some cases, BMs may be the first manifestation of metastatic disease in individuals with BRCA (5). Important risk factors related to BRCA and BM incidence include age, estrogen receptor (ER) and progesterone receptor (PR) status, human epidermal growth factor receptor 2 (HER2) status, numbers of BMs, the presence or absence of extracranial metastases, pathological stage, and histological grade (6). As BRCA is a highly heterogeneous and complex disease, predicting and preventing BM development remains largely impossible (7). How the expression of specific BM-related genes (BMRGs) is associated with the overall progression and pathogenesis of BM also remains uncertain. Advances in bioinformatics techniques have enabled the development of novel anticancer treatments through the screening of tumor-associated genes, the assessment of therapeutic efficacy, and the prediction of patient prognostic outcomes (8). Targeted therapeutics are frequently used to treat BRCA and many other malignancies, and bioinformatics studies can help clarify the most optimal targets for drug design. Immunotherapy is also an active area of research interest, and the immune cell infiltration status of a given tumor can help predict the degree to which patients are likely to respond to immunotherapeutic interventions (9). Differences in the expression levels of key immune checkpoint genes such as PD1, PD-L1, and PD-L2 have been reported when comparing primary and metastatic tumor sites, which may have important implications for immunotherapeutic treatment (10).
Here, bioinformatics approaches were employed to evaluate the roles of BMRGs in BRCA. In total, 12 BM-related differentially expressed genes (BM_DEGs) were selected and utilized to generate a risk scoring model, with the risk scores derived from this model offering value as predictors of patient immunotherapy responses. These risk scores were additionally combined with age and stage information to generate a predictive nomogram capable of gauging the overall survival (OS) of patients with BRCA. We present this article in accordance with the TRIPOD reporting checklist (available at https://tcr.amegroups.com/article/view/10.21037/tcr-23-1123/rc).
Methods
Data source
RNA-sequencing data and corresponding clinical data for 1,226 samples (1,113 BRCA tissue samples and 113 normal tissue samples) were obtained with the R The Cancer Genome Atlas (TCGA) biolinks package (11) in the transcripts per million (TPM) format (Table 1). Of these samples, 1,014 BRCA patient samples designated as “01A” with available information regarding OS and survival status and a time greater than 0 were retained for analysis. Among the included data of patients, 912 and 102 patients were alive and dead respectively at last follow-up. Additionally, 99 control patients with the “11A” designation were retained for this study. With respect to the TPM gene expression data, protein-coding genes were retained and half of those genes that were not detectable or expressed at low levels were omitted from analyses, with the remaining 17,374 genes being retained for further study.
Table 1
Variables | Alive (n=912) | Dead (n=102) | Total (n=1,014) |
---|---|---|---|
Age (years) | |||
Mean | 57.9 | 60.9 | 58.2 |
Median | 58 | 62 | 58 |
>58, n (%) | 442 (48.5) | 57 (55.9) | 499 (49.2) |
≤58, n (%) | 470 (51.5) | 45 (44.1) | 515 (50.8) |
Gender, n (%) | |||
Female | 900 (98.7) | 102 (100.0) | 1,002 (98.8) |
Male | 12 (1.3) | 0 (0.0) | 12 (1.2) |
T, n (%) | |||
T1 | 244 (26.8) | 25 (24.5) | 269 (26.5) |
T2 | 526 (57.7) | 50 (49.0) | 576 (56.8) |
T3 | 112 (12.3) | 16 (15.7) | 128 (12.6) |
T4 | 28 (3.1) | 10 (9.8) | 38 (3.7) |
TX | 2 (0.2) | 1 (1.0) | 2 (0.2) |
N, n (%) | |||
N0 | 442 (48.5) | 27 (26.5) | 469 (46.3) |
N1 | 298 (32.7) | 42 (41.2) | 340 (33.5) |
N2 | 100 (11.0) | 15 (14.7) | 115 (11.3) |
N3 | 63 (6.9) | 10 (9.8) | 73 (7.2) |
NX | 9 (1.0) | 8 (7.8) | 17 (1.7) |
M, n (%) | |||
M0 | 756 (82.9) | 86 (84.3) | 842 (83.0) |
M1 | 13 (1.4) | 9 (8.8) | 22 (2.2) |
MX | 143 (15.7) | 7 (6.9) | 150 (14.8) |
Stage, n (%) | |||
I | 161 (17.7) | 12 (11.8) | 173 (17.1) |
II | 518 (56.8) | 44 (43.1) | 562 (55.4) |
III | 207 (22.7) | 29 (28.4) | 236 (23.3) |
IV | 11 (1.2) | 9 (8.8) | 20 (2.0) |
X | 15 (1.6) | 8 (7.9) | 23 (2.3) |
T, tumor; N, node; M, metastasis.
TCGA biolinks were used to download masked somatic mutation data from 981 patients, with visualization performed using the R ‘maftools’ packages (12). TCGA biolinks were also used to download masked copy number segment data from 1,084 BRCA patients, with the R ‘ggplot2’ package being used for visualization. The R ‘GEOquery’ package (13) was installed, followed by the downloading of two BRCA gene expression datasets [GSE42568 (14) and GSE20711 (15)] from the Gene Expression Omnibus (GEO) database (16) (https://www.ncbi.nlm.nih.gov/geo/), with these datasets respectively including 104 and 88 BRCA patient samples. Moreover, this package was used to download a BRCA chemotherapy dataset (GSE41998) (17) containing 201 and 69 patients classified as exhibiting complete response/partial response (CR/PR) and stable disease/progressive disease (SD/PD), respectively.
