Metabolomics studies of prostate cancer using gas chromatography-mass spectrometery
IntroductionOther Section
There have been numerous clinical studies into the methods for early tumor diagnosis, including identifying tumor markers and pathology, ultrasonic techniques, and nuclear magnetic resonance. Despite these advances, many patients are still diagnosed with advanced or metastatic cancer after the optimum treatment point (1,2). Therefore, identification of additional biomarkers or development of new technologies that allow early diagnosis of cancers remains an important issue.
Recently, metabolomics has attracted increasing attention and been widely used for early cancer diagnosis (3). The occurrence and development of tumors in the human body inevitably cause changes in metabolites, and nucleotide metabolism has been suggested as a cancer marker (4). In clinical practice, more than three-quarters of patients with ovarian cancer were diagnosed with advanced cancer and must undergo surgical removal and chemotherapy. Even with intensive therapy, about one-half of these patients experienced relapse within 2 years (5). However, early diagnosis of ovarian cancer would greatly improve the patient survival rate (6). A previous study showed that combination of the tumor markers CA125, CA72-4, CA15-3, and M-CSF and detection through artificial neural network analysis improved the accuracy of early diagnosis of ovarian cancer; the specificity of this method reached 98% with 71% sensitivity compared to 46% sensitivity for CA125 alone (7). In addition, metabolomics testing has been applied in the early diagnosis of breast cancer, illustrating that metabolomics analysis can provide broader more screening indicators (8).
Prostate cancer mainly occurs in middle-aged men and is often diagnosed at late stage. Thus, early diagnosis of prostate cancer would have far-reaching significance for patients (9,10). With the aid of high-throughput liquid/gas chromatography-mass spectrometry (LC/GC-MS), a metabolomics study performed on 262 clinical prostate cancer specimens identified 1,126 intermediate metabolites (10). For example, creatinine levels gradually increased from early to metastatic prostate cancer, and sarcosine was detected in rectal specimens from patients with prostate cancer, suggesting that LC/GC-MS is an effective method for early diagnosis and clinical staging of prostate cancer.
Current cancer therapeutics is mainly directed against tumor cells, targeting development and growth processes, proliferation, and metastasis arising from abnormal pathways. Metabolomics can help to identify key metabolites during the development of the tumor and improve the efficacy by tumor targeted therapy, thereby establishing a new direction for research and clinical applications (11). The most important evaluation of this application is identification of metabolic biomarkers for cancer diagnosis and prognosis. Recently, the application of magnetic resonance spectroscopy imaging (MRSI) technologies for tumor evaluation has attracted a lot of attention (12). However, in clinical work, the co-existence of breast cancer with metabolic or other integrated disease such as insulin resistance, obesity, hypertension, or dyslipidemia will result in relatively poor diagnosis (13).
In this study, metabolic differences between normal prostate epithelial cells and prostate cancer cells at various stages were analyzed with GC-MS to identify prostate cancer-specific metabolites. The analysis of prostate cancer markers with diagnostic significance will provide more meaningful tumor biomarkers for prostate cancer diagnosis and treatment, further our understanding of prostate cancer pathogenesis, and establish a foundation for new ideas.
MethodsOther Section
Materials
Reagents for cell growth media (e.g., Leibovitz’s L-15 medium, RPMI medium 1640, epidermal growth factor, Ham’s F-12 nutrient mixture, McCoy’s 5A medium) and methanol were purchased from CNW Technologies GmbH (Germany). Other commercially available reagents were analytical grade. Derivatives reagents were purchased from AMP (USA). Watsons distilled water was used for all experimental equipment.
Growth medium
Dulbecco’s Modified Eagle’s Medium (DMEM) containing 80–90% basal medium supplemented with 10–20% fetal bovine serum and 1% bis anti-stock solution (penicillin and streptomycin) were purchased from Gibco (USA). The final concentrations of penicillin and streptomycin were 100 U/mL and 100 µg/mL, respectively (10).
Cell culture
Prostate cancer cell lines PC-3, DU145, and LNCaP and the normal prostate epithelial cell line RWPE were purchased from the American Type Culture Collection (ATCC, USA). The cell lines were cultured in DMEM at 37 °C in 5% CO2 under sterile conditions as previously described (14,15). The filtered culture supernatant was stored in a sealed bottle at 4 °C (16).
