A nomogram based on clinical features and molecular abnormalities for predicting the prognosis of patients with acute myeloid leukemia
Highlight box
Key findings
• In this study, we combined patient characteristics and molecular abnormalities to develop a prognostic model for patients with acute myeloid leukemia (AML).
What is known and what is new?
• Many AML prognosis models exist, but most are specific to a particular type of patient and lacks external validation.
• In this study, we constructed a novel prognostic model based on data from 238 AML patients in the Gene Expression Omnibus database and externally validated it with 135 patients from a single centre in northwest China.
What is the implication, and what should change now?
• The nomogram and free web-based calculator allow for the rapid assessment and accurate calculation of individual survival probabilities for the needs of precision medicine and personalised cancer management.
Introduction
Acute myeloid leukemia (AML) is a highly aggressive hematological malignancy involving myeloid precursor cells and it is more common in adults and has a poorer prognosis, particularly in the elderly. Survival rates for adults with AML are significantly poorer compared to children and adolescents. The 5-year survival rates for patients aged 20–49, 50–64 and 65 years and older have been estimated at 58%, 35% and 9% respectively (1). With the increasing research on the molecular genetics of leukemogenesis in recent years, novel agents (e.g., CPX-351), targeted drugs [e.g., BCL-2 inhibitor (venetoclax), FLT3 inhibitor (gilteritinib), IDH1/2 inhibitor (ivosidenib and enasidenib), TP53 activator (APR-246), etc.] and emerging therapeutic modalities (e.g., microtransplantation, immunotherapy) have been promoted for use in patients of all ages.
AML has a high degree of clinical and genomic heterogeneity, and therefore risk stratification at diagnosis plays a crucial role in the development of treatment plans. Current risk stratification for AML and treatment options are determined by a relatively limited number of genetic characteristics. The 2017 European Leukemia Network (ELN) risk stratification criteria rely heavily on cytogenetic abnormalities (2), but this scoring system is still limited by a variety of heterogeneities. A variety of models have been reported that can be used to assess the prognosis of AML patients, such as the model for elderly AML patients with normal karyotype, proposed by Wang et al. (3), the scoring model for AML with biallelic CEBPA mutations developed by Xu et al. (4), and a novel molecular marker-based prognostic model for AML patients developed by Qu et al. (5). However, most models in the literature are specific to certain types of AML patients and lack external data validation, which further reduces the extrapolation and credibility of the models. Therefore, there is an urgent need to integrate clinical and genomic features of AML patients to construct new prognostic models.
Our research focuses on developing risk model that incorporate additional baseline characteristics to help clinicians estimate the survival of AML patients. We present this article in accordance with the TRIPOD reporting checklist (available at https://tcr.amegroups.com/article/view/10.21037/tcr-23-1192/rc).
Methods
Ethical approval
The study was conducted in accordance with the Declaration of Helsinki (as revised in 2013). The study was approved by the Ethics Committee of the First Hospital of Lanzhou University (No. LDYYLL-2023-372). The requirement for informed consent was waived due to the retrospective nature of the study.
Data collection
GSE146173 (6) was downloaded from the Gene Expression Omnibus (GEO) database to construct the model. Adult patients diagnosed with AML were included in the statistical analysis and were required to have complete baseline characteristics and follow-up data. Study variables included age, sex, white blood cell count, hemoglobin concentration, type of AML (primary or secondary AML) and genetic mutations including ASXL1, biallelic CEBPA, DNMT3A, EZH2, FLT3-ITD, FLT3-TKD, IDH1, IDH2, NPM1, RUNX1, TET2, TP53. The primary endpoint was overall survival (OS) at 1, 2 and 3 years, with OS defined as the period from the initial diagnosis to either death or the final follow-up. In addition, we gathered clinical data from 135 adult AML patients attending the First Hospital of Lanzhou University from December 2017 to June 2022 to externally validate the model.
Variable selection
We started with random grouping using the ‘createDataPartion’ function in the ‘CARET’ package of the R software. The entire GEO set was divided into a training set and a validation set on a 1:1 ratio. In the training set, the ‘survival’ package was used for univariate and multivariate Cox regression analysis, and the ‘glmnet’ package was used for least absolute shrinkage and selection operator (LASSO) regression analysis to address multicollinearity.
