Use of survival support vector machine combined with random survival forest to predict the survival of nasopharyngeal carcinoma patients
Highlight box
Key findings
• Compared with Cox regression, the machine learning method can significantly improve the accuracy of survival prognosis prediction in nasopharyngeal carcinoma (NPC) patients.
What is known and what is new?
• The Cox regression model is not sufficiently accurate to predict the survival prognosis of NPC patients.
• Utilizing random survival forest and semi-supervised support vector machine methodologies for the development of a prognostic survival prediction system tailored to individuals afflicted with NPC.
What is the implication, and what should change now?
• Enhancing medical adherence and augmenting survival outcomes among individuals diagnosed with NPC through the application of machine learning techniques.
Introduction
Nasopharyngeal carcinoma (NPC) is a special kind of squamous-cell carcinoma originating from the nasopharynx (1). Considering the concealment of its location, early cases of NPC are easily misdiagnosed or missed. However, NPC has a high degree of malignancy. Lymph node metastasis can occur in the early stage (2), and its prognosis is not optimistic. Although the current comprehensive treatment based on radiotherapy is considered as the mainstream treatment method of NPC, for patients with non-endemic locally advanced NPC, induction TPF (docetaxel, cisplatin, and fluorouracil) chemotherapy plus concurrent chemoradiotherapy (IC + CCRT) with CCRT is more effective than CCRT alone in locally advanced NPC (3).
The predictive model of NPC is still based on the Cox model. Regarding the survival prognosis of NPC, literature research has found that most of the studies are exploring the impact of single or several factors on the survival prognosis of NPC patients. Sun et al. (3) studied the effect of local tumor resection and pharyngectomy on the survival of patients with NPC. Patel et al.’s findings (4) suggested that racial and ethnic disparities had an impact on nasopharyngeal cancer survival. Wang et al. (5) reported in their study that insurance and marital status affected NPC survival. Non-insured and unmarried NPC patients had a significantly increased risk of remote metastasis at diagnosis and lower disease-specific 5-year survival. Moreover, they were less likely to receive radiation therapy than married and insured patients. Although Pan’s nomogram (6) based on the tumor, node, and metastasis (TNM) staging system of the American Joint Committee on Cancer (AJCC)/Union for International Cancer Control (UICC) and nomogram was specifically developed for locoregional NPC by OuYang et al. (7), we would like to try to develop a more accurate prognosticative system for NPC patients based on machine learning methods considering the development of machine learning technology.
Random survival forests (RSFs) are currently used for the abovementioned high-throughput data and are applied to the prediction of other diseases. For example, Wongvibulsin et al. (8) used RSF for clinical risk prediction in myocardial infarction. Zhang et al. (9) used RSF to predict an individual’s risk of death in acute-on-chronic liver failure. Zhang et al. (10) predicted the prognosis of elderly patients with sepsis, and Mohammed et al. (11) predicted this based on gene expression data on colorectal cancer. However, no relevant studies on the survival prognosis of NPC patients using RSF have been found. Survival-support vector machine (SVM) was applied to the survival analysis of prostate cancer patients in the SEER database by Liu et al. (12) as early as 2010. However, few methods utilized SVM for survival analysis in the literature because of its computational complexity. We planned to perform a survival analysis of NPC patients by using the survival-SVM model. We present this article in accordance with the TRIPOD reporting checklist (available at https://tcr.amegroups.com/article/view/10.21037/tcr-23-316/rc).
