Identification of cuproptosis and ferroptosis-related subgroups and development of a signature for predicting prognosis and tumor microenvironment landscape in hepatocellular carcinoma
Introduction
Primary liver cancer ranks seventh among all cancers worldwide and is the second leading cause of cancer-related death (1). Hepatocellular carcinoma (HCC) accounts for around 75% of all cases of liver cancer worldwide (2). The overall survival rate of HCC patients remains dismal despite the widespread use of surgical resection, interventional therapy, chemoradiotherapy, targeted therapy, and liver transplantation (3,4). Research is accumulating in favor of the multigene signature’s ability to aid in risk stratification and prognosis prediction in patients with HCC (5,6). As a result, there is a pressing need to define sensitive and implementable molecular markers for precise HCC diagnosis, tailored therapy, and prognostic evaluation.
Ferroptosis is a novel kind of programmed cellular death marked by lipid peroxidation that is exceptionally iron-dependent (7). Ferroptosis has garnered substantial interest as a potential cancer treatment pathway since its introduction in 2012 (8). Extensive studies have shown that ferroptosis plays a crucial role in the elimination of tumor cells and the suppression of their growth (9,10). As a result, inducing ferroptosis has promise as a therapeutic strategy for accelerating cancer cell death (11). Numerous genes have been identified as having important functions in either controlling or detecting ferroptosis. For instance, tumor growth and proliferation are both encouraged by ACSL4 up-regulation, but the cells become more susceptible to ferroptosis as a result (12). A crucial regulator of cancer formation, the P53 tumor suppressor is also associated with ferroptosis. Together, the metabolic processes of ferroptosis and lipids aid P53 in its role as a tumor suppressor (13,14).
New research has shed light on the roles of cuproptosis in a wide variety of diseases, from heart failure and neurodegenerative disorders to metabolic syndrome and inherited disorders (15-17). Throughout the cell, copper plays a crucial role as a structural and catalytic cofactor for enzymes involved in processes as diverse as antioxidant defense, mitochondrial respiration, redox signaling, autophagy, kinase signaling, and many more (18-20). Furthermore, it can serve as a signal to enable responses to the heightened host defenses that immunological activation inevitably produces (21). Cuproptosis is the name given to the process of cell death brought on by copper, as described in a paper published in Science (22). Copper produces a cascade of toxic protein stress and, eventually, cell death by creating a covalent link with lipoacylated components of the tricarboxylic acid (TCA) cycle (23). These findings clarify the significance of cuproptosis in the maintenance, differentiation, and proliferation of cancer cells and shed light on the tumor copper and mitochondrial homeostasis linked with cuproptosis plasticity.
Yet, the relationship between cuproptosis combined with ferroptosis-related genes (FRGs) and the tumor microenvironment (TME) is not fully understood. Consequently, this study intends to utilize integrative bioinformatics analysis to learn more about cuproptosis-related genes (CRGs) and FRGs, such as their functions in HCC prognosis, TME infiltration, and therapeutic response. We present this article in accordance with the TRIPOD reporting checklist (available at https://tcr.amegroups.com/article/view/10.21037/tcr-23-685/rc).
Methods
Data collection
Gene expression data and associated clinicopathological information were made available through the openly accessible databases Cancer Genome Atlas (TCGA), Gene Expression Omnibus (GEO), and International Cancer Genome Consortium (ICGC). In order to decrease the variability, patients who were lacking vital clinical data were discarded. Finally, 374 TCGA-HCC samples, 115 GSE76427 samples, and 80 GSE10141 samples were involved in the TCGA-GEO cohort that was set as the training cohort. In order to create a new merging gene expression matrix, we first removed the batch effect, normalized the data, and corrected for batch variation. For further verification, we used the ICGC-LIRI-JP dataset, which contains clinical details and RNA expression data from 231 Japanese HCC patients. The 259 FRGs were retrieved from the FerrDb website and prior publications. The 19 CRGs were compiled from the best available research. The study was conducted in accordance with the Declaration of Helsinki (as revised in 2013).
Multiomics landscape analysis based on DEGs in the TCGA-HCC dataset
A correlation analysis between CRGs and FRGs to determine which FRGs were most strongly linked with CRGs (corrfilter >0.30, P<0.05). Additionally, we looked for differentially expressed genes (DEGs) associated with ferroptosis and cuproptosis with the appropriate cut-off criteria: |logFC| >1 and false discovery rate (FDR) <0.05. The waterfall graphic illustrates the findings from the analysis and evaluation of somatic mutation data from these DEGs and the tumor mutation burden (TMB). Additionally, copy number variants (CNVs) were analyzed to determine their prevalence in DEGs.
Identification of the ferroptosis subtypes and the ferroptosis-related DEGs
Consensus clustering was used to classify TCGA-GEO cohorts into distinct ferroptosis-related groups (FRGclusters) based on FRGs expression using the “ConsensusClusterPlus” R package (24) with reps =1,000 and pItem =0.8. When determining the appropriate number of clusters, the cumulative distribution function (CDF), delta area plot, matrix heatmap, and tracking plot are taken into consideration. A principal component analysis was performed to illustrate the different ways in which CRGs can be grouped together. Subgroup OS data were analyzed using Kaplan-Meier survival analysis. The ferroptosis-associated DEGs between the different subtypes were identified with |logFC| >1 and FDR <0.05.
Identification of the cuproptosis subtypes and the cuproptosis-related DEGs
Subjects were classified into separate cuproptosis-related groups (CRGclusters) based on their CRGs expression levels using a consensus unsupervised clustering analysis, and the parameters reps =1,000 and pItem =0.8. A principal component analysis was performed to visualize the distribution of subtypes. The differences in the overall survival among subtypes were compared using Kaplan-Meier survival analysis. By employing the limma R package, we identified DEGs linked to cuproptosis across all subtypes, with FDR <0.05 and |logFC| >1 as the cutoffs for significant changes in expression.
