HTRA1 expression is associated with immune-cell infiltration and survival in breast cancer
Highlight box
Key findings
• Decreased high temperature requirement A1 (HTRA1) expression was found to be significantly associated with breast cancer (BRCA) and inferior survival of patients.
What is known and what is new?
• Previous studies on HTRA1 generally support its role as a tumor suppressor. However, contrasting findings also exist.
• Here, we report associations between HTRA1 expression and abundance of different types of tumor-associated immune cells and immuno-biomarkers in BRCA, including resting mast cells, follicular helper T cells, PD-L1, TP53, and Ki67. HTRA1 levels were also correlated to BRCA subtype, response to therapy, and survival.
What is the implication, and what should change now?
• The potential value of HTRA1 in BRCA is achieved by regulating tumor cell proliferation and tumor immunity. HTRA1 serves as a potential therapeutic target and prognostic biomarker. Further investigations would be necessary to depict the complicated regulatory effects of HTRA1 in BRCA in future.
Introduction
Breast cancer (BRCA) is currently the most frequently diagnosed cancer type and one of the leading causes of cancer-related deaths among women worldwide (1). In clinical practice, immunohistochemical staining for estrogen receptor (ER), progesterone receptor (PR), and erb-b2 receptor tyrosine kinase 2 (ERBB2)/human epidermal growth factor receptor 2 (HER2) are utilized as diagnostic markers to categorize BRCA into luminal A, luminal B, HER2-enriched (non-luminal), and basal [triple-negative breast cancer (TNBC)] subtypes (2). Advances in early detection, treatment, and promising therapeutic opportunities have significantly improved the clinical outcomes and survival rates of BRCA patients (3,4). However, the heterogeneity of BRCA subtypes contributes to varying clinicopathological characteristics, treatment options, prognoses, and recurrence patterns (5).
The composition of immune cells within the BRCA microenvironment plays a crucial role in disease progression and clinical outcome. Innate immune cells (macrophages, neutrophils, dendritic cells, innate lymphoid cells, myeloid-derived suppressor cells, and natural killer cells) and adaptive immune cells (T cells and B cells) within this microenvironment contribute cooperatively to tumor progression and therapeutic response (6). Therefore, elucidating the intricate interplay between molecular characteristics of BRCA and multiple immune cells within the tumor microenvironment can lead to favorable outcomes.
High temperature requirement A (HTRA) proteases are a family of serine proteases involved in various cellular processes (7), among which HTRA1 is the first identified and extensively studied member (8). It plays a crucial role in several fundamental physiological and pathological processes, such as cell proliferation and invasion, and cell adhesion (9). Current studies on HTRA1 generally support its role as a tumor suppressor. Decreased HTRA1 expression is associated with poor prognosis in ovarian cancer (10), metastatic melanoma (11), prostate cancer (12), lung cancer (13), endometrial cancer (14), and BRCA (15). However, the mechanisms underlying the effects of decreased HTRA1 expression and its role in tumor immune-cell infiltration, immune checkpoints, diagnosis, and prognosis of BRCA have not been fully elucidated. In this study, we conducted a comprehensive bioinformatics analysis utilizing The Cancer Genome Atlas (TCGA) (https://portal.gdc.cancer.gov/) and Gene Expression Omnibus (GEO) (https://www.ncbi.nlm.nih.gov/geo/) to assess the important effects of HTRA1 in BRCA (16). We present this article in accordance with the STREGA reporting checklist (available at https://tcr.amegroups.com/article/view/10.21037/tcr-23-773/rc).
Methods
The study was conducted in accordance with the Declaration of Helsinki (as revised in 2013).
Data collection
The RNAseq data in the Fragments Per Kilobase per Million format (level 3 HTSeq-FPKM) and the corresponding clinicopathological information of 1,222 samples (1,109 BRCA and 113 normal breast tissue samples, August 2021) were downloaded from the Breast Invasive Carcinoma Project in TCGA. Then the FPKM format data were converted into transcripts per million (TPM) reads for subsequent analysis. Two BRCA datasets, GSE109169 (https://www.ncbi.nlm.nih.gov/geo/query/acc.cgi?acc=GSE109169) (17) and GSE10780 (https://www.ncbi.nlm.nih.gov/geo/query/acc.cgi?acc=GSE10780) (18) from GEO database were also downloaded for validation of HTRA1 expression. GSE109169 was used to assess the gene expression profiles of 25 paired normal breast and tumor tissues based on the detection platform GPL5175 (HuEx-1_0-st) Affymetrix Human Exon 1.0 ST Array [transcript (gene) version]. GSE10780 was used to analyze the gene expression profiles of 143 normal breast tissues and 42 BRCA tissues based on the detection platform GPL570 (HG-U133_Plus_2) Affymetrix Human Genome U133 Plus 2.0 Array.
Analysis of HTRA1 mRNA expression level in BRCA and normal breast tissue samples
The expression level of HTRA1 mRNA was firstly compared with data from 33 types of human cancer extracted from TCGA (19). For TCGA-BRCA datasets, we further analyzed HTRA1 mRNA expression in tumor samples and their paired normal controls based on BRCA subtypes. The data of HTRA1 extracted from GSE109169 and GSE10780 datasets were used to validate TCGA-BRCA results. Then the TCGA-BRCA samples were divided into high- and low-HTRA1 expression group based on the median expression of HTRA1. Differentially expressed genes (DEGs) between the two groups were screened out and analyzed by “DESeq2” (v1.26.0) R package (20) with log fold change absolute value >1.5 and P value <0.05 as a threshold. The volcano plot and heat map of the DEGs were visualized using the “ggplot2” (v3.3.3) R package.
Functional enrichment analysis of HTRA1-associated DEGs in BRCA
The “ClusterProfiler” (v3.14.3) R package (21) was used for the functional annotation and Gene Set Enrichment Analysis (GSEA) (22) for the selected DEGs. The curated reference gene sets c2. cp.v7.2. symbols.gmt from MSigDB (http://www.gsea-msigdb.org/gsea/msigdb) were designated for GSEA (23). We identified significantly enriched clusters using false discovery rate (FDR) <0.25 and Padjust<0.05 as a threshold. A protein-protein interaction (PPI) analysis was performed using the STRING database (24) and visualized by Cytoscape software (v3.9.1) (25). The interaction threshold was set to 0.4.