Sample grouping
The samples of normal human prostate epithelial cells (RWPE cells), normal human prostate epithelial extracellular fluid (RWPE solution), androgen-independent human prostate cancer cells (DU145 cells), androgen-independent human prostate cancer cells extracellular fluid (DU145 solution), androgen-dependent LNCaP human prostate cancer cells (LNCaP cells), androgen-dependent LNCaP human prostate cancer cells extracellular fluid (LNCaP solution), androgen-independent PC-3 human prostate cancer cells (PC-3 cells), and androgen-independent PC-3 human prostate cancer cells extracellular fluid (PC-3 solution) were divided into groups 1, 2, 3, 4, 5, 6, 7 and 8, respectively (n=2 each). Group 9 was included as a quality control and indicates instrument stability during the detection process.
GC-MS analysis
A 200-µL sample was used for GC-MS metabolomic analysis on an Agilent 7890A/5975C GC-MS as previously described (17) using an Agilent J&W HP-5ms capillary column (30 m × 0.25 mm × 0.25 µm). Instrument parameters were set as follows: inlet temperature, 280 °C; electrospray ionization ion source temperature, 230 °C; quadrupole temperature, 150 °C; carrier gas, high-purity helium (purity greater than 99.999%); splitless injection volume, 1.0 µL (18). The following temperature program was used: 80 °C for 2 min, increase to 320 °C at 10 °C/min, and maintain at 320 °C for 6 min. Mass spectra were collected in full scan mode from m/z 50–550. Random sequences of consecutive samples were analyzed to avoid random errors from instrumental signal fluctuations (19). Calculation of the fold change: First, we calculated the difference in the mean value of the peak intensity for metabolites in two groups and the ratio between the mean value of the two groups. Then we calculated the log2 value of the ratio. The final result indicates the fold change. Positive values indicate significant metabolites differences between the two groups, and negative values indicate the opposite.
Data analysis
The data were analyzed in R using the XCMS software package for GC-MS data preprocessing (20), including removal of column bleed and impurity peaks introduced during sample preparation. The final result was stored as a two-dimensional data matrix including each observable (sample) and peak intensities (21). All data were normalized to the total signal integration. The data matrix was edited using Simca-P software (version 13.0) and analyzed using principal component analysis (PCA). The data of the metabolomes in extracellular and intracellular fluid were analyzed by PCA, partial least squares discriminant analysis (PLS-DA), and Student’s t-test. A P value <0.05 was considered significant.
ResultsOther Section
Overall analysis
We recovered 1,474 samples of fragment ion. Metabolic differences within a group and comparisons between samples were determined by body composition analysis. The most intuitive data format is unit variance (UV) scaling and mean-centering after formal analysis in Simca-P. However, differences between groups were analyzed using PCA. We identified two main components with cumulative R2X =0.568 and Q2 =0.409. The PCA score plot is shown in Figure 1. R2X is a non-supervisory metric that indicates the quality of the PCA model for identifying the main parameters. When R2X is greater than 0.4, the modeling is reliable. Differences in metabolism can be explained using PCA in combination with relative PLS-DA to reflect real differences in the experimental group in the middle of the figure (Figure 1).
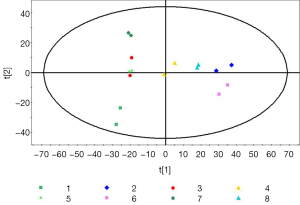
Metabolic differences between groups 1 and 2
The differences of metabolites were compared between group 1 (RWPE cells) and group 2 (RWPE solution). PCA identified two main components accounting for metabolic differences between the groups with cumulative R2X =0.918 and Q2 =0.612. To uncover any significant differences in metabolite levels, we used supervisory PLS-DA. The PLS-DA score plot showed significant differences between the two groups, and we calculated the variable influence on projection (VIP) scores using an orthogonal (O) PLS-DA model and P values using Student’s t-test. VIP >1 and P<0.05 were taken as the thresholds for significance. We identified more than 42 differentially expressed metabolites, mainly sugars, organic acids, and amino acids (Table 1), between the groups, indicating differences between the intracellular fluid and extracellular fluid metabolomes of normal prostate epithelial cells.
