Predictive nomograms for risk and prognostic factors in metastatic bladder cancer: a population-based study
Highlight box
Key findings
• We successfully constructed nomograms to evaluate the risk and prognostic factors of metastatic bladder cancer (MBC) with superior discrimination, ex-cellent calibration abilities, and great clinical benefit.
What is known and what is new?
• MBC has a poor prognosis and is associated with many clinical factors.
• New nomograms were created to predict metastatic and prognostic risk based on extensive patient data.
What is the implication, and what should change now?
• Nomograms constructed on the basis of clinical information can easily predict the risk and prognosis of metastasis bladder cancer patients, which has positive implications for patient management.
Introduction
Bladder cancer is a malignant disease with high morbidity and mortality rate, which ranks among the top ten cancers in the world, and the sixth in male population. Despite recent advances in surgical techniques and drug therapies, according to a global survey in 2020, there were 573,278 new cases of bladder cancer and 212,536 new deaths reported worldwide (1), causing a global economic burden on healthcare industry. It has been widely recognized that the high incidence of bladder cancer is associated with smoking and secondhand smoke exposure, and the degree of smoking in patients is also linked linearly to tumor malignancy (2). Studies have shown that nearly 4% of all patients diagnosed with bladder cancer are diagnosed as metastatic bladder cancer (MBC) at initial diagnosis (3). MBC means a worse prognosis, with a median overall survival (OS) of only 13–15 months, even when treated with rigorous chemotherapy regimens (4).
Tumor metastasis is a process that involves multiple mechanisms in the final stages of the tumor process and also ultimately leads to the death of patients (5). The main metastatic sites of bladder cancer are bone, lung, liver and brain, etc. (6). Bone metastases are the most common site of MBC, the majority of patients with bladder cancer can be evaluated by routine chest , abdomen and pelvic enhancement Computed Tomography (CT), but sometimes there exists inadequate staging, so positron emission tomography-computed tomography (PET-CT) is increasingly used in the detection of bladder cancer, especially in patients with muscle-invasive bladder cancer and recurrence after radical cystectomy (7). In the evaluation of the prognosis of patients with MBC, there have been nomograms constructed for bone metastasis and brain metastasis to predict the prognosis of patients (6,8), however, predictions for a single metastatic site have limitations, such as the difficulty of applying them broadly to patients with bladder cancer, and they lack application of common clinical indicators. Therefore, the use of common clinical indicators to evaluate the risk and prognosis of MBC is currently needed for providing guidelines to clinicians.
We obtained clinical data of bladder cancer from the Surveillance, Epidemiology, and End Results (SEER) database. After screening, a total of 22,788 patients were included in our study. Two practical nomograms were constructed by clinical information, the risk nomogram for predicting the risk of bladder cancer metastasis and the prognosis nomogram for predicting 1-, 2- and 3-year survival in patients with MBC. Two prediction models have been validated to have favorable clinical utility through the receiver operating characteristic (ROC), decision curve analysis (DCA) curves, clinical impact curves (CIC) and Kaplan-Meier (K-M) survival curves. We present this article in accordance with the TRIPOD reporting checklist (available at https://tcr.amegroups.com/article/view/10.21037/tcr-23-1229/rc).
Methods
Ethical statement
The ethical approval of this study was exempted by the Ethics Committee of the First Affiliated Hospital of Nanchang University as the data were from the publicly accessible database, SEER. The study was conducted in accordance with the Declaration of Helsinki (as revised in 2013).
Patients
We downloaded clinical information from SEER state software (version 8.4.1) of patients with bladder cancer registered from 2010 to 2019. The inclusion criteria were: (I) bladder cancer was the only primary carcinoma; (II) histology type was known; and (III) definitive site of metastasis. Exclusion principles were: (I) unknown cause of death and survival time; (II) unknown race; (III) unknown tumor size; (IV) undetermined T and N stage and grade; (V) lack of surgical, radiotherapy and chemotherapy information. Finally, there were 22,788 patients included in the cohort to study the risk factors of metastasis in bladder cancer patients and to establish a risk nomogram. For the exploration of prognostic factors for MBC, a total of 1,150 patients were enrolled in the whole cohort.
In the analysis of metastatic risk, we included the following clinical information: age at diagnosis, sex, race, histological type, tumor size, grade, T-stage (AJCC 7th edition), N-stage (AJCC 7th edition), primary site. Within the analysis of prognostic influences, besides the above factors, we included treatment and metastatic information, such as bone, lung, liver and brain metastases, surgery, radiotherapy and chemotherapy information.
Statistical analysis and nomogram construction
All statistical analyses were performed by SPSS (version 25.0), x-tile software (version 3.6.1), and software packages (rms, pROC, ggDCA, ggplot and rmda) in R software (version 4.2.3). We used x-tile software to calculate the optimal cut-off values for tumor size and age that affect the prognosis of bladder cancer patients, the results are shown in Figure 1. Chi-squared test or Fisher exact test were deployed to compare categorical data. Through univariate logistic regression analysis, variables with a P<0.05 were included in multivariate logistic regression analysis, the odds ratios (OR) and 95% confidence intervals (CI) were calculated, then the significant risk factors were screened out. Next, risk factors for prognosis in MBC patients were analyzed. All MBC patients were randomly divided into training (n=806) and validation (n=344) cohorts according to the ratio of 7:3 (9). In the training cohort, variables with P<0.05 in univariate cox regression analysis were included in the multivariate cox regression analysis to identify significant prognostic factors, the hazard ratios (HR) and 95% CI were calculated. Finally, we established two nomograms based on risk factors and prognostic factors to predict the risk and OS of MBC. In the risk nomogram, its accuracy and discrimination were evaluated by ROC curves (10), bootstrapping (1,000 resamples) calibration curves (11), and the area under curve (AUC) of ROC. Its clinical utility was assessed by clinical DCA and CIC (12). In the prognostic nomogram, accuracy and discrimination were assessed by ROC curves and calibration curves for 1-, 2-, and 3-year, respectively, and AUC. Its clinical utility was assessed by DCA curves and KM curves. Two tailed P values ≤0.05 was considered statistically significant.