BMRGs were selected from the PubMed database (18), including TP53, CDH1, MAP3K1, FAT1, FLT3, ATM, CHEK2, KMT2C, RB1, ZFHX3, BRCA2, HER2, PIK3CA, COL6A3, KMT2D, MLH1, PTEN, ATR, IGFN1, ARID1A, BRCA1, and MET. This study was conducted in accordance with the Declaration of Helsinki (as revised in 2013).
BMRG-based identification of BRCA subtypes
The R ‘ConsensusClusterPlus’ package was used to perform an unsupervised consensus clustering analysis (19,20), enabling the establishment of BRCA subtypes based on the expression of BMRGs in the TCGA dataset using the following parameters: 80% item resampling, cluster Alg = “pam”, distance = “canberra”, two-eight clusters, and 1,000 repetitions.
Gene set variation analysis (GSVA)
Enrichment analyses were performed via a GSVA approach based on the gene expression data in the TCGA-BRCA database (21). GSVA analyses were performed by downloading “h.all. v7.5.1. symbols” from the MSigDB database (22), with an adjusted P<0.05 as the significance threshold when comparing groups.
BM_DEG identification
Differences among BRCA molecular subtypes were compared using the R ‘limma’ package (23), based on the molecular subtypes defined for patients in the TCGA database. BM_DEGs were defined as genes meeting the following criteria: |logfold change (FC)| >1.5 and adjusted P value <0.01.
BM_DEG-based identification of BRCA subtypes
To better explore the link between BM_DEGs and BRCA in patient prognosis, the ‘ConsensusClusterPlus’ package was used for the unsupervised clustering of samples in the TCGA dataset based on BM_DEG expression to define BRCA subtypes using the following parameters: 80% item resampling, clusterAlg = “pam”, distance = “euclidean”, two-eight clusters, and 1,000 repetitions.
Functional enrichment analyses
Gene Ontology (GO) (24) and Kyoto Encyclopedia of Genes and Genomes (KEGG) pathway (25) enrichment analyses were performed using Metascape. GO analyses were used to annotate genes based on specific molecular function (MF), biological process (BP), and cellular component (CC) terms, while KEGG analyses were used to systematically explore the pathways related to individual genes and gene sets to gain insight into their functional roles. The R ‘clusterProfiler’ package was used for GO and KEGG enrichment analyses (26), with the following significance criteria: a Benjamini-Hochberg adjusted P value <0.05, q value <0.05, and false discovery rate (FDR) <0.05.
DEG-based risk model construction
A least absolute selection and shrinkage operator (LASSO)-Cox analysis was used for the establishment of an efficient predictive model. Initially, the relationship between OS and the identified BM_DEGs was explored through a univariate Cox regression approach. The genes that were significant (P<0.05) in these analyses were subjected to LASSO analysis in order to minimize multicollinearity and screen for the most meaningful genes. A multivariate Cox regression analysis was used to more accurately define independent prognostic factors (prognostic eigengenes), and a stepwise regression strategy was used for the final screening. A risk score formula was then established based on the expression of the identified significant genes and multivariate Cox regression coefficients.
The ‘surv_cutpoint’ package was used to establish an optimal cut-off threshold, with TCGA patients then being separated into low- and high-risk groups according to their scores as compared to this threshold. OS rates were then compared between these two patient cohorts with Kaplan-Meier curves and log-rank tests with the ‘survival’ R package.
Data validation was performed with the GSE42568 and GSE20711 datasets, with the formula established above being used to compute risk scores in both datasets. OS rates in low- and high-risk patients in these datasets were compared as above.
Gene set enrichment analysis (GSEA)
A GSEA approach was used to compare differences in BP activity in the low- and high-risk groups (27), using gene expression data from the TCGA-BRCA cohort for these analyses. To perform this GSEA, “h.all. v7.5.1. symbols” was downloaded from the MSigDB database, and an adjusted P value <0.05 was the threshold for significance in these groups.
Immune infiltration analysis
Immune cell infiltration within tumors can provide valuable insights on disease pathogenesis and can help predict treatment outcomes. An immune-related gene list comprised of 782 genes and 28 cell types was downloaded from a previously published source (28). A single-sample GSEA (ssGSEA) approach was then used for immune infiltration analyses of samples in the TCGA-BRCA dataset with the ‘GSVA’ package. The ‘corrplot’ package was utilized to plot the graphs for the resultant immune cell correlations.
Immunotherapy analyses
BRCA patient immunophenoscore (IPS) data were obtained from The Cancer Immunome Atlas (TCIA; https://tcia.at/home) database and were analyzed with the R ‘ggplot2’ package. Tumor Immune Dysfunction and Exclusion (TIDE) (http://tide.dfci.harvard.edu) (29) scores were computed based on standardized TCGA-BRCA expression profile-derived data to assess low- and high-risk patient responses to immunotherapy, with the ‘ggplot2’ package being employed for result visualization.