Methods
Data for this study were obtained from SEER (The Surveillance, Epidemiology, and End Results) database from January 2010 to December 2015. Patients were included according to the International Classification of Oncologic Diseases, Third Edition (ICD-0-3) HNC diagnosis for oral, oropharyngeal, hypopharyngeal, and laryngeal codes (13). We included squamous-cell carcinoma defined by the following histological codes: 8052, 8070 to 8074, 8082, 8083, and 8090. A total of 1,638 patients met the criteria. Sex, race, primary site-labeled, grade, AJCC Stage Group, AJCC T, AJCC N, AJCC M, reason no cancer-directed surgery, radiation recode, chemotherapy recode, Mets at DX-bone, Mets at DX-brain, Mets at DX-liver, Mets at DX-lung, Surg/Rad Seq, and Marital status at diagnosis were used in the survival analysis. Patients with postoperative death time of less than 3 months, patients with missing statistical information, and some extreme individual data (mainly referring to the data accounting for less than 1%) were not included in the analysis due to consideration of study results’ accuracy. The descriptive part of the statistical analysis described the censoring of vital status or follow-up data but did not include survival analysis (N=1,638). This study was conducted in accordance with the Declaration of Helsinki (as revised in 2013). Guangxi Medical University Institutional Review Board deemed this study exempt from review.
The Cox regression model, also known as the proportional hazards model, is a semi-parametric regression model proposed by British statistician Cox [1972] (14). Although regression is widely used for clinical research due to its advantages, such as no requirements for survival time distribution and wide scope of application, it is proposed as a semi-parametric model for the condition that the mortality risk of different individuals remains at a constant proportion at all times (15). Most individuals cannot meet this condition in real-world situations, and the Cox regression model may not achieve the best-fitting effect for each data (16).
An integrated tree method for analyzing right-censored data in survival data was proposed by Ishwaran et al. (17) in 2008. Then, the survival forest extended Breiman’s method, and the RSF selected the final prediction result by training a large number of survival trees and weighting them from individual trees in the form of voting. The RSF model’s advantage is that it is not constrained by the proportional hazard’s assumption, log-linear assumption, and other conditions. At the same time, the RSF has the advantage of the random forests; the overfitting problem of its algorithm can be prevented through two random sampling processes (18-20). In addition, the RSF can also be used for survival analysis and changeable screening of high-dimensional data; it can also be applied to the analysis of competing risk (21). RSF can rank the importance of variables, and the VIMP (variable important) and the minimum depth methods are the most commonly used methods (22): varying VIMP values less than 0 indicated that this variable reduced the accuracy of prediction, whereas VIMP values greater than 0 indicated that this variable improved the accuracy of prediction. The minimum depth method gives the importance of each variable for outcome events by calculating the minimum depth when running to the final node.
Survival-SVM was proposed by Van Belle in 2011 (23). Survival-SVM is a new applicable small sample learning method with a solid theoretical foundation. It does not involve probability measurement and the law of large numbers, and it also simplifies the usual classification and regression problems. Survival-SVM is insensitive to outliers (12), which helps elucidate key samples and “eliminate” a large number of redundant samples. It is a simple algorithm that has pleasant “robustness”. Survival-SVM has a decent generalization ability.
We used the concordance index (C-index) as an evaluation index of exemplary accuracy. We also used the C-index and log-rank P value as the metrics to evaluate model accuracy. For Cox regression models, we added area under the receiver operating characteristic (ROC) curve (AUC) values and area under the ROC curve to achieve an ideal evaluation. For RSF models, we ranked mutable importance using the VIMP and the minimum depth methods. The VIMP values less than 0 indicated that the variable reduced the accuracy of prediction, whereas values greater than 0 indicated that the variable improved the accuracy of prediction. The minimum depth method gave the importance of each variable for outcome events by calculating the minimum depth when running to the final node.
Statistical analysis
In this study, we conducted univariate and multivariate Cox regression analyses on a dataset of 1,638 patients and constructed a nomogram for survival prognosis. Following the results of the Cox model’s univariate analysis, we performed univariate analysis using a non-proportional hazards regression model. Additionally, we conducted survival analysis on relevant factors using the non-parametric Kaplan-Meier method. Subsequently, we heavily relied on the R packages “survival” and “survminer” to build and validate the Cox regression models. When constructing the Cox model in R, the key function employed was coxph(), preceded by the generation of a survival object using the Surv() function. Finally, we utilized the Cox regression model to predict the 3-year survival rate of NPC patients. Given the distinctive attributes of our sample size, this study opted to use the RSF method and the survival-SVM method to construct survival prognosis models for NPC patients. Throughout these analytical undertakings, we made extensive use of various R packages housed within R version 4.0.5, including but not limited to randomForestSRC, ggRandomForests, survival, survminer, survivalsvm, and ggplot2. A two-tailed P value smaller than 0.05 was recognized as statistically significant.
