Noval ceRNA axis-mediated high expression of TOP2A correlates with poor prognosis and tumor immune infiltration of hepatocellular carcinoma
Highlight box
Key findings
• Three long non-coding RNAs (lncRNAs) increased TOP2A in hepatocellular carcinoma (HCC) through a competing endogenous RNA (ceRNA) mechanism, resulting in poorer outcomes for patients. In addition, TOP2A also affected tumor-related immunity, leading to HCC progression. A nomogram composed of these lncRNAs and clinicopathological features accurately predicted patient prognosis.
What is known and what is new?
• Multiple lncRNAs can influence the activity of HCC through a ceRNA mechanism.
• LncRNAs that promoted TOP2A in HCC were newly discovered. A nomogram composed of three lncRNAs and clinicopathological factors was constructed for the first time, and its predictive ability was tested.
What is the implication, and what should change now?
• This study provides multiple layers of evidence for the role and regulatory mechanisms of TOP2A in HCC, pointing to its potential as a target for HCC treatment in the future.
Introduction
Hepatocellular carcinoma (HCC) is the predominant primary malignancy of the liver and represents a global health burden. With an estimated global incidence ranking HCC as the sixth most common cancer, it is also the third most prevalent cause of cancer-related mortality (1). HCC can manifest as a result of various etiological factors (2), including liver cirrhosis (3), hepatitis B (4) and C (5) viruses, alcohol consumption (6), non-alcoholic fatty liver disease (7), and autoimmunity (8). Despite the availability of several treatment modalities for advanced stage HCC, such as transarterial chemotherapy (9), targeted therapy (10), and immunotherapy (11,12), the overall prognosis remains suboptimal. Therefore, identifying novel therapeutic targets and diagnostic biomarkers is crucial to address this unmet clinical need.
DNA topoisomerase family enzymes are widely distributed in the animal kingdom and play an essential role in modulating DNA topology. These enzymes serve as important therapeutic targets for anticancer drugs (13). Among them, human DNA topoisomerase II, which consists of α (TOP2A) and β (TOP2B) isoforms, plays a vital role in regulating DNA topology during replication, transcription, and chromosome organization and segregation (14). TOP2A is involved in crucial functions, such as chromosome condensation, chromatid separation, and alleviation of torsional stress during transcription and replication (15). TOP2A is prominently expressed during mitosis, and its activity is necessary for cell division, rendering it a biomarker for cell proliferation (15). A growing body of evidence suggests that TOP2A transcript levels are increased in multiple cancer types, including lung (16), stomach (17), breast (18), pancreatic (19) cancer, and HCC, indicating a correlation with disease progression and poor prognosis. Notably, high TOP2A expression levels have been identified in HCC and are associated with recurrence after radical hepatectomy (20).
Tumor-infiltrating immune cells (TIICs) serve as a crucial biomarker of the host’s antitumor immunity, and they are a critical component of the tumor immune microenvironment, along with tumor cells, stromal cells, and extracellular components (21). The role of TIICs in immunotherapy is of particular interest, as they play a pivotal role in mediating responses to cancer immunotherapy. The immune checkpoint protein programmed death 1 (PD-1), which is expressed in TIICs, is a vital target for immunotherapy (22) and has a high safety profile. In the latest research, another immune checkpoint, cytotoxic T-lymphocyte-associated protein 4 (CTLA-4), has been found to interfere with important TIIC-Treg cells by blocking the transmission of CD28 signals, thereby enhancing anti-tumor immunity (23). Despite the significant interest in TIICs, to date, there have been no systematic studies exploring the correlation between TOP2A and TIICs, particularly in the context of HCC.
Recent studies have highlighted the importance of competing endogenous RNA (ceRNA) in regulating gene expression at the post-transcriptional level by competing for shared microRNAs (miRNAs) (24). This hypothesis has been supported by growing experimental evidence, indicating that multiple non-coding RNA transcripts, such as pseudogenes (25), long non-coding RNAs (lncRNAs) (26), and circular RNAs (circRNAs) (27), can function as ceRNAs. Acting as a sponge, ceRNAs can adsorb miRNAs, thereby blocking their interaction with target mRNAs and increasing mRNA expression (28). This mechanism has been found to activate numerous oncogenes, including those implicated in HCC. Among these, lncRNA has been identified as a crucial ceRNA, and its upregulation has been associated with multiple oncogenic processes, promoting the activation of oncogenes. A variety of ceRNAs have been identified in HCC, suggesting their involvement in the development of this carcinoma (29,30).
We employed bioinformatics techniques and utilized data retrieved from online databases for the design of this research. Our study represents the first comprehensive investigation of TOP2A expression and copy number variation in various human cancers, with a particular focus on HCC. We also analyzed the potential regulatory mechanisms of TOP2A, including its relationship with miRNAs and lncRNAs acting as ceRNAs. Additionally, we explored the association between TOP2A expression and TIICs, immune cell biomarkers, and immune checkpoints in HCC. Our findings support the notion that lncRNAs hold promise as diagnostic markers for HCC, and a nomogram incorporating lncRNAs could aid in clinical decision-making. Ultimately, our study highlights the significance of ceRNA-mediated TOP2A upregulation in the context of HCC prognosis and immune cell infiltration. We present this article in accordance with the TRIPOD reporting checklist (available at https://tcr.amegroups.com/article/view/10.21037/tcr-23-755/rc).