Construction of a predictive clinical model
Univariate and multivariate Cox regression analyses were used to assess the ability of risk score values to predict patient OS alone and in combination with clinicopathological characteristics, with those characteristics significantly related to patient OS (P<0.05) ultimately being incorporated into a model nomogram that was constructed with the R ‘rms’ package.
Statistical analysis
R (v4.1.1) was used for all statistical testing. Normally and non-normally distributed data were respectively compared with Student’s t-tests and Mann-Whitney U tests for continuous variables, with categorical variables instead being compared with χ2 tests or Fisher’s exact test. Survival outcomes were compared with Kaplan-Meier curves and the log-rank test. Univariate and multivariate Cox regression analyses were performed with the R ‘survival’ package, while the ‘glmnet’ package was used for LASSO analysis (30).
Results
Figure 1 depicts the flow chart of this investigation’s analytical approach.
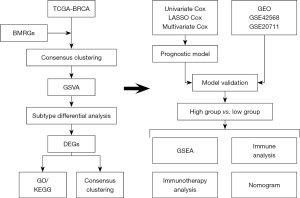
The relationship between BMRGs and BRCA
Initially, BMRGs of BRCA were selected from the PubMed database (18). The R prcomp function was used to perform a principal component analysis (Figure 2A), which revealed that BMRGs were able to effectively distinguish the majority of BRCA tissue samples from normal control samples. Analyses of the mRNA expression levels of these genes revealed that over 85% of BMRGs differed significantly in expression between BRCA and normal control samples (Figure 2B). Single nucleotide polymorphism analyses of BMRGs performed with the ‘maftools’ package revealed that 22 BMRGs were mutated in 699 samples at an overall mutation frequency of 77.84% (Figure 2C). The most frequently mutated BMRG was TP53, which was mutated in 34% of samples. Copy number variation analyses revealed that such variations were present in most BRCA samples, with deletions being the most common variations, potentially impacting BMRG expression (Figure 2D).
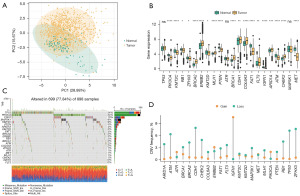
Establishment of BMRG-based BRCA subtypes
To explore the interrelated nature of the BMRGs identified in BRCA patients, correlation heatmaps were constructed revealing positive correlations among the majority of these genes (Figure 3A). Notably, KMT2C and KMT2D were strongly positively correlated (cor =0.778, P=2.792E−206), as were PIK3CA and ATR (cor =0.715, P=1.515E−159), whereas CHEK2 and COL6A3 were negatively correlated (cor =−0.233, P=5.532E−14). The relationship between the expression of these BMRGs and BRCA patient prognostic outcomes was explored by using the expression profiles of 22 BMRGs for consistent BRCA sample clustering, revealing an optimal cluster number of three (Figure 3B-3D). Subsequent prognostic assessment revealed that survival outcomes differed significantly among these three BRCA subtypes [cluster (C)1, C2, and C3] (log-rank P=0.021; Figure 3E). BMRG expression was additionally compared among these different groups (Figure 3F), demonstrating significant variations in all identified BMRGs (t-tests, P<0.05). Heatmaps revealed lower levels of ARID1A, KMT2C, and ATM in the C3 BRCA subtype that exhibited better prognostic outcomes, whereas these genes were expressed at higher levels in the C1 and C2 subtypes exhibiting worse prognoses (Figure 3G).
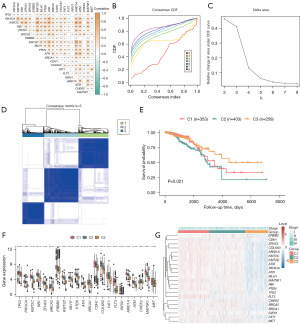
GSVAs
To better understand functional differences among BRCA subtypes, hallmark GSEAs were performed with the GSVA package (Figure 4A, table available at https://cdn.amegroups.cn/static/public/tcr-23-1123-1.xlsx). When assessing the relationship between these subtypes and staging, a higher frequency of node (N)0-stage tumors was evident in the C3 subtype which exhibited a better prognosis as compared to the C1 and C2 subtypes that exhibited worse prognoses (Figure 4B). Sankey diagrams were used to further explore these relationships between staging and BRCA subtypes (Figure 4C), revealing that most patients in the C3 subtype had stage I–III disease, whereas the majority of stage IV patients were present in the C1 and C2 subtypes facing a poorer prognosis. Differences in age were also compared among these subtypes (Figure 4D), revealing that patients classified in the C3 subtype were significantly older than C1 subtype patients that exhibited a poorer prognosis (P=0.026).