Results
Patient profile
A total of 1,638 eligible patients were included. Among them, the Cox regression and survival-SVM models were used to divide the data set into the training and validation sets in a 7:3 ratio using random with release sampling. The basic situation of patients is shown in Table 1.
Table 1
Characteristics | Data (n=1,638), n (%) |
---|---|
Age (years) | |
<40 | 244 (14.9) |
40–49 | 310 (18.9) |
50–59 | 480 (29.3) |
60–69 | 369 (22.5) |
≥70 | 235 (14.3) |
Sex | |
Male | 1,143 (69.8) |
Female | 495 (30.2) |
Race | |
White | 761 (46.5) |
Black | 174 (10.6) |
American Indian/Alaska Native | 28 (1.7) |
Asian or Pacific Islander | 675 (41.2) |
Primary site | |
C11.0-superior wall of nasopharynx | 28 (1.7) |
C11.1-posterior wall of nasopharynx | 210 (12.8) |
C11.2-lateral wall of nasopharynx | 149 (9.1) |
C11.3-anterior wall of nasopharynx | 18 (1.1) |
C11.8-overlapping lesion of nasopharynx | 67 (4.1) |
C11.9-nasopharynx, NOS | 1,166 (71.2) |
Grade | |
Well differentiated; grade I | 45 (2.7) |
Moderately differentiated; grade II | 204 (12.5) |
Poorly differentiated; grade III | 682 (41.6) |
Undifferentiated; anaplastic; grade IV | 707 (43.2) |
Stage | |
I | 155 (9.5) |
II | 360 (22.0) |
III | 500 (30.5) |
IV | 623 (38.0) |
T | |
T1 | 593 (36.2) |
T2 | 324 (19.8) |
T3 | 338 (20.6) |
T4 | 383 (23.4) |
N | |
N0 | 401 (24.5) |
N1 | 538 (32.8) |
N2 | 475 (29.0) |
N3 | 224 (13.7) |
M | |
M0 | 1,519 (92.7) |
M1 | 119 (7.3) |
Reason no cancer-directed surgery | |
Not recommended | 1,413 (86.3) |
Recommended but not performed | 27 (1.6) |
Surgery performed | 198 (12.1) |
Radiation | |
No | 11 (0.7) |
Yes | 1,627 (99.3) |
Chemotherapy | |
No | 212 (12.9) |
Yes | 1,426 (87.1) |
Bone | |
No | 1,584 (96.7) |
Yes | 54 (3.3) |
Brain | |
No | 1,629 (99.5) |
Yes | 9 (0.5) |
Liver | |
No | 1,620 (98.9) |
Yes | 18 (1.1) |
Lung | |
No | 1,597 (97.5) |
Yes | 41 (2.5) |
Surg/Rad Seq | |
No radiation and/or cancer-directed surgery | 1,127 (68.8) |
Radiation after surgery | 495 (30.2) |
Radiation prior to surgery | 13 (0.8) |
Radiation before and after surgery | 3 (0.2) |
Ethnicity | |
White | 761 (46.5) |
Black | 174 (10.6) |
Chinese | 355 (21.7) |
South and Southeast Asia | 174 (10.6) |
Pacific Islanders | 74 (4.5) |
Other Asian | 100 (6.1) |
Marital status | |
Have a partner | 1,029 (62.8) |
No partner | 609 (37.2) |
NOS, not otherwise specified.
According to the Cox regression model results, the training set C index was 0.740, and the AUC value was 0.723. The validation set C index was 0.721, and the AUC value was 0.718. Calibration curve for 3-year survival for patient survival prognosis was derived by the Cox regression model, as shown in Figure 1.