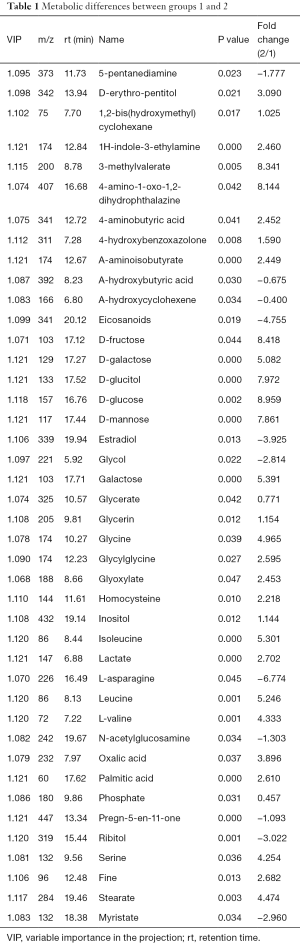
Full table
Metabolic differences between groups 1 and 3
Group 1 was compared with group 3 (DU145 cells) to reveal any differences between the intracellular fluid metabolites. PCA revealed two main components with cumulative R2X =0.862 and Q2 =0.341. To reveal any significant differences between the metabolomes of the two groups, further analysis was performed using supervisory PLS-DA. The PLS-DA score plot showed significant differences between the two groups (P<0.05), indicating three main components and a quadrature component specific to the metabolites, and yielded more than 23 differentially expressed metabolites (Table 2), including cholesterol, stearic acid, 4-aminobutyric acid, and estradiol.
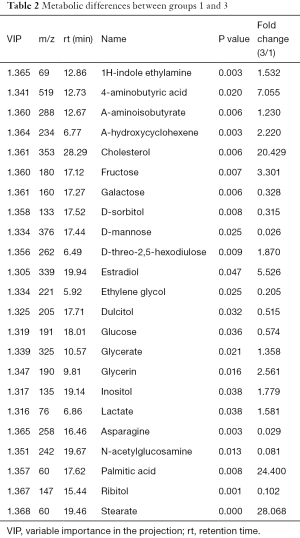
Full table
Metabolic differences between groups 1 and 5
The differences of intracellular fluid metabolites between group 1 and group 5 (LNCaP cells) were compared. PCA determined two main components with cumulative R2X =0.859 and Q2 =0.355, revealing metabolic differences between the groups. PLS-DA further revealed more than 46 metabolites that were significantly different between the two groups (Table 3), including cholesterol, glycine, homocysteine, proline, and serine.
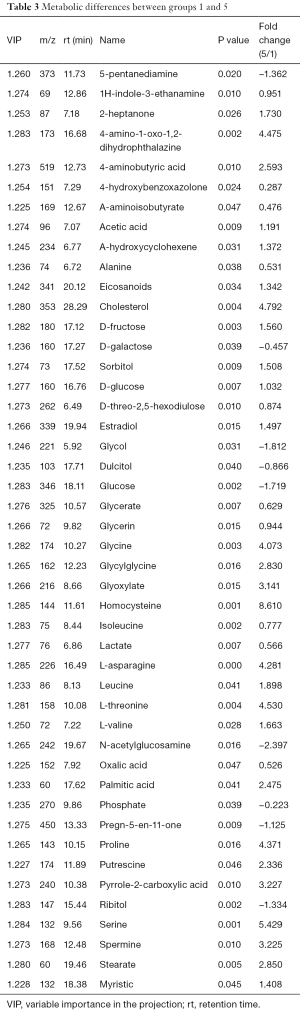
Full table
Metabolic differences between groups 1 and 7
The differences of intracellular fluid metabolites between group 1 and group 7 (PC-3 cells) were compared. PCA determined two main components with cumulative R2X =0.88 and Q2 =0.425. The PLS-DA score plot revealed significant differences (P<0.05) with two main components and identified more than 36 significant metabolite differences (Table 4), with the largest differences for glucose, homocysteine, and serine.