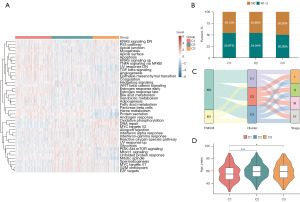
Identification of the functional and prognostic implications of BM_DEGs
Differences in biological functionality among BRCA subtypes were further explored by using the ‘limma’ package to compare gene expression patterns, leading to the identification of 224 BM_DEGs in BRCA patient samples (table available at https://cdn.amegroups.cn/static/public/tcr-23-1123-2.xlsx). The relationship between these genes and BRCA patient outcomes was additionally evaluated through an unsupervised clustering analysis, with k=3 being selected as the optimal number of clusters. Prognostic analyses revealed significant survival differences among groups (log-rank P=0.017), confirming that clustering results were accurate (Figure 5A,5B). GO and KEGG enrichment analyses of these BM_DEGs were next performed, revealing close associations between these genes and the following GO terms: morphogenesis of a BP (Figure 5C), CC (Figure 5D), MF (Figure 5E, Table 2). These BM_DEGs were also closely associated with PI3K-Akt signaling and Focal adhesion pathways in KEGG pathways (Figure 5F, Table 3). Correlations between BM_DEG-based BRCA subtypes and BRCA patient characteristics were next assessed, revealing significant differences in age among these subtypes such that the age of grade (G)3 subtype patients exhibiting a better prognosis was significantly lower than that of G2 subtype patients facing a worse prognosis (P<0.001; Figure 5G). Moreover, more patients with N0 staging were included in the G3 subtype than the G2 subtype, whereas the C1 subtype was more common among G1 subtype patients facing a worse prognosis than among G3 subtype patients (Figure 5H,5I).
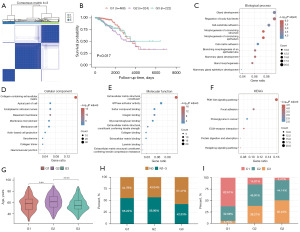
Table 2
Ontology | ID | Description | Adjusted P value |
---|---|---|---|
BP | GO:0061138 | Morphogenesis of a branching epithelium | 8.57E−07 |
GO:0001763 | Morphogenesis of a branching structure | 1.28E−06 | |
GO:0030879 | Mammary gland development | 5.56E−06 | |
GO:0022612 | Gland morphogenesis | 7.46E−06 | |
GO:0050878 | Regulation of body fluid levels | 7.90E−06 | |
GO:0048754 | Branching morphogenesis of an epithelial tube | 9.07E−06 | |
GO:0048732 | Gland development | 5.65E−05 | |
GO:0031589 | Cell-substrate adhesion | 6.91E−05 | |
GO:0007160 | Cell-matrix adhesion | 0.000154 | |
GO:0061180 | Mammary gland epithelium development | 0.000268 | |
CC | GO:0062023 | Collagen-containing extracellular matrix | 3.55E−16 |
GO:0005604 | Basement membrane | 7.51E−09 | |
GO:0005788 | Endoplasmic reticulum lumen | 0.004009 | |
GO:0005581 | Collagen trimer | 0.004009 | |
GO:0045121 | Membrane raft | 0.019748 | |
GO:0098857 | Membrane microdomain | 0.019748 | |
GO:0098858 | Actin-based cell projection | 0.034541 | |
GO:0042383 | Sarcolemma | 0.034541 | |
GO:0031594 | Neuromuscular junction | 0.034814 | |
GO:0045177 | Apical part of cell | 0.034814 | |
MF | GO:0005201 | Extracellular matrix structural constituent | 8.57E−17 |
GO:0030020 | Extracellular matrix structural constituent conferring tensile strength | 8.55E−05 | |
GO:0005178 | Integrin binding | 0.000125 | |
GO:0050840 | Extracellular matrix binding | 0.004828 | |
GO:0030021 | Extracellular matrix structural constituent conferring compression resistance | 0.009597 | |
GO:0005518 | Collagen binding | 0.010511 | |
GO:1901681 | Sulfur compound binding | 0.014436 | |
GO:0043236 | Laminin binding | 0.014436 | |
GO:0005096 | GTPase activator activity | 0.014436 | |
GO:0005539 | Glycosaminoglycan binding | 0.014842 |
GO, Gene Ontology; BP, biological process; CC, cellular component; MF, molecular function.
Table 3
ID | Description | Adjusted P value |
---|---|---|
hsa04340 | Hedgehog signaling pathway | 0.07 |
hsa04151 | PI3K-Akt signaling pathway | 0.16 |
hsa04512 | Extracellular matrix -receptor interaction | 0.08 |
hsa04510 | Focal adhesion | 0.10 |
hsa04974 | Protein digestion and absorption | 0.07 |
hsa05205 | Proteoglycans in cancer | 0.09 |
KEGG, Kyoto Encyclopedia of Genes and Genomes.