Methods
Data preparation, differential expression and mutation analysis
The mRNA expression data of all 33 kinds of cancers in the The Cancer Genome Atlas (TCGA, https://www.cancer.gov/tcga) database and the corresponding normal tissue data in the Genotype-Tissue Expression (GTEx, https://gtexportal.org) database (31,32) were downloaded from UCSC XENA (https://xenabrowser.net/datapages/) (33), all of them were RNAseq data in TPM format which had been processed by the Toil process (34). TOP2A’s non-normal distribution across diverse tumor types necessitated the application of the Wilcoxon rank-sum test in R software (v3.6.3) for assessing differential expression against normal tissues. Visualization of results was achieved using the ggplot2 (35) R package. In HCC (TCGA-LIHC data), the somatic mutation of TOP2A was available from the cBioPortal database (http://www.cbioportal.org/) (36,37); R’s survminer package drawn the Kaplan-Meier (K-M) plot after the survival package had performed survival analysis on TOP2A; HPA (http://www.proteinatlas.org/) (38-40) searches were carried out to verify that TOP2A was expressed at the protein level. This study adhered to the principles outlined in the Helsinki Declaration (revised in 2013).
Upstream miRNA prediction and analysis
The Encyclopedia of RNA Interactomes (ENCORI, http://starbase.sysu.edu.cn/) is a website for miRNA-based interaction analysis (41). In the ENCORI, miRNA-mRNA target was used to predict upstream miRNAs relevant to TOP2A. We downloaded the list of miRNAs negatively associated with TOP2A in pan-cancer. The list showed the results of various prediction programs, including PITA, RNA22, miRmap, microT, miRanda, PicTar, and TargetScan. Only the predicted miRNA, which typically occured in more than three of the procedures mentioned above, was included in the subsequent analysis as candidate miRNAs of TOP2A. Relationship graph of candidate genes and TOP2A were made using Cytoscape software (v3.8.2) (42). The ggRadar package in R visualized a radar chart showing the correlation between miRNAs and TOP2A. We selected the miRNAs most negatively correlated with TOP2A as upstream miRNAs, and used ENCORI to graph differential expression in HCC and relevant diagrams. K-M plot of upstream miRNA in HCC was plotted in the K-M plotter database (http://kmplot.com/analysis/) (43).
Identification of ceRNAs
ENCORI mRNA-lncRNA module was used to screen upstream miRNA-associated lncRNAs, then relationship diagram was constructed. The lncRNAs that were positively associated with TOP2A were retained. On the basis of survival analysis in HCC, the filtered lncRNAs were assessed, and those found significant were selected as ceRNAs.
Immune cell infiltration and immune checkpoints analysis
TIMER database (http://timer.cistrome.org/) is a comprehensive tool for assessing immune infiltration in various tumor types (44,45). We evaluated immune cell infiltration and analytical immune checkpoint correlation with TOP2A. GEPIA2 (http://gepia2.cancer-pku.cn/) is a web application for cancer and normal gene-expression profiling and interactive analyses derived from TCGA and GTEx data (46). Afterward, we proceeded to analyze the correlation of markers of TIICs (47) and immune checkpoints with TOP2A by using GEPIA and TIMER. Lastly, R was applied to analyze the co-expression heatmap of TIICs and immune checkpoints in the TCGA-LIHC sample, the ssGSEA method of the GSVA package (48) was applied in order to investigate differences in TIIC infiltration between high and low TOP2A groups.
Diagnostic value analysis
K-M plots displayed the survival of three ceRNAs in overall survival (OS), disease-specific survival (DSS) and progression-free interval (PFI). Univariate COX regression of lncRNA and clinicopathological characteristics was performed. Multivariate Cox regression was conducted and nomogram was plotted for lncRNA and clinicopathological characteristics. The calibration graph was designed to measure the diagnostic efficiency of the Nomogram. Receiver operating characteristic (ROC) curves guided the determination of whether there was any significant difference between lncRNAs in liver tissue and HCC. The above analysis was performed with R and R packages (survminer, survival, pROC, rms).
Statistical analysis
The statistical analysis in this study was calculated automatically by R software and the online database mentioned above. P value <0.05 was considered as statistically significant.
Results
Tumorigenicity of TOP2A overexpression and prognostic value in HCC
Figure 1A shows that the expression of TOP2A analyzed in 33 different types of cancers from the TCGA database, and compared with the corresponding normal tissues. The results revealed that in 29 types of tumors, including adrenocortical cancer (ACC), bladder urothelial carcinoma (BLCA), breast invasive carcinoma (BRCA), cervical & endocervical cancer (CESC), cholangiocarcinoma (CHOL), colon adenocarcinoma (COAD), diffuse large B-cell lymphoma (DLBC), esophageal carcinoma (ESCA), glioblastoma multiforme (GBM), head & neck squamous cell carcinoma (HNSC), kidney chromophobe (KICH), kidney clear cell carcinoma (KIRC), kidney papillary cell carcinoma (KIRP), brain lower grade glioma (LGG), liver hepatocellular carcinoma (LIHC), lung adenocarcinoma (LUAD), lung squamous cell carcinoma (LUSC), ovarian serous cystadenocarcinoma (OV), pancreatic adenocarcinoma (PAAD), pheochromocytoma & paraganglioma (PCPG), prostate adenocarcinoma (PRAD), rectum adenocarcinoma (READ), skin cutaneous melanoma (SKCM), stomach adenocarcinoma (STAD), thyroid carcinoma (THCA), thymoma (THYM), uterine corpus endometrioid carcinoma (UCEC), and uterine carcinosarcoma (UCS), TOP2A was significantly increased, while in acute myeloid leukemia (LAML), it was markedly decreased. This suggests that TOP2A may have an oncogenic potential in several types of cancers.
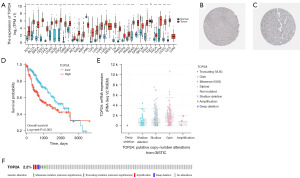
Initially, we examined the baseline characteristics of clinicopathological factors in HCC to determine the significance of differences in TOP2A expression (Table 1). We observed aberrant overexpression of TOP2A in tumor specimens, prompting us to investigate its expression in HCC. Immunohistochemical staining from the HPA database confirmed the dysregulation of TOP2A in HCC samples (Figure 1B,1C). Subsequently, we evaluated the association between TOP2A expression and OS in HCC using K-M curves, and found that high TOP2A expression was significantly linked to lower OS (Figure 1D).