Functional enrichment analysis
The differentially enriched pathways (adjusted P value <0.05) of the FRGclusters and CRGclusters were compared using gene set variation analysis (GSVA), with the help of the assisted gene set from the Molecular Signature Database (25). Then, a total of 355 ferroptosis- and cuproptosis-related DEGs were identified by analyzing the intersections between FRGclusters and CRGclusters. Gene Ontology (GO) analysis and Kyoto Encyclopedia of Genes and Genomes (KEGG) pathway analysis were used to delve deeper into the enriched biological activities and pathways among these DEGs (26).
Generation and validation prognostic signature related to ferroptosis and cuproptosis
The prognostic signature was identified and validated using data from the TCGA-GEO cohort and the ICGC cohort. The prognostic significance of DEGs was evaluated using univariate Cox regression analysis. To avoid the overfitting problem, LASSO regression analysis was conducted. Finally, candidate genes for the predictive signature were then chosen using multivariate Cox analysis, yielding the following formula: . The risk coefficient, denoted by coef, and the degree of gene expression, indicated by exp, are presented separately for each gene. Patients were categorized as high-risk or low-risk based on the median risk score. Kaplan-Meier analysis was then performed using the “survminer” program. The effectiveness of the model was evaluated with the use of ROC curves. By including clinical features in the model, we were able to estimate each patient’s likelihood of surviving 1-, 3-, and 5-year post-diagnosis using the “rms” R package to generate a nomogram and associated calibration plots. Better prognostic ability from the nomogram and a calibration curve closer to the 45° line led to a greater prediction effect.
Correlations of the signature with TME
Cancer’s development and progression are both heavily influenced by the TME (27). By examining the levels of expression of critical biomarkers, the ESTIMATE algorithm implemented in the “estimate” R package can detect the existence of TME (28). Using the ssGSEA and CIBERSORT algorithms, we were able to confirm that the high-risk group and the low-risk group had distinct disparities in terms of their immune cells. Finally, we used information about the transcriptome and epigenome of the samples to evaluate the stem cell-like properties of tumors and perform correlation study into the link between the risk score and the cancer stem cell index.
Assessment of TMB, immunotherapy, and chemotherapy
The total number of mutations in each HCC sample was analyzed to evaluate the frequency of TMB between the two groups. Spearman’s analysis revealed a correlation between the risk score and TMB. We also compared TMB between high- and low-risks groups as well as survival rates. After that, the expression of genes linked to immune checkpoint inhibitor (ICI), such as programmed cell death 1 (PD-1), programmed cell death-ligand 1 (PD-L1), cytotoxic T lymphocyte associated antigen 4 (CTLA4), and others, was compared between the groups at low and high risk. The immunophenotype score (IPS) has been shown to be a reliable predictor of how well a patient will respond to immunotherapy (29). The effectiveness of immunotherapy was evaluated using IPS. Further, the “pRRophetic” R package was used to predict the IC50 values of drugs currently used to treat HCC in both high- and low-risk categories (30).
Comparison with published models
We compared the predictive ability of our model to that of other models already in circulation. In order to ensure that the samples were reliable, multivariate Cox regression analysis was utilized to generate a risk score for each. The samples were divided into high-risk and low-risk groups based on the median risk score. Following incorporation of the pertinent genes into each of the models, the ROC curve was generated.
Statistical analysis
All statistical analyses were conducted in R, version 4.2.1. Differences between the groups were examined using student t-tests and analysis of variance. Spearman’s correlation analysis was utilized to determine correlation coefficients between CRGs and FRGs expression and TME. The level of statistical significance used in this research was determined to be P<0.05.
Results
Analysis of the TCGA-HCC multiomic landscape
The workflow of the study is outlined in Figure 1. Based on the analysis of the correlation, we discovered 199 FRGs and CRGs. The protein- protein interaction network of these genes indicates that FRGs and CRGs have substantial connections (Figure 2A). Compared to normal liver tissues, the TCGA-HCC dataset identifies 29 genes, 9 of which are down-regulated and 20 of which are up-regulated, as DEGs (Figure 2B). Then, we performed a comprehensive review of the somatic mutation status of these 29 DEGs (Figure 2C). Overall, there were 82 mutations found in 371 samples, for a somatic mutation incidence of 22.1%; ALB appeared to have the greatest mutation rate (13%). Figure 2D depicts the placements of these 29 DEGs along the rcircos of the chromosomes. Almost every gene experienced CNV, with some showing considerable increases (RPL8, MAFG, PCK2, and TFRC) and others showing significant decreases (STMN1, CDKN2A, and others) (Figure 2E).