Correlation analysis between HTRA1 expression, immune-cell infiltration, and immuno-related biomarkers in BRCA
The ssGSEA algorithm in the “GSVA” (v1.34.0) R package (26) was used to evaluate the tumor infiltration status of 24 types of immune cell (27). Spearman correlation analysis was performed to determine the relationship between HTRA1 expression, immune-cell infiltration status, and immunity-related biomarkers. Among them, PDCD1 [programmed cell death protein 1 (PD-1)], CD274 [programmed cell death ligand 1 (PD-L1)], CTLA4 and LAG3 are important immune checkpoint markers that are associated with tumor immune escape (28); TIGIT, HAVCR2, LAYN, and CXCL3 are T cell exhaustion markers; IFNG [interferon γ (IFN-γ)], IL2 [interleukin 2 (IL-2)], GZMB (granzyme B), and PRF1 (perforin) are T cell effector function markers. TP53 is a tumor suppressor (29). MKI67 (Ki67) is a proliferation marker.
Analysis of HTRA1 genovariation and DNA methylation in the CpG islands of HTRA1 DNA in BRCA samples
The genomic variation in HTRA1 gene was analyzed by cBioPortal database (https://www.cbioportal.org/) (30,31) including eight BRCA datasets: TCGA (19), METABRIC (32), INSERM (33), MBC project (https://mbcproject.org/), BCCRC (34), Broad (35), MSKCC (36), and Sanger (37). Kaplan-Meier survival analysis and log-rank test were performed to determine the prognostic significance of the genomic variation in HTRA1 gene. The DNA methylation in the CpG sites of HTRA1 gene and their prognostic values were analyzed by MethSurv database (https://biit.cs.ut.ee/methsurv/) (38). The association between CpG methylation of HTRA1 DNA and the overall survival (OS) of BRCA patients was also evaluated.
Correlation analysis between HTRA1 expression and the clinicopathological characteristics of BRCA patients
The clinicopathological information of BRCA patients was extracted from TCGA-BRCA (39). The differences of various clinicopathological parameters were compared between the high- and low-HTRA1 expression group by the Shapiro-Wilk normality test, Kruskal-Wallis test, and Dunn’s multiple hypothesis test. The results were corrected by the Bonferroni method and visualized using the “ggplot2” (v3.3.3) R package. Logistic regression analysis was used to evaluate the relationship between HTRA1 expression and the clinicopathological characteristics of BRCA patients.
Evaluation of the prognostic significance of HTRA1 expression in BRCA patients and their therapeutic response
The survival data of BRCA patients from TCGA-BRCA (39) and GEO (16,40) were analyzed by the “survival” (v3.2-10) and “survminer” (v.0.4.9) R package. Kaplan-Meier survival analysis as well as univariate and multivariate Cox regression were conducted to determine the survival of BRCA patients based on HTRA1 expression. The diagnostic and time-dependent survival ROC curve were generated using the “pROC” (v1.17.0.1) and “timeROC” (v0.4) R package. The online tool ROC Plotter (http://www.rocplot.org/site/index) was used to evaluate the impact of HTRA1 expression on the therapeutic response of breast patients from GEO (41).
Statistical analysis
The differences in HTRA1 expression between groups were analyzed using the Wilcoxon signed-rank test and one-way ANOVA. The correlations between HTRA1 expression and clinicopathological characteristics of patients were assessed using Fisher’s exact test, Chi-squared test, Wilcoxon signed-rank test, and logistic regression. Other statistical analyses were detailed above. P<0.05 was considered to be statistically significant.
Results
HTRA1 expression was significantly dysregulated in multiple types of cancer including BRCA
Figure 1A shows HTRA1 expression in 33 types of cancer in TCGA. The mRNA level of HTRA1 was found to be downregulated in seven types of cancer (BLCA: P=0.003; BRCA: P=0.003, shown also in Figure 1B; CESC: P=0.004; KICH: P<0.001; KIRP: P<0.001; PRAD: P<0.001, and UCEC: P<0.001), and upregulated in eight types of cancer (CHOL: P=0.003; ESCA: P=0.002; HNSC: P<0.001; KIRC: P<0.001; LIHC: P<0.001; PCPG: P=0.015; STAD: P<0.001, and THCA: P<0.001). HTRA1 mRNA expression in breast tumor tissues was also assessed using data from GSE109169 and GSE10780 datasets (Figure 1C,1D, respectively). The comparative results showed that HTRA1 mRNA expression in tumor tissues was significantly lower than that in normal breast tissues (Padj=0.0233, Padj=0.0111).
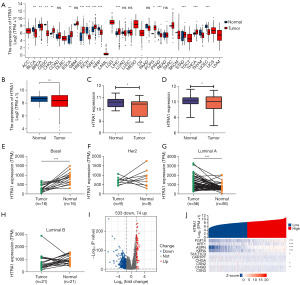
Figure 1E-1H shows HTRA1 expression in tumor tissues and their matched normal controls from TCGA-BRCA by subtype. HTRA1 expression was significantly lower in Basal-like BRCA than that in the paired normal tissues (n=16), while it was not significantly different for either HER2 positive (n=9) or luminal B BRCA (n=21), but was significantly higher for luminal A BRCA (n=56).
The median HTRA1 expression value was used to classify TCGA-BRCA samples into high- and low-HTRA1 expression group. Using the absolute log fold change >1.5 and P<0.05 as a threshold, we screened out 607 DEGs (74 upregulated and 533 downregulated) in the high-HTRA1 expression group compared to the low-HTRA1 expression group (Figure 1I). The ten most significant DEGs are shown in the single gene co-expression heat map in Figure 1J.
Furthermore, the immunohistochemical results from Human Protein Atlas (https://www.proteinatlas.org/) (42,43) verified the expression of HTRA1 protein. In 17 out of 23 samples, HTRA1 staining was absent in breast tumor cells, whereas its weak staining was observed in the cytoplasm or cytomembrane of tumor cells in the remaining six samples (Tables S1,S2).