Table 1
Characteristic | Levels | Low expression of TOP2A (n=187) | High expression of TOP2A (n=187) | P |
---|---|---|---|---|
T stage | T1 | 107 (28.8%) | 76 (20.5%) | 0.009 |
T2 | 40 (10.8%) | 55 (14.8%) | ||
T3 | 32 (8.6%) | 48 (12.9%) | ||
T4 | 5 (1.3%) | 8 (2.2%) | ||
Pathologic stage | Stage I | 101 (28.9%) | 72 (20.6%) | 0.004 |
Stage II | 39 (11.1%) | 48 (13.7%) | ||
Stage III | 32 (9.1%) | 53 (15.1%) | ||
Stage IV | 4 (1.1%) | 1 (0.3%) | ||
Tumor status | Tumor free | 113 (31.8%) | 89 (25.1%) | 0.016 |
With tumor | 65 (18.3%) | 88 (24.8%) | ||
Gender | Female | 52 (13.9%) | 69 (18.4%) | 0.077 |
Male | 135 (36.1%) | 118 (31.6%) | ||
Age | ≤60 | 77 (20.6%) | 100 (26.8%) | 0.020 |
>60 | 110 (29.5%) | 86 (23.1%) | ||
Residual tumor | R0 | 167 (48.4%) | 160 (46.4%) | 0.90 |
R1 | 8 (2.3%) | 9 (2.6%) | ||
R2 | 1 (0.3%) | 0 | ||
Child-Pugh grade | A | 119 (49.4%) | 100 (41.5%) | >0.99 |
B | 11 (4.6%) | 10 (4.1%) | ||
C | 1 (0.4%) | 0 | ||
Vascular invasion | No | 113 (35.5%) | 95 (29.9%) | 0.35 |
Yes | 53 (16.7%) | 57 (17.9%) |
In TCGA (The Cancer Genome Atlas) database, a subset of data is devoid of clinical-pathological information.
In order to understand the underlying mechanism behind the high expression of TOP2A in HCC, we conducted a genomic and copy number analysis. Using cBioportal, we found that TOP2A was primarily associated with gain, except for diploid, which suggests a correlation with its increased expression (Figure 1E). According to Oncoprint analysis, TOP2A in the HCC dataset was mainly associated with amplification, although the frequency of variation was only 2.2% (Figure 1F). Despite copy number amplification being a potential reason for high TOP2A expression in HCC, the low frequency of variation suggests that additional factors may be involved in regulating its expression.
Prediction and analysis of miRNAs upstream of TOP2A
It is widely acknowledged that miRNAs play a negative regulatory role in gene expression. To investigate whether certain miRNAs could inhibit TOP2A, we identified 12 miRNAs that bound to TOP2A upstream. We visualized the modulation network of these miRNAs with TOP2A (Figure 2A) and found that miR-139-5p exhibited the most significant negative correlation with TOP2A compared to other miRNAs, as shown in Figure 2B. To better visualize the differences between miR-139-5p and other miRNAs, we created a Radar Chart visualization (Figure 2C). Figure 2D shows that the miR-139-5p level was significantly reduced in HCC, and Figure 2E demonstrates a linear negative correlation between miR-139-5p and TOP2A. The K-M curves in Figure 2F suggest that low expression of miR-139-5p in HCC was associated with poorer OS. Therefore, based on the evidence, miR-139-5p is the most critical upstream miRNA.
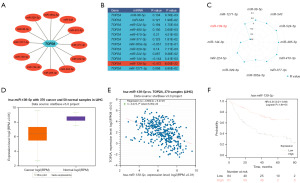
Prediction and analysis of ceRNAs associated with miR-139-5p
We utilized miRNA-lncRNA interactions to predict the 69 lncRNAs that had interacted with miR-139-5p and mapped out the regulatory network (Figure 3A). According to the ceRNA mechanism, lncRNAs act as ceRNAs by competitively binding miRNAs, leading to elevated mRNA expression. After analysis, we found that three lncRNAs, namely AC078846.1, AC124798.1, and SNHG3, were positively correlated with TOP2A (Figure 3B-3D). Further analysis revealed that the expression of these three lncRNAs was significantly higher in HCC than in adjacent non-tumor tissues (Figure 3E,3F). Additionally, K-M curves indicated that patients with high expression levels of these lncRNAs had a poorer OS compared to those with low expression levels (Figure 3G-3I). These findings suggest that AC078846.1, AC124798.1, and SNHG3 act as ceRNAs in the TOP2A pathway, and the TOP2A-miR-139-5p-AC078846.1/AC124798.1/SNHG3 axis plays a crucial role in the progression of HCC.
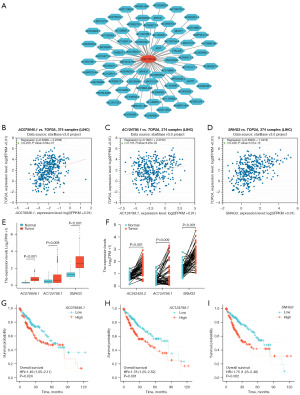
Relationship between TOP2A and TIICs
TIICs are closely associated with oncogenesis, development, and metastasis as components of the tumor microenvironment. As demonstrated in Figure 4A-4F, after purity adjustment, TOP2A was positively correlated with TIICs, including B cells, CD4+ T cells, CD8+ T cells, neutrophils, macrophages, and dendritic cells in HCC. Based on the difference in TOP2A expression, there were significant differences in CD4+ T cells, CD8+ T cells, neutrophils, and dendritic cell infiltration, B cells and macrophages were not associated with TOP2A expression (Figure 4G).