Nomogram construction
The nomogram function in the rms R package was used to construct a nomogram containing all the key prognostic factors. By matching each variable in the model, we calculated the total score and the survival rates at 1, 2 and 3 years.
Statistical analysis methods
The discriminatory power of the nomogram was mainly indicated by the consistency index (C-index) and the area under the curve (AUC). The calibration curve reflected the extent to which the model’s predicted risk was consistent with the actual risk of morbidity. A risk score consisting of the five factors in the nomogram was calculated based on the regression coefficients from the multivariate Cox regression analysis, and the Kaplan-Meier curve was used as the threshold for prognostic survival analysis of patients with high-risk scores versus those with low-risk scores, using the median risk score as the cut-off point. Decision curve analysis (DCA) was performed using the R software package ‘ggDCA’ to analyse the impact of the model on the net clinical benefit at different positive thresholds. In addition, these metrics were also evaluated in the validation set, the entire GEO set and the northwest China set.
Comparisons of categorical and continuous variables were made using the Chi-squared test and Wilcoxon rank sum test, respectively. Statistics and graphs were generated using R 4.2.2 (www.r-project.org), and commands used with R software were available in the Appendix 1.
Results
Patient characteristics
In this study, data containing 246 adult AML cases were downloaded from the GEO database. A total of 238 adult AML patients were screened. Patients were randomised 1:1 into two sets with similar clinical characteristics. Patients of the training set had a median OS of 21.9 months [interquartile range (IQR), 7.3–46.2 months], while patients of the validation set had a median OS of 21.7 months (IQR, 7.1–45.9 months). We also listed the clinical characteristics of the patients in both sets (Tables 1,2).
Table 1
Variable | Level | Entire GEO set | P† | Northwest China set (n=135) | |
---|---|---|---|---|---|
Training set (n=120) | Validation set (n=118) | ||||
Sex | Female | 64 (53.33) | 60 (50.85) | 0.80 | 67 (49.63) |
Male | 56 (46.67) | 58 (49.15) | 68 (50.37) | ||
Age (years) | <65 | 92 (76.67) | 76 (64.41) | 0.05 | 99 (73.33) |
≥65 | 28 (23.33) | 42 (35.59) | 36 (26.67) | ||
Type of AML | pAML | 100 (83.33) | 96 (81.36) | 0.82 | 119 (88.15) |
sAML | 20 (16.67) | 22 (18.64) | 16 (11.85) | ||
WBC (×109/L) | <50 | 90 (75.0) | 98 (83.05) | 0.17 | 99 (73.33) |
≥50 | 30 (25.0) | 20 (16.95) | 36 (26.67) | ||
Hb (g/L) | <60 | 4 (3.33) | 3 (2.54) | 0.77 | 36 (26.67) |
60 to <100 | 83 (69.17) | 78 (66.1) | 80 (59.26) | ||
≥100 | 33 (27.5) | 37 (31.36) | 19 (14.07) | ||