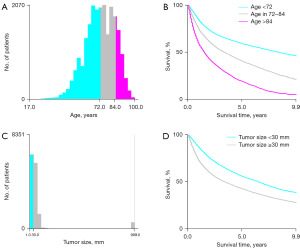
Results
Patient characteristics
After the screening process, date of a total of 22,788 bladder cancer patients were obtained from the SEER database. Among them, 1,150 patients were diagnosed as MBC, 806 cases were grouped into training cohort and 344 cases as validation cohort. Among bladder cancer patients with and without metastasis, there was a difference in the percentage of all risk and prognostic factors except surgery. Whites (88.9%) accounted for the largest proportion of all bladder cancer patients, with little difference in the proportion of transitional cell carcinoma and papillary transitional cell carcinoma (44.8% vs. 48.9%). In terms of treatment style, the vast majority of patients underwent local tumor excision (LTE). Bone metastasis accounted for the highest percentage (36.3%), brain metastasis accounted for the least (2.1%) of patients with MBC. The specific clinical information of patients are shown in Table 1.
Table 1
Characteristics | Without MBC, n (%) | With MBC, n (%) | χ2 | P |
---|---|---|---|---|
All | 21,638 | 1,150 | ||
Age (years) | 16.767 | <0.001 | ||
<72 | 9,669 (44.7) | 564 (49.0) | ||
72–84 | 8,373 (38.7) | 444 (38.6) | ||
>84 | 3,596 (16.6) | 142 (12.3) | ||
Sex | 11.786 | 0.001 | ||
Female | 5,102 (23.5) | 322 (28.0) | ||
Male | 16,536 (76.5) | 828 (72.0) | ||
Race | 14.117 | 0.001 | ||
White | 19,247 (89.1) | 1,001 (87.0) | ||
Black | 1,324 (6.1) | 101 (8.8) | ||
Others | 1,067 (4.9) | 48 (4.2) | ||
Histologic type | 275.751 | <0.001 | ||
Transitional cell carcinoma | 9,546 (44.1) | 674 (58.6) | ||
Papillary transitional cell carcinoma | 10,821 (50.0) | 313 (27.2) | ||
Others | 1,271 (5.9) | 163 (14.2) | ||
Tumor size (mm) | 121.829 | <0.001 | ||
<30 | 4,090 (18.9) | 69 (6.0) | ||
≥30 | 17,548 (81.1) | 1,081 (94.0) | ||
Grade | 68.595 | <0.001 | ||
Well differentiated; I | 630 (2.9) | 12 (1.0) | ||
Moderately differentiated; II | 2,189 (10.1) | 48 (4.2) | ||
Poorly differentiated; III | 4,836 (22.3) | 321 (27.9) | ||
Undifferentiated; anaplastic; IV | 13,983 (64.6) | 769 (66.9) | ||
AJCC T stage | 848.243 | <0.001 | ||
T1 | 11,337 (52.4) | 176 (15.3) | ||
T2 | 7,002 (32.4) | 605 (52.6) | ||
T3 | 2,131 (5.8) | 131 (11.4) | ||
T4 | 1,168 (9.4) | 238 (20.7) | ||
AJCC N stage | 1,671.043 | <0.001 | ||
N0 | 20,057 (92.7) | 692 (60.2) | ||
N1 | 665 (3.1) | 114 (9.9) | ||
N2 | 762 (3.5) | 245 (21.3) | ||
N3 | 154 (0.7) | 99 (8.6) | ||
Primary site | 99.943 | <0.001 | ||
Anterior wall of bladder | 1,088 (5.0) | 54 (4.7) | ||
Bladder neck | 1,238 (5.7) | 88 (7.7) | ||
Dome of bladder | 1,752 (8.1) | 78 (6.8) | ||
Lateral wall of bladder | 6,581(30.4) | 257 (22.3) | ||
Overlapping lesion of bladder | 4,873 (22.5) | 384 (33.4) | ||
Posterior wall of bladder | 3,162 (14.6) | 134 (11.7) | ||
Trigone of bladder | 2,127 (9.8) | 118 (10.3) | ||
Ureteric orifice | 817 (3.8) | 37 (3.2) | ||
Surgery | 0.59 | 0.899 | ||
No | 2,337 (10.8) | 117 (10.2) | ||
LTE | 17,209 (79.5) | 919 (79.9) | ||
PC | 272 (1.3) | 16 (1.4) | ||
RC | 1,820 (8.4) | 98 (8.5) | ||
Radiation | 203.391 | <0.001 | ||
No | 19,537 (90.3) | 887 (77.1) | ||
Yes | 2,101 (9.7) | 263 (22.9) | ||
Chemotherapy | 239.493 | <0.001 | ||
No | 14,003 (64.7) | 486 (42.3) | ||
Yes | 7,635 (35.3) | 664 (57.7) | ||
Bone metastasis | – | – | ||
No | – | 732 (63.7) | ||
Yes | – | 418 (36.3) | ||
Brain metastasis | – | – | ||
No | – | 1,126 (97.9) | ||
Yes | – | 24 (2.1) | ||
Liver metastasis | – | – | ||
No | – | 936 (81.4) | ||
Yes | – | 214 (18.6) | ||
Lung metastasis | – | – | ||
No | – | 781 (67.9) | ||
Yes | – | 369 (32.1) |
MBC, metastatic bladder cancer; LTE, local tumor excision; PC, partial cystectomy; RC, radical cystectomy.