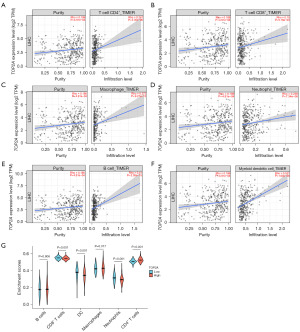
As a further way to explore the role of TOP2A in tumor immunity, we examined the correlation between TOP2A and the surface marker expression of TIICs. As displayed in Table S1, positive relationships were observed for CD8+ surface markers (CD8A, CD8B), M1 macrophage surface markers (IRF5), M2 macrophage surface markers (CD163, VSIG4, MS4A4A), neutrophil surface markers (ITGAM) and dendritic cell surface markers (HLA-DPB1, HLA-DQB1, HLA-DPA1, CD1C, NRP1, ITGAX), which partially proved the association with immune cell infiltration. Moreover, we compared TOP2A expression with surface markers of TIICs and created a heatmap (Figure S1A).
Relationship between TOP2A and immune checkpoints
The modification of immune checkpoints is a significant mechanism by which tumors escape from immune surveillance. Novel anti-cancer drugs include immune checkpoint inhibitors, and thus, we investigated the relationship between TOP2A and common immune checkpoints. Using TIMER, we identified a positive correlation between TOP2A and CD27 (TNFRSF7), CD274 (PD-L1), CTLA4, HAVCR2 (TIM-3), IDO1, LAG3, PDCD1 (PD-1), and PDCD1LG2 (PD-L2). However, these correlations are relatively weak, as depicted in Figure 5A-5H. Furthermore, there was no correlation between SIGLEC15 and TOP2A, as illustrated in Figure 5I.
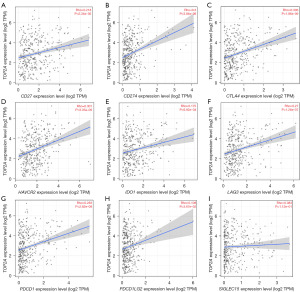
Table S2 obtained from GEPIA reveals significant positive correlations between TOP2A and several immune checkpoints, including PDCD1 (PD-1), CD274 (PD-L1), PDCD1LG2 (PD-L2), CTLA4, LAG3, and CD27 (TNFRSF7), as well as near positive correlations for HAVCR2 (TIM-3) and IDO1. Conversely, there was no significant correlation between TOP2A and SIGLEC15 expression. These results largely corroborate those shown in Figure 5. Moreover, we generated a heatmap that depicted the expression levels of TOP2A and immune checkpoints (Figure S1B).
Diagnostic value of ceRNAs and prediction of prognosis of HCC patients
Upon analyzing the effects of three lncRNAs, namely AC078846.1, AC124798.1, and SNHG3, on the survival of HCC patients, K-M curves demonstrated that patients with high expression of these lncRNAs had significantly lower OS (Figure 6A-6C) and DSS (Figure 6D-6F). However, the K-M curves of PFI showed that AC124798.1 had no effect, while AC078846.1 and SNHG3 had a significant effect (Figure 6G-6I).
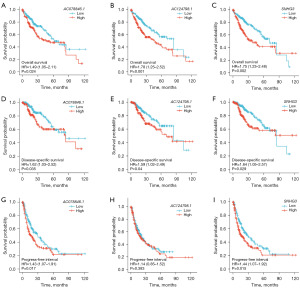
To identify factors that impact HCC patient outcomes, we conducted both univariate and multivariate COX regression analyses on both lncRNAs and clinicopathological indicators. The univariate regression analysis revealed that elevated expression levels of lncRNAs were associated with reduced OS (Table 2) and DSS (Table S3), and with reduced PFI, with the exception of AC124798.1 (Table S4), which corroborated the K-M curve results. Among the clinicopathological indicators, T stage, pathologic stage, and tumor status were associated with reduced OS (Table 2), while T stage, pathologic stage, and Child-Pugh grade were associated with poorer DSS (Table S3). Additionally, T stage, pathologic stage, tumor status, and vascular invasion were associated with reduced PFI (Table S4).
Table 2
Characteristics | Total (N) | Univariate analysis | Multivariate analysis | |||
---|---|---|---|---|---|---|
Hazard ratio (95% CI) | P value | Hazard ratio (95% CI) | P value | |||
T stage (T3 & T4 vs. T1 & T2) | 371 | 2.598 (1.826–3.697) | <0.001* | 1.411 (0.191–10.421) | 0.73 | |
Pathologic stage (stage III & stage IV vs. stage I & stage II) | 350 | 2.504 (1.727–3.631) | <0.001* | 1.432 (0.196–10.470) | 0.72 | |
Tumor status (with tumor vs. tumor free) | 355 | 2.317 (1.590–3.376) | <0.001* | 1.909 (1.269–2.870) | 0.002* | |
Gender (male vs. female) | 374 | 0.793 (0.557–1.130) | 0.20 | |||
Age (>60 vs. ≤60) | 373 | 1.205 (0.850–1.708) | 0.29 | |||
Residual tumor (R1 & R2 vs. R0) | 345 | 1.604 (0.812–3.169) | 0.17 | |||
Child-Pugh grade (B & C vs. A) | 241 | 1.643 (0.811–3.330) | 0.16 | |||
Vascular invasion (yes vs. no) | 318 | 1.344 (0.887–2.035) | 0.16 | |||
AC078846.1 (high vs. low) | 373 | 1.489 (1.053–2.106) | 0.024* | 1.210 (0.808–1.812) | 0.35 | |
AC124798.1 (high vs. low) | 373 | 1.776 (1.250–2.522) | 0.001* | 1.460 (0.970–2.198) | 0.07 | |
SNHG3 (high vs. low) | 373 | 1.748 (1.232–2.480) | 0.002* | 1.559 (1.023–2.376) | 0.03* |
*, statistically significant. HCC, hepatocellular carcinoma.
Multivariate regression analysis revealed that only SNHG3 was significantly associated with low OS (Table 2) and poor DSS (Table S3), while none of the lncRNAs were significantly associated with PFI (Table S4). In terms of clinical factors, tumor status and Child-Pugh grade were associated with low OS (Table 2), vascular invasion was linked to poor DSS (Table S3), and vascular invasion was linked to low PFI (Table S4).
