The ultrasound-based radiomics-clinical machine learning model to predict papillary thyroid microcarcinoma in TI-RADS 3 nodules
Highlight box
Key findings
• Ultrasound-based radiomics approach is effective in predicting papillary thyroid microcarcinoma (PTMC) in patients with Thyroid Imaging Reporting and Data System (TI-RADS) 3 nodules.
What is known and what is new?
• Ultrasound-based radiomics has demonstrated good diagnostic performance for various diseases such as ovarian epithelial cancer, intrahepatic cholangiocarcinoma, and breast cancer.
• This is the first study to investigate the potential of ultrasound-based radiomics for predicting PTMC in patients with TI-RADS 3 nodules.
What is the implication, and what should change now?
• The application of a radiomics approach to ultrasound images can effectively predict PTMC in patients with thyroid nodules with a TI-RADS score of 3. Incorporating radiomics features into clinical variables can improve the accuracy of PTMC prediction compared to using clinical variables alone. Further investigation is needed to test the value of our findings on a broader multicenter patient sample.
Introduction
Thyroid carcinoma is the most common malignant tumor in endocrine system. Over the years, there has been a gradual rise in its occurrence, making it a subject of growing concern within the medical and scientific community. Presently, the incidence of thyroid carcinoma has surpassed that of all other malignant tumors in terms of its increment (1). The escalation in its rates can be attributed to papillary carcinoma, particularly in its early stages (2-4). Another significant factor contributing to the upsurge in thyroid carcinoma cases is the higher occurrence of papillary thyroid microcarcinoma (PTMC), which comprises carcinomas measuring 1.0 cm or less (3). Thus, studying PTMC has gained more and more importance.
The small size of the cancer, its subtle onset, slow advancement, lack of apparent clinical symptoms, and frequent co-occurrence with other thyroid disorders pose difficulties in making an accurate preoperative diagnosis, resulting in a certain degree of both underdiagnosis and misdiagnosis. Despite concerns regarding ultrasound’s ability to differentiate between benign and malignant thyroid nodules, it remains the preferred imaging technique for evaluating the morphological features of thyroid nodules. This is due to its numerous advantages, including high resolution, lack of ionizing radiation, portability, and ease of use (5-7). Recently, several ultrasound centers have embraced Thyroid Imaging Reporting and Data System (TI-RADS) criteria to evaluate benign and malignant thyroid nodules. However, due to significant overlap in the ecomorphological features of both types of nodules, some thyroid nodules categorized as TI-RADS 3 are later confirmed to be PTMC through pathology examination (8). Furthermore, the interpretation of conventional ultrasound (CUS) characteristics is subject to personal judgment and operator-dependent. This further leads to differences in readings by various individuals (9). A prior study has shown that the risk of malignancy for TI-RADS 3 thyroid nodules is 2.1% (10).
Ultrasound-guided fine-needle aspiration biopsy (FNAB) is widely utilized as the primary diagnostic approach for thyroid nodules. It is recognized as a highly efficient, uncomplicated, and secure technique for detecting head and neck anomalies, notably thyroid nodules (11-13). Research has revealed that diagnosing PTMC via FNAB of thyroid nodules can be difficult. In nodules with a size of less than 1 cm, there is a relatively high incidence of false-negative outcomes due to inadequate cytology samples (14,15). Moreover, the rate of metastasis of PTMC to the cervical lymph nodes has been reported to be high (16-18). Importantly, lymph node metastasis is closely associated with PTMC recurrence. Additionally, certain patients may experience distant metastases to the lungs or bones (19). Therefore, the early and accurate diagnosis of PTMC holds immense importance. Consequently, there is an urgent need for a reliable and non-invasive method to classify and identify PTMC.
Radiomics is a rapidly developing research field by incorporating computational methods (9). Radiomics harnesses the extensive and intricate digital data obtained from imaging modalities to uncover a plethora of quantitative disease features that may not be visible to the human eye (20-22). This powerful technique allows us to extract and analyze a wide range of intricate details, providing valuable insights into the disease process. CUS-based radiomics has demonstrated good diagnostic performance for various diseases such as ovarian epithelial cancer, intrahepatic cholangiocarcinoma, and breast cancer (23-25). The integration of clinical information and radiomics may further improve diagnostic performance. Hence, the principal aim of this study was to assess the efficacy of CUS-based radiomics for distinguishing between benign thyroid nodules and PTMC. We present this article in accordance with the TRIPOD reporting checklist (available at https://tcr.amegroups.com/article/view/10.21037/tcr-23-1375/rc).