HTRA1-associated DEGs in BRCA were mainly involved in cell proliferation and cell cycle process
The functional enrichment annotation for the HTRA1-associated DEGs in BRCA was conducted by the “clusterProfiler” R package. The results of Gene Ontology (GO) and Kyoto Encyclopedia of Genes and Genomes (KEGG) pathway enrichment, encompassing highly enriched biological process, cellular component, and molecular function (P<0.05), are displayed in Figure 2A and Table S3. The most enriched biological process were extracellular structure organization, extracellular matrix organization, and antimicrobial humoral response. Collagen-containing extracellular matrix, nucleosome, and collagen trimer emerged as the most enriched cellular component. Regarding molecular function, ion channel activity, extracellular matrix structural constituent, and collagen binding exhibited the highest levels of enrichment. The top-ranked KEGG pathways included neuroactive ligand-receptor interaction, alcoholism, and protein digestion and absorption. The PPI network of the DEGs revealed an association between HTRA1 and other genes (Figure 2B and Figure S1). GSEA analysis demonstrated that HTRA1-associated DEGs were significantly enriched in cell proliferation-related clusters (Figure 2C-2I), such as M phase (NES =−1.600, Padj=0.036, FDR =0.032), G2/M checkpoints (NES =−1.729, Padj=0.036, FDR =0.032), G0 and early G1 (NES =−1.733, Padj=0.037, FDR =0.033), cell cycle checkpoints (NES =−1.737, Padj=0.036, FDR =0.032), cell cycle (NES =−1.625, Padj=0.036, FDR =0.032; NES =−1.640, Padj=0.036, FDR =0.032), and DNA replication (NES =−1.731, Padj=0.036, FDR =0.032). HTRA1-associated DEGs were also enriched in cellular senescence (NES =−1.803, Padj=0.036, FDR =0.032), DNA double strand break repair (NES =−1.685, Padj=0.036, FDR =0.032), estrogen dependent gene expression (NES =−1.627, Padj=0.036, FDR =0.032), DNA double strand break response (NES =−1.804, Padj=0.036, FDR =0.032), and epigenetic regulation of gene expression (NES =−1.805, Padj=0.036, FDR =0.032) (Figure 2J-2N).
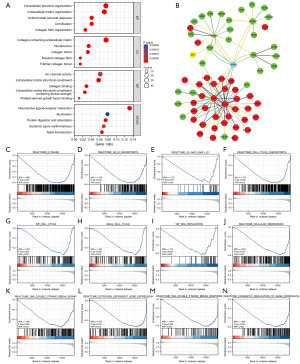
HTRA1 expression was correlated with the infiltration of multiple immune cells in BRCA tissues
HTRA1 showed a negative correlation with aDC (R=−0.114, P<0.001) and Th2 cells (R=−0.228, P<0.001), but a positive correlation with CD8 T cells (R=0.201, P<0.001), cytotoxic cells (R=0.091, P=0.003), DC (R=0.137, P<0.001), eosinophils (R=0.267, P<0.001), iDC (R=0.485, P<0.001), macrophages (R=0.316, P<0.001), mast cells (R=0.590, P<0.001), neutrophils (R=0.333, P<0.001), NK CD56bright cells (R=0.140, P<0.001), NK CD56dim cells (R=0.063, P=0.035), NK cells (R=0.537, P<0.001), pDC (R=0.195, P<0.001), T cells (R=0.091, P=0.002), Tcm (R=0.081, P=0.007), Tem (R=0.191, P<0.001), TFH (R=0.099, P<0.001), Tgd (R=0.169, P<0.001), and Th1 cells (R=0.164, P<0.001) (Figure 3A). The tumor infiltration levels of neutrophils, cytotoxic cells, DC, aDC, NK, and Th2 cells in the high- and low-HTRA1 expression group were consistent with the Spearman analysis results (Figure 3B-3G).
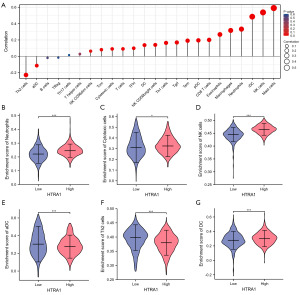
Regarding immune cell markers, HTRA1 expression was positively correlated with specific biomarkers for CD8+ T cells (CD8A, CD8B), TFH (BCL6), Th1 (T-bet/TBX21, STAT4), Th2 (CCR3, GATA3, STAT5A, STAT6), Th9 (IRF4, PU.1/SPI1, TGFBR2), Th17 (IL-21R, IL-23R, STAT3), Th22 (AHR, CCR10), Treg (CCR8, FOXP3), M1 macrophage (COX2/PTGS2, INOS/NOS2), M2 macrophage (CD206/MRC1, CD115/CSF1R), and TAM (PDCD1LG2, CD80, CD40); whereas it was negatively correlated with biomarkers for B cells (CD38), Th1 (IL2RB2), and Th17 (IL-17A) in BRCA (Table S4).
HTRA1 expression was correlated with immuno-related markers, TP53, and Ki67 in BRCA
In BRCA tissues, HTRA1 expression showed a positive correlation with the levels of CD274 (PD-L1), TIGIT, HAVCR2, LAYN, IL2 (IL-2), PRF1 (perforin), and TP53. Additionally, it exhibited a negative correlation with the levels of LAG3, GZMB (granzyme B), and MKI67 (Ki-67) (Figure 4).
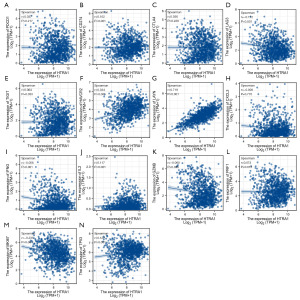
HTRA1 genovariation had no impact on the survival of BRCA patients
Only 1% genovariation was observed in HTRA1 based on data from eight BRCA datasets, including TCGA (n=1,084), METABRIC (n=2,509), INSERM (n=216), MBC project (n=237), BCCRC (n=65), Broad (n=103), MSKCC (n=70), and Sanger (n=100) (Figure 5A). Kaplan-Meier survival curve and log-rank test results showed that there was no significant difference in OS (P=0.726), progression free survival (P=0.456), relapse free status (P=0.543), disease free survival (P=0.430), and disease-specific survival (DSS) (P=0.400) between patients with or without HTRA1 genovariation (Figure 5B-5F). Further analysis revealed the incidence of HTRA1 mutation was 0.42% in MBC project, 0.46% in INSERM, and 0.37% in TCGA BRCA samples, respectively (Figure 5G). The highest level of HTRA1 amplification was observed in the MBC project dataset (2.53%), followed by METABRIC and TCGA (both 0.74%) and INSERM (0.46%). The mRNA expression level of HTRA1 showed a slight increase in tumors with amplified HTRA1 gene compared to those with diploid status (median, 11.7013 vs. 11.4394) (Figure 5H).