Establishment of a BM_DEG-based risk score model
The relationships between BM_DEGs and BRCA patient outcomes were next explored by developing a risk scoring model. Briefly, univariate analyses were used to screen the 224 identified BM_DEGs (Figure 6A, table available at https://cdn.amegroups.cn/static/public/tcr-23-1123-3.xlsx), with the 52 genes significantly associated with patient prognosis (P<0.05) being subjected to LASSO regression screening to remove collinearity. The remaining BM_DEGs were then subjected to 10-fold cross-validation to select an optimal lambda value (Figure 6B, table available at https://cdn.amegroups.cn/static/public/tcr-23-1123-4.xlsx). This approach ultimately led to the construction of risk scoring model based on the expression of 12 genes (CLIC6, NPY1R, PTPRT, SCUBE2, FAM234B, AFF4, FLT3, WNK4, HCAR1, GREB1, PCSK6, and SPOPL) through a multivariate Cox regression and stepwise regression approach (Figure 6C). The final risk score formula was as follows:
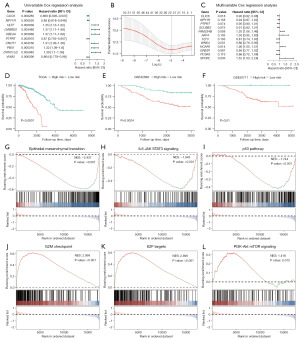
To validate this model, patients in the TCGA-BRCA cohort were stratified into low- and high-risk groups based on an established cut-off value (3.634477), with survival analyses revealing that low-risk patients exhibited better survival outcomes than high-risk individuals (P<0.0001; Figure 6D). Patients from the GSE42568 and GSE20711 datasets were similarly separated into low- and high-risk subsets based on appropriate cut-off values (GSE42568:1.059499 and GSE20711:0.03437818), demonstrating significantly better survival rates in low-risk individuals in both GSE42568 (P=0.0024) and GSE20711 (P=0.01) datasets relative to high-risk patients (Figure 6E,6F). A GSEA approach was then used to explore the BPs related to these differences in risk scores, revealing significant enrichment of pathways including the epithelial-mesenchymal transition [normalized enrichment score (NES) =−2.437; Figure 6G], IL6 JAK STAT3 signaling (NES =−1.943; Figure 6H), and p53 (NES =−1.744; Figure 6I) pathways in low-risk patients. In contrast, high-risk patients exhibited significant enrichment for the G2M checkpoint (NES =2.999; Figure 6J), E2F targets (NES =2.896; Figure 6K), and PI3K-Akt mTOR signaling (NES =1.418; Figure 6L) pathways (Table 4).
Table 4
ID | NES | Adjusted P value |
---|---|---|
HALLMARK_ALLOGRAFT_REJECTION | −2.15287 | 1.00E−10 |
HALLMARK_E2F_TARGETS | 2.896092 | 1.00E−10 |
HALLMARK_EPITHELIAL_MESENCHYMAL_TRANSITION | −2.4371 | 1.00E−10 |
HALLMARK_ESTROGEN_RESPONSE_EARLY | −2.23573 | 1.00E−10 |
HALLMARK_ESTROGEN_RESPONSE_LATE | −2.19939 | 1.00E−10 |
HALLMARK_G2M_CHECKPOINT | 2.998763 | 1.00E−10 |
HALLMARK_MITOTIC_SPINDLE | 2.368505 | 1.00E−10 |
HALLMARK_MTORC1_SIGNALING | 2.686514 | 1.00E−10 |
HALLMARK_PROTEIN_SECRETION | 2.688466 | 1.00E−10 |
HALLMARK_TNFA_SIGNALING_VIA_NFKB | −2.39287 | 1.00E−10 |
GSEA, gene set enrichment analysis; NES, normalized enrichment score.
Immune cell infiltration analyses
Using the ssGSEA algorithm, associations between immune cell infiltration levels and BM_DEG-based risk scores were next explored in BRCA patients (table available at https://cdn.amegroups.cn/static/public/tcr-23-1123-5.xlsx). Higher levels of predicted infiltration by cell types including CD56dim natural killer cell, regulatory T cells, and central memory CD4+ T cells were evident in the low-risk group, whereas higher levels of predicted infiltration by type 2 T helper cell and type 17 T helper cell were evident in the high-risk group (Figure 7A). Correlation heatmaps revealed that infiltration by most analyzed immune cell types was positively correlated (Figure 7B). Over 65% of immune cell types differed significantly in their predicted infiltration levels between the low- and high-risk groups, with most exhibiting significantly increased infiltration in the tumors of low-risk patients (Figure 7C). Correlation heatmaps that were constructed to assess the relationship between BM_DEGs and immune cell infiltration revealed that genes such as NPY1R and HCAR1 were expressed at high levels in most immune cell types, whereas GREB1 and FLT3 were expressed at low levels in most of these cells (Figure 7D).
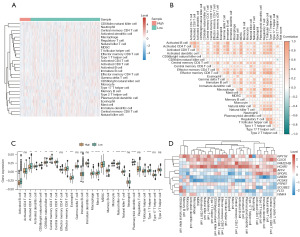
Risk scores predict immunotherapeutic efficacy in BRCA
The established risk score cut-off value (1.888723) was used to classify patients from the IMvigor210 immunotherapy dataset as being either low- or high-risk, with the survival odds of low-risk patients being significantly better than that of high-risk patients (Figure 8A). Immunotherapy response frequencies were compared between these groups (Figure 8B), revealing that there were more patients that achieved CR/PR in the low-risk group relative to the high-risk group (35% vs. 19%), whereas SD/PD were more common among high-risk patients relative to low-risk patients (81% vs. 65%).