A nomogram was developed to provide a quantitative method for predicting the prognosis of HCC patients by using three lncRNAs and clinicopathological indicators (Figure 7A). The best model was selected by screening variables using Forward selection. Each variable was assigned a score between 0 and 100, and the sum of all variable scores was considered as the final score. By intersecting the vertical line of the total score with the 1-, 2-, and 3-year predicted survival rates, the survival rates for HCC patients can be obtained. For instance, a patient with a high SNHG3 risk [70], T3 stage [57.5], Child-Pugh B [80], and tumor-free status [0] had a total score of 207.5 points and approximately 81%, 60%, and 52% survival rates at 1, 2, and 3 years, respectively. The c-index value of this line plot was 0.876 (95% CI: 0.856–0.897), indicating a relatively high predictive efficiency (Figure 7B).
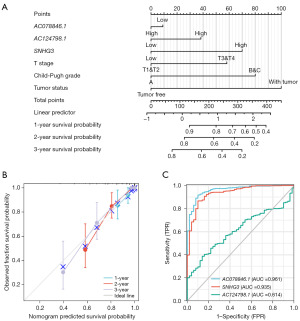
To assess the ability of the three lncRNAs to differentiate HCCs from normal liver tissue, a ROC analysis was performed (Figure 7C). The area under the curve (AUC) was calculated for each lncRNA, revealing an AUC of 0.961 for AC078846.1, 0.935 for SNHG3, and 0.614 for AC124798.1. Elevated expression of AC078846.1 and SNHG3 were associated with a higher probability of HCC.
Discussion
HCC is a leading malignancy globally (1,49) and poses significant challenges in treatment and healthcare costs (50). Despite advances in traditional and emerging therapies such as transarterial chemotherapy (9,51) and immune checkpoint inhibitors (11,12), the overall prognosis for HCC patients remains poor. Consequently, elucidating the mechanisms of HCC progression, identifying diagnostic biomarkers, discovering novel therapeutic targets, and investigating tumor-associated immunity continue to be the focal points for oncologists. As a widely recognized cancer driver, TOP2A has an important role in tumorigenesis, progression, and metastasis. Nonetheless, high-quality studies exploring the implications of TOP2A overexpression in HCC are lacking. Moreover, the relationship between TOP2A and immunity in HCC and its impact on ceRNA prediction is relatively unexplored. Additionally, ceRNA, a significant modulator of tumor growth, has not been extensively investigated in HCC. In this study, we attempted to correlate the tumorigenicity of TOP2A with immunity and ceRNA, then applied it to systematically predict the prognosis of HCC.
In our study, we conducted an initial analysis of the tumorigenicity of TOP2A. We first examined its expression in TCGA data and found that TOP2A was highly expressed in most tumors, consistent with previous research suggesting that it is a non-specific oncogene. As reported in prior studies, abnormal expression of TOP2A is known to promote cancer growth, metastasis, and chemoresistance by modulating DNA topology, making it a target for cancer therapy. This foundation enabled further examination of TOP2A expression in HCC and confirmed through immunohistochemistry of HPA that TOP2A was indeed highly expressed. We also examined the reasons for its high expression and copy number amplification and identified post-transcriptional regulation as a contributing factor, with the ceRNA mechanism playing a crucial role.
CeRNA has emerged as a significant epigenetic regulatory mechanism in tumor development and has become a recent focus of tumor research. The regulation of TOP2A expression by ceRNAs has been previously reported in other tumor types. However, this is the first study to report the ceRNA regulation of TOP2A expression in HCC. In this study, we identified miR-139-5p as a highly relevant miRNA for TOP2A, and based on the ceRNA hypothesis, three lncRNAs were found to be of the strongest correlations with miR-139-5p. Among these lncRNAs, SNHG3 has been previously reported to regulate HCC (52) and other tumors (53-55), indicating its potential as an important lncRNA with a wide range of regulatory effects on tumors. This study further validates its clinical potential. Two other lncRNAs, AC078846.1 and AC124798.1, have not been previously studied in HCC or other tumors. Our study is the first to identify the effects of these two lncRNAs on TOP2A expression in HCC. We found that increasing levels of these lncRNAs induced TOP2A expression, suggesting that they have epigenetic regulation of TOP2A. Furthermore, high expression of these three lncRNAs significantly impacted OS, DSS, and PFI in HCC patients, indicating a poor prognosis. These findings provide a basis for further studies and suggest that the TOP2A-miR-139-5p-AC078846.1/AC124798.1/SNHG3 axis is an important control mechanism for HCC development and prognosis.
Our study aimed to investigate the impact of TOP2A, an important oncogene, on tumor immunity in HCC. In recent years, tumor immunology has emerged as a promising frontier in cancer research (56), with immune checkpoint inhibitors showing great potential in the treatment of HCC (57,58). The therapeutic effect of immunotherapy is closely related to the quantity and type of TIICs and the expression of immune checkpoint molecules. Moreover, the predictive value of TIICs on the prognosis of cancer patients has also been reported. Our findings demonstrated that TOP2A expression was positively correlated with B cells, CD8+ T cells, CD4+ T cells, macrophages, neutrophils, and dendritic cells, which are the main TIICs. Furthermore, TOP2A was positively associated with the expression of most immune checkpoints, especially CTLA4 and LAG3, as shown by the results of TIMER and GEPIA analysis. These findings suggest that targeting CTLA4 and LAG3 may be a promising immunotherapy strategy for HCC patients with high TOP2A expression. In summary, our study highlights the impact of TOP2A on tumor immunity and suggests that further investigation is warranted to better understand its mechanism in HCC. Our results provide a theoretical basis for future clinical studies in immunotherapy for HCC.