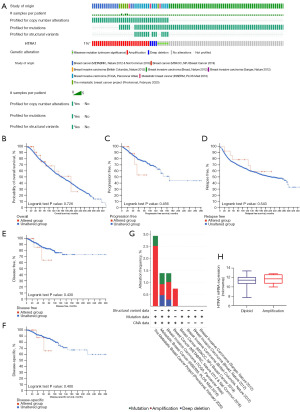
HTRA1 DNA methylation affected the prognosis of BRCA patients
In the HTRA1 DNA, there were 13 CpG islands showed aberrant DNA methylation, including cg05723130, cg15868400, cg06777002, cg07863439, cg15719652, cg01145353, cg09553346, cg17875153, cg05426956, cg22701672, cg00701951, cg03974204, and cg23791011 (Figure 6). Moreover, the methylation levels of seven CpG islands, namely cg00701951, cg01145353, cg01962937, cg04768425, cg06474225, cg15719652, and cg26466234, were associated with the prognosis of BRCA (Table 1). Increased methylation levels in these seven CpG islands, particularly cg01145353, cg15719652, and cg00701951, were associated with poorer OS in BRCA patients.
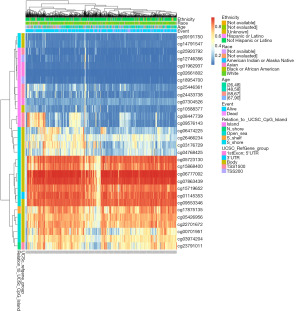
Table 1
CpG island | Hazard ratio | P value |
---|---|---|
Body-Open_Sea-cg00701951 | 0.557 | 0.022 |
3'UTR-Open_Sea-cg01145353 | 0.599 | 0.015 |
1stExon;5'UTR-Island-cg01962937 | 0.514 | 0.0013 |
1stExon;5'UTR-Island-cg02661802 | 0.718 | 0.14 |
Body-S_Shore-cg03176729 | 0.816 | 0.38 |
Body-Open_Sea-cg03974204 | 0.713 | 0.089 |
Body-S_Shore-cg04768425 | 0.526 | 0.0021 |
Body-Open_Sea-cg05426956 | 1.519 | 0.11 |
Body-Open_Sea-cg05723130 | 1.151 | 0.57 |
Body-Open_Sea-cg06474225 | 0.543 | 0.0059 |
Body-Open_Sea-cg06777002 | 1.397 | 0.2 |
1stExon;5'UTR-Island-cg07304526 | 0.593 | 0.055 |
Body-Open_Sea-cg07863439 | 1.391 | 0.2 |
TSS1500-Island-cg08447739 | 0.828 | 0.46 |
Body-S_Shore-cg09191750 | 0.817 | 0.37 |
Body-Open_Sea-cg09553346 | 0.775 | 0.27 |
TSS1500-Island-cg09576143 | 0.646 | 0.11 |
Body-Island-cg10588377 | 1.359 | 0.21 |
TSS200-Island-cg12746356 | 0.668 | 0.11 |
Body-S_Shore-cg14791547 | 1.449 | 0.083 |
Body-Open_Sea-cg15719652 | 0.561 | 0.0039 |
Body-Open_Sea-cg15868400 | 1.408 | 0.099 |
Body-S_Shelf-cg17875135 | 1.252 | 0.26 |
TSS200-Island-cg18954700 | 0.753 | 0.16 |
Body-Open_Sea-cg22701672 | 0.756 | 0.16 |
TSS1500-N_Shore-cg23791011 | 0.909 | 0.69 |
TSS1500-Island-cg24433738 | 0.899 | 0.59 |
TSS1500-Island-cg25446361 | 1.13 | 0.54 |
TSS1500-Island-cg25920792 | 0.804 | 0.28 |
Body-Open_Sea-cg26466234 | 0.476 | 0.0065 |
HTRA1, high temperature requirement A1; BRCA, breast cancer.
HTRA1 expressions correlated with multiple clinicopathological characteristics in BRCA
Table 2 displays the association between HTRA1 expression and clinicopathological characteristics in BRCA patients. Significant differences were observed between the high- and low-HTRA1 expression group in terms of clinical T stage, histological type, PR status, ER status, PAM50 subtype, and age. Additionally, HTRA1 expression also varied significantly with age, pathologic stage, histological type, T stage, N stage, ER status, PR status, and PAM50 subtype in BRCA (Figure 7). Logistic regression analysis further confirmed a positive correlation of HTRA1 expression with N stage, age, ER status, PR status, histological type, and PAM50 subtype in BRCA (Table 3).
Table 2
Characteristic | Low expression of HTRA1 (n=541) | High expression of HTRA1 (n=542) | P value |
---|---|---|---|
T stage, n (%) | 0.014 | ||
T1 | 115 (10.6) | 162 (15) | |
T2 | 331 (30.6) | 298 (27.6) | |
T3 | 72 (6.7) | 67 (6.2) | |
T4 | 20 (1.9) | 15 (1.4) | |
N stage, n (%) | 0.054 | ||
N0 | 278 (26.1) | 236 (22.2) | |
N1 | 168 (15.8) | 190 (17.9) | |
N2 | 49 (4.6) | 67 (6.3) | |
N3 | 37 (3.5) | 39 (3.7) | |
M stage, n (%) | 1.000 | ||
M0 | 457 (49.6) | 445 (48.3) | |
M1 | 10 (1.1) | 10 (1.1) | |
Pathologic stage, n (%) | 0.587 | ||
Stage I | 85 (8) | 96 (9.1) | |
Stage II | 321 (30.3) | 298 (28.1) | |
Stage III | 116 (10.9) | 126 (11.9) | |
Stage IV | 9 (0.8) | 9 (0.8) | |
Age, n (%) | 0.016 | ||
≤60 years | 280 (25.9) | 321 (29.6) | |
>60 years | 261 (24.1) | 221 (20.4) | |
Histological type, n (%) | <0.001 | ||
Infiltrating ductal carcinoma | 412 (42.2) | 360 (36.8) | |
Infiltrating lobular carcinoma | 64 (6.6) | 141 (14.4) | |
PR status, n (%) | <0.001 | ||
Negative | 232 (22.4) | 110 (10.6) | |
Indeterminate | 3 (0.3) | 1 (0.1) | |
Positive | 278 (26.9) | 410 (39.7) | |
ER status, n (%) | <0.001 | ||
Negative | 179 (17.3) | 61 (5.9) | |
Indeterminate | 2 (0.2) | 0 (0) | |
Positive | 333 (32.2) | 460 (44.4) | |
HER2 status, n (%) | 0.165 | ||
Negative | 275 (37.8) | 283 (38.9) | |
Indeterminate | 7 (1) | 5 (0.7) | |
Positive | 65 (8.9) | 92 (12.7) | |
PAM50, n (%) | <0.001 | ||
Normal | 12 (1.1) | 28 (2.6) | |
LumA | 193 (17.8) | 369 (34.1) | |
LumB | 128 (11.8) | 76 (7) | |
Her2 | 45 (4.2) | 37 (3.4) | |
Basal | 163 (15.1) | 32 (3) |
HTRA1, high temperature requirement A1; BRCA, breast cancer; PR, progesterone receptor; ER, estrogen receptor; HER2, human epidermal growth factor receptor 2; PAM50, prediction analysis of microarray 50; LumA, luminal A; LumB; luminal B.