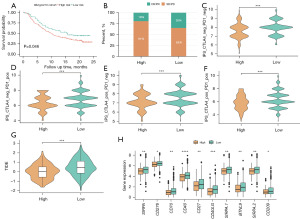
Next, IPS values from the TCIA database were utilized to gauge the ability of this risk scoring model to predict immunotherapeutic outcomes (table available at https://cdn.amegroups.cn/static/public/tcr-23-1123-6.xlsx), with the results being assembled into boxplots. These analyses revealed clear differences in IPS values between the low- and high-risk groups. Specifically, IPS (Figure 8C), IPS-PD1/PD-L1/PD-L2 (Figure 8D), IPS-CTLA4 (Figure 8E), and IPS-PD1/PD-L1/PD-L2 + CTLA4 (Figure 8F) values in the low-risk group were all significantly higher than those in the high-risk group (P<0.001). Immune checkpoint blockade (ICB) treatments can offer long-term benefits to patients. To gauge the utility of these risk scores as predictors of ICB treatment response, potential immunotherapeutic efficacy was assessed in BRCA patients with the TIDE algorithm (Figure 8G, table available at https://cdn.amegroups.cn/static/public/tcr-23-1123-7.xlsx). Significant variations in TIDE scores were observed among risk groups, with a significant increase in these scores among low-risk individuals as compared to high-risk individuals (P<0.001). To evaluate the relationship between risk scores and immune checkpoints, the expression of these immune checkpoint genes in specific risk groups was analyzed and graphed (Figure 8H). The majority of these genes were expressed at higher levels in the low-risk group relative to the high-risk group.
Development of a risk score-based predictive model
The independent prognostic utility of risk score values and clinicopathological characteristics were assessed through univariate and multivariate Cox regression analyses. In univariate analyses, risk scores (P<0.001), stage (P<0.001), N (P<0.001), age (P<0.001), and M (P=0.00753) were all correlated with OS (Figure 9A, Table 5), while multivariate analyses indicated that risk scores (P<0.001), stage IV (P<0.001), and age (P=0.049) were significantly related to OS (Figure 9B, Table 6). These three variables were then incorporated into a nomogram used to predict BRCA patient OS (Figure 9C), and calibration curves revealed that the predictions of 1-, 3-, and 5-year OS made by this nomogram were consistent with actual survival outcomes (Figure 9D).
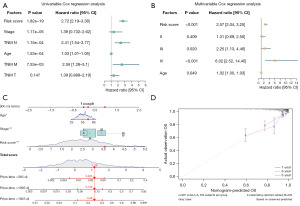
Table 5
Variables | HR (95% CI) | P value |
---|---|---|
Risk score | 2.72 (2.19–3.38) | 1.82E−19 |
Stage | 1.39 (0.732–2.62) | 1.17E−05 |
N | 2.41 (1.54–3.77) | 1.15E−04 |
Age | 1.03 (1.01–1.05) | 1.53E−04 |
M | 2.56 (1.28–5.1) | 7.53E−03 |
T | 1.39 (0.889–2.19) | 0.147 |
HR, hazard ratio; CI, confidence interval; N, node; M, metastasis; T, tumor.
Table 6
Variables | HR (95% CI) | P value |
---|---|---|
Risk score | 2.57 (2.04–3.25) | <0.001 |
Stage | ||
II | 1.31 (0.69–2.50) | 0.409 |
III | 2.25 (1.13–4.46) | 0.020 |
IV | 6.02 (2.52–14.40) | <0.001 |
Age (years) | 1.02 (1.00–1.03) | 0.049 |
HR, hazard ratio; CI, confidence interval.
Discussion
BM incidence is among the most serious complications associated with many different cancers, as even in mild cases these metastases can result in severe neurological dysfunction such that the affected patients exhibit a median survival duration of just 6 months (31). In patients with BRCA, metastases typically spread throughout adjacent organs during the earlier stages of PD, only spreading to sites such as the brain during the most advanced stages (32). While BRCA patients exhibit a relatively good prognosis relative to that associated with many other forms of cancer, BM onset is associated with a serious drop in survival rates and leading to negative outcomes in these patients. The blood-brain barrier can severely hamper the ability of chemotherapeutic drugs to achieve satisfactory efficacy within the brain, and the benefits of immunotherapy when seeking to treat metastases within the brain are also limited given the poorly characterized immunological characteristics of this compartment (33). Surgical resection can only be successfully performed in a limited subset of patients, including those with relatively stable primary lesions, intracranial oligometastatic tumors, and tumors affecting non-important functional areas (34). During surgery, BMs in BRCA patients are often found to be closely adherent to the dura matter with leptomeningeal infiltration in some cases. As such, the risk of recurrence remains high even when these metastases are resected under microscopic visualization. Given these factors, accurate prognostic assessment is crucial for individualized treatment of BRCA patients. In one prior study, Liu et al. developed a prognostic model focused on the evaluation of BRCA patients diagnosed with BMs (35). Cheng et al. (36) further used the MRI results from triple-negative BRCA patients to generate a model to predict BM risk, while Gao et al. generated a model to predict BM risk in BRCA patients based upon data from the public TCGA and GEO databases (37). Unlike these prior studies, a risk score model was herein developed based on the identification of BMRGs, with 224 BM_DEGs ultimately having been observed in BRCA patients. A risk score model was utilized to quantify the effects of BM_DEGs on BRCA patient outcomes, with two external datasets being employed for model validation. In univariate analyses, risk scores, stage, N, age, and M were all correlated with patient OS, while just risk scores, stage, and age were independently related to OS in multivariate analyses. These three variables were then combined to establish a predictive nomogram to assess BRCA patient OS, and calibration curves demonstrated that this nomogram yielded predictions of 1-, 3-, and 5-year OS consistent with actual patient outcomes.