Aside from that, this study investigated the value of ceRNAs in the diagnosis and prognosis of HCC patients, so that ceRNAs can exert a greater substantial clinical effect. The important clinicopathological factors in HCC and the three lncRNAs were subjected by COX regression to explore how that affects OS, DSS, PFI and other indicators. According to the results, patients with high levels of the three ceRNAs had a poorer OS and DSS, as well as clinicopathological factors such as T stage, pathologic stage, tumor status, Child-Pugh grade, and vascular invasion. All three lncRNAs may accelerate the progression of HCC and reduce patient survival. Due to the number of factors affecting OS, we chose DSS, which reflects tumor characteristics, and utilized three linear ncRNAs and clinicopathological indicators to produce nomogram in order to predict the likelihood of patient survival at 1, 2 and 3 years. Following screening, the six-factor nomogram, comprised of the T stage, Child-Pugh grade, Tumor status and three lncRNAs, had high predictive accuracy and practical predictive value for clinical purposes. A ROC curve was used to assess the diagnostic value of the three lncRNAs for HCC. AC078846.1 and SNHG3 exhibits excellent diagnostic value.
Furthermore, the other aim of this research was to investigate the value of ceRNAs in the diagnosis and prognosis of HCC patients, in order to enhance their clinical impact. By subjecting the three lncRNAs and important clinicopathological factors in HCC to COX regression, the study explored their impact on OS, DSS, PFI, and other indicators. The results indicated that patients with high levels of the three lncRNAs had poorer OS and DSS, as well as clinicopathological factors such as T stage, pathologic stage, tumor status, Child-Pugh grade, and vascular invasion. It was also observed that all three lncRNAs may accelerate the progression of HCC and reduce patient survival. In order to prognosticate the likelihood of patient survival at 1, 2, and 3 years, given the multifactorial influences on OS, we opted for DSS, which embodies the tumor’s hallmark traits, and constructed a nomogram incorporating three lncRNAs and clinicopathological indicators. The six-factor nomogram, consisting of the T stage, Child-Pugh grade, Tumor status, and three lncRNAs, was found to have high predictive accuracy and practical value for clinical purposes. Additionally, the diagnostic value of the three lncRNAs for HCC was assessed using a ROC curve, and AC078846.1 and SNHG3 were found to exhibit excellent diagnostic value.
Based on the above, our study investigated the expression of TOP2A in various human cancers, with a particular focus on HCC. Our analysis revealed that high expression of TOP2A in HCC is associated with poor prognosis. We also explored the mechanisms underlying TOP2A upregulation, and identified an important ceRNA regulatory axis involving TOP2A-miR-139-5p-AC078846.1/AC124798.1/SNHG3. Of note, AC078846.1 and AC124798.1 represent the first reported involvement in HCC. We further demonstrated that the tumor immunity mediated by TOP2A can affect immune checkpoints and immune cells. Finally, our nomograms based on lncRNAs and clinicopathological factors have practical implications for assessing the survival of HCC patients, as well as uncovering the clinical significance of lncRNAs.
Conclusions
In summary, this work provides evidence that three lncRNAs promote the upregulation of TOP2A in HCC, leading to adverse outcomes in patients. A nomogram incorporating these three lncRNAs and clinicopathological features also accurately predicts patient prognosis. All analyses highlight the significance of these lncRNAs.
Acknowledgments
Funding: None.
Footnote
Reporting Checklist: The authors have completed the TRIPOD reporting checklist. Available at https://tcr.amegroups.com/article/view/10.21037/tcr-23-755/rc
Peer Review File: Available at https://tcr.amegroups.com/article/view/10.21037/tcr-23-755/prf
Conflicts of Interest: All authors have completed the ICMJE uniform disclosure form (available at https://tcr.amegroups.com/article/view/10.21037/tcr-23-755/coif). The authors have no conflicts of interest to declare.
Ethical Statement: The authors are accountable for all aspects of the work in ensuring that questions related to the accuracy or integrity of any part of the work are appropriately investigated and resolved. The study was conducted in accordance with the Declaration of Helsinki (as revised in 2013).
Open Access Statement: This is an Open Access article distributed in accordance with the Creative Commons Attribution-NonCommercial-NoDerivs 4.0 International License (CC BY-NC-ND 4.0), which permits the non-commercial replication and distribution of the article with the strict proviso that no changes or edits are made and the original work is properly cited (including links to both the formal publication through the relevant DOI and the license). See: https://creativecommons.org/licenses/by-nc-nd/4.0/.