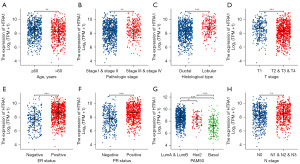
Table 3
Characteristics | Total (N) | Odds ratio (OR) | P value |
---|---|---|---|
T stage (T3 & T4 vs. T1 & T2) | 1,080 | 0.864 (0.624–1.196) | 0.379 |
N stage (N1 & N2 & N3 vs. N0) | 1,064 | 1.373 (1.079–1.748) | 0.010 |
M stage (M1 vs. M0) | 922 | 1.027 (0.418–2.526) | 0.953 |
Pathologic stage (stage III & IV vs. stage I & II) | 1,060 | 1.113 (0.841–1.473) | 0.454 |
Age (>60 vs. ≤60 years) | 1,083 | 0.739 (0.581–0.939) | 0.013 |
Histological type (infiltrating lobular carcinoma vs. infiltrating ductal carcinoma) | 977 | 2.521 (1.825–3.516) | <0.001 |
PR status (positive vs. negative) | 1,030 | 3.111 (2.372–4.098) | <0.001 |
ER status (positive vs. negative) | 1,033 | 4.054 (2.950–5.634) | <0.001 |
HER2 status (positive vs. negative) | 715 | 1.375 (0.963–1.974) | 0.081 |
Radiation therapy (yes vs. no) | 987 | 1.062 (0.826–1.366) | 0.639 |
Menopause status (peri & post vs. pre) | 972 | 0.862 (0.640–1.159) | 0.326 |
PAM50 (Basal vs. LumA & LumB & HER2) | 1,043 | 0.149 (0.098–0.220) | <0.001 |
HTRA1, high temperature requirement A1; BRCA, breast cancer; PR, progesterone receptor; ER, estrogen receptor; HER2, human epidermal growth factor receptor 2; PAM50, prediction analysis of microarray 50; LumA, luminal A; LumB; luminal B.
High HTRA1 expression was associated with favorable prognosis of BRCA patients from GEO datasets, but not TCGA
Kaplan-Meier survival curves demonstrated that the relative HTRA1 expression level (high or low) in TCGA-BRCA patients was not correlated with OS (P=0.776), DSS (P=0.551), or progression-free interval (PFI, P=0.446). However, when examining the GEO database (2017, n=5,143, HTRA1 Affymetrix ID is 201185_at) (40,44), high HTRA1 expression was associated with favorable OS and relapse-free survival (RFS) in BRCA patients (Figure 8), indicating a significantly reduced risk for recurrence and death.
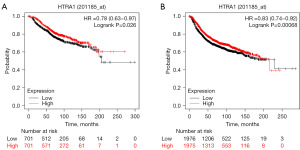
Furthermore, our ROC curves indicated that HTRA1 expression may not have diagnostic value in distinguishing tumor from adjacent normal tissue [area under the curve (AUC) =0.572]. Additionally, we used the online tool ROC Plotter to assess the impact of HTRA1 expression on therapeutic response in BRCA patients from GEO (41). The selected datasets were all from clinical trials including GSE16446 (NCT00017095, NCT00336791), GSE41998 (NCT00455533), GSE50948 (ISRCTN86043495), GSE66305 (NCT00429299), and GSE16391 (NCT00004205). The results showed that for the luminal B subtype, patients with lower HTRA1 expression had a higher likelihood of achieving pathological complete response to chemotherapy (Figure 9 and Table S5).
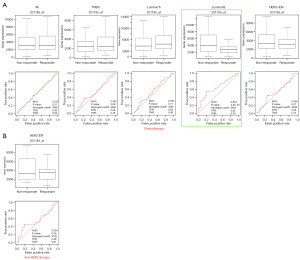
Discussion
By evaluating HTRA1 expression in human cancers within TCGA database, we observed dysregulation of HTRA1 in 15 out of 33 types of cancer. Specifically, in BRCA, both TCGA and GEO datasets showed a significant reduction in HTRA1 expression in tumor tissues. However, low HTRA1 expression was associated with an unfavorable prognosis in patients from GEO, but not TCGA. These results are consistent with previous studies. Wang et al. reported a notable decrease or absence of HTRA1 expression in in situ or invasive ductal BRCA tissues compared to its prominent expression in normal breast ductal epithelium tissues (45). Lehner et al. measured HTRA1 mRNA expression in 131 BRCA patients (15) and found significantly higher levels of HTRA1 in lower tumor stage, which correlated with longer OS and disease free survival. Franco et al. evaluated 66 sentinel node positive BRCA cases and discovered a negative correlation between HTRA1 expression and metastasis development as well as local relapse (46). However, a retrospective analysis suggested that six epithelial mesenchymal transition genes including LUM, SFRP4, COL6A3, MMP2, CXCL12, and HTRA1 were continuously expressed at higher levels in samples from patients with metastatic BRCA (47). We also observed a significant correlation between HTRA1 expression in BRCA tissues and various factors such as age, clinical N stage, histological type, PR status, ER status, and PAM50 subtype. However, it is important to note that HTRA1 expression exhibits high heterogeneity among different BRCA subtypes. This finding requires further investigation due to limitations in sample size and variations in sampling sites and procedures.