This study is subject to a few important limitations that warrant consideration, including the fact that the dataset of this study is derived from BRCA patients where the occurrence of BM is not explicitly known, while the BMRGs are obtained from literature concerning BRCA patients who have definitively experienced BM. This difference could potentially introduce a confounding factor. Besides, although having the ability to predict the survival of BRCA patients, this study does not extend its capability to forecasting BM. Furthermore, the multivariate analysis did not incorporate the molecular subtype (luminal, HER2, or triple-negative), which holds significance as a crucial prognostic factor.
Conclusions
Overall, this study analyzed the impact of BMRGs on BRCA and successfully generated a clinical predictive model exhibiting superior sensitivity and specificity on the evaluation of BRCA patients.
Acknowledgments
Funding: This study was supported by
Footnote
Reporting Checklist: The authors have completed the TRIPOD reporting checklist. Available at https://tcr.amegroups.com/article/view/10.21037/tcr-23-1123/rc
Peer Review File: Available at https://tcr.amegroups.com/article/view/10.21037/tcr-23-1123/prf
Conflicts of Interest: All authors have completed the ICMJE uniform disclosure form (available at https://tcr.amegroups.com/article/view/10.21037/tcr-23-1123/coif). The authors have no conflicts of interest to declare.
Ethical Statement: The authors are accountable for all aspects of the work in ensuring that questions related to the accuracy or integrity of any part of the work are appropriately investigated and resolved. The study was conducted in accordance with the Declaration of Helsinki (as revised in 2013).
Open Access Statement: This is an Open Access article distributed in accordance with the Creative Commons Attribution-NonCommercial-NoDerivs 4.0 International License (CC BY-NC-ND 4.0), which permits the non-commercial replication and distribution of the article with the strict proviso that no changes or edits are made and the original work is properly cited (including links to both the formal publication through the relevant DOI and the license). See: https://creativecommons.org/licenses/by-nc-nd/4.0/.
References
- Yousefi M, Bahrami T, Salmaninejad A, et al. Lung cancer-associated brain metastasis: Molecular mechanisms and therapeutic options. Cell Oncol (Dordr) 2017;40:419-41. [Crossref] [PubMed]
- Hosonaga M, Saya H, Arima Y. Molecular and cellular mechanisms underlying brain metastasis of breast cancer. Cancer Metastasis Rev 2020;39:711-20. [Crossref] [PubMed]
- Kim YJ, Kim JS, Kim IA. Molecular subtype predicts incidence and prognosis of brain metastasis from breast cancer in SEER database. J Cancer Res Clin Oncol 2018;144:1803-16. [Crossref] [PubMed]
- Bartmann C, Wischnewsky M, Stüber T, et al. Pattern of metastatic spread and subcategories of breast cancer. Arch Gynecol Obstet 2017;295:211-23. [Crossref] [PubMed]
- Murawa D, Nowaczyk P, Szymkowiak M, et al. Brain metastasis as the first symptom of gastric cancer--case report and literature review. Pol Przegl Chir 2013;85:401-6. [Crossref] [PubMed]
- Sun YS, Zhao Z, Yang ZN, et al. Risk Factors and Preventions of Breast Cancer. Int J Biol Sci 2017;13:1387-97. [Crossref] [PubMed]
- Pedrosa RMSM, Mustafa DA, Soffietti R, et al. Breast cancer brain metastasis: molecular mechanisms and directions for treatment. Neuro Oncol 2018;20:1439-49. [Crossref] [PubMed]
- Jia Q, Chu H, Jin Z, et al. High-throughput single-cell sequencing in cancer research. Signal Transduct Target Ther 2022;7:145. [Crossref] [PubMed]
- Li Q, Pan Y, Cao Z, et al. Comprehensive Analysis of Prognostic Value and Immune Infiltration of Chromobox Family Members in Colorectal Cancer. Front Oncol 2020;10:582667. [Crossref] [PubMed]
- Zhang X, Yin X, Zhang H, et al. Differential expressions of PD-1, PD-L1 and PD-L2 between primary and metastatic sites in renal cell carcinoma. BMC Cancer 2019;19:360. [Crossref] [PubMed]
- Silva TC, Colaprico A, Olsen C, et al. TCGA Workflow: Analyze cancer genomics and epigenomics data using Bioconductor packages. F1000Res 2016;5:1542. [Crossref] [PubMed]
- Mayakonda A, Lin DC, Assenov Y, et al. Maftools: efficient and comprehensive analysis of somatic variants in cancer. Genome Res 2018;28:1747-56. [Crossref] [PubMed]