ASXL1 | Wild-type | 105 (87.5) | 101 (85.59) | 0.81 | 124 (91.85) |
Mutated | 15 (12.5) | 17 (14.41) | 11 (8.15) | ||
Biallelic CEBPA | Wild-type | 114 (95.0) | 116 (98.31) | 0.29 | 118 (87.41) |
Mutated | 6 (5.0) | 2 (1.69) | 17 (12.59) | ||
DNMT3A | Wild-type | 77 (64.17) | 86 (72.88) | 0.19 | 118 (87.41) |
Mutated | 43 (35.83) | 32 (27.12) | 17 (12.59) | ||
EZH2 | Wild-type | 119 (99.17) | 115 (97.46) | 0.60 | 128 (94.81) |
Mutated | 1 (0.83) | 3 (2.54) | 7 (5.19) | ||
FLT3-ITD | Wild-type | 95 (79.17) | 97 (82.2) | 0.67 | 104 (77.04) |
Mutated | 25 (20.83) | 21 (17.8) | 31 (22.96) | ||
FLT3-TKD | Wild-type | 107 (89.17) | 109 (92.37) | 0.53 | 126 (93.33) |
Mutated | 13 (10.83) | 9 (7.63) | 9 (6.67) | ||
IDH1 | Wild-type | 112 (93.33) | 109 (92.37) | 0.97 | 127 (94.07) |
Mutated | 8 (6.67) | 9 (7.63) | 8 (5.93) | ||
IDH2 | Wild-type | 105 (87.5) | 102 (86.44) | 0.96 | 117 (86.67) |
Mutated | 15 (12.5) | 16 (13.56) | 18 (13.33) | ||
NPM1 | Wild-type | 75 (62.5) | 84 (71.19) | 0.20 | 100 (74.07) |
Mutated | 45 (37.5) | 34 (28.81) | 35 (25.93) | ||
RUNX1 | Wild-type | 103 (85.83) | 89 (75.42) | 0.06 | 126 (93.33) |
Mutated | 17 (14.17) | 29 (24.58) | 9 (6.67) | ||
TET2 | Wild-type | 98 (81.67) | 101 (85.59) | 0.52 | 129 (95.56) |
Mutated | 22 (18.33) | 17 (14.41) | 6 (4.44) | ||
TP53 | Wild-type | 110 (91.67) | 110 (93.22) | 0.84 | 128 (94.81) |
Mutated | 10 (8.33) | 8 (6.78) | 7 (5.19) |
Data are shown as n (%). †, P values represent the statistical difference between the training and validation sets for each feature. GEO, Gene Expression Omnibus; AML, acute myeloid leukemia; sAML, secondary AML; pAML, primary AML; WBC, white blood cell count; Hb, hemoglobin.
Table 2
Variable | Entire GEO set | Northwest China set (n=135) | |
---|---|---|---|
Training set (n=120) | Validation set (n=118) | ||
Age (years) | 57 [45.75–64] | 59 [45–67] | 51 [44–66] |
WBC (×109/L) | 16.34 [6.05–47.25] | 13.95 [3.62–35.43] | 13.68 [2.95–51.9] |
Hb (g/L) | 90 [81–102] | 89.5 [82–103.75] | 73 [58.5–88] |
Data are shown as median [IQR]. GEO, Gene Expression Omnibus; WBC, white blood cell count; Hb, hemoglobin; IQR, interquartile range.
Screening for prognosis-related variables
We used univariate Cox regression to screen all prognostic risk factors (age, sex, white blood cell count, hemoglobin, type of AML, 12 AML-associated gene mutations) in the training set (Table 3). Univariate Cox regression analysis showed that age, type of AML, ASXL1, CEBPA, DNMT3A, IDH2, RUNX1 and TP53 mutations were all associated with OS, and further analysis was conducted using LASSO-Cox regression analysis (Figure 1A,1B). Finally, age, type of AML, DNMT3A, IDH2 and TP53 mutations were identified as key independent prognostic factors by multivariate Cox regression analysis (Table 3).