Analysis of independent risk and prognostic factors
In the univariate logistic regression analysis, a total of nine clinical factors were associated with metastasis, while in the multivariate logistic regression analysis, age, histological type, T stage, N stage and tumor size were found to be associated with metastasis, the analysis results are shown in Table 2. In order to construct the prognosis nomogram of MBC, we divided the patients into training cohort and validation cohort, the Chi-squared test and Fisher’s exact test showed that none of the 16 factors were different between the two groups (Table 3). After inclusion of 16 prognostic factors in the training cohort, the prognostic factor of P<0.05 such as age, histological type, tumor size, T stage, N stage, chemotherapy, bone metastasis, liver metastasis and lung metastasis were included in the multivariate cox regression analysis by univariate cox regression analysis. Finally, age, histological type, chemotherapy, bone metastasis, liver metastasis, and lung metastasis were considered to be significantly associated with MBC patient prognosis. The analysis results are shown in Table 4.
Table 2
Characteristics | Univariate analysis | Multivariate analysis | |||
---|---|---|---|---|---|
OR (95% CI) | P | OR (95%CI) | P | ||
Age (years) | |||||
<72 | Reference | Reference | |||
72–84 | 0.895 (0.895–0.797) | 0.088 | 1.017 (0.887–1.167) | 0.807 | |
>84 | 0.672 (0.557–0.811) | <0.001 | 0.799 (0.655–0.976) | 0.028 | |
Sex | |||||
Female | Reference | Reference | |||
Male | 0.793 (0.905–0.906) | 0.001 | 0.930 (0.806–1.074) | 0.322 | |
Race | |||||
White | Reference | Reference | |||
Black | 1.467 (1.186–1.813) | <0.001 | 1.250 (0.994–1.572) | 0.056 | |
Others | 0.865 (0.643–1.163) | 0.337 | 0.888 (0.649–1.216) | 0.46 | |
Histologic type | |||||
Transitional cell carcinoma | Reference | Reference | |||
Papillary transitional cell carcinoma | 0.410 (0.357–0.470) | <0.001 | 0.745 (0.641–0.865) | <0.001 | |
Others | 1.816 (1.516–2.176) | <0.001 | 1.635 (1.330–2.010) | <0.001 | |
Tumor size (mm) | |||||
<30 | Reference | Reference | |||
≥30 | 3.652 (2.856–4.669) | <0.001 | 2.915 (2.263–3.755) | <0.001 | |
Grade | |||||
Well differentiated; I | Reference | Reference | |||
Moderately differentiated; II | 1.151 (0.608–2.181) | 0.666 | 0.910 (0.467–1.774) | 0.782 | |
Poorly differentiated; III | 3.485 (1.947–6.238) | <0.001 | 1.721 (0.933–3.174) | 0.082 | |
Undifferentiated; anaplastic; IV | 2.887 (1.623–5.135) | <0.001 | 1.573 (0.857–2.888) | 0.144 | |
AJCC T stage | |||||
T1 | Reference | Reference | |||
T2 | 5.566 (4.693–6.600) | <0.001 | 3.574 (2.977–4.290) | <0.001 | |
T3 | 3.960 (3.144–4.988) | <0.001 | 1.281 (0.984–1.669) | 0.066 | |
T4 | 13.126 (10.704–16.095) | <0.001 | 3.766 (2.960–4.791) | <0.001 | |
AJCC N stage | |||||
N0 | Reference | Reference | |||
N1 | 4.969 (4.017–6.146) | <0.001 | 3.293 (2.616–4.145) | <0.001 | |
N2 | 9.319 (7.920–10.965) | <0.001 | 6.016 (4.987–7.257) | <0.001 | |
N3 | 18.633 (14.315–24.253) | <0.001 | 13.487 (10.130–17.958) | <0.001 | |
Primary site | |||||
Anterior wall of bladder | Reference | ||||
Bladder neck | 1.432 (0.011–2.029) | 0.043 | 1.332 (0.921–1.927) | 0.127 | |
Dome of bladder | 0.897 (0.625–1.279) | 0.549 | 0.972 (0.670–1.411) | 0.882 | |
Lateral wall of bladder | 0.787 (0.583–1.602) | 0.118 | 0.879 (0.641–1.205) | 0.422 | |
Overlapping lesion of bladder | 1.588 (1.185–2.127) | 0.002 | 1.208 (0.887–1.646) | 0.23 | |
Posterior wall of bladder | 0.854 (0.618–1.180) | 0.338 | 1.011 (0.719–1.421) | 0.95 | |
Trigone of bladder | 1.118 (0.803–1.555) | 0.509 | 1.074 (0.758–1.421) | 0.687 | |
Ureteric orifice | 0.912 (0.595–1.400) | 0.675 | 1.301 (0.830–2.038) | 0.251 |
MBC, metastatic bladder cancer; OR, odds ratios; CI, confidence interval.