References
- Sung H, Ferlay J, Siegel RL, et al. Global Cancer Statistics 2020: GLOBOCAN Estimates of Incidence and Mortality Worldwide for 36 Cancers in 185 Countries. CA Cancer J Clin 2021;71:209-49. [Crossref] [PubMed]
- Llovet JM, Kelley RK, Villanueva A, et al. Hepatocellular carcinoma. Nat Rev Dis Primers 2021;7:6. [Crossref] [PubMed]
- Garrido A, Djouder N. Cirrhosis: A Questioned Risk Factor for Hepatocellular Carcinoma. Trends Cancer 2021;7:29-36. [Crossref] [PubMed]
- Mak LY, Wong DK, Pollicino T, et al. Occult hepatitis B infection and hepatocellular carcinoma: Epidemiology, virology, hepatocarcinogenesis and clinical significance. J Hepatol 2020;73:952-64. [Crossref] [PubMed]
- Baumert TF, Hoshida Y. Addressing the Challenges of Hepatitis C Cure and Persistent Risk of Hepatocellular Carcinoma. Viruses 2019;11:441. [Crossref] [PubMed]
- Huang DQ, Tan DJH, Ng CH, et al. Hepatocellular Carcinoma Incidence in Alcohol-Associated Cirrhosis: Systematic Review and Meta-analysis. Clin Gastroenterol Hepatol 2023;21:1169-77. [Crossref] [PubMed]
- Degasperi E, Colombo M. Distinctive features of hepatocellular carcinoma in non-alcoholic fatty liver disease. Lancet Gastroenterol Hepatol 2016;1:156-64. [Crossref] [PubMed]
- Tansel A, Katz LH, El-Serag HB, et al. Incidence and Determinants of Hepatocellular Carcinoma in Autoimmune Hepatitis: A Systematic Review and Meta-analysis. Clin Gastroenterol Hepatol 2017;15:1207-1217.e4. [Crossref] [PubMed]
- Li QJ, He MK, Chen HW, et al. Hepatic Arterial Infusion of Oxaliplatin, Fluorouracil, and Leucovorin Versus Transarterial Chemoembolization for Large Hepatocellular Carcinoma: A Randomized Phase III Trial. J Clin Oncol 2022;40:150-60. [Crossref] [PubMed]
- Kudo M, Finn RS, Qin S, et al. Lenvatinib versus sorafenib in first-line treatment of patients with unresectable hepatocellular carcinoma: a randomised phase 3 non-inferiority trial. Lancet 2018;391:1163-73. [Crossref] [PubMed]
- Finn RS, Qin S, Ikeda M, et al. Atezolizumab plus Bevacizumab in Unresectable Hepatocellular Carcinoma. N Engl J Med 2020;382:1894-905. [Crossref] [PubMed]
- Ren Z, Xu J, Bai Y, et al. Sintilimab plus a bevacizumab biosimilar (IBI305) versus sorafenib in unresectable hepatocellular carcinoma (ORIENT-32): a randomised, open-label, phase 2-3 study. Lancet Oncol 2021;22:977-90. [Crossref] [PubMed]
- Fathi-Karkan S, Arshad R, Rahdar A, et al. Recent advancements in the targeted delivery of etoposide nanomedicine for cancer therapy: A comprehensive review. Eur J Med Chem 2023;259:115676. [Crossref] [PubMed]
- Szlachta K, Manukyan A, Raimer HM, et al. Topoisomerase II contributes to DNA secondary structure-mediated double-stranded breaks. Nucleic Acids Res 2020;48:6654-71. [Crossref] [PubMed]
- Wu CC, Li TK, Farh L, et al. Structural basis of type II topoisomerase inhibition by the anticancer drug etoposide. Science 2011;333:459-62. [Crossref] [PubMed]
- Kou F, Sun H, Wu L, et al. TOP2A Promotes Lung Adenocarcinoma Cells' Malignant Progression and Predicts Poor Prognosis in Lung Adenocarcinoma. J Cancer 2020;11:2496-508. [Crossref] [PubMed]
- Cui Y, Pu R, Ye J, et al. LncRNA FAM230B Promotes Gastric Cancer Growth and Metastasis by Regulating the miR-27a-5p/TOP2A Axis. Dig Dis Sci 2021;66:2637-50. [Crossref] [PubMed]
- Hajibabaei S, Nafissi N, Azimi Y, et al. Targeting long non-coding RNA MALAT1 reverses cancerous phenotypes of breast cancer cells through microRNA-561-3p/TOP2A axis. Sci Rep 2023;13:8652. [Crossref] [PubMed]
- Liu SL, Cai C, Yang ZY, et al. DGCR5 is activated by PAX5 and promotes pancreatic cancer via targeting miR-3163/TOP2A and activating Wnt/β-catenin pathway. Int J Biol Sci 2021;17:498-513. [Crossref] [PubMed]
- Cai H, Zhu X, Qian F, et al. High expression of TOP2A gene predicted poor prognosis of hepatocellular carcinoma after radical hepatectomy. Transl Cancer Res 2020;9:983-92. [Crossref] [PubMed]
- Schürch CM, Bhate SS, Barlow GL, et al. Coordinated Cellular Neighborhoods Orchestrate Antitumoral Immunity at the Colorectal Cancer Invasive Front. Cell 2020;182:1341-1359.e19. [Crossref] [PubMed]
- Tang J, Yu JX, Hubbard-Lucey VM, et al. Trial watch: The clinical trial landscape for PD1/PDL1 immune checkpoint inhibitors. Nat Rev Drug Discov. 2018;17:854-5. [Crossref] [PubMed]
- Zappasodi R, Serganova I, Cohen IJ, et al. CTLA-4 blockade drives loss of T(reg) stability in glycolysis-low tumours. Nature 2021;591:652-8. [Crossref] [PubMed]
- Karreth FA, Pandolfi PP. ceRNA cross-talk in cancer: when ce-bling rivalries go awry. Cancer Discov 2013;3:1113-21. [Crossref] [PubMed]
- Lou W, Ding B, Fu P. Pseudogene-Derived lncRNAs and Their miRNA Sponging Mechanism in Human Cancer. Front Cell Dev Biol 2020;8:85. [Crossref] [PubMed]
- Zhan DT, Xian HC. Exploring the regulatory role of lncRNA in cancer immunity. Front Oncol 2023;13:1191913. [Crossref] [PubMed]
- Zhang F, Jiang J, Qian H, et al. Exosomal circRNA: emerging insights into cancer progression and clinical application potential. J Hematol Oncol 2023;16:67. [Crossref] [PubMed]
- Tay Y, Rinn J, Pandolfi PP. The multilayered complexity of ceRNA crosstalk and competition. Nature 2014;505:344-52. [Crossref] [PubMed]