Table 3
Variable | Comparison groups | Univariate analysis | Multivariable analysis | |||
---|---|---|---|---|---|---|
HR (95% CI) | P | HR (95% CI) | P | |||
Gender | Male vs. female | 1.05 (0.66–1.67) | 0.83 | |||
Age (years) | ≥65 vs. <65 | 2.26 (1.37–3.72) | <0.001 | 2.04 (1.22–3.43) | 0.007 | |
WBC (×109/L) | ≥50 vs. <50 | 1.21 (0.71–2.07) | 0.48 | |||
Hb (g/L) | 60 to 100 vs. <60 | 0.65 (0.2–2.08) | 0.47 | |||
≥100 vs. <60 | 0.47 (0.14–1.62) | 0.23 | ||||
Type of AML | sAML vs. pAML | 1.69 (0.95–2.99) | 0.07 | 2.16 (1.15–4.06) | 0.02 | |
ASXL1 | Mutated vs. wild-type | 1.54 (0.81–2.93) | 0.19 | 1.44 (0.59–3.52) | 0.43 | |
CEBPA | Mutated vs. wild-type | 0.22 (0.03–1.58) | 0.13 | 0.48 (0.07–3.6) | 0.48 | |
DNMT3A | Mutated vs. wild-type | 1.63 (1.02–2.61) | 0.04 | 1.65 (1–2.71) | 0.05 | |
EZH2 | Mutated vs. wild-type | 1.92 (0.26–13.89) | 0.52 | |||
FLT3-ITD | Mutated vs. wild-type | 0.99 (0.56–1.75) | 0.97 | |||
FLT3-TKD | Mutated vs. wild-type | 1.07 (0.53–2.16) | 0.84 | |||
IDH1 | Mutated vs. wild-type | 1.58 (0.68–3.64) | 0.29 | |||
IDH2 | Mutated vs. wild-type | 1.85 (0.99–3.44) | 0.05 | 2.43 (1.27–4.64) | 0.007 | |
NPM1 | Mutated vs. wild-type | 0.76 (0.47–1.23) | 0.26 | |||
RUNX1 | Mutated vs. wild-type | 1.63 (0.89–2.99) | 0.11 | 1.24 (0.55–2.78) | 0.61 | |
TET2 | Mutated vs. wild-type | 0.71 (0.37–1.34) | 0.28 | |||
TP53 | Mutated vs. wild-type | 5.14 (2.54–10.39) | <0.001 | 7.19 (3.37–15.34) | <0.001 |
OS, overall survival; HR, hazard ratio; CI, confidence interval; WBC, white blood cell count; Hb, hemoglobin; AML, acute myeloid leukemia; sAML, secondary AML; pAML, primary AML.
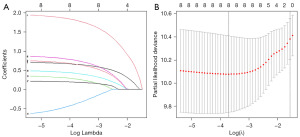
Model development
Based on the prognosis-related independent factors screened by the final multivariate Cox regression analysis, we constructed an OS-related nomogram, as shown in Figure 2. The model, although incorporating only five variables, was more cumbersome to calculate the total score. To facilitate clinicians, we used the ‘DynNom’ package and borrowed shinyapp. io to set up an online server (https://gyc2415940441.shinyapps.io/dynnomapp/) that allows for more intuitive access to the predicted values of patient survival with 95% confidence intervals (CI) (Figure 3).
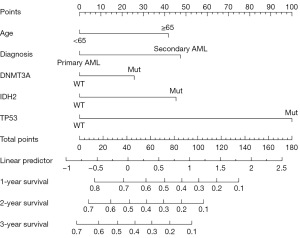
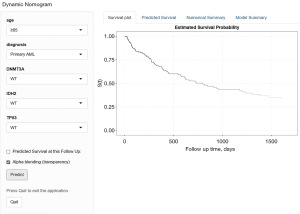
Model validation and performance
Each set was assessed and validated using receiver operating characteristic (ROC) curves, calibration curves, and DCA (Figure 4). In the training set, the C-index for predicting OS was 0.70. The AUC values for 1-, 2- and 3-year OS were 0.755 (95% CI: 66.30 to 84.77), 0.745 (95% CI: 65.90 to 83.17) and 0.757 (95% CI: 67.22 to 84.13), respectively (Figure 4A). In the validation set, the C-index was 0.61 and the AUC values for 1-, 2- and 3-year OS were 0.648 (95% CI: 54.23 to 75.46), 0.648 (95% CI: 54.91 to 74.58) and 0.654 (95% CI: 55.40 to 75.31), respectively (Figure 4B). Meanwhile, the calibration curves showed that the predicted probabilities of OS in the training set and the validation set were generally consistent with the actual probabilities (Figure 4D,4E).