Subsequently, we screened for 607 HTRA1-related DEGs from TCGA-BRCA samples based on median HTRA1 expression. These DEGs primarily participate in extracellular matrix organization processes and exhibit molecular functions related to these processes. GSEA results demonstrated that the HTRA1-related DEGs were predominantly involved in cell proliferation, cell cycle regulation, cellular senescence, and the estrogen-dependent gene expression clusters. Wang et al. also reported decreased HTRA1 expression in BRCA cell lines (45). HTRA1 siRNA-transfected non-tumorigenic MCF10A normal breast epithelium cells exhibited smaller size, increased growth rate, as well as enhanced migration and invasion capabilities. Protease nexin-1 (PN-1) promotes cancer cell metastasis by remodeling the tumor matrix. Tang et al. demonstrated that upregulated PN-1 in BRCA promotes cell invasion, migration, and stemness through the EGF/EGFR/PKCδ/MEK/ERK/EGR1 axis, with HTRA1 being a target of PN-1. Consequently, HTRA1 overexpression inhibits migration and invasion of BRCA cells, while PN-1 overexpression nullifies this inhibitory effect (48). Collectively, these findings confirm the inhibitory impact of HTRA1 on cell proliferation in breast cells. Nevertheless, there are discrepancies among studies, and the current evidence does not definitively establish HTRA1 as a tumor suppressor (9).
Genetic variation has been implicated in the diagnosis, treatment, and prognosis of BRCA patients (49). However, in our analyses, HTRA1 genovariation was not found to be correlated with survival of BRCA patients. We hypothesize that this may be due to the minimal impact of genovariation on HTRA1 expression. DNA methylation is an epigenetic mechanism which plays a crucial role in tumorigenesis. Alterations in HTRA1 DNA methylation can affect its expression in BRCA (15). Notably, there is an inverse correlation between mRNA expression and DNA methylation at the transcription start site of the HTRA1 promoter in BRCA cells (45). In our assessment of the relationship between HTRA1 DNA methylation and prognosis of BRCA patients, we observed that increased methylation at three CpG islands (cg01145353, cg15719652, and cg00701951) was associated with poorer OS compared to decreased methylation.
The immune contexture within the breast tumor microenvironment can influence tumor growth and metastasis. A previous study evaluated tumor-infiltrating lymphocytes (TILs) and their relevance to therapeutic response and prognosis in 3,771 patients with primary BRCA undergoing neoadjuvant chemotherapy (50). The results demonstrated that increased TIL density predicted chemotherapy response across all BRCA subtypes. Furthermore, TIL density was associated with a survival benefit in HER2-positive and TNBC patients (50). Our study revealed potential correlations between HTRA1 expression and the proportion of tumor-infiltrating cells, as well as immune cell markers such as CD8A, CCR3, GATA3, and STAT6. Additionally, significant associations were found between HTRA1 expression and the immune checkpoint PD-L1, T cell exhaustion marker, T cell effector function marker, TP53, and Ki67. These findings support the potential suppressive role of HTRA1 in BRCA. Recent studies have investigated the use of anti-PD-1/PD-L1 agents in BRCA, similar to melanoma or non-small cell lung cancer, particularly in TNBC. Encouraging outcomes have been observed with monotherapy or combination treatment (51). The signature of exhausted T cells can also predict immunotherapy response in BRCA (52). Therefore, our results suggest that HTRA1 may serve as a potential target to enhance the efficacy of immunotherapy in BRCA patients.
Folgueira et al. identified predictive markers for neoadjuvant chemotherapy response in BRCA patients (53). They highlighted a trio of genes, including HTRA1, CLPTM1, and MTSS1 genes, which could accurately differentiate responsive from non-responsive tumors. Another study involving 333 non-metastatic locally advanced BRCA patients who received neoadjuvant chemotherapy demonstrated that higher HTRA1 expression was associated with increased recurrence risk and cancer-related death (54). Our findings indicate that HTRA1 expression can only discriminate between responsive and non-responsive Luminal B patients undergoing chemotherapy. However, the utility of HTRA1 as a biomarker for BRCA prognosis and treatment response requires further comprehensive understanding.
There were some limitations in our study. Firstly, we solely analyzed HTRA1 expression in BRCA samples from TCGA and GEO public database, and it would be beneficial to validate our findings using clinical samples. Secondly, we did not directly assess the molecular mechanism and activity of HTRA1 through in vitro and in vivo experiments, therefore our hypothesis about the effects of genetic variation, expression levels, and progression/prognosis/response to therapy are speculative. Thirdly, the relationships between HTRA1 expression and the function of tumor-infiltrating immune cells within the breast tumor microenvironment needs to be elucidated through well-designed studies. Further investigations are necessary to unravel the complex regulatory role of HTRA1 in BRCA.
Conclusions
In conclusion, our analysis revealed a significant decrease in HTRA1 expression in BRCA datasets. Our findings suggest that HTRA1 may exert its influence on BRCA development through the modulation of genes involved in tumor cell proliferation and immunity. Additionally, we observed that HTRA1 expression might play a crucial role in chemotherapy response and DNA methylation. Thus, HTRA1 expression is associated with BRCA prognosis. Collectively, these findings propose HTRA1 as a potential therapeutic target and a useful prognostic biomarker in BRCA.
Acknowledgments
Funding: This work was supported by
Footnote
Reporting Checklist: The authors have completed the STREGA reporting checklist. Available at https://tcr.amegroups.com/article/view/10.21037/tcr-23-773/rc
Peer Review File: Available at https://tcr.amegroups.com/article/view/10.21037/tcr-23-773/prf
Conflicts of Interest: All authors have completed the ICMJE uniform disclosure form (available at https://tcr.amegroups.com/article/view/10.21037/tcr-23-773/coif). The authors have no conflicts of interest to declare.
Ethical Statement: The authors are accountable for all aspects of the work in ensuring that questions related to the accuracy or integrity of any part of the work are appropriately investigated and resolved. The study was conducted in accordance with the Declaration of Helsinki (as revised in 2013).