Table 3
Characteristics | Training cohort, N or N (%) | Validation cohort, N or N (%) | χ2 | P |
---|---|---|---|---|
All | 806 | 344 | ||
Age (years) | 0.363 | 0.834 | ||
<72 | 402 (49.9) | 166 (48.3) | ||
72–84 | 307 (38.1) | 133 (38.7) | ||
>84 | 97 (12.0) | 45 (13.1) | ||
Sex | 0.451 | 0.502 | ||
Female | 221 (27.4) | 101 (29.4) | ||
Male | 585 (72.6) | 243 (70.6) | ||
Race | 3.389 | 0.384 | ||
White | 708 (87.8) | 293 (85.2) | ||
Black | 70 (8.7) | 31 (9.0) | ||
Others | 28 (3.5) | 20 (5.8) | ||
Histologic type | 0.179 | 0.914 | ||
Transitional cell carcinoma | 473 (58.7) | 201 (58.6) | ||
Papillary transitional cell carcinoma | 217 (26.9) | 96 (27.2) | ||
Others | 116 (14.4) | 47 (14.2) | ||
Tumor size (mm) | 0.03 | 0.893 | ||
<30 | 49 (6.1) | 20 (5.8) | ||
≥30 | 757 (93.9) | 324 (94.2) | ||
Grade | 4.554 | 0.21 | ||
Well differentiated; I | 11 (1.4) | 1 (0.3) | ||
Moderately differentiated; II | 35 (4.3) | 13 (3.8) | ||
Poorly differentiated; III | 215 (26.7) | 106 (30.8) | ||
Undifferentiated; anaplastic; IV | 545 (67.6) | 224 (65.1) | ||
AJCC T stage | 1.954 | 0.582 | ||
T1 | 122 (15.1) | 54 (15.7) | ||
T2 | 416 (51.6) | 189 (54.9) | ||
T3 | 93 (11.5) | 38 (11.0) | ||
T4 | 175 (21.7) | 63 (18.3) | ||
AJCC N stage | 2.618 | 0.454 | ||
N0 | 482 (59.8) | 210 (61.0) | ||
N1 | 83 (10.3) | 31 (9.0) | ||
N2 | 166 (20.6) | 79 (23.0) | ||
N3 | 75 (9.3) | 24 (7.0) | ||
Primary site | 3.939 | 0.787 | ||
Anterior wall of bladder | 38 (4.7) | 16 (4.7) | ||
Bladder neck | 62 (7.7) | 26 (7.6) | ||
Dome of bladder | 57 (7.1) | 21 (6.1) | ||
Lateral wall of bladder | 178 (22.1) | 79 (23.0) | ||
Overlapping lesion of bladder | 271 (33.6) | 113 (32.8) | ||
Posterior wall of bladder | 99 (12.3) | 35 (10.2) | ||
Trigone of bladder | 79 (9.8) | 39 (11.3) | ||
Ureteric orifice | 22 (2.7) | 15 (4.4) | ||
Surgery | 2.533 | 0.469 | ||
No | 79 (9.8) | 38 (11.0) | ||
LTE | 651 (80.8) | 268 (77.9) | ||
PC | 13 (1.6) | 3 (0.9) | ||
RC | 63 (7.8) | 35 (10.2) | ||
Radiation | 2.199 | 0.138 | ||
No | 612 (75.9) | 275 (79.9) | ||
Yes | 194 (24.1) | 69 (20.1) | ||
Chemotherapy | 1.193 | 0.275 | ||
No | 349 (43.3) | 137 (39.8) | ||
Yes | 457 (56.7) | 207 (60.2) | ||
Bone metastasis | 0.165 | 0.684 | ||
No | 510 (63.3) | 222 (64.5) | ||
Yes | 296 (36.7) | 122 (35.5) | ||
Brain metastasis | 1.615 | 0.204 | ||
No | 792 (98.3) | 334 (97.1) | ||
Yes | 14 (1.7) | 10 (2.9) | ||
Liver metastasis | 1.759 | 0.185 | ||
No | 648 (80.4) | 288 (83.7) | ||
Yes | 158 (19.6) | 56 (16.3) | ||
Lung metastasis | 1.415 | 0.234 | ||
No | 556 (69.0) | 225 (65.4) | ||
Yes | 250 (31.0) | 119 (34.6) |
MBC, metastatic bladder cancer; LTE, local tumor excision; PC, partial cystectomy; RC, radical cystectomy.