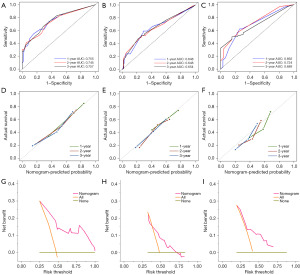
To further assess the validity and performance of the prognostic model, 135 patients from a single-centre set in northwest China were used for validation (Tables 1,2). The C-index for predicting OS was 0.65. The AUC values for 1-, 2- and 3-year OS were 0.692 (95% CI: 60.13 to 78.26), 0.724 (95% CI: 62.27 to 82.53) and 0.689 (95% CI: 56.86 to 80.92), respectively (Figure 4C). In addition, the calibration curves for the predicted probabilities of 1-, 2-, and 3-year OS also showed adequate consistency (Figure 4F). Furthermore, the model achieved better net benefits in the training set, the internal validation set and the northwest China set, relative to the case of intervention for all and no intervention for all (Figure 4G-4I).
Risk stratification
We divided the patients in each set into high and low risk groups based on the median risk score (cut off at 1.64) in the training set. Both risk score scatter plots and Kaplan-Meier analysis showed poorer survival in high-risk patients (Figure 5A,5B). Similarly, risk scores equally well differentiated between high-risk and low-risk patients in the validation set (Figure 5C,5D), the entire GEO set (Figure 5E,5F) and the northwest China set (Figure 5G,5H).
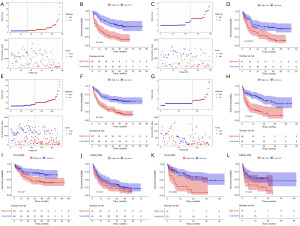
Both young (age <60 years) and elderly patients (age ≥60 years) in the entire GEO set were divided into low- and high-risk groups based on the median risk score, with survival curves showing significantly poorer survival in the high-risk group than in the low-risk group (Figure 5I,5J). Similarly, the model achieved good risk stratification in the northwest China set for young and elderly patients (Figure 5K,5L).
Model comparison
DCA was performed to compare the clinical usability of the nomogram and the ELN 2022 risk stratification model. DCA showed that the nomogram had better clinical applicability, as it added more net benefits compared with the ELN 2022 risk stratification for most of the threshold probabilities in the northwest China set, and with both the treat-all-patients scheme and the treat-none scheme (Figure 6).
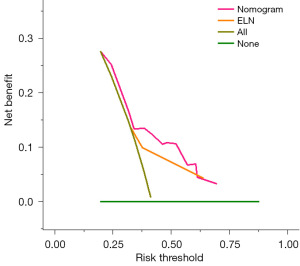
Discussion
In this study, we developed and validated a novel prognostic model for adult AML patients by combining clinical characteristics and 12 prognosis-related gene mutations, and constructed dynamic nomogram. 238 patients with AML were separated into the training set and validation set. The key prognostic variables, including age, AML type, DNMT3A, IDH2 and TP53 mutations, were determined through Cox regression and LASSO regression analysis. Various assessments were undertaken from different perspectives to evaluate the nomograms’ predictive performance and applicability. Additionally, validation of the model was conducted using 135 AML patients from a single centre.
Age at diagnosis has a significant impact on predicting long-term survival in AML patients (7,8). Elderly patients with AML often have an atypical clinical features and poor compliance, they are more likely to have unfavorable cytogenetics and expresses multidrug resistance, all of which contribute to the very poor survival of elderly AML patients (9). In 2020, a study by Martínez-Cuadrón et al. randomised 3,637 elderly AML patients to intensive chemotherapy, standard chemotherapy (10), reduced-intensity chemotherapy and supportive therapy and showed that median OS was 4.7 months, with 29% surviving at 1 year and 7% at 5 years. Many prognostic models have identified secondary AML as a risk factor for early death. Compared to primary AML, patients with secondary AML, who tend to be older and whose vital organs have been affected by radiotherapy for their primary disease, have a poorer prognosis, with significantly lower rates of complete remission (CR), relapse-free survival and OS (11).