Open Access Statement: This is an Open Access article distributed in accordance with the Creative Commons Attribution-NonCommercial-NoDerivs 4.0 International License (CC BY-NC-ND 4.0), which permits the non-commercial replication and distribution of the article with the strict proviso that no changes or edits are made and the original work is properly cited (including links to both the formal publication through the relevant DOI and the license). See: https://creativecommons.org/licenses/by-nc-nd/4.0/.
References
- Sung H, Ferlay J, Siegel RL, et al. Global Cancer Statistics 2020: GLOBOCAN Estimates of Incidence and Mortality Worldwide for 36 Cancers in 185 Countries. CA Cancer J Clin 2021;71:209-49. [Crossref] [PubMed]
- Sørlie T, Perou CM, Tibshirani R, et al. Gene expression patterns of breast carcinomas distinguish tumor subclasses with clinical implications. Proc Natl Acad Sci U S A 2001;98:10869-74. [Crossref] [PubMed]
- Ye F, Dewanjee S, Li Y, et al. Advancements in clinical aspects of targeted therapy and immunotherapy in breast cancer. Mol Cancer 2023;22:105. [Crossref] [PubMed]
- Huppert LA, Gumusay O, Idossa D, et al. Systemic therapy for hormone receptor-positive/human epidermal growth factor receptor 2-negative early stage and metastatic breast cancer. CA Cancer J Clin 2023;73:480-515. [Crossref] [PubMed]
- Szymiczek A, Lone A, Akbari MR. Molecular intrinsic versus clinical subtyping in breast cancer: A comprehensive review. Clin Genet 2021;99:613-37. [Crossref] [PubMed]
- Qayoom H, Sofi S, Mir MA. Targeting tumor microenvironment using tumor-infiltrating lymphocytes as therapeutics against tumorigenesis. Immunol Res 2023;71:588-99. [Crossref] [PubMed]
- Wang Y, Nie G. Overview of Human HtrA Family Proteases and Their Distinctive Physiological Roles and Unique Involvement in Diseases, Especially Cancer and Pregnancy Complications. Int J Mol Sci 2021;22:10756. [Crossref] [PubMed]
- Zumbrunn J, Trueb B. Primary structure of a putative serine protease specific for IGF-binding proteins. FEBS Lett 1996;398:187-92. [Crossref] [PubMed]
- Altobelli E, Marzioni D, Lattanzi A, et al. HtrA1: Its future potential as a novel biomarker for cancer. Oncol Rep 2015;34:555-66. [Crossref] [PubMed]
- Chien J, Staub J, Hu SI, et al. A candidate tumor suppressor HtrA1 is downregulated in ovarian cancer. Oncogene 2004;23:1636-44. [Crossref] [PubMed]
- Baldi A, De Luca A, Morini M, et al. The HtrA1 serine protease is down-regulated during human melanoma progression and represses growth of metastatic melanoma cells. Oncogene 2002;21:6684-8. [Crossref] [PubMed]
- Welsh JB, Sapinoso LM, Su AI, et al. Analysis of gene expression identifies candidate markers and pharmacological targets in prostate cancer. Cancer Res 2001;61:5974-8. [PubMed]
- Esposito V, Campioni M, De Luca A, et al. Analysis of HtrA1 serine protease expression in human lung cancer. Anticancer Res 2006;26:3455-9. [PubMed]
- Mullany SA, Moslemi-Kebria M, Rattan R, et al. Expression and functional significance of HtrA1 loss in endometrial cancer. Clin Cancer Res 2011;17:427-36. [Crossref] [PubMed]
- Lehner A, Magdolen V, Schuster T, et al. Downregulation of serine protease HTRA1 is associated with poor survival in breast cancer. PLoS One 2013;8:e60359. [Crossref] [PubMed]
- Barrett T, Wilhite SE, Ledoux P, et al. NCBI GEO: archive for functional genomics data sets--update. Nucleic Acids Res 2013;41:D991-5. [Crossref] [PubMed]
- Chang JW, Kuo WH, Lin CM, et al. Wild-type p53 upregulates an early onset breast cancer-associated gene GAS7 to suppress metastasis via GAS7-CYFIP1-mediated signaling pathway. Oncogene 2018;37:4137-50. [Crossref] [PubMed]
- Chen DT, Nasir A, Culhane A, et al. Proliferative genes dominate malignancy-risk gene signature in histologically-normal breast tissue. Breast Cancer Res Treat 2010;119:335-46. [Crossref] [PubMed]
- Hoadley KA, Yau C, Hinoue T, et al. Cell-of-Origin Patterns Dominate the Molecular Classification of 10,000 Tumors from 33 Types of Cancer. Cell 2018;173:291-304.e6. [Crossref] [PubMed]
- Love MI, Huber W, Anders S. Moderated estimation of fold change and dispersion for RNA-seq data with DESeq2. Genome Biol 2014;15:550. [Crossref] [PubMed]
- Yu G, Wang LG, Han Y, et al. clusterProfiler: an R package for comparing biological themes among gene clusters. OMICS 2012;16:284-7. [Crossref] [PubMed]
- Subramanian A, Tamayo P, Mootha VK, et al. Gene set enrichment analysis: a knowledge-based approach for interpreting genome-wide expression profiles. Proc Natl Acad Sci U S A 2005;102:15545-50. [Crossref] [PubMed]
- Liberzon A, Birger C, Thorvaldsdóttir H, et al. The Molecular Signatures Database (MSigDB) hallmark gene set collection. Cell Syst 2015;1:417-25. [Crossref] [PubMed]
- Szklarczyk D, Gable AL, Nastou KC, et al. The STRING database in 2021: customizable protein-protein networks, and functional characterization of user-uploaded gene/measurement sets. Nucleic Acids Res 2021;49:D605-12. [Crossref] [PubMed]
- Shannon P, Markiel A, Ozier O, et al. Cytoscape: a software environment for integrated models of biomolecular interaction networks. Genome Res 2003;13:2498-504. [Crossref] [PubMed]
- Hänzelmann S, Castelo R, Guinney J. GSVA: gene set variation analysis for microarray and RNA-seq data. BMC Bioinformatics 2013;14:7. [Crossref] [PubMed]