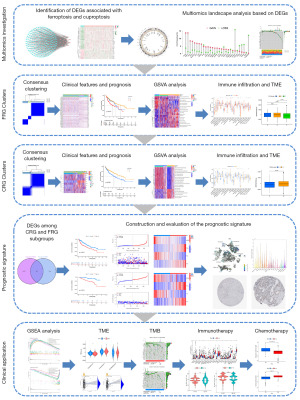
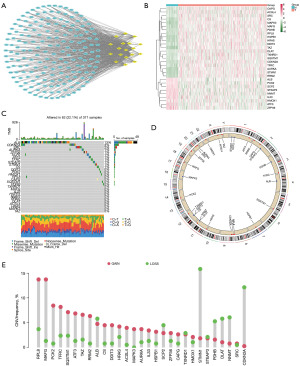
Identification of FRGclusters in HCC
According to the expression of FRGs, the ConsensusClusterPlus R package was used to classify HCC samples into k groups (k=2–9). According to Figure 3A, when k=3, the clustering effect was shown to be the greatest. Disparities in ferroptosis transcription were found among the three FRGclusters, as determined by principal component analysis (Figure 3B). Kaplan-Meier analysis revealed that patients in FRGcluster B had the best chance of survival, while those in FRGcluster C had the worst (Figure 3C). Moreover, FRGs expression levels and clinicopathological features also varied significantly between subtypes (Figure S1). A further finding of the GSVA study was the identification of numerous additional differentially enriched KEGG pathways between FRGclusters. Enrichment levels for several metabolic activities were higher in FRGcluster C, while those for the basal transcription factors, spliceosome, and ribosome were higher in FRGcluster B. FRGcluster A was primarily abundant in Calcium signaling pathways and complement and coagulation cascades (Figure 3D,3E, Figure S1). Further comparison using ssGSEA revealed a substantial variation in the immune infiltration scores of these three FRGclusters. Activated B cell, activated CD4 T+ cell, gamma delta T cell, myeloid-derived suppressor cells (MDSC), and mast cell were all considerably concentrated in FRGcluster B (Figure S2A,S2B), making it the cluster with the highest amount of immune infiltration and associated functions overall. In addition, discrepancies in TME scores between the three FRGclusters were investigated, and the results are presented in Figure S2C-S2F, with reference to the “estimate” R program. Based on the boxplots, it was clear that patients in FRGcluster B had the highest stromal score and estimate score, whereas FRGcluster C had the highest tumor purity. This research provided more evidence that FRGcluster B was comparable to the “hot” tumor, which was more amenable to immunotherapy as a result of its increased immune cell infiltration.
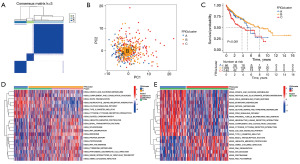
Identification of CRGclusters in HCC
The ConsensusClusterPlus R software was used to reclassify HCC samples into k groups (k=2–9) based on the expression of CRGs. The clustering effect was found to be greatest when k=2 (Figure 4A). Principal component analysis revealed discrepancies in cuproptosis transcription between the two CRG clusters (Figure 4B). As the Kaplan-Meier curve showed, the prognosis for cases in CRGcluster B was more favorable (Figure 4C). Gene clusters were plotted on a heatmap, which revealed associations between gene expression, CRGclusters, and clinical features (Figure 4D). The GSVA enrichment analysis showed that metabolism-related processes were mainly enriched in CRGcluster B, while some cancer-related pathways were mainly enriched in CRGcluster A (Figure 4E). The bulk of immune cells were better at infiltration functions in CRGcluster B, as determined by further ssGSEA analysis of these two clusters (Figure S3A,S3B). In addition, we calculated TME scores for both CRGclusters, discovering that patients in cluster B had higher scores for Stromal and estimate (Figure S3C-S3F). Immunological characteristics allowed us to categorize CRGcluster B tumors as “hot” cancers.
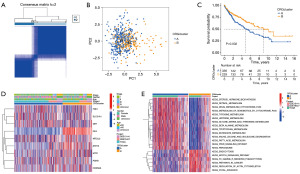
Construction and evaluation of the ferroptosis- and cuproptosis-related prognostic signature
Using the “limma” R package, we identified 355 DEGs among CRG and FRG subgroups to create a prognostic signature (Figure 5A). GO and KEGG enrichment analysis revealed that these 355 DEGs were likely enriched in the RNA splicing, mRNA processing, cadherin binding, Spliceosome, Endocytosis, PI3K-Akt signaling pathway, Hippo signaling pathway (Figure 5B,5C). The prediction model was developed using univariate Cox, LASSO, and multivariate Cox regression on DEGs. In order to determine which genes contribute to HCC survival, a LASSO regression analysis was performed, and ten genes were chosen based on the lowest partial likelihood deviation. Finally, four genes were pinpointed using a multivariate Cox regression analysis (Figure S4). The following equation was used to determine a risk score for each of these genes: risk score = (−0.0012 * HMGCS2 expression) + (0.0331 * PPM1G expression) + (-0.0037 * PON1 expression) + (0.0597 * GNL2 expression). The median threshold value for each patient was used to determine their risk category. The distribution of patients into three FRGclusters and two CRGclusters was depicted in Figure 5D. Meanwhile, the risk score in FRGclusters followed the pattern C > B > A, but in CRGclusters the order was B > A (Figure 5E,5F). Patients with low-risk had a better survival rate than those with higher scores in TCGA-GEO cohort and ICGC cohort (P<0.001). The area under the curves (AUCs) of the ROC curves related to survival rates in the TCGA-GEO cohort (1-, 3-, and 5-year AUCs: 0.730, 0.649, 0.608, respectively) and in the ICGC cohort (1-, 3-, and 5-year AUCs: 0.742, 0.715, 0.599, respectively) were calculated (Figure 5G-5L). Heat map was used to display the differential expression of model genes between high- and low-risk groups in the TCGA-GEO and ICGC cohort (Figure S4).
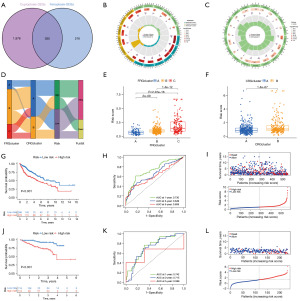
We also investigated the expression of HMGCS2, PPM1G, PON1, and GNL2 in HCC and normal liver tissues using single-cell RNA-seq data. Higher expression of PPM1G and GNL2 was observed in carcinoma cells than in hepatocytes. More importantly, the single-cell RNA-seq analysis results suggested that these genes were not only expressed in liver cells but also expressed in other types of cells, such as Kupffer cells, cDC2, and SAMs (Figure 6A-6D). Further analysis of these genes’ protein expression levels was performed utilizing the HPA database. Both PPM1G and GNL2 protein levels were found to be greater in HCC tissues compared to those of healthy liver tissues (Figure 6E).