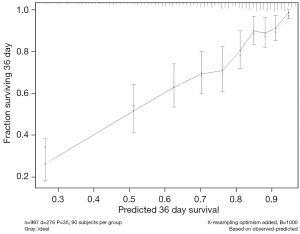
The modeling process of the RSF is shown in Figure 2. Using the RSF, we obtained the survival curves of the NPC patients, as well as curves for out-of-bag (OOB) brier, OOB CRPS, and OOB mortality vs. time over time, as shown in Figure 3. In Figure 4, the OOB error rate shows that the OOB error rate tended to be stable when the number of trees reached 60, which indicated that our model had good stability. According to RSF results, the C-index of the model was 0.729, which indicated that the model had good accuracy. The advantage of RSF is that it can calculate the VIMP value of each variable by the VIMP method and thus rank the importance of variables. When the value of VIMP was greater than 0, the accuracy of prediction improved, and the accuracy of the model was reduced. Figure 5 shows the importance ranking of each variable and the influence of variables on the accuracy of the model. Among them, the M stage had the largest weight, followed by grade, and lung and bone metastases had a greater impact on the assessment of distant tumor metastasis. Whether chemotherapy or radiotherapy had been performed was also an important factor affecting the survival prognosis of NPC patients. In addition, marital status, gender, and race decreased the accuracy of the model prediction.
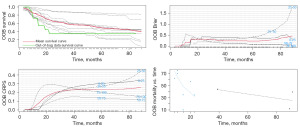
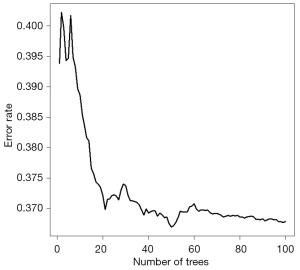
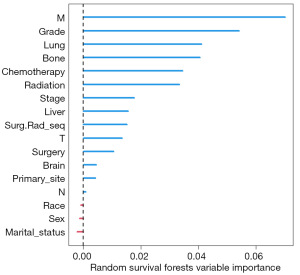
The C-index of survival-SVM was 0.785, which showed that the model had good predictive ability.
Discussion
In this retrospective clinical data study, the survival prognosis of NPC patients was investigated by using the Cox regression model, RSF, and survival-SVM. Survival-SVM was better than the other two methods. Survival SVM is evolved from SVM upgrade. Therefore, it inherits the advantages of SVM, that is, the model is suitable for small sample sizes of data (12). This study relied on the SEER database and included a total of 1,638 patients. However, in practical plain work, the sample size of a single hospital may be less. So, survival-SVM had a good unadorned application value. RSF can rank and visualize the importance of variables (24), which can elucidate the analysis results more clearly and intuitively. As a semi-parametric model, the accuracy of the Cox model was slightly inferior to the other two models. With the advent of the machine learning era, the competitive advantage of traditional Cox may be slowly masked.
The results of this study showed that sex, race, primary site-labeled, grade, AJCC Stage Group, AJCC T, AJCC N, AJCC M, reason no cancer-directed surgery, radiation recode, chemotherapy recode, Mets at DX-bone, Mets at DX-brain, Mets at DX-liver, Mets at DX-lung, Surg/Rad Seq, and Marital status at diagnosis were all independent factors affecting the survival prognosis of NPC patients. Age is among the most significant factors affecting NPC. The incidence of NPC was more common after the age of 40 years old (25), but incidence also tended to be younger than this age. The older the patient was, the worse the prognosis was; this may be associated with factors such as body immunity and drug resistance in the elderly (26). At present, several studies suggested that NPC pathogenesis may be related to genetics (26-28). So, groups with a family history of inheritance should attach great importance to regular physical examination and undergo assessment regularly. This study found that gender had no significant effect on the survival prognosis of NPC patients. Existing studies showed that NPC is prevalent in yellow people (28), and in addition to genetic factors, differences in culture, diet, and lifestyle habits among different ethnic groups, may be responsible for ethnic differences (26,29). From the traditional histological grade and tumor stage of clinical malignant tumors, the prognosis of well-differentiated tumors was much better than that of poorly differentiated tumors. From the AJCC stage, the prognosis of patients at the T1N0M0 stage was generally better than that of patients at a later stage. The study further found that patients who underwent tumor resection surgery, as well as patients who underwent systemic therapy, tended to have a better prognosis. NPC, as a special squamous cell carcinoma of the head and neck, is considerably sensitive to both radiotherapy and chemotherapy (30), and the results of this study suggest that patients can improve their prognosis regardless of whether they choose to undergo chemotherapy or radiotherapy. The survival prognosis of patients who already developed tumor metastasis was poor, and for such patients, radiotherapy plus chemotherapy treatment modalities can improve survival prognosis. For the marital status of patients, this study found that patients with spouses had a better survival prognosis than patients without spouses, which may be related to the pacifying care of spouses (31). We found during the literature survey that no study applied survival-SVM and survival analysis of NPC patients, and RSF has not yet been carried out in the field of survival analysis of NPC patients. With the development of random machine learning, NPC as a specific squamous-cell carcinoma, has a relatively higher degree of malignancy compared with other cancers (32), and the treatment is based on chemoradiotherapy (33). Therefore, the development of a prediction system specifically for NPC can contribute to more accurate treatment research for NPC patients.