- Bindea G, Mlecnik B, Tosolini M, et al. Spatiotemporal dynamics of intratumoral immune cells reveal the immune landscape in human cancer. Immunity 2013;39:782-95. [Crossref] [PubMed]
- Ibrahim R, Saleh K, Chahine C, et al. LAG-3 Inhibitors: Novel Immune Checkpoint Inhibitors Changing the Landscape of Immunotherapy. Biomedicines 2023;11:1878. [Crossref] [PubMed]
- Wang H, Guo M, Wei H, et al. Targeting p53 pathways: mechanisms, structures, and advances in therapy. Signal Transduct Target Ther 2023;8:92. [Crossref] [PubMed]
- Cerami E, Gao J, Dogrusoz U, et al. The cBio cancer genomics portal: an open platform for exploring multidimensional cancer genomics data. Cancer Discov 2012;2:401-4. [Crossref] [PubMed]
- Gao J, Aksoy BA, Dogrusoz U, et al. Integrative analysis of complex cancer genomics and clinical profiles using the cBioPortal. Sci Signal 2013;6:pl1. [Crossref] [PubMed]
- Pereira B, Chin SF, Rueda OM, et al. The somatic mutation profiles of 2,433 breast cancers refines their genomic and transcriptomic landscapes. Nat Commun 2016;7:11479. [Crossref] [PubMed]
- Lefebvre C, Bachelot T, Filleron T, et al. Mutational Profile of Metastatic Breast Cancers: A Retrospective Analysis. PLoS Med 2016;13:e1002201. [Crossref] [PubMed]
- Shah SP, Roth A, Goya R, et al. The clonal and mutational evolution spectrum of primary triple-negative breast cancers. Nature 2012;486:395-9. [Crossref] [PubMed]
- Banerji S, Cibulskis K, Rangel-Escareno C, et al. Sequence analysis of mutations and translocations across breast cancer subtypes. Nature 2012;486:405-9. [Crossref] [PubMed]
- Nixon MJ, Formisano L, Mayer IA, et al. PIK3CA and MAP3K1 alterations imply luminal A status and are associated with clinical benefit from pan-PI3K inhibitor buparlisib and letrozole in ER+ metastatic breast cancer. NPJ Breast Cancer 2019;5:31. [Crossref] [PubMed]
- Stephens PJ, Tarpey PS, Davies H, et al. The landscape of cancer genes and mutational processes in breast cancer. Nature 2012;486:400-4. [Crossref] [PubMed]
- Modhukur V, Iljasenko T, Metsalu T, et al. MethSurv: a web tool to perform multivariable survival analysis using DNA methylation data. Epigenomics 2018;10:277-88. [Crossref] [PubMed]
- Liu J, Lichtenberg T, Hoadley KA, et al. An Integrated TCGA Pan-Cancer Clinical Data Resource to Drive High-Quality Survival Outcome Analytics. Cell 2018;173:400-416.e11. [Crossref] [PubMed]
- Lánczky A, Győrffy B. Web-Based Survival Analysis Tool Tailored for Medical Research (KMplot): Development and Implementation. J Med Internet Res 2021;23:e27633. [Crossref] [PubMed]
- Fekete JT, Győrffy B. ROCplot.org: Validating predictive biomarkers of chemotherapy/hormonal therapy/anti-HER2 therapy using transcriptomic data of 3,104 breast cancer patients. Int J Cancer 2019;145:3140-51. [Crossref] [PubMed]
- Uhlén M, Björling E, Agaton C, et al. A human protein atlas for normal and cancer tissues based on antibody proteomics. Mol Cell Proteomics 2005;4:1920-32. [Crossref] [PubMed]
- Uhlen M, Zhang C, Lee S, et al. A pathology atlas of the human cancer transcriptome. Science 2017;357:eaan2507. [Crossref] [PubMed]
- Győrffy B. Survival analysis across the entire transcriptome identifies biomarkers with the highest prognostic power in breast cancer. Comput Struct Biotechnol J 2021;19:4101-9. [Crossref] [PubMed]
- Wang N, Eckert KA, Zomorrodi AR, et al. Down-regulation of HtrA1 activates the epithelial-mesenchymal transition and ATM DNA damage response pathways. PLoS One 2012;7:e39446. [Crossref] [PubMed]
- Franco R, Collina F, Di Bonito M, et al. HtrA1 loss is related to aggressive behavior parameters in sentinel node positive breast cancer. Histol Histopathol 2015;30:707-14. [PubMed]
- Wei LY, Zhang XJ, Wang L, et al. A Six-Epithelial-Mesenchymal Transition Gene Signature May Predict Metastasis of Triple-Negative Breast Cancer. Onco Targets Ther 2020;13:6497-509. [Crossref] [PubMed]
- Tang T, Zhu Q, Li X, et al. Protease Nexin I is a feedback regulator of EGF/PKC/MAPK/EGR1 signaling in breast cancer cells metastasis and stemness. Cell Death Dis 2019;10:649. [Crossref] [PubMed]
- Harbeck N, Penault-Llorca F, Cortes J, et al. Breast cancer. Nat Rev Dis Primers 2019;5:66. [Crossref] [PubMed]
- Denkert C, von Minckwitz G, Darb-Esfahani S, et al. Tumour-infiltrating lymphocytes and prognosis in different subtypes of breast cancer: a pooled analysis of 3771 patients treated with neoadjuvant therapy. Lancet Oncol 2018;19:40-50. [Crossref] [PubMed]
- Emens LA, Cruz C, Eder JP, et al. Long-term Clinical Outcomes and Biomarker Analyses of Atezolizumab Therapy for Patients With Metastatic Triple-Negative Breast Cancer: A Phase 1 Study. JAMA Oncol 2019;5:74-82. [Crossref] [PubMed]
- Terranova-Barberio M, Pawlowska N, Dhawan M, et al. Exhausted T cell signature predicts immunotherapy response in ER-positive breast cancer. Nat Commun 2020;11:3584. [Crossref] [PubMed]
- Folgueira MA, Carraro DM, Brentani H, et al. Gene expression profile associated with response to doxorubicin-based therapy in breast cancer. Clin Cancer Res 2005;11:7434-43. [Crossref] [PubMed]
- Carrara GFA, Evangelista AF, Scapulatempo-Neto C, et al. Analysis of RPL37A, MTSS1, and HTRA1 expression as potential markers for pathologic complete response and survival. Breast Cancer 2021;28:307-20. [Crossref] [PubMed]