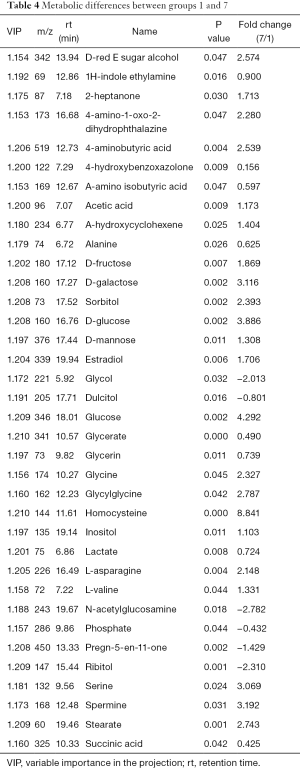
Full table
Metabolic differences between groups 2 and 4
The differences of extracellular fluid metabolites fluid metabolites between group 2 and group 4 (DU145 solution) were compared. PCA determined two main principal components with cumulative R2X =0.83 and Q2 =0.183. The PLS-DA score plot showed significant differences between the groups and received a master component and a quadrature component (Table 5). The appearance of the master component indicates that the main component in the metabolic compound has been extracted, and the quadrature component represents the removal of interferents. The OPLS-DA model identified more than 20 differential metabolites, with significantly elevated cholesterol in group 4 (P<0.05).
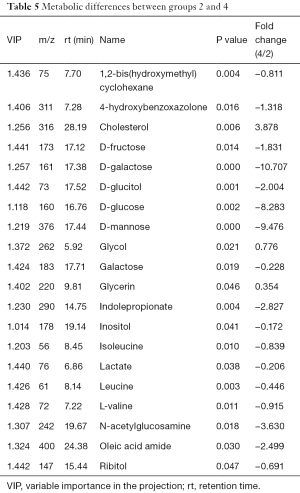
Full table
Metabolic differences between groups 2 and 6
The differences of extracellular metabolites between group 2 and group 6 (LNCaP solution) were compared. PCA determined two main components with cumulative R2X =0.822 and Q2 =−0.0154, indicating significant metabolic differences between the two groups (P<0.05). The PLS-DA score plot indicated significant difference between the two groups and received a master component and a quadrature component (Table 6). Fifteen metabolites were significantly increased in group 6 relative to group 2, including cholesterol, serine, alanine, and saccharides.
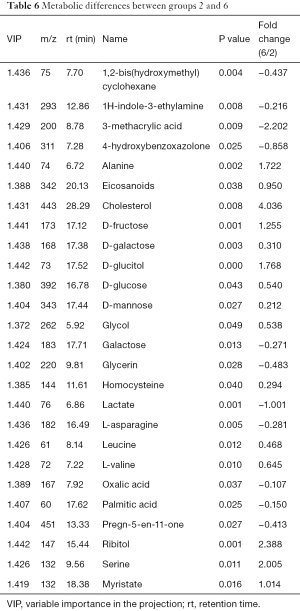
Full table
Metabolic differences between groups 2 and 8
The differences of extracellular metabolites between group 2 and group 8 (PC-3 solution) were compared. Two principal components were obtained with cumulative R2X =0.888 and Q2 =0.54 by PCA. The PLS-DA score plot demonstrated significant differences (P<0.05) between the two groups and received a master component and a quadrature component. More than 30 significantly different metabolites were identified (Table 7) with 10 increased in group 8, including cholesterol, alanine, serine, stearic acid, arachidic acid, and carbohydrates.
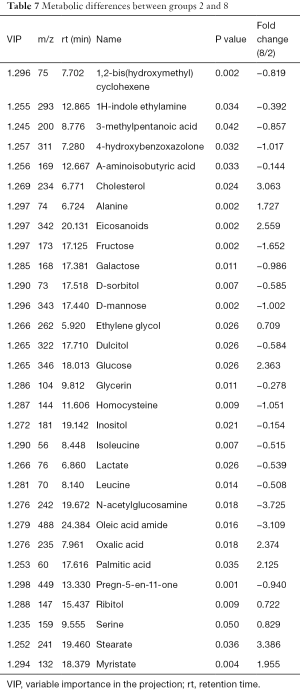
Full table
Metabolic differences between groups 3 and 4
The differences of metabolites were compared between group 3 and group 4. PCA determined two main components with cumulative R2X =0.823 and Q2 =0.102, indicating significant metabolic differences between the two groups (P<0.05). Furthermore, the PLS-DA score plot revealed significant differences and received a master component and a quadrature component. The OPLS-DA model identified 27 significantly different metabolites (Table 8), with galactose, sugars, leucine, L-valine, and phosphoric acid significantly increased.