The VIMP of RSF showed that M and grade were the factors that accounted for a finer weight, and M stage had a much finer impact than T and N compared with traditional clinical stage. It was equally important to find that radiotherapy and chemotherapy were the key factors affecting the survival prognosis of NPC patients and affected whether the patients who underwent systemic therapy were closely related to the survival prognosis of surgery (3). The study also demonstrated that the survival prognosis of yellow people, especially Asians, was much lower than that of other races.
This study still had some limitations. In terms of data, the data in this paper were all from the data in the SEER database. Considering that the current data collection work was affected by many factors, the data collection and analysis in China will be introduced in the next article. In addition, due to the sample’s unitedness, the generalization ability of the model may have some limitations Subsequently, we plan to apply the model to other types of data to verify its generalization ability. Limitations are as follows. In terms of data volume, data collection mainly in Europe and America was affected by a variety of factors. Data collection in China will be introduced in the next article. The model came from SEER and mainly contained European and American data. There may be limitations in the generalization ability of the model. The subsequent validation of the generalization ability of the model on other data will be considered.
Conclusions
The research findings have demonstrated the superior performance of survival-SVM in the survival analysis of nasopharyngeal cancer patients when compared to the other two methods. Survival-SVM, inheriting the advantages of SVM, particularly excels in scenarios with limited sample sizes, underscoring its potential clinical value.
The application of the RSF method proves effective in quantifying variable importance and facilitating its visualization. This contributes to a more lucid comprehension of the analytical outcomes, providing an intuitive data analysis tool.
Traditional Cox regression, as a model, exhibits a slightly inferior precision when contrasted with the other two models and may progressively lose its competitive edge in the era of machine learning.
Through a comprehensive literature review, it has come to light that research regarding the utilization of survival-SVM in the context of survival analysis for nasopharyngeal cancer patients is notably absent. Similarly, the application of the RSF method in this domain remains unexplored territory. This presents intriguing avenues for future research endeavors.
In summary, with the advancement of machine learning, survival analysis for nasopharyngeal cancer patients, particularly employing modern methodologies such as survival-SVM and RSF, holds promising clinical applicability. These studies bear significant relevance in the formulation of more precise treatment strategies and the development of prognostic systems for nasopharyngeal cancer patients.
Acknowledgments
Funding: Funding was provided by
Footnote
Reporting Checklist: The authors have completed the TRIPOD reporting checklist. Available at https://tcr.amegroups.com/article/view/10.21037/tcr-23-316/rc
Peer Review File: Available at https://tcr.amegroups.com/article/view/10.21037/tcr-23-316/prf
Conflicts of Interest: All authors have completed the ICMJE uniform disclosure form (available at https://tcr.amegroups.com/article/view/10.21037/tcr-23-316/coif). The authors have no conflicts of interest to declare.