As shown in the nomogram, TP53 mutations are strong predictors in our model. TP53 mutations are associated with complex karyotypes, haplotypes and specific chromosomal aneuploidies (e.g., -5/5q-, -7/7q-) (12), they come with an extremely poor prognosis and have been included in the 2022 ELN risk stratification poor prognosis group. In addition, TP53 mutations are the most frequently mutated gene in secondary AML (13). The high mutations rate of TP53 in secondary AML and the selection of resistant clones after mutations together lead to resistance to chemotherapeutic agents in secondary AML, which partly explains the poor prognosis of secondary AML and highlights the need for novel therapies. Similar to the prognostic model of Ma et al. (14), our model includes DNMT3A mutations. The impact of DNMT3A mutations on clinical decision making remains controversial, but numerous studies have shown that AML patients with DNMT3A mutations, especially R882 mutations, have a significantly worse clinical prognosis (15,16). IDH2 mutations occur in 12% of AML patients (17), and there has been controversy regarding the prognostic role of IDH2, as its impact on patient prognosis appears to be influenced by the mutations site and other co-occurring mutational events (18). The IDH2R140 mutation appears to be a favorable independent prognostic factor, while patients with IDH2R172 mutated AML have an extremely poor prognosis (18), with relapse rates comparable to those of patients with poor risk cytogenetics. In conclusion, the association between DNMT3A, IDH2 and AML needs to be further investigated.
Our new model has several advantages. First, the DCA showed that our model outperforms the 2022 ELN stratification in terms of clinical utility. Second, the model was externally validated using an AML dataset from a single centre in northwest China, thereby considerably enhancing the predictive model’s practicality. Above all, the nomogram has been uploaded onto a website that is readily accessible.
There are some limitations in this study. Firstly, cytogenetic classification was not included in this study as most cytogenetic information was missing from the published data. In addition, the northwest China set was retrospective and therefore it was inevitably subject to selection and recall bias. Finally, NPM1 and FLT3-ITD were not mentioned in this prognostic model, probably because the statistical significance of the different effect indicators (usually expressed as P values) depends on the size of the effect, the sample size, and the distribution (variance) of the data. Also, the prognosis of patients with NPM1 mutations or FLT3-ITD is affected by allele frequency and co-mutations (e.g., TP53, EZH2, ASXL1, etc.).
Conclusions
In summary, we have integrated patient- and AML-related information to develop and validate a new scoring system. With the nomogram and free web-based calculator, individual survival probabilities can be quickly assessed and accurately calculated to meet the needs of precision medicine and personalised cancer management. The model’s parameters are routinely assessed and can be easily adopted into clinical practice, enabling clinicians to quickly complete survival assessments, risk stratification and treatment decisions at the time of diagnosis.
Acknowledgments
We thank the patients and investigators who submitted in GEO database for providing high-quality data for analysis in this study. Thanks to the patients who provided clinical data, and the medical testing institutions that provided support.
Funding: This study was supported by
Footnote
Reporting Checklist: The authors have completed the TRIPOD reporting checklist. Available at https://tcr.amegroups.com/article/view/10.21037/tcr-23-1192/rc
Data Sharing Statement: Available at https://tcr.amegroups.com/article/view/10.21037/tcr-23-1192/dss
Peer Review File: Available at https://tcr.amegroups.com/article/view/10.21037/tcr-23-1192/prf
Conflicts of Interest: All authors have completed the ICMJE uniform disclosure form (available at https://tcr.amegroups.com/article/view/10.21037/tcr-23-1192/coif). The authors have no conflicts of interest to declare.
Ethical Statement: The authors are accountable for all aspects of the work in ensuring that questions related to the accuracy or integrity of any part of the work are appropriately investigated and resolved. The study was conducted in accordance with the Declaration of Helsinki (as revised in 2013). The study was approved by the Ethics Committee of the First Hospital of Lanzhou University (No. LDYYLL-2023-372). The requirement for informed consent was waived due to the retrospective nature of the study.
Open Access Statement: This is an Open Access article distributed in accordance with the Creative Commons Attribution-NonCommercial-NoDerivs 4.0 International License (CC BY-NC-ND 4.0), which permits the non-commercial replication and distribution of the article with the strict proviso that no changes or edits are made and the original work is properly cited (including links to both the formal publication through the relevant DOI and the license). See: https://creativecommons.org/licenses/by-nc-nd/4.0/.