Methods
Patient enrollment and data acquisition
From January 2019 to October 2022, a retrospective study was conducted on 221 patients who had TI-RADS 3 nodules and were pathologically diagnosed with PTMC and benign thyroid nodules. The study was conducted in accordance with the Declaration of Helsinki (as revised in 2013). This study was approved by institutional ethics committee of the Second Affiliated Hospital of Wenzhou Medical University (No. 2023-K-43-01), which waived the need for informed consent from each patient. However, written consent was obtained from each patient before surgery or biopsy. The inclusion criteria consisted of the following categories: (I) patients with pathological results of PTMC or benign thyroid nodules; (II) patients with clear B-mode ultrasound images; (III) patients with detailed clinical information. The exclusion criteria consisted of the following categories: (I) patients with low-quality ultrasound images; (II) patients who received preoperative therapy; (III) patients who have a history of other malignancies or coexisting malignancies (Figure 1).
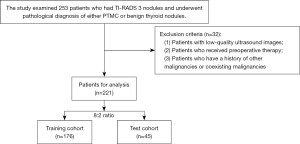
CUS examination and interpretation of CUS features
All ultrasound examinations were carried out by board-certified radiologists who possess a minimum of five years of experience in conducting ultrasound imaging specifically for superficial tissue, utilizing ultrasound machines, including Resona7 (Mindray, Shenzhen, China), Aplio 500 (Toshiba Medical Systems, Tokyo, Japan) and ESAOTE (MyLab 90 X-vision, Genoa, Italy) with corresponding high-frequency probes. Images of the largest long axis cross-section of target nodules were obtained for subsequent analysis.
Two experienced radiologists (with over five years of experience in thyroid sonography) independently reviewed all images without knowledge of clinical information or final diagnoses. The CUS features reinterpreted included tumor dimension, echotexture (homogeneous, heterogeneous), echogenicity (hypoechoic, iso/hyperechoic, or mixed), margin (well-defined and ill-defined), presence of calcification (absent, macrocalcification, and microcalcification), aspect ratio (>1 or ≤1).
The radiomics analysis process consisted of segmenting the lesions, extracting features, selecting relevant features, and constructing a model. Two blinded radiologists manually segmented the regions of interest to ensure accuracy. Prior to feature extraction, intensity normalization was performed. The patients were then randomly divided into a training and test cohort in an 8:2 ratio. This division provided a suitable dataset for training the model and evaluating its performance.
Segmenting the lesions and extracting radiomics features
The radiomics analysis workflow is shown in Figure 2. An experienced radiologist carefully performed the manual segmentation of a region of interest (ROI). To ensure impartiality, both the radiologist who conducted the segmentation and the one who confirmed it were unaware of any clinical information associated with the patient. The ROI was delineated using itk-SNAP software (http://www.itksnap.org; version 4.0.1) around the lesion contour, and intensity normalization was performed on ultrasound images prior to radiomics feature extraction to standardize gray intensity values. Then a total of 1,477 radiomics features were extracted. In this study, the handcrafted features can be classified into three distinct groups: geometry, intensity, and texture. The 3-dimensional (3D) shape characteristics of the tumor are described by the geometry features, whereas the first-order statistical distribution of voxel intensities within the tumor is described by the intensity features. On the other hand, texture features characterize patterns that are obtained from second- and high-order spatial distributions of intensities. In order to extract texture features, several methods were employed, including the utilization of techniques such as the gray-level co-occurrence matrix (GLCM), gray-level size zone matrix (GLSZM), gray-level run length matrix (GLRLM), and neighborhood gray-tone difference matrix (NGTDM). The Pyradiomics 2.2.0 open-source python package was used for implementing feature extraction. More information about this package can be found at http://www.radiomics.io/pyradiomics.html.
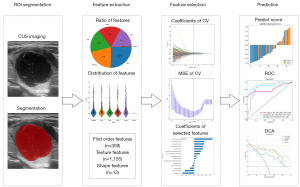
Feature selection and radiomics model establishment
To analyze our data statistically, we utilized different tests based on the distribution of the features. For features that followed a normal distribution, we conducted a Student’s t-test. On the other hand, for features that did not exhibit a normal distribution, we employed the Mann-Whitney U test. We set the significance level at 0.05 and retained only those features with a P value below this threshold. This rigorous approach allowed us to identify statistically significant features that could potentially contribute to the study findings. To ensure the reliability of our analysis, we examined the repeatability of features. For features that exhibited high repeatability, we calculated the correlation between them using Spearman’s rank correlation coefficient. If the correlation coefficient between any two features was greater than 0.9, we retained only one of them to avoid redundancy.
To further enhance the comprehensiveness of our feature set, we implemented a greedy recursive deletion strategy for feature filtering. This involved iteratively removing the feature with the highest redundancy in the current set. After this process, we retained fifteen features. In order to construct the signature, we employed the least absolute shrinkage and selection operator (LASSO) regression model on the discovery data set. The LASSO method shrinks regression coefficients towards zero based on the regularization weight λ and selectively forces coefficients of irrelevant features to be precisely zero. To determine the optimal λ, we conducted ten-fold cross-validation using minimum criteria, and selected the value of λ that resulted in the lowest cross-validation error. The features with nonzero coefficients were utilized for fitting the regression model, and subsequently combined to form a radiomics signature. To calculate the radiomics score for each patient, we computed a linear combination of the retained features, which were weighted by their respective model coefficients. The LASSO regression modeling was conducted using the Python scikit-learn package.