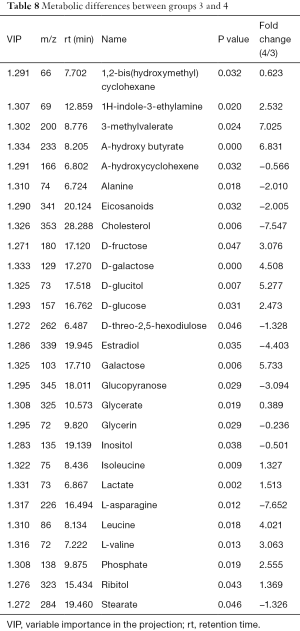
Full table
Metabolic differences between groups 5 and 6
The differences of metabolites were compared between group 5 and group 6. PCA determined two main components with cumulative R2X =0.898 and Q2 =0.521, indicating significant metabolic differences between the two groups (P<0.05). A PLS-DA score plot showed significant differences and received a master component and a quadrature component. More than 37 differentially expressed metabolites were identified (Table 9), with 20 increased in the extracellular fluid, including sugars, L-valine, leucine, and 3-methylvaleric acid.
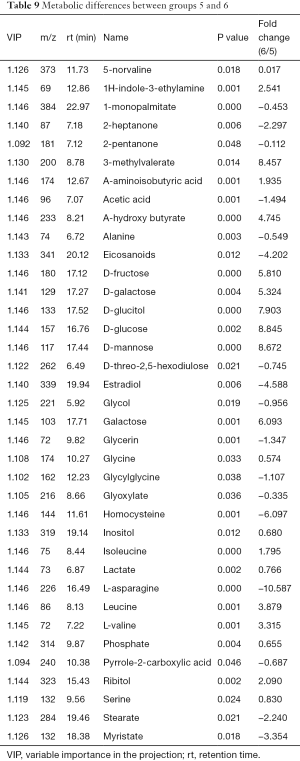
Full table
Metabolic differences between groups 7 and 8
The differences of metabolites were compared between group 7 and group 8. PCA determined two main components with cumulative R2X =0.921 and Q2 =0.661. The PLS-DA score plot indicated significant differences between the two groups and received a master component and a quadrature component. The OPLS-DA model identified more than 40 differences in metabolites (Table 10), including carbohydrates, glycine, leucine, serine, L-threonine, and other organic acids.
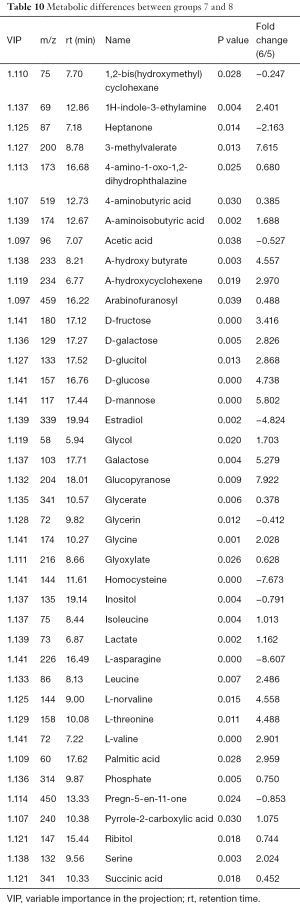
Full table
DiscussionOther Section
Metabolomics can reveal the metabolic microenvironment of cells, thereby identifying changes in gene regulation and metabolic activity and reactions (22,23). Tumor cell metabolism is inseparable from the surroundings because tumor cell growth requires key metabolites from the microenvironment (24-27). In evaluating the diagnosis, prognosis, and therapeutic efficacy for a disease, biomarkers play an increasingly important role. GC-MS-based metabolomics analyses have potential effects in evaluating the development of cancer through metabolic screening and earlier identification of more sensitive diagnostic markers (24-27). In this study, we found significant differences in metabolite expression between prostate cancer cells and normal prostate epithelial cells. These biomarkers, including cholesterol, amino acid, homocysteine, proline, serine, alanine, and saccharides, showed significantly different average levels between tumor groups and the normal control group; detection of the intracellular and extracellular fluids showed good consistency.