References
- Miller KD, Nogueira L, Devasia T, et al. Cancer treatment and survivorship statistics, 2022. CA Cancer J Clin 2022;72:409-36. [Crossref] [PubMed]
- Döhner H, Estey E, Grimwade D, et al. Diagnosis and management of AML in adults: 2017 ELN recommendations from an international expert panel. Blood 2017;129:424-47. [Crossref] [PubMed]
- Wang J, Ma Z, Wang Q, et al. Prognostic utility of six mutated genes for older patients with acute myeloid leukemia. Int J Cancer 2018;142:1664-70. [Crossref] [PubMed]
- Xu X, Cai W, Cai P, et al. Prognostic Nomogram for Acute Myeloid Leukemia Patients With Biallelic CEBPA Mutations. Front Oncol 2021;11:628248. [Crossref] [PubMed]
- Qu Y, Zhang S, Qu Y, et al. Novel Gene Signature Reveals Prognostic Model in Acute Myeloid Leukemia. Front Genet 2020;11:566024. [Crossref] [PubMed]
- Bamopoulos SA, Batcha AMN, Jurinovic V, et al. Clinical presentation and differential splicing of SRSF2, U2AF1 and SF3B1 mutations in patients with acute myeloid leukemia. Leukemia 2020;34:2621-34. [Crossref] [PubMed]
- Hilberink JR, van Zeventer IA, Chitu DA, et al. Age and sex associate with outcome in older AML and high risk MDS patients treated with 10-day decitabine. Blood Cancer J 2023;13:93. [Crossref] [PubMed]
- Lalayanni C, Demosthenous C, Iskas M, et al. Adolescents and young adults (AYA) with acute myeloid leukemia (AML): real-world long-term results and age-specific outcomes. Leuk Lymphoma 2022;63:3128-37. [Crossref] [PubMed]
- de Leeuw DC, Ossenkoppele GJ, Janssen JJWM. Older Patients with Acute Myeloid Leukemia Deserve Individualized Treatment. Curr Oncol Rep 2022;24:1387-400. [Crossref] [PubMed]
- Martínez-Cuadrón D, Serrano J, Gil C, et al. Evolving treatment patterns and outcomes in older patients (≥60 years) with AML: changing everything to change nothing? Leukemia 2021;35:1571-85. [Crossref] [PubMed]
- Granfeldt Østgård LS, Medeiros BC, Sengeløv H, et al. Epidemiology and Clinical Significance of Secondary and Therapy-Related Acute Myeloid Leukemia: A National Population-Based Cohort Study. J Clin Oncol 2015;33:3641-9. [Crossref] [PubMed]
- Grob T, Al Hinai ASA, Sanders MA, et al. Molecular characterization of mutant TP53 acute myeloid leukemia and high-risk myelodysplastic syndrome. Blood 2022;139:2347-54. [Crossref] [PubMed]
- Rahmé R, Braun T, Manfredi JJ, et al. TP53 Alterations in Myelodysplastic Syndromes and Acute Myeloid Leukemia. Biomedicines 2023;11:1152. [Crossref] [PubMed]
- Ma TT, Lin XJ, Cheng WY, et al. Development and validation of a prognostic model for adult patients with acute myeloid leukaemia. EBioMedicine 2020;62:103126. [Crossref] [PubMed]
- Yuan XQ, Chen P, Du YX, et al. Influence of DNMT3A R882 mutations on AML prognosis determined by the allele ratio in Chinese patients. J Transl Med 2019;17:220. [Crossref] [PubMed]
- Wakita S, Marumo A, Morita K, et al. Mutational analysis of DNMT3A improves the prognostic stratification of patients with acute myeloid leukemia. Cancer Sci 2023;114:1297-308. [Crossref] [PubMed]
- Issa GC, DiNardo CD. Acute myeloid leukemia with IDH1 and IDH2 mutations: 2021 treatment algorithm. Blood Cancer J 2021;11:107. [Crossref] [PubMed]
- Bill M, Jentzsch M, Bischof L, et al. Impact of IDH1 and IDH2 mutation detection at diagnosis and in remission in patients with AML receiving allogeneic transplantation. Blood Adv 2023;7:436-44. [Crossref] [PubMed]