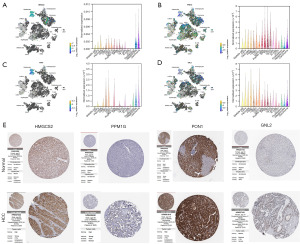
Independent prognostic analysis and establishment of a nomogram
To further validate the signature’s predictive power, nomograms were generated utilizing clinical characteristics and risk scores to predict 1-, 3-, and 5-year OS in HCC. There was considerable concordance between the data and calibration curves. From the decision curve analysis (DCA) curves, it appears that the nomogram may be more effective than the risk score and other clinical variables in predicting the prognosis of HCC patients (Figure S5).
Correlations of the signature with TME
In order to evaluate the differences between the biological processes of high- and low-risk populations, a GSEA was carried out (Figure 7A,7B). We observed that immune-related activities, such as ECM receptor interaction, hematopoietic cell lineage, and leishmania infection, were most highly correlated with a high-risk score. Next, immunological infiltration was analyzed using ssGSEA, which revealed that high-risk individuals had a markedly enhanced population of activated CD4+ T cell, natural killer (NK) cell, and type II T helper cell (Figure 7C). M2 macrophages were prevalent in the high-risk population, as determined by CIBERSORT. In addition, activated macrophages M2 and mast cells activated both positively correlated with risk score, while naïve B cells and resting mast cells negatively correlated (Figure 7D-7H). We determined the relationship between model genes and the amount of immune cells and discovered that several categories of immune cells, such as M2 macrophages, M1 macrophages, and resting mast cells, were substantially connected with the genes (Figure 7I). We also examined the TME scores of these two groups and found that those at high risk had more immune cells (Figure 7J). In addition, there was a negative correlation between risk scores and stromal cells and tumor purity, and a positive correlation between risk scores and immune cells and estimated scores (Figure 7K-7N).
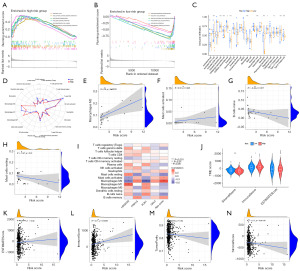
To better understand the distinctions between the two groups, the TCGA samples were separated into a variety of immunological subgroups. C3 and C4 were the two predominant subtypes. The risk scores for the C2 immunological subtype were lower than those for the C1 subtype but significantly higher than those for the C3 and C4 subtypes (Figure 8A,8B). Significantly higher immunogenicity prediction scores (IPS) were seen in the low-risk group (P<0.001) (Figure 8C, Figure S3). We found that the expression levels of 29 immunological checkpoints varied among risk groups when we correlated them with our model (Figure 8D). Several immunological checkpoints, including CD276, PDCD1, and CTLA4, had higher expression levels as the risk score increased.
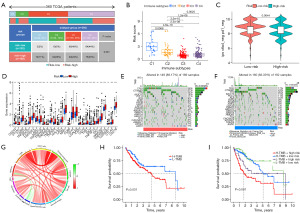
Relationships between the signature and tumor stem cells as well as TMB
The waterfall plots of the TMB for the low-risk and high-risk groups were depicted in Figure 8E,8F, respectively. The top 10 most mutated genes included TP53, CTNNB1, TTN, MUC16, PCLO, ALB, RYR2, APOB, XIRP2, and LRP1B. There was a positive relationship between the risk score and TMB (Figure 8G). Moreover, the patients in the highest TMB and highest risk cohorts had the worst prognosis (Figure 8H,8I). When analyzing samples with RNAss to establish the regulatory influence of risk score, a positive correlation was found between risk score and cancer stem cells (R=0.27, P<0.001), showing that samples with higher scores had more pronounced stem cell features and a lower degree of cell differentiation (Figure S5).
Drug sensitivity analysis
The low-risk and high-risk groups showed considerable disparities in drug sensitivity, as shown by our examination of IC50 values for a number of medications. The IC50 values for drugs including doxorubicin, epothilone B, gemcitabine, IPA.3, mitomycin C, and paclitaxel were significantly lower in the high-risk group than in the low-risk group, indicating that they were more sensitive to these drugs (Figure 9A-9F). We draw the conclusion that Axitinib, Vinorelbine, and Sorafenib are more efficacious in this population since their IC50 values were significantly lower in the low-risk group (Figure 9G-9I).
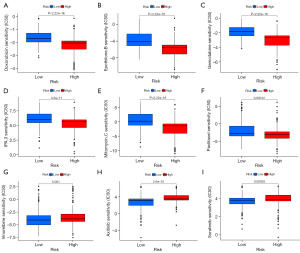
New model as a new predictor of HCC
We compared our own model to 12 other published HCC prognostic models to better highlight its predictive capability (31-42). Using multivariate analysis, we determined the risk value and prognosis evaluation for each dataset, making the 13 models comparable. Overall, the survival curves across all 13 models showed a grim outlook for those at high risk (Figure S6). The ROC curve demonstrates that the AUC values of competing models are less than that of our model (Figure 10A-10M). This alone illustrates the superior predictive ability of our model. We next determined the C-index for each prognostic factor by using the restricted mean survival (RMS) software tool. When compared to other models, ours has a higher C-index of 0.658 (Figure 10N). RMS can be used to assess the impact of gene features across time on prediction. Our genetic traits were at their peak performance around the eighth year (Figure 10O). This demonstrates that, in comparison to competing models, ours provides the most accurate predictions.