Table 4
Characteristic | Univariate analysis | Multivariate analysis | |||
---|---|---|---|---|---|
HR (95% CI) | P | HR (95% CI) | P | ||
Age (years) | |||||
<72 | Reference | Reference | |||
72–84 | 1.381 (1.215–1.571) | <0.001 | 1.440 (1.114–1.863) | 0.005 | |
>84 | 1.730 (1.435–2.084) | <0.001 | 1.500 (1.040–2.163) | 0.03 | |
Sex | |||||
Female | Reference | ||||
Male | 0.919 (0.806–1.049) | 0.211 | |||
Race | |||||
White | Reference | ||||
Black | 1.076 (0.874–1.325) | 0.488 | |||
Others | 0.795 (0.585–1.079) | 0.141 | |||
Histologic type | |||||
Transitional cell carcinoma | Reference | Reference | |||
Papillary transitional cell carcinoma | 0.745 (0.580–0.957) | 0.021 | 0.699 (0.537–0.909) | 0.008 | |
Others | 0.847 (0.612–1.174) | 0.32 | 0.729 (0.514–1.035) | 0.077 | |
Tumor size (mm) | |||||
<30 | Reference | Reference | |||
≥30 | 0.683 (0.528–0.883) | 0.004 | 1.539 (0.921–2.571) | 0.1 | |
Grade | |||||
Well differentiated; I | Reference | ||||
Moderately differentiated; II | 0.750 (0.396–1.417) | 0.375 | |||
Poorly differentiated; III | 0.868 (0.488–1.547) | 0.632 | |||
Undifferentiated; anaplastic; IV | 0.789 (0.446–1.396) | 0.415 | |||
AJCC T stage | |||||
T1 | Reference | Reference | |||
T2 | 0.984 (0.829–1.167) | 0.853 | 0.939 (0.683–1.292) | 0.7 | |
T3 | 0.712 (0.563–0.901) | 0.005 | 0.878 (0.558–1.383) | 0.575 | |
T4 | 0.970 (0.795–1.184) | 0.762 | 1.074 (0.720–1.602) | 0.727 | |
AJCC N stage | |||||
N0 | Reference | Reference | |||
N1 | 0.805 (0.656–0.989) | 0.039 | 0.729 (0.483–1.100) | 0.132 | |
N2 | 1.042 (0.898–1.208) | 0.59 | 1.176 (0.891–1.551) | 0.253 | |
N3 | 0.770 (0.619–0.958) | 0.019 | 1.057 (0.663–1.685) | 0.817 | |
Primary site | |||||
Anterior wall of bladder | Reference | ||||
Bladder neck | 1.139 (0.802–1.618) | 0.467 | |||
Dome of bladder | 0.902 (0.629–1.292) | 0.574 | |||
Lateral wall of bladder | 1.090 (0.804–1.477) | 0.581 | |||
Overlapping lesion of bladder | 1.110 (0.826–1.492) | 0.49 | |||
Posterior wall of bladder | 1.124 (0.810–1.559) | 0.484 | |||
Trigone of bladder | 1.192 (0.854–1.663) | 0.301 | |||
Ureteric orifice | 1.334 (0.869–2.084) | 0.188 | |||
Surgery | |||||
No | Reference | ||||
LTE | 1.250 (0.980–1.593) | 0.072 | |||
PC | 1.325 (0.719–2.440) | 0.367 | |||
RC | 1.272 (0.905–1.788) | 0.166 | |||
Radiation | |||||
No | Reference | ||||
Yes | 1.013 (0.880–1.167) | 0.857 | |||
Chemotherapy | |||||
No | Reference | Reference | |||
Yes | 0.451 (0.399–0.509) | <0.001 | 0.330 (0.256–0.425) | <0.001 | |
Bone metastasis | |||||
No | Reference | Reference | |||
Yes | 1.388 (1.227–1.570) | <0.001 | 1.470 (1.147–1.883) | 0.002 | |
Brain metastasis | |||||
No | Reference | ||||
Yes | 1.456 (0.970–2.183) | 0.07 | |||
Liver metastasis | |||||
No | Reference | Reference | |||
Yes | 1.577 (1.354–1.836) | <0.001 | 1.778 (1.300–2.432) | <0.001 | |
Lung metastasis | |||||
No | Reference | Reference | |||
Yes | 1.226 (1.080–1.392) | 0.002 | 1.558 (1.224–1.983) | <0.001 |
MBC, metastatic bladder cancer; LTE, local tumor excision; PC, partial cystectomy; RC, radical cystectomy; HR, hazard ratios; CI, confidence intervals.
Risk nomogram construction and validation
With the results of multivariate logistic regression analysis, we constructed the final risk nomogram of bladder cancer, as shown in Figure 2. The probability of prediction can be obtained by summing up the scores obtained through the projection of each predictive factor. The AUC of the ROC analysis reached 0.80, demonstrating the excellent discriminatory ability of the risk nomogram (Figure 3A). The high overlap between the observed and predicted results in the calibration curve also showed the good reliability of the nomogram (Figure 3B). In the evaluation of clinical effect, both DCA and CIC curves showed that patients could get excellent clinical net benefit by this nomogram (Figure 3C,3D).
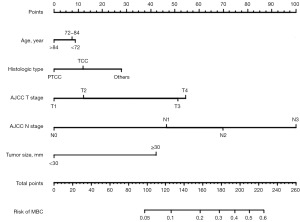
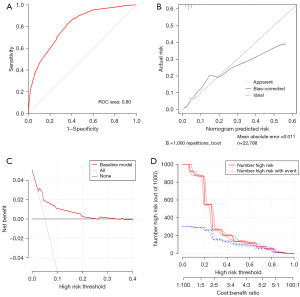
Prognostic nomogram construction and validation
Based on the results obtained from the multivariate cox regression analysis of the training cohort, we constructed the prognostic nomogram of MBC (Figure 4). The survival rate of prediction can be obtained by summing up the scores obtained through the projection of each predictive factor. The AUC of the ROC curve analysis of the nomogram revealed 1-, 2- and 3-year OS respectively reached 0.74, 0.71 and 0.71 in the training cohort (Figure 5A), 1-, 2- and 3-year OS respectively reached 0.81, 0.77 and 0.77 in the validation cohort (Figure 5B). The calibration curve of nomogram revealed an excellent consistency between actual observation and prediction both training cohort and validation cohort (Figure 5C-5H). As shown in Figure 6A-6C, the nomogram demonstrated a significant net benefit of 1-, 2- and 3-year OS, indicating its great clinical practical value in predicting OS of MBC in training cohort, the same results were also shown in validation cohort (Figure 6D-6F). Similarly, the prognostic risk factors by nomogram showed differences in the prognosis of all patients with MBC, training cohort and validation cohort in the KM survival analysis, further validated the clinical utility of the prognostic nomogram (Figure 7A-7C).