Cholesterol was identified with a relatively high significance in all comparisons. Therefore, we focused on the role of cholesterol metabolism in the growth of prostate cancer cells. Cholesterol is an important building block for cell membranes, synthesis of bile acids, vitamin D, raw steroid hormones, and their derivatives (28). Acetyl-CoA is the starting material for forming isopentenyl pyrophosphate (IPP), which is converted into squalene and subsequently into cholesterol. As a synthetic steroid hormone precursor, cholesterol can be enzymatically converted into androgens, which may affect the androgen receptor signaling pathway and promote the acceptance of androgen deprivation therapy for prostate cancer patients with castration-resistant tumor growth (29,30). High physiological cholesterol levels can promote androgen synthesis in the prostate gland, and studies have demonstrated a connection between the occurrence of prostate cancer and high cholesterol levels (31). Rapid reproduction of tumor cells might also be associated with high cholesterol levels, as previous studies showed that artificially raising cholesterol levels promoted prostate cancer proliferation, migration, and invasion, whereas lowering cholesterol levels and interfering with growth factor signaling pathways could induce tumor cell apoptosis (29,30).
Glucose was also significantly increased in prostate cancer groups herein. A previous report indicated that patients with diabetes for 2–5 years exhibited increased incidence of prostate cancer; thus, patients with diabetes should pay attention to cholesterol levels in screening for prostate cancer (32). Because tumor cell energy metabolism occurs mainly through anaerobic glycolysis of glucose, hyperglycemia will promote tumor cell growth. Furthermore, long-term exposure to elevated blood sugar levels can gradually thicken the basement membrane of capillaries, decrease the permeability, impair mitochondrial respiratory enzymes and cellular respiration barriers, and increase glycolysis, which ultimately selects for tumor cells (33).
The serine protease hepsin and cysteine-rich secretory protein 3 (CRISP3) have been reported as diagnostic markers for prostate cancer. Immunohistochemical studies demonstrated high concentrations of serine protease in prostate cancer and prostatic intraepithelial neoplasia (PIN) (34). CRISP3 is a secreted protein produced in the male reproductive tract and is present at high levels in seminal plasma samples. Immunohistochemical staining of prostate tissue showed high levels of CRISP3 in PIN and prostate cancer samples. The relationship between CRISP3 and prostate cancer accompanied by radical prostatectomy in patients with tissue micro-β1 protamine was evaluated, and CRISP3 was identified as an independent predictor for prostate cancer recurrence (35,36).
In summary, our results showed that GC-MS-based metabolomics comparison of normal prostate epithelial cells and prostate cancer cells could reveal similarities and differences in prostate cancer-specific capture. Our OPLS-DA pairwise modeling analysis, combined with Student’s t-test, identified significant differences in metabolite expression, including amino acids, organic acids, sugars, and cholesterol. Of the four categories, combined with prostate-specific antigen (PSA), cholesterol showed obvious potential as a biomarker for clinical diagnosis of prostate cancer. Furthermore, our pairwise comparisons of the extracellular fluid and intracellular fluid of normal and cancer cells reflected the differences between normal prostate epithelial cells and prostate cancer cells, which could reduce the necessity for invasive biopsy. Thus, metabolomics studies of prostate cancer using GC-MS can help identify more meaningful tumor markers for prostate cancer diagnosis and treatment, facilitate exploration of the pathogenesis of prostate cancer, and provide the basis for new ideas.
AcknowledgmentsOther Section
Funding: This work was supported by grants from the Heilongjiang Province Natural Science Fund Project (No. D201225) and Research Subject of Jiamusi University (No. 12Z1201504, Sz2010-004).
FootnoteOther Section
Conflicts of Interest: All authors have completed the ICMJE uniform disclosure form (available at http://dx.doi.org/10.21037/tcr.2016.06.15). The authors have no conflicts of interest to declare.
Ethical Statement: The authors are accountable for all aspects of the work in ensuring that questions related to the accuracy or integrity of any part of the work are appropriately investigated and resolved. The study was conducted in accordance with the Declaration of Helsinki (as revised in 2013).
Open Access Statement: This is an Open Access article distributed in accordance with the Creative Commons Attribution-NonCommercial-NoDerivs 4.0 International License (CC BY-NC-ND 4.0), which permits the non-commercial replication and distribution of the article with the strict proviso that no changes or edits are made and the original work is properly cited (including links to both the formal publication through the relevant DOI and the license). See: https://creativecommons.org/licenses/by-nc-nd/4.0/.