Ethical Statement: The authors are accountable for all aspects of the work in ensuring that questions related to the accuracy or integrity of any part of the work are appropriately investigated and resolved. This study was conducted in accordance with the Declaration of Helsinki (as revised in 2013). Guangxi Medical University Institutional Review Board deemed this study exempt from review.
Open Access Statement: This is an Open Access article distributed in accordance with the Creative Commons Attribution-NonCommercial-NoDerivs 4.0 International License (CC BY-NC-ND 4.0), which permits the non-commercial replication and distribution of the article with the strict proviso that no changes or edits are made and the original work is properly cited (including links to both the formal publication through the relevant DOI and the license). See: https://creativecommons.org/licenses/by-nc-nd/4.0/.
References
- Chen YP, Chan ATC, Le QT, et al. Nasopharyngeal carcinoma. Lancet 2019;394:64-80. [Crossref] [PubMed]
- Kuan EC, Alonso JE, Arshi A, et al. Nasopharyngeal adenocarcinoma: A population-based analysis. Am J Otolaryngol 2017;38:297-300. [Crossref] [PubMed]
- Sun J, Huang Z, Hu Z, et al. Benefits of local tumor excision and pharyngectomy on the survival of nasopharyngeal carcinoma patients: a retrospective observational study based on SEER database. J Transl Med 2017;15:116. [Crossref] [PubMed]
- Patel VJ, Chen NW, Resto VA. Racial and Ethnic Disparities in Nasopharyngeal Cancer Survival in the United States. Otolaryngol Head Neck Surg 2017;156:122-31. [Crossref] [PubMed]
- Wang CC, Yang CC, Yeh SA, et al. The Impact of Insurance and Marital Status on Survival in Patients with Nasopharyngeal Carcinoma. Biology (Basel) 2020;9:84. [Crossref] [PubMed]
- Pan JJ, Ng WT, Zong JF, et al. Prognostic nomogram for refining the prognostication of the proposed 8th edition of the AJCC/UICC staging system for nasopharyngeal cancer in the era of intensity-modulated radiotherapy. Cancer 2016;122:3307-15.
- OuYang PY, You KY, Zhang LN, et al. External validity of a prognostic nomogram for locoregionally advanced nasopharyngeal carcinoma based on the 8th edition of the AJCC/UICC staging system: a retrospective cohort study. Cancer Commun (Lond) 2018;38:55.
- Wongvibulsin S, Wu KC, Zeger SL. Clinical risk prediction with random forests for survival, longitudinal, and multivariate (RF-SLAM) data analysis. BMC Med Res Methodol 2019;20:1. [Crossref] [PubMed]
- Zhang ZQ, He G, Luo ZW, et al. Individual mortality risk predictive system of patients with acute-on-chronic liver failure based on a random survival forest model. Chin Med J (Engl) 2021;134:1701-8. [Crossref] [PubMed]
- Zhang L, Huang T, Xu F, et al. Prediction of prognosis in elderly patients with sepsis based on machine learning (random survival forest). BMC Emerg Med 2022;22:26. [Crossref] [PubMed]
- Mohammed M, Mboya IB, Mwambi H, et al. Predictors of colorectal cancer survival using cox regression and random survival forests models based on gene expression data. PLoS One 2021;16:e0261625. [Crossref] [PubMed]
- Liu Z, Chen D, Tian G, et al. Efficient support vector machine method for survival prediction with SEER data. Adv Exp Med Biol 2010;680:11-8. [Crossref] [PubMed]
- Janz TA, Graboyes EM, Nguyen SA, et al. A Comparison of the NCDB and SEER Database for Research Involving Head and Neck Cancer. Otolaryngol Head Neck Surg 2019;160:284-94. [Crossref] [PubMed]
- Cox DR. Regression Models and Life-Tables. Journal of the Royal Statistical Society: Series B (Methodological) 1972;34:187-202. [Crossref]