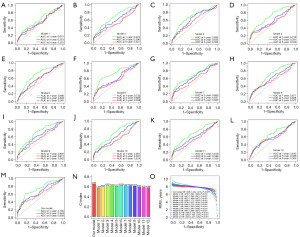
Discussion
Homeostasis in biological systems, the evolution of complex tissues, and the prevention of cancer by means of regulated cell division are all dependent on programmed cell death (43). Cuproptosis, first recognized by Todd R. Golub, explains how mitochondrial malfunction and copper metabolism contribute to cell death. Since its introduction in 2012, ferroptosis has also gained considerable interest as a potential cancer treatment method. Numerous research has examined the significance of individual cuproptosis and ferroptosis regulator genes in cancer, but the combined effect of multiple CRGs and FRGs remains a mystery. Despite the large number of studies confirming that ferroptosis and cuproptosis are indeed associated with HCC prognosis, there is still a lack of a prognostic model for HCC that combines the characteristics of ferroptosis and cuproptosis (44-47). Therefore, the aim of this study was to determine to identify ferroptosis and cuproptosis subtypes in HCC and to determine whether ferroptosis and cuproptosis are associated with HCC and, if so, whether they can be used to develop a new scoring system to predict prognosis and treatment outcome.
Multiomics study of the TCGA-HCC cohort yielded in-depth descriptions of somatic mutations, CNV frequency, chromosome position, and interactions connected to CRGs and FRGs. The expression of FRGs and CRGs permitted the classification of HCC patients into three FRGclusters and two CRGclusters, with each cluster exhibiting significantly different survival, recurrence, and biological function activities. Tumors in FRGcluster B and CRGcluster B were deemed “hot” due to their high levels of immune cell infiltration, indicating that they will respond well to immunotherapy. GSVA analysis found that the majority of the divergent pathways across the three FRGclusters and two CRGclusters were connected to metabolism, a factor commonly recognized to be critical for the genesis and progression of tumors.
To better assess the cuproptosis and ferroptosis pattern in individual patients with HCC, we further constructed a predictive model incorporating HMGCS2, PPM1G, PON1, and GNL2. HMGCS2 is the rate-limiting enzyme in the ketogenesis pathway (48). HMGCS2 knockdown has been demonstrated to reduce ketone generation, promote cell proliferation, migration, and xenograft carcinogenesis in HCC via upregulating c-Myc/cyclinD1 and EMT signaling and downregulating caspase-dependent apoptotic pathways, and decrease ketone levels (49). Additionally, HMGCS2 is implicated in metabolic pathways, and downregulation of HMGCS2 in tumors is related with poor OS in HCC patients (50). PPM1G is an essential member of the PP2C family of serine/threonine protein phosphatases and plays a crucial function in the regulation of cell cycle progression (51,52). Phosphorylation of the alternative splicing protein SRSF3 has recently been shown to be regulated by PPM1G, providing more evidence that this protein promotes HCC proliferation, invasion, and metastasis (53). Paraoxonase-1 (PON1) gene polymorphisms are strongly linked to the development of advanced malignancies (54). Low PON1 activity impairs its inherent functions and heightens oxidative stress (55,56), suggesting a worse prognosis in patients with cancer (57). Huang et al. (58) hypothesized that serum PON1 levels may serve as a biomarker for microvascular invasion. As a protein-encoding gene, G protein nucleolar 2 (GNL2) goes by several other names, including NGP1, Nog2, Nug2, Ngp-1, and HUMAUANTIG. Dong et al. (59) reported that HCC patients with high GNL2 expression had lower survival rates, and in vitro knockdown investigations demonstrated that decreasing GNL2 using siRNA inhibited HCC cell proliferation, migration, and invasion capabilities. Consequently, the model may be utilized to assess the prognosis of HCC.
TME, also known as the tumor microenvironment, is the environment that surrounds a tumor in the body and is thought to be the main driver of tumor development (60). Given the abundant evidence that copper and iron are involved in immune modulation, it is possible that understanding the role of ferroptosis and cuproptosis in TME cell infiltration will aid in our understanding of the response to HCC anticancer therapy and guide the development of more effective immunotherapy techniques (61). In comparing high- and low-risk groups, immunological pathways were discovered to play a significant influence by GSEA enrichment analysis. Subjects in the high-risk group had higher levels of immune cells in their blood than those in the low-risk group, including M2 macrophages, activated CD4+ T cells, NK cells, and Type II T helper cells. Macrophages play a variety of vital roles crucial to immune system function, normal development, and cancer progression (62). Tumor-associated macrophages (TAMs), also known as M2 macrophages, are an immune cell subset that infiltrates tumors and promotes their growth and metastasis. (63). Multiple pieces of evidence point to the role of TAMs in facilitating tumor angiogenesis, immunosuppressive actions, and the spread of tumor cells during tumor initiation and metastasis (64). Nearly half of the liver’s lymphocytes are NK cells, which possess cytotoxic capabilities that enable them to combat malignancies (65). NK cells’ primary purpose is to detect and destroy target cells, but they also regulate antiviral immune responses by secreting cytokines such as interferon-gamma (IFN-γ) and tumor necrosis factor-alpha (TNF-α) (66). Other immune-related evaluations have demonstrated that high-risk groups have higher immunological scores, and as a result, high-risk individuals respond more favorably to immunotherapy. Tumors belonging to the high-risk group, FRGcluster B, and CRGcluster B are deemed “hot” because they exhibit substantial immune infiltration and activation and are therefore more likely to respond favorably to immunotherapy based on these findings.
Immunotherapy, specifically checkpoint inhibitors, has led to significant improvements in survival (67). The analysis suggests that CD276, PDCD1, TIGIT, and CTLA4 were all more highly expressed in the high-risk patients. These four genes have been the subject of extensive research, establishing their viability as therapeutic targets (68,69). According to recent studies (70), TMB can be used to predict how well cancer patients would respond to immunotherapy. Our results are consistent with those of a large body of tumor cases that found a higher TMB score to be predictive of success with immunotherapy. Our findings are comparable to a substantial corpus of tumor cases showing that a higher TMB score is indicative of immunotherapy efficacy. Therefore, research offered more proof that immunotherapy may be more effective in high-risk groups. Paclitaxel, doxorubicin, epothilone B, gemcitabine, IPA.3, and mitomycin C were all discovered to be beneficial for patients in the high-risk group.