- Lu L, Li S, Zhang Y, et al. GFI1-Mediated Upregulation of LINC00675 as a ceRNA Restrains Hepatocellular Carcinoma Metastasis by Sponging miR-942-5p. Front Oncol 2021;10:607593. [Crossref] [PubMed]
- Sun JR, Kong CF, Xiao KM, et al. Integrated Analysis of lncRNA-Mediated ceRNA Network Reveals a Prognostic Signature for Hepatocellular Carcinoma. Front Genet 2020;11:602542. [Crossref] [PubMed]
- The Genotype-Tissue Expression (GTEx) project. Nat Genet 2013;45:580-5. [Crossref] [PubMed]
- Human genomics. The Genotype-Tissue Expression (GTEx) pilot analysis: multitissue gene regulation in humans. Science 2015;348:648-60. [Crossref] [PubMed]
- Goldman MJ, Craft B, Hastie M, et al. Visualizing and interpreting cancer genomics data via the Xena platform. Nat Biotechnol 2020;38:675-8. [Crossref] [PubMed]
- Vivian J, Rao AA, Nothaft FA, et al. Toil enables reproducible, open source, big biomedical data analyses. Nat Biotechnol 2017;35:314-6. [Crossref] [PubMed]
- Wickham H. ggplot2: Elegant Graphics for Data Analysis [Internet]. Springer-Verlag New York; 2016. Available online: https://ggplot2.tidyverse.org
- Gao J, Aksoy BA, Dogrusoz U, et al. Integrative analysis of complex cancer genomics and clinical profiles using the cBioPortal. Sci Signal 2013;6:pl1. [Crossref] [PubMed]
- Cerami E, Gao J, Dogrusoz U, et al. The cBio cancer genomics portal: an open platform for exploring multidimensional cancer genomics data. Cancer Discov 2012;2:401-4. [Crossref] [PubMed]
- Uhlen M, Zhang C, Lee S, et al. A pathology atlas of the human cancer transcriptome. Science 2017;357:eaan2507. [Crossref] [PubMed]
- Thul PJ, Åkesson L, Wiking M, et al. A subcellular map of the human proteome. Science 2017;356:eaal3321. [Crossref] [PubMed]
- Uhlén M, Fagerberg L, Hallström BM, et al. Proteomics. Tissue-based map of the human proteome. Science 2015;347:1260419. [Crossref] [PubMed]
- Li JH, Liu S, Zhou H, et al. starBase v2.0: decoding miRNA-ceRNA, miRNA-ncRNA and protein-RNA interaction networks from large-scale CLIP-Seq data. Nucleic Acids Res 2014;42:D92-7. [Crossref] [PubMed]
- Shannon P, Markiel A, Ozier O, et al. Cytoscape: a software environment for integrated models of biomolecular interaction networks. Genome Res 2003;13:2498-504. [Crossref] [PubMed]
- Győrffy B. Survival analysis across the entire transcriptome identifies biomarkers with the highest prognostic power in breast cancer. Comput Struct Biotechnol J 2021;19:4101-9. [Crossref] [PubMed]
- Li T, Fan J, Wang B, et al. TIMER: A Web Server for Comprehensive Analysis of Tumor-Infiltrating Immune Cells. Cancer Res 2017;77:e108-10. [Crossref] [PubMed]
- Li T, Fu J, Zeng Z, et al. TIMER2.0 for analysis of tumor-infiltrating immune cells. Nucleic Acids Res 2020;48:W509-14. [Crossref] [PubMed]
- Tang Z, Kang B, Li C, et al. GEPIA2: an enhanced web server for large-scale expression profiling and interactive analysis. Nucleic Acids Res 2019;47:W556-60. [Crossref] [PubMed]
- Bindea G, Mlecnik B, Tosolini M, et al. Spatiotemporal dynamics of intratumoral immune cells reveal the immune landscape in human cancer. Immunity 2013;39:782-95. [Crossref] [PubMed]
- Hänzelmann S, Castelo R, Guinney J. GSVA: gene set variation analysis for microarray and RNA-seq data. BMC Bioinformatics 2013;14:7. [Crossref] [PubMed]
- Chen W, Zheng R, Baade PD, et al. Cancer statistics in China, 2015. CA Cancer J Clin 2016;66:115-32. [Crossref] [PubMed]
- Liu Z, Jiang Y, Yuan H, et al. The trends in incidence of primary liver cancer caused by specific etiologies: Results from the Global Burden of Disease Study 2016 and implications for liver cancer prevention. J Hepatol 2019;70:674-83. [Crossref] [PubMed]
- Li SH, Mei J, Cheng Y, et al. Postoperative Adjuvant Hepatic Arterial Infusion Chemotherapy With FOLFOX in Hepatocellular Carcinoma With Microvascular Invasion: A Multicenter, Phase III, Randomized Study. J Clin Oncol 2023;41:1898-908. [Crossref] [PubMed]
- Zhang PF, Wang F, Wu J, et al. LncRNA SNHG3 induces EMT and sorafenib resistance by modulating the miR-128/CD151 pathway in hepatocellular carcinoma. J Cell Physiol 2019;234:2788-94. [Crossref] [PubMed]
- Shi J, Li J, Yang S, et al. LncRNA SNHG3 is activated by E2F1 and promotes proliferation and migration of non-small-cell lung cancer cells through activating TGF-β pathway and IL-6/JAK2/STAT3 pathway. J Cell Physiol 2020;235:2891-900. [Crossref] [PubMed]
- Dai G, Huang C, Yang J, et al. LncRNA SNHG3 promotes bladder cancer proliferation and metastasis through miR-515-5p/GINS2 axis. J Cell Mol Med 2020;24:9231-43. [Crossref] [PubMed]
- Rao J, Fu J, Meng C, et al. LncRNA SNHG3 Promotes Gastric Cancer Cells Proliferation, Migration, and Invasion by Targeting miR-326. J Oncol 2021;2021:9935410. [Crossref] [PubMed]
- Hegde PS, Chen DS. Top 10 Challenges in Cancer Immunotherapy. Immunity 2020;52:17-35. [Crossref] [PubMed]
- Xiong Z, Chan SL, Zhou J, et al. Targeting PPAR-gamma counteracts tumour adaptation to immune-checkpoint blockade in hepatocellular carcinoma. Gut 2023;72:1758-73. [Crossref] [PubMed]
- Pinter M, Jain RK, Duda DG. The Current Landscape of Immune Checkpoint Blockade in Hepatocellular Carcinoma: A Review. JAMA Oncol 2021;7:113-23. [Crossref] [PubMed]