- Wilhite SE, Barrett T. Strategies to explore functional genomics data sets in NCBI's GEO database. Methods Mol Biol 2012;802:41-53. [Crossref] [PubMed]
- Clarke C, Madden SF, Doolan P, et al. Correlating transcriptional networks to breast cancer survival: a large-scale coexpression analysis. Carcinogenesis 2013;34:2300-8. [Crossref] [PubMed]
- Dedeurwaerder S, Desmedt C, Calonne E, et al. DNA methylation profiling reveals a predominant immune component in breast cancers. EMBO Mol Med 2011;3:726-41. [Crossref] [PubMed]
- Barrett T, Wilhite SE, Ledoux P, et al. NCBI GEO: archive for functional genomics data sets--update. Nucleic Acids Res 2013;41:D991-5. [Crossref] [PubMed]
- Horak CE, Pusztai L, Xing G, et al. Biomarker analysis of neoadjuvant doxorubicin/cyclophosphamide followed by ixabepilone or Paclitaxel in early-stage breast cancer. Clin Cancer Res 2013;19:1587-95. [Crossref] [PubMed]
- Morgan AJ, Giannoudis A, Palmieri C. The genomic landscape of breast cancer brain metastases: a systematic review. Lancet Oncol 2021;22:e7-e17. [Crossref] [PubMed]
- Wilkerson MD, Hayes DN. ConsensusClusterPlus: a class discovery tool with confidence assessments and item tracking. Bioinformatics 2010;26:1572-3. [Crossref] [PubMed]
- Brière G, Darbo É, Thébault P, et al. Consensus clustering applied to multi-omics disease subtyping. BMC Bioinformatics 2021;22:361. [Crossref] [PubMed]
- Hänzelmann S, Castelo R, Guinney J. GSVA: gene set variation analysis for microarray and RNA-seq data. BMC Bioinformatics 2013;14:7. [Crossref] [PubMed]
- Liberzon A, Birger C, Thorvaldsdóttir H, et al. The Molecular Signatures Database (MSigDB) hallmark gene set collection. Cell Syst 2015;1:417-25. [Crossref] [PubMed]
- Ritchie ME, Phipson B, Wu D, et al. limma powers differential expression analyses for RNA-sequencing and microarray studies. Nucleic Acids Res 2015;43:e47. [Crossref] [PubMed]
- Gaudet P, Škunca N, Hu JC, et al. Primer on the Gene Ontology. Methods Mol Biol 2017;1446:25-37. [Crossref] [PubMed]
- Slizen MV, Galzitskaya OV. Comparative Analysis of Proteomes of a Number of Nosocomial Pathogens by KEGG Modules and KEGG Pathways. Int J Mol Sci 2020;21:7839. [Crossref] [PubMed]
- Wu T, Hu E, Xu S, et al. clusterProfiler 4.0: A universal enrichment tool for interpreting omics data. Innovation (Camb) 2021;2:100141. [Crossref] [PubMed]
- Innis SE, Reinaltt K, Civelek M, et al. GSEAplot: A Package for Customizing Gene Set Enrichment Analysis in R. J Comput Biol 2021;28:629-31. [Crossref] [PubMed]
- Charoentong P, Finotello F, Angelova M, et al. Pan-cancer Immunogenomic Analyses Reveal Genotype-Immunophenotype Relationships and Predictors of Response to Checkpoint Blockade. Cell Rep 2017;18:248-62. [Crossref] [PubMed]
- Jiang P, Gu S, Pan D, et al. Signatures of T cell dysfunction and exclusion predict cancer immunotherapy response. Nat Med 2018;24:1550-8. [Crossref] [PubMed]
- Engebretsen S, Bohlin J. Statistical predictions with glmnet. Clin Epigenetics 2019;11:123. [Crossref] [PubMed]
- Árkosy P, Tóth J, Béres E, et al. Prognosis and Treatment Outcomes of Patients Undergoing Resection of Brain Metastases from Breast Cancer. Anticancer Res 2020;40:1759-70. [Crossref] [PubMed]
- Epaillard N, Bassil J, Pistilli B. Current indications and future perspectives for antibody-drug conjugates in brain metastases of breast cancer. Cancer Treat Rev 2023;119:102597. [Crossref] [PubMed]
- McMahon JT, Faraj RR, Adamson DC. Emerging and investigational targeted chemotherapy and immunotherapy agents for metastatic brain tumors. Expert Opin Investig Drugs 2020;29:1389-406. [Crossref] [PubMed]
- Otani R, Sadato D, Yamada R, et al. CHD5 gene variant predicts leptomeningeal metastasis after surgical resection of brain metastases of breast cancer. J Neurooncol 2023;163:657-62. [Crossref] [PubMed]
- Liu Q, Kong X, Wang Z, et al. NCCBM, a Nomogram Prognostic Model in Breast Cancer Patients With Brain Metastasis. Front Oncol 2021;11:642677. [Crossref] [PubMed]
- Cheng X, Xia L, Sun S. A pre-operative MRI-based brain metastasis risk-prediction model for triple-negative breast cancer. Gland Surg 2021;10:2715-23. [Crossref] [PubMed]
- Gao Y, Liu J, Qian X, et al. Identification of markers associated with brain metastasis from breast cancer through bioinformatics analysis and verification in clinical samples. Gland Surg 2021;10:924-42. [Crossref] [PubMed]