Finally, we selected 12 prognostic risk models for HCC from the literature and assessed their capacity to predict outcomes. Our model’s prediction performance was really excellent. However, current research is still hampered by significant limitations. To begin, there is a paucity of clinical data and experimental study to further verify the conclusions, and all inferences are made based on the processing and analysis of data available from public databases. To further validate the model’s efficacy in clinical settings, it will be necessary to gather additional HCC cases and conduct a large number of prospective clinical assessments in the future.
Conclusions
Our in-depth analysis of CRGs and FRGs confirmed their value for examining TME, clinical characteristics, and HCC prognosis. These findings emphasized the possible clinical relevance of CRGs and FRGs and suggested that ferroptosis and cuproptosis may be therapeutic targets for HCC patients.
Acknowledgments
Funding: The study was supported by fundings from
Footnote
Reporting Checklist: The authors have completed the TRIPOD reporting checklist. Available at https://tcr.amegroups.com/article/view/10.21037/tcr-23-685/rc
Peer Review File: Available at https://tcr.amegroups.com/article/view/10.21037/tcr-23-685/prf
Conflicts of Interest: All authors have completed the ICMJE uniform disclosure form (available at https://tcr.amegroups.com/article/view/10.21037/tcr-23-685/coif). The authors have no conflicts of interest to declare.
Ethical Statement: The authors are accountable for all aspects of the work in ensuring that questions related to the accuracy or integrity of any part of the work are appropriately investigated and resolved. The study was conducted in accordance with the Declaration of Helsinki (as revised in 2013).
Open Access Statement: This is an Open Access article distributed in accordance with the Creative Commons Attribution-NonCommercial-NoDerivs 4.0 International License (CC BY-NC-ND 4.0), which permits the non-commercial replication and distribution of the article with the strict proviso that no changes or edits are made and the original work is properly cited (including links to both the formal publication through the relevant DOI and the license). See: https://creativecommons.org/licenses/by-nc-nd/4.0/.
References
- Bray F, Ferlay J, Soerjomataram I, et al. Global cancer statistics 2018: GLOBOCAN estimates of incidence and mortality worldwide for 36 cancers in 185 countries. CA Cancer J Clin 2018;68:394-424. [Crossref] [PubMed]
- Petrick JL, Florio AA, Znaor A, et al. International trends in hepatocellular carcinoma incidence, 1978-2012. Int J Cancer 2020;147:317-30. [Crossref] [PubMed]
- Feng D, Wang M, Hu J, et al. Prognostic value of the albumin-bilirubin grade in patients with hepatocellular carcinoma and other liver diseases. Ann Transl Med 2020;8:553. [Crossref] [PubMed]
- Li W, Chen QF, Huang T, et al. Identification and Validation of a Prognostic lncRNA Signature for Hepatocellular Carcinoma. Front Oncol 2020;10:780. [Crossref] [PubMed]
- Wang Y, Song F, Zhang X, et al. Mitochondrial-Related Transcriptome Feature Correlates with Prognosis, Vascular Invasion, Tumor Microenvironment, and Treatment Response in Hepatocellular Carcinoma. Oxid Med Cell Longev 2022;2022:1592905. [Crossref] [PubMed]
- Lin Z, Xu Q, Miao D, et al. An Inflammatory Response-Related Gene Signature Can Impact the Immune Status and Predict the Prognosis of Hepatocellular Carcinoma. Front Oncol 2021;11:644416. [Crossref] [PubMed]
- Qiu Y, Cao Y, Cao W, et al. The Application of Ferroptosis in Diseases. Pharmacol Res 2020;159:104919. [Crossref] [PubMed]
- Dixon SJ. Ferroptosis: bug or feature? Immunol Rev 2017;277:150-7. [Crossref] [PubMed]
- Mou Y, Wang J, Wu J, et al. Ferroptosis, a new form of cell death: opportunities and challenges in cancer. J Hematol Oncol 2019;12:34. [Crossref] [PubMed]
- Hassannia B, Vandenabeele P, Vanden Berghe T. Targeting Ferroptosis to Iron Out Cancer. Cancer Cell 2019;35:830-49. [Crossref] [PubMed]
- Liang C, Zhang X, Yang M, et al. Recent Progress in Ferroptosis Inducers for Cancer Therapy. Adv Mater 2019;31:e1904197. [Crossref] [PubMed]
- Yuan H, Li X, Zhang X, et al. Identification of ACSL4 as a biomarker and contributor of ferroptosis. Biochem Biophys Res Commun 2016;478:1338-43. [Crossref] [PubMed]
- Xie Y, Zhu S, Song X, et al. The Tumor Suppressor p53 Limits Ferroptosis by Blocking DPP4 Activity. Cell Rep 2017;20:1692-704. [Crossref] [PubMed]
- Tarangelo A, Magtanong L, Bieging-Rolett KT, et al. p53 Suppresses Metabolic Stress-Induced Ferroptosis in Cancer Cells. Cell Rep 2018;22:569-75. [Crossref] [PubMed]
- Liu Y, Miao J. An Emerging Role of Defective Copper Metabolism in Heart Disease. Nutrients 2022;14:700. [Crossref] [PubMed]
- Bisaglia M, Bubacco L. Copper Ions and Parkinson's Disease: Why Is Homeostasis So Relevant?. Biomolecules 2020;10:195. [Crossref] [PubMed]