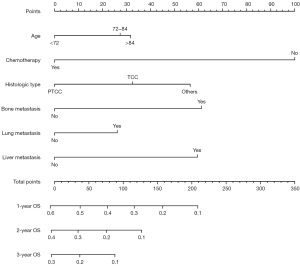
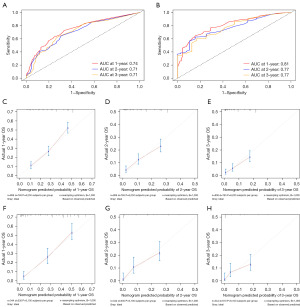
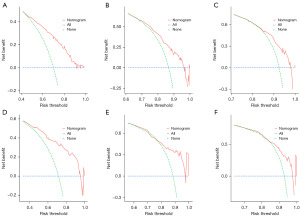
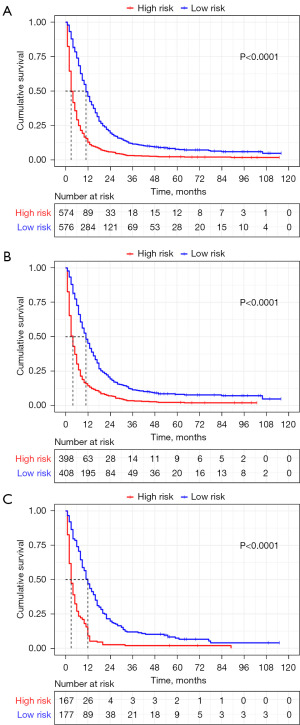
Discussion
As a highly heterogeneous disease, bladder cancer is mainly classified into non-muscle invasive bladder cancer (NMIBC), muscle invasive bladder cancer (MIBC) and MBC, with different subtypes also imply different clinical outcomes (13). The main treatment for patients with MIBC is neoadjuvant therapy followed by radical cystectomy, and urinary diversion, or a bladder-sparing protocol, such as chemoradiation or partial cystectomy (14). Treatment of MIBC primarily aims at preventing local recurrence (15). Even so, approximately half of the postoperative patients still experience distant metastasis (16,17), necessitating more management and monitoring measures for the treatment of MBC patients and those who experience metastasis. There are currently prognostic nomograms constructed based on bone metastasis (8) and brain metastasis, but comprehensive nomograms constructed based on clinical information may lead to better management of MBC patients. We screened 22,788 patients with bladder cancer from the SEER database, including 1,150 patients with MBC, independent clinical risk factors related to risk and prognosis were identified by logistic and cox regression analyses, finally, age, histological type, tumor size, T stage, and N stage were considered as independent risk factors for MBC, while age, histological type, chemotherapy, bone, liver and lung metastases were independent risk factors for the prognosis of MBC; ROC, CIC and DCA curves, etc. showed the powerful predictive ability and clinical application ability of the prediction model, which enable doctors to provide better clinical consultation and follow-up strategies for patients in clinical practice.
In all patients with bladder cancer, especially MIBC, the possibility of lymph node metastasis is very high (18), and it means a worse prognosis. Although the exact surgical procedure for lymphadenectomy is controversial (19), there is no doubt that Lymphadenectomy can be beneficial to patients. It is very important to evaluate the patient’s lymph node metastasis status by imaging methods before surgery (20), however, a quarter of patients are still found with lymph node metastasis after undergoing surgery (21). Therefore, lymph node metastasis is critical for treatment assessment, and in our study, lymph node staging had the highest score for assessing patients’ risk of metastasis. In addition, histological type, T stage, and tumor size also play a role in predicting the risk of metastasis.
Unfortunately, bladder cancer is diagnosed at an older age than other types of cancer (22). Most elderly patients pose a substantial challenge to treatment because of the high incidence of complications and frail status. With the aging of society, the number of patients with bladder cancer will also increase and it is noteworthy that cardiovascular disease is a common illness among elderly patients, however a study found that cardiovascular disease was an independent protective factor for bladder cancer, but this effect was not observed in high-risk tumors (23), therefore, for such high risk tumor, to construct an effective assessment tool can help physicians make better decisions (24). In our analysis, the best cut-off value of age was obtained by x-tile software and age acted as the predictive factor of prognosis in patients with MBC. It showed the association of older age with worse prognosis. Therefore, geriatric assessment of elderly patients is recommended as a practice, but it has not been validated on a large-scale study and we were unable to obtain relevant information from the SEER database. Similarly, in the study of metastatic patterns of MBC (25), the largest proportion of patients with bone metastases and the poorest prognosis of patients with liver metastases were observed, and few patients with brain metastases were not included in our model to assess the prognosis of MBC.