- Bice N, Kirby N, Bahr T, et al. Deep learning-based survival analysis for brain metastasis patients with the national cancer database. J Appl Clin Med Phys 2020;21:187-92. [Crossref] [PubMed]
- Zhou X, Nakamura K, Sahara N, et al. Deep Learning-Based Recurrence Prediction of Atrial Fibrillation After Catheter Ablation. Circ J 2022;86:299-308. [Crossref] [PubMed]
- Ishwaran H, Kogalur UB, Blackstone EH, et al. Random survival forests. The Ann Appl Stat 2008;2:841-60. [Crossref]
- Farhadian M, Dehdar Karsidani S, Mozayanimonfared A, et al. Risk factors associated with major adverse cardiac and cerebrovascular events following percutaneous coronary intervention: a 10-year follow-up comparing random survival forest and Cox proportional-hazards model. BMC Cardiovasc Disord 2021;21:38. [Crossref] [PubMed]
- Leger S, Zwanenburg A, Pilz K, et al. A comparative study of machine learning methods for time-to-event survival data for radiomics risk modelling. Sci Rep 2017;7:13206. [Crossref] [PubMed]
- Park JH, Choi J, Lee S, et al. Use of Time-to-Event Analysis to Develop On-Scene Return of Spontaneous Circulation Prediction for Out-of-Hospital Cardiac Arrest Patients. Ann Emerg Med 2022;79:132-44. [Crossref] [PubMed]
- Liu Y, Zhou S, Wei H, et al. A comparative study of forest methods for time-to-event data: variable selection and predictive performance. BMC Med Res Methodol 2021;21:193. [Crossref] [PubMed]
- Zhou Y, McArdle JJ. Rationale and Applications of Survival Tree and Survival Ensemble Methods. Psychometrika 2015;80:811-33. [Crossref] [PubMed]
- Van Belle V, Pelckmans K, Van Huffel S, et al. Support vector methods for survival analysis: a comparison between ranking and regression approaches. Artif Intell Med 2011;53:107-18. [Crossref] [PubMed]
- Howard FM, Kochanny S, Koshy M, et al. Machine Learning-Guided Adjuvant Treatment of Head and Neck Cancer. JAMA Netw Open 2020;3:e2025881. [Crossref] [PubMed]
- Unsal AA, Booth JR, Rossi NA, et al. Basaloid nasopharyngeal carcinoma: A population-based analysis of a rare tumor. Laryngoscope 2019;129:2727-32. [Crossref] [PubMed]
- Chen Y, Chang ET, Liu Z, et al. Residence characteristics and risk of nasopharyngeal carcinoma in southern China: A population-based case-control study. Environ Int 2021;151:106455. [Crossref] [PubMed]
- Liu W, Yu B, Luo Y, et al. Survival benefit of induction chemotherapy for locally advanced nasopharyngeal carcinoma: prognosis based on a new risk estimation model. BMC Cancer 2021;21:639. [Crossref] [PubMed]
- Guo R, Wu H, Wang J, et al. Lymph Node Status and Outcomes for Nasopharyngeal Carcinoma According to Histological Subtypes: A SEER Population-Based Retrospective Analysis. Adv Ther 2019;36:3123-33. [Crossref] [PubMed]
- Huang Y, Chen W, Haque W, et al. The impact of comorbidity on overall survival in elderly nasopharyngeal carcinoma patients: a National Cancer Data Base analysis. Cancer Med 2018;7:1093-101. [Crossref] [PubMed]
- Pan XB, Li L, Qu S, et al. The efficacy of chemotherapy in survival of stage II nasopharyngeal carcinoma. Oral Oncol 2020;101:104520. [Crossref] [PubMed]
- Zhou L, Shen N, Li G, et al. The racial disparity of nasopharyngeal carcinoma based on the database analysis. Am J Otolaryngol 2019;40:102288. [Crossref] [PubMed]
- Gabani P, Barnes J, Lin AJ, et al. Induction chemotherapy in the treatment of nasopharyngeal carcinoma: Clinical outcomes and patterns of care. Cancer Med 2018;7:3592-603. [Crossref] [PubMed]
- Hu J, Kong L, Gao J, et al. Use of Radiation Therapy in Metastatic Nasopharyngeal Cancer Improves Survival: A SEER Analysis. Sci Rep 2017;7:721. [Crossref] [PubMed]