- Członkowska A, Litwin T, Dusek P, et al. Wilson disease. Nat Rev Dis Primers 2018;4:21. [Crossref] [PubMed]
- Kim BE, Nevitt T, Thiele DJ. Mechanisms for copper acquisition, distribution and regulation. Nat Chem Biol 2008;4:176-85. [Crossref] [PubMed]
- Rae TD, Schmidt PJ, Pufahl RA, et al. Undetectable intracellular free copper: the requirement of a copper chaperone for superoxide dismutase. Science 1999;284:805-8. [Crossref] [PubMed]
- Denoyer D, Masaldan S, La Fontaine S, et al. Targeting copper in cancer therapy: ‘Copper That Cancer’. Metallomics 2015;7:1459-76. [Crossref] [PubMed]
- Hodgkinson V, Petris MJ. Copper homeostasis at the host-pathogen interface. J Biol Chem 2012;287:13549-55. [Crossref] [PubMed]
- Tsvetkov P, Coy S, Petrova B, et al. Copper induces cell death by targeting lipoylated TCA cycle proteins. Science 2022;375:1254-61. [Crossref] [PubMed]
- Davis CI, Gu X, Kiefer RM, et al. Altered copper homeostasis underlies sensitivity of hepatocellular carcinoma to copper chelation. Metallomics 2020;12:1995-2008. [Crossref] [PubMed]
- Wilkerson MD, Hayes DN. ConsensusClusterPlus: a class discovery tool with confidence assessments and item tracking. Bioinformatics 2010;26:1572-3. [Crossref] [PubMed]
- Hänzelmann S, Castelo R, Guinney J. GSVA: gene set variation analysis for microarray and RNA-seq data. BMC Bioinformatics 2013;14:7. [Crossref] [PubMed]
- Chen L, Zhang YH, Wang S, et al. Prediction and analysis of essential genes using the enrichments of gene ontology and KEGG pathways. PLoS One 2017;12:e0184129. [Crossref] [PubMed]
- Arneth B. Tumor Microenvironment. Medicina (Kaunas) 2019;56:15. [Crossref] [PubMed]
- Yoshihara K, Shahmoradgoli M, Martínez E, et al. Inferring tumour purity and stromal and immune cell admixture from expression data. Nat Commun 2013;4:2612. [Crossref] [PubMed]
- Xu Q, Chen S, Hu Y, et al. Landscape of Immune Microenvironment Under Immune Cell Infiltration Pattern in Breast Cancer. Front Immunol 2021;12:711433. [Crossref] [PubMed]
- Geeleher P, Cox N, Huang RS. pRRophetic: an R package for prediction of clinical chemotherapeutic response from tumor gene expression levels. PLoS One 2014;9:e107468. [Crossref] [PubMed]
- Wang XX, Wu LH, Ji H, et al. A novel cuproptosis-related prognostic signature and potential value in HCC immunotherapy. Front Mol Biosci 2022;9:1001788. [Crossref] [PubMed]
- Wang G, Xiao R, Zhao S, et al. Cuproptosis regulator-mediated patterns associated with immune infiltration features and construction of cuproptosis-related signatures to guide immunotherapy. Front Immunol 2022;13:945516. [Crossref] [PubMed]
- Chen X, Hu G, Xiong L, et al. Relationships of Cuproptosis-Related Genes With Clinical Outcomes and the Tumour Immune Microenvironment in Hepatocellular Carcinoma. Pathol Oncol Res 2022;28:1610558. [Crossref] [PubMed]
- Zhao C, Zhang Z, Jing T. A novel signature of combing cuproptosis- with ferroptosis-related genes for prediction of prognosis, immunologic therapy responses and drug sensitivity in hepatocellular carcinoma. Front Oncol 2022;12:1000993. [Crossref] [PubMed]
- Zhang B, Zhao J, Liu B, et al. Development and Validation of a Novel Ferroptosis-Related Gene Signature for Prognosis and Immunotherapy in Hepatocellular Carcinoma. Front Mol Biosci 2022;9:940575. [Crossref] [PubMed]
- Li W, Liu J, Zhang D, et al. The Prognostic Significance and Potential Mechanism of Ferroptosis-Related Genes in Hepatocellular Carcinoma. Front Genet 2022;13:844624. [Crossref] [PubMed]
- Wang H, Yang C, Jiang Y, et al. A novel ferroptosis-related gene signature for clinically predicting recurrence after hepatectomy of hepatocellular carcinoma patients. Am J Cancer Res 2022;12:1995-2011. [PubMed]
- Wang W, Pan F, Lin X, et al. Ferroptosis-Related Hub Genes in Hepatocellular Carcinoma: Prognostic Signature, Immune-Related, and Drug Resistance Analysis. Front Genet 2022;13:907331. [Crossref] [PubMed]
- Zhao C, Zhang Z, Tao J. A Novel Ferroptosis-Related Signature for Prediction of Prognosis, Immune Profiles and Drug Sensitivity in Hepatocellular Carcinoma Patients. Curr Oncol 2022;29:6992-7011. [Crossref] [PubMed]
- Zhang Y, Ren H, Zhang C, et al. Development and validation of four ferroptosis-related gene signatures and their correlations with immune implication in hepatocellular carcinoma. Front Immunol 2022;13:1028054. [Crossref] [PubMed]
- Long S, Chen Y, Wang Y, et al. Identification of Ferroptosis-related molecular model and immune subtypes of hepatocellular carcinoma for individual therapy. Cancer Med 2023;12:2134-47. [Crossref] [PubMed]
- Luo L, Yao X, Xiang J, et al. Identification of ferroptosis-related genes for overall survival prediction in hepatocellular carcinoma. Sci Rep 2022;12:10007. [Crossref] [PubMed]
- Strasser A, Vaux DL. Cell Death in the Origin and Treatment of Cancer. Mol Cell 2020;78:1045-54. [Crossref] [PubMed]