The prognosis for patients with untreated bladder cancer is very poor, with low survival rates even at 1 year (26), in a multicenter study of bladder carcinoma in situ, 70 years old was used as cut-off value, it was found that patients over 70 years old had an increased risk of recurrence and progression with a poor recurrence free survival (27), but in our study, multivariate logistic regression analysis showed that patients over 84 years old had a reduced risk of metastasis, but multivariate cox regression analysis showed that with increasing age, OS became worsened. According to treatment guidelines, the first-line therapy for MBC is cisplatin-based cytotoxic chemotherapy (28). Vinflunine is the only approved second-line therapy drug in Europe (29), in addition, second-line immunotherapy with programmed cell death protein 1/programmed cell death-ligand 1 (PD-1/PD-L1) checkpoint inhibitors has also been established (30). In general, chemotherapy has brought some benefits to the survival of patients with bladder cancer (31), however, the proportion of patients without chemotherapy in our study population with MBC reached 76.6%, which also had the most significant impact on prognosis, such a low chemotherapy rate also means a worse prognosis for these patients, which is consistent with the above results.
In conclusion, our nomograms are constructed based on clinical information, which have the convenience for clinical application, and their differentiation and validity have been verified by ROC, DCA and other curves, which can provide better reference for patients by judging the risk of metastasis and prognosis. Inevitably, our study has certain limitations. Firstly, this was a retrospective study, excluded many cases with some missing data, which may cause bias. Secondly, there are also clinical factors such as laboratory test results affecting the prognosis of MBC patients, in addition, some diagnostic tools based on artificial intelligence (AI) technology that have shown perfect results in cystoscopy, urine testing, and imaging analysis can be used for MBC patients (32), unfortunately, these data are not available in the SEER database. Thirdly, the efficacy of nomogram has only been internally validated, and we need more data of external clinical application to test its performance in the future.
Conclusions
In summary, we constructed risk and prognosis nomograms for MBC patients based on clinical risk factors and demonstrated their perfect utility through multiple validations. It could be used to provide counseling recommendations for patients and follow-up guidance for clinicians.
Acknowledgments
Funding: This study was supported by
Footnote
Reporting Checklist: The authors have completed the TRIPOD reporting checklist. Available at https://tcr.amegroups.com/article/view/10.21037/tcr-23-1229/rc
Peer Review File: Available at https://tcr.amegroups.com/article/view/10.21037/tcr-23-1229/prf
Conflicts of Interest: All authors have completed the ICMJE uniform disclosure form (available at https://tcr.amegroups.com/article/view/10.21037/tcr-23-1229/coif). The authors have no conflicts of interest to declare.
Ethical Statement: The authors are accountable for all aspects of the work in ensuring that questions related to the accuracy or integrity of any part of the work are appropriately investigated and resolved. The study was conducted in accordance with the Declaration of Helsinki (as revised in 2013). The SEER Program has a strict policy to protect patient privacy, and all data were de-identified prior to analysis. The ethical approval of this study was exempted by the Ethics Committee of the First Affiliated Hospital of Nanchang University as the data were from the publicly accessible database, SEER. No informed consent was required for this retrospective study.
Open Access Statement: This is an Open Access article distributed in accordance with the Creative Commons Attribution-NonCommercial-NoDerivs 4.0 International License (CC BY-NC-ND 4.0), which permits the non-commercial replication and distribution of the article with the strict proviso that no changes or edits are made and the original work is properly cited (including links to both the formal publication through the relevant DOI and the license). See: https://creativecommons.org/licenses/by-nc-nd/4.0/.
References
- Sung H, Ferlay J, Siegel RL, et al. Global Cancer Statistics 2020: GLOBOCAN Estimates of Incidence and Mortality Worldwide for 36 Cancers in 185 Countries. CA Cancer J Clin 2021;71:209-49. [Crossref] [PubMed]
- Cumberbatch MG, Rota M, Catto JW, et al. The Role of Tobacco Smoke in Bladder and Kidney Carcinogenesis: A Comparison of Exposures and Meta-analysis of Incidence and Mortality Risks. Eur Urol 2016;70:458-66. [Crossref] [PubMed]
- Lenis AT, Lec PM, Chamie K, et al. Bladder Cancer: A Review. JAMA 2020;324:1980-91. [Crossref] [PubMed]
- Tsai TH, Su PJ, Huang SY, et al. The prognostic significance of histologic variant on survival outcomes in patients with metastatic urothelial carcinoma receiving immune checkpoint inhibitor therapy. BMC Cancer 2023;23:871. [Crossref] [PubMed]