ReferencesOther Section
- Mäbert K, Cojoc M, Peitzsch C, et al. Cancer biomarker discovery: current status and future perspectives. Int J Radiat Biol 2014;90:659-77. [Crossref] [PubMed]
- Rubin G, Berendsen A, Crawford SM, et al. The expanding role of primary care in cancer control. Lancet Oncol 2015;16:1231-72. [Crossref] [PubMed]
- Athersuch T. Metabolome analyses in exposome studies: Profiling methods for a vast chemical space. Arch Biochem Biophys 2016;589:177-86. [Crossref] [PubMed]
- Cheng YS, Xu F. Anticancer function of polyinosinic-polycytidylic acid. Cancer Biol Ther 2010;10:1219-23. [Crossref] [PubMed]
- Donahue RN, McLaughlin PJ, Zagon IS. Low-dose naltrexone suppresses ovarian cancer and exhibits enhanced inhibition in combination with cisplatin. Exp Biol Med (Maywood) 2011;236:883-95. [Crossref] [PubMed]
- Menon U, Jacobs IJ. Recent developments in ovarian cancer screening. Curr Opin Obstet Gynecol 2000;12:39-42. [Crossref] [PubMed]
- Zhang Z, Yu Y, Xu F, et al. Combining multiple serum tumor markers improves detection of stage I epithelial ovarian cancer. Gynecol Oncol 2007;107:526-31. [Crossref] [PubMed]
- Chan DA, Sutphin PD, Nguyen P, et al. Targeting GLUT1 and the Warburg effect in renal cell carcinoma by chemical synthetic lethality. Sci Transl Med 2011;3:94ra70 [Crossref] [PubMed]
- Beheshti M, Vali R, Waldenberger P, et al. Detection of bone metastases in patients with prostate cancer by 18F fluorocholine and 18F fluoride PET-CT: a comparative study. Eur J Nucl Med Mol Imaging 2008;35:1766-74. [Crossref] [PubMed]
- Sreekumar A, Poisson LM, Rajendiran TM, et al. Metabolomic profiles delineate potential role for sarcosine in prostate cancer progression. Nature 2009;457:910-4. [Crossref] [PubMed]
- Evelhoch J, Garwood M, Vigneron D, et al. Expanding the use of magnetic resonance in the assessment of tumor response to therapy: workshop report. Cancer Res 2005;65:7041-4. [Crossref] [PubMed]
- Rosen MA, Schnall MD. Dynamic contrast-enhanced magnetic resonance imaging for assessing tumor vascularity and vascular effects of targeted therapies in renal cell carcinoma. Clin Cancer Res 2007;13:770s-776s. [Crossref] [PubMed]
- Healy LA, Ryan AM, Carroll P, et al. Metabolic syndrome, central obesity and insulin resistance are associated with adverse pathological features in postmenopausal breast cancer. Clin Oncol (R Coll Radiol) 2010;22:281-8. [Crossref] [PubMed]
- Giangreco AA, Dambal S, Wagner D, et al. Differential expression and regulation of vitamin D hydroxylases and inflammatory genes in prostate stroma and epithelium by 1,25-dihydroxyvitamin D in men with prostate cancer and an in vitro model. J Steroid Biochem Mol Biol 2015;148:156-65. [Crossref] [PubMed]
- Mikyšková R, Štěpánek I, Indrová M, et al. Dendritic cells pulsed with tumor cells killed by high hydrostatic pressure induce strong immune responses and display therapeutic effects both in murine TC-1 and TRAMP-C2 tumors when combined with docetaxel chemotherapy. Int J Oncol 2016;48:953-64. [PubMed]
- Phuyal S, Skotland T, Hessvik NP, et al. The ether lipid precursor hexadecylglycerol stimulates the release and changes the composition of exosomes derived from PC-3 cells. J Biol Chem 2015;290:4225-37. [Crossref] [PubMed]
- Wilton JH, Titus MA, Efstathiou E, et al. Androgenic biomarker prof|ling in human matrices and cell culture samples using high throughput, electrospray tandem mass spectrometry. Prostate 2014;74:722-31. [Crossref] [PubMed]