- Du Y, Gao Y. Development and validation of a novel pseudogene pair-based prognostic signature for prediction of overall survival in patients with hepatocellular carcinoma. BMC Cancer 2020;20:887. [Crossref] [PubMed]
- Dai Y, Qiang W, Lin K, et al. An immune-related gene signature for predicting survival and immunotherapy efficacy in hepatocellular carcinoma. Cancer Immunol Immunother 2021;70:967-79. [Crossref] [PubMed]
- Wan S, Lei Y, Li M, et al. A prognostic model for hepatocellular carcinoma patients based on signature ferroptosis-related genes. Hepatol Int 2022;16:112-24. [Crossref] [PubMed]
- Deng M, Sun S, Zhao R, et al. The pyroptosis-related gene signature predicts prognosis and indicates immune activity in hepatocellular carcinoma. Mol Med 2022;28:16. [Crossref] [PubMed]
- Shafqat N, Turnbull A, Zschocke J, et al. Crystal structures of human HMG-CoA synthase isoforms provide insights into inherited ketogenesis disorders and inhibitor design. J Mol Biol 2010;398:497-506. [Crossref] [PubMed]
- Wang YH, Liu CL, Chiu WC, et al. HMGCS2 Mediates Ketone Production and Regulates the Proliferation and Metastasis of Hepatocellular Carcinoma. Cancers (Basel) 2019;11:1876. [Crossref] [PubMed]
- Ding R, Chen T, Zhang Y, et al. HMGCS2 in metabolic pathways was associated with overall survival in hepatocellular carcinoma: A LASSO-derived study. Sci Prog 2021;104:368504211031749. [Crossref] [PubMed]
- Sun C, Wang G, Wrighton KH, et al. Regulation of p27(Kip1) phosphorylation and G1 cell cycle progression by protein phosphatase PPM1G. Am J Cancer Res 2016;6:2207-20. [PubMed]
- Beli P, Lukashchuk N, Wagner SA, et al. Proteomic investigations reveal a role for RNA processing factor THRAP3 in the DNA damage response. Mol Cell 2012;46:212-25. [Crossref] [PubMed]
- Chen D, Zhao Z, Chen L, et al. PPM1G promotes the progression of hepatocellular carcinoma via phosphorylation regulation of alternative splicing protein SRSF3. Cell Death Dis 2021;12:722. [Crossref] [PubMed]
- Aldonza MBD, Son YS, Sung HJ, et al. Paraoxonase-1 (PON1) induces metastatic potential and apoptosis escape via its antioxidative function in lung cancer cells. Oncotarget 2017;8:42817-35. [Crossref] [PubMed]
- Ferré N, Camps J, Prats E, et al. Serum paraoxonase activity: a new additional test for the improved evaluation of chronic liver damage. Clin Chem 2002;48:261-8. [Crossref] [PubMed]
- Wang B, Yang RN, Zhu YR, et al. Involvement of xanthine oxidase and paraoxonase 1 in the process of oxidative stress in nonalcoholic fatty liver disease. Mol Med Rep 2017;15:387-95. [Crossref] [PubMed]
- Ellidag HY, Eren E, Aydin O, et al. Multiple myeloma: relationship to antioxidant esterases. Med Princ Pract 2014;23:18-23. [Crossref] [PubMed]
- Huang C, Wang Y, Liu S, et al. Quantitative proteomic analysis identified paraoxonase 1 as a novel serum biomarker for microvascular invasion in hepatocellular carcinoma. J Proteome Res 2013;12:1838-46. [Crossref] [PubMed]
- Dong Y, Cai Q, Fu L, et al. Study of the G Protein Nucleolar 2 Value in Liver Hepatocellular Carcinoma Treatment and Prognosis. Biomed Res Int 2021;2021:4873678. [Crossref] [PubMed]
- Li BL, Wan XP. Prognostic significance of immune landscape in tumour microenvironment of endometrial cancer. J Cell Mol Med 2020;24:7767-77. [Crossref] [PubMed]
- Culbertson EM, Culotta VC. Copper in infectious disease: Using both sides of the penny. Semin Cell Dev Biol 2021;115:19-26. [Crossref] [PubMed]
- Kashfi K, Kannikal J, Nath N. Macrophage Reprogramming and Cancer Therapeutics: Role of iNOS-Derived NO. Cells 2021;10:3194. [Crossref] [PubMed]
- Xu F, Cui WQ, Wei Y, et al. Astragaloside IV inhibits lung cancer progression and metastasis by modulating macrophage polarization through AMPK signaling. J Exp Clin Cancer Res 2018;37:207. [Crossref] [PubMed]
- Singh Y, Pawar VK, Meher JG, et al. Targeting tumor associated macrophages (TAMs) via nanocarriers. J Control Release 2017;254:92-106. [Crossref] [PubMed]
- Robinson MW, Harmon C, O'Farrelly C. Liver immunology and its role in inflammation and homeostasis. Cell Mol Immunol 2016;13:267-76. [Crossref] [PubMed]
- Sajid M, Liu L, Sun C. The Dynamic Role of NK Cells in Liver Cancers: Role in HCC and HBV Associated HCC and Its Therapeutic Implications. Front Immunol 2022;13:887186. [Crossref] [PubMed]
- De Felice F, Marchetti C, Tombolini V, et al. Immune check-point in endometrial cancer. Int J Clin Oncol 2019;24:910-6. [Crossref] [PubMed]
- Miao Y, Wang J, Li Q, et al. Prognostic value and immunological role of PDCD1 gene in pan-cancer. Int Immunopharmacol 2020;89:107080. [Crossref] [PubMed]
- Liu JN, Kong XS, Huang T, et al. Clinical Implications of Aberrant PD-1 and CTLA4 Expression for Cancer Immunity and Prognosis: A Pan-Cancer Study. Front Immunol 2020;11:2048. [Crossref] [PubMed]
- Chalmers ZR, Connelly CF, Fabrizio D, et al. Analysis of 100,000 human cancer genomes reveals the landscape of tumor mutational burden. Genome Med 2017;9:34. [Crossref] [PubMed]