- Liu QL, Luo M, Huang C, et al. Epigenetic Regulation of Epithelial to Mesenchymal Transition in the Cancer Metastatic Cascade: Implications for Cancer Therapy. Front Oncol 2021;11:657546. [Crossref] [PubMed]
- Yao Z, Zheng Z, Ke W, et al. Prognostic nomogram for bladder cancer with brain metastases: a National Cancer Database analysis. J Transl Med 2019;17:411. [Crossref] [PubMed]
- Bouchelouche K. PET/CT in Bladder Cancer: An Update. Semin Nucl Med 2022;52:475-85. [Crossref] [PubMed]
- Fan Z, Huang Z, Hu C, et al. Risk factors and nomogram for newly diagnosis of bone metastasis in bladder cancer: A SEER-based study. Medicine (Baltimore) 2020;99:e22675. [Crossref] [PubMed]
- Lei Z, Li J, Wu D, et al. Nomogram for Preoperative Estimation of Microvascular Invasion Risk in Hepatitis B Virus-Related Hepatocellular Carcinoma Within the Milan Criteria. JAMA Surg 2016;151:356-63. [Crossref] [PubMed]
- Verbakel JY, Steyerberg EW, Uno H, et al. ROC curves for clinical prediction models part 1. ROC plots showed no added value above the AUC when evaluating the performance of clinical prediction models. J Clin Epidemiol 2020;126:207-16. [Crossref] [PubMed]
- Kandi S, Savaryn JP, Ji QC, et al. Use of in-sample calibration curve approach for quantification of peptides with high-resolution mass spectrometry. Rapid Commun Mass Spectrom 2022;36:e9377. [Crossref] [PubMed]
- Vickers AJ, Holland F. Decision curve analysis to evaluate the clinical benefit of prediction models. Spine J 2021;21:1643-8. [Crossref] [PubMed]
- Kirkali Z, Chan T, Manoharan M, et al. Bladder cancer: epidemiology, staging and grading, and diagnosis. Urology 2005;66:4-34. [Crossref] [PubMed]
- Patel VG, Oh WK, Galsky MD. Treatment of muscle-invasive and advanced bladder cancer in 2020. CA Cancer J Clin 2020;70:404-23. [Crossref] [PubMed]
- Nadal R, Bellmunt J. Management of metastatic bladder cancer. Cancer Treat Rev 2019;76:10-21. [Crossref] [PubMed]
- Adjuvant chemotherapy in invasive bladder cancer: a systematic review and meta-analysis of individual patient data Advanced Bladder Cancer (ABC) Meta-analysis Collaboration. Eur Urol 2005;48:189-199. [Crossref] [PubMed]
- Stein JP, Lieskovsky G, Cote R, et al. Radical cystectomy in the treatment of invasive bladder cancer: long-term results in 1,054 patients. J Clin Oncol 2001;19:666-75. [Crossref] [PubMed]
- Del Bene G, Calabrò F, Giannarelli D, et al. Neoadjuvant vs. Adjuvant Chemotherapy in Muscle Invasive Bladder Cancer (MIBC): Analysis From the RISC Database. Front Oncol 2018;8:463. [Crossref] [PubMed]
- Gschwend JE, Heck MM, Lehmann J, et al. Extended Versus Limited Lymph Node Dissection in Bladder Cancer Patients Undergoing Radical Cystectomy: Survival Results from a Prospective, Randomized Trial. Eur Urol 2019;75:604-11. [Crossref] [PubMed]
- Mirmomen SM, Shinagare AB, Williams KE, et al. Preoperative imaging for locoregional staging of bladder cancer. Abdom Radiol (NY) 2019;44:3843-57. [Crossref] [PubMed]
- Meeks JJ, Bellmunt J, Bochner BH, et al. A systematic review of neoadjuvant and adjuvant chemotherapy for muscle-invasive bladder cancer. Eur Urol 2012;62:523-33. [Crossref] [PubMed]
- VanderWalde NA, Chi MT, Hurria A, et al. Treatment of muscle invasive bladder cancer in the elderly: navigating the trade-offs of risk and benefit. World J Urol 2016;34:3-11. [Crossref] [PubMed]
- Barone B, Finati M, Cinelli F, et al. Bladder Cancer and Risk Factors: Data from a Multi-Institutional Long-Term Analysis on Cardiovascular Disease and Cancer Incidence. J Pers Med 2023;13:512. [Crossref] [PubMed]
- Hatakeyama S, Narita S, Okita K, et al. Management of bladder cancer in older patients. Jpn J Clin Oncol 2022;52:203-13. [Crossref] [PubMed]
- Shou J, Zhang Q, Zhang D. The prognostic effect of metastasis patterns on overall survival in patients with distant metastatic bladder cancer: a SEER population-based analysis. World J Urol 2021;39:4151-8. [Crossref] [PubMed]
- Dobruch J, Oszczudłowski M. Bladder Cancer: Current Challenges and Future Directions. Medicina (Kaunas) 2021;57:749. [Crossref] [PubMed]
- Ferro M, Chiujdea S, Musi G, et al. Impact of Age on Outcomes of Patients With Pure Carcinoma In Situ of the Bladder: Multi-Institutional Cohort Analysis. Clin Genitourin Cancer 2022;20:e166-72. [Crossref] [PubMed]
- Bellmunt J, Petrylak DP. New therapeutic challenges in advanced bladder cancer. Semin Oncol 2012;39:598-607. [Crossref] [PubMed]
- Bellmunt J, Théodore C, Demkov T, et al. Phase III trial of vinflunine plus best supportive care compared with best supportive care alone after a platinum-containing regimen in patients with advanced transitional cell carcinoma of the urothelial tract. J Clin Oncol 2009;27:4454-61. [Crossref] [PubMed]
- Bellmunt J, de Wit R, Vaughn DJ, et al. Pembrolizumab as Second-Line Therapy for Advanced Urothelial Carcinoma. N Engl J Med 2017;376:1015-26. [Crossref] [PubMed]
- Cathomas R, Lorch A, Bruins HM, et al. The 2021 Updated European Association of Urology Guidelines on Metastatic Urothelial Carcinoma. Eur Urol 2022;81:95-103. [Crossref] [PubMed]
- Ferro M, Falagario UG, Barone B, et al. Artificial Intelligence in the Advanced Diagnosis of Bladder Cancer-Comprehensive Literature Review and Future Advancement. Diagnostics (Basel) 2023;13:2308. [Crossref] [PubMed]