- Ueda K, Tatsuguchi A, Saichi N, et al. Plasma low-molecular-weight proteome profiling identified neuropeptide-Y as a prostate cancer biomarker polypeptide. J Proteome Res 2013;12:4497-506. [Crossref] [PubMed]
- Brennan JC, Denison MS, Holstege DM, et al. 2,3-cis-2R,3R-(-)-epiafzelechin-3-O-p-coumarate, a novel flavan-3-ol isolated from Fallopia convolvulus seed, is an estrogen receptor agonist in human cell lines. BMC Complement Altern Med 2013;13:133. [Crossref] [PubMed]
- Uppal K, Soltow QA, Strobel FH, et al. xMSanalyzer: automated pipeline for improved feature detection and downstream analysis of large-scale, non-targeted metabolomics data. BMC Bioinformatics 2013;14:15. [Crossref] [PubMed]
- Huang X, Chen YJ, Cho K, et al. X13CMS: global tracking of isotopic labels in untargeted metabolomics. Anal Chem 2014;86:1632-9. [Crossref] [PubMed]
- Mendes P, Kell DB, Westerhoff HV. Channelling can decrease pool size. Eur J Biochem 1992;204:257-66. [Crossref] [PubMed]
- Mendes P, Kell DB, Westerhoff HV. Why and when channelling can decrease pool size at constant net flux in a simple dynamic channel. Biochim Biophys Acta 1996;1289:175-86. [Crossref] [PubMed]
- Azmi AS, Bao B, Sarkar FH. Exosomes in cancer development, metastasis, and drug resistance: a comprehensive review. Cancer Metastasis Rev 2013;32:623-42. [Crossref] [PubMed]
- Hannafon BN, Ding WQ. Intercellular communication by exosome-derived microRNAs in cancer. Int J Mol Sci 2013;14:14240-69. [Crossref] [PubMed]
- Roma-Rodrigues C, Fernandes AR, Baptista PV. Exosome in tumour microenvironment: overview of the crosstalk between normal and cancer cells. Biomed Res Int 2014;2014:179486.
- Yu DD, Wu Y, Shen HY, et al. Exosomes in development, metastasis and drug resistance of breast cancer. Cancer Sci 2015;106:959-64. [Crossref] [PubMed]
- Charlton-Menys V, Durrington PN. Human cholesterol metabolism and therapeutic molecules. Exp Physiol 2008;93:27-42. [Crossref] [PubMed]
- Dillard PR, Lin MF, Khan SA. Androgen-independent prostate cancer cells acquire the complete steroidogenic potential of synthesizing testosterone from cholesterol. Mol Cell Endocrinol 2008;295:115-20. [Crossref] [PubMed]
- Montgomery RB, Mostaghel EA, Vessella R, et al. Maintenance of intratumoral androgens in metastatic prostate cancer: a mechanism for castration-resistant tumor growth. Cancer Res 2008;68:4447-54. [Crossref] [PubMed]
- Gribbestad IS, Sitter B, Lundgren S, et al. Metabolite composition in breast tumors examined by proton nuclear magnetic resonance spectroscopy. Anticancer Res 1999;19:1737-46. [PubMed]
- Bjelakovic G, Gluud LL, Nikolova D, et al. Vitamin D supplementation for prevention of mortality in adults. Cochrane Database Syst Rev 2014;CD007470 [PubMed]
- Lappe JM, Travers-Gustafson D, Davies KM, et al. Vitamin D and calcium supplementation reduces cancer risk: results of a randomized trial. Am J Clin Nutr 2007;85:1586-91. [PubMed]
- Bathen TF, Jensen LR, Sitter B, et al. MR-determined metabolic phenotype of breast cancer in prediction of lymphatic spread, grade, and hormone status. Breast Cancer Res Treat 2007;104:181-9. [Crossref] [PubMed]
- Bjartell AS, Al-Ahmadie H, Serio AM, et al. Association of cysteine-rich secretory protein 3 and beta-microseminoprotein with outcome after radical prostatectomy. Clin Cancer Res 2007;13:4130-8. [Crossref] [PubMed]
- Schubert SM, Arendt LM, Zhou W, et al. Ultra-sensitive protein detection via Single Molecule Arrays towards early stage cancer monitoring. Sci Rep 2015;5:11034. [Crossref] [PubMed]