Following the LASSO feature screening, we selected the final set of features to be used for constructing the risk model. To achieve this, we employed the Light Gradient Boosting Machine (LightGBM) model. To ensure the reliability and generalizability of our model, we implemented five-fold cross-validation. By averaging the results across the five folds, we obtained the final radiomics signature.
The building of the clinical model and radiomics-clinical model
All of the thyroid nodules that were classified as TI-RADS 3 had well-defined margins, no calcifications, and an aspect ratio of ≤1. We just selected ultrasound features of tumor dimension, echotexture (homogeneous or heterogeneous), and echogenicity (hypoechoic, iso/hyperechoic, or mixed) to differentiate between benign thyroid nodules and PTMC. The term “tumor dimension” refers to the measurement of the largest long axis cross-section of the target thyroid nodule on the image, indicating its diameter. The term “echotexture” refers to the overall appearance and texture of an ultrasound image. It describes the patterns and characteristics observed within tissues or structures visualized through ultrasound scans, such as the level of echogenicity, homogeneity, and presence of any abnormalities or variations. In this study, we focus on investigating whether the echotexture of thyroid nodules is homogeneous or heterogeneous. In brief, the term “echogenicity” refers to the ability of a tissue or structure to reflect ultrasound waves. In this study, the echogenicity is defined based on a comparison with the surrounding parenchyma, categorized as hypoechoic, isoechoic, hyperechoic, or mixed. The process of constructing the clinical model closely resembled that of the radiomics model. The selection of features for the clinical model was based on baseline statistics, considering features with a P value <0.05. Additionally, the same machine learning model was employed in both the radiomics and clinical model building procedures. To ensure fairness in comparison, we maintained a fixed test cohort and implemented five-fold cross-validation during the process.
To efficiently evaluate the prognostic significance of the radiomics signature alongside clinical risk factors, we introduced a radiomics nomogram on the validation data set. Utilizing logistic regression analysis, the nomogram was developed by combining the radiomics signature with clinical risk factors.
Statistical analysis
In order to evaluate the comparability of patient characteristics among different cohorts, we conducted independent t-tests for normally distributed data and used Mann-Whitney U tests to express non-normally distributed data as medians (interquartile range). The Chi-squared tests were utilized to analyze the categorical variables. To assess the predictive performance of the models, we employed several evaluation metrics. First, we constructed receiver operating characteristic (ROC) curves, which illustrate the trade-off between sensitivity and specificity at various classification thresholds. We calculated the area under receiver operating curve (AUC), which serves as an indicator of the model’s discriminatory ability. Additionally, we determined the balanced specificity and sensitivity of the cut-off point that maximized the Youden index. To ensure the generalizability of the models, we evaluated their performance in both the training and test cohorts. To compare the AUC between the three models, we used the Delong test. To assess the clinical utility of the three models, decision curve analysis (DCA) was employed. We conducted all statistical analyses using SPSS (version 21.0; IBM Corp., Armonk, NY, USA), with statistical significance defined as a two-sided P value ≤0.05.
Results
Baseline characteristics of patients
A total of 130 patients with benign thyroid nodules and 91 patients with PTMC were enrolled for the study. We conducted independent sample t-tests, Mann-Whitney U tests, or Chi-squared tests, as appropriate, to compare the clinical characteristics of the patients. Tables 1,2 display the baseline characteristics of patients.
Table 1
Parameter | Malignant (n=91) | Benign (n=130) |
---|---|---|
Age (years) | 50.6±1.089 | 49.88±1.079 |
Sex | ||
Male | 24 (26.4) | 22 (16.9) |
Female | 67 (73.6) | 108 (83.1) |
Tumor diameter (mm) | 15.94±1.397 | 28.7±1.163 |
Echogenicity | ||
Hypoechoic | 61 (67.0) | 44 (33.8) |
Iso/hyperechoic | 9 (9.9) | 10 (7.7) |
Mixed | 21 (23.1) | 76 (58.5) |
Echotexture | ||
Homogeneous | 19 (20.9) | 11 (8.5) |
Heterogeneous | 72 (79.1) | 119 (91.5) |
Data presentation: the number of patients is presented as n (%), while the age and tumor diameter in ultrasound images are displayed as mean ± standard deviation.
Table 2
Parameter | Malignant (n=71) | Benign (n=105) | P value |
---|---|---|---|
Age (years) | 50.44±10.09 | 49.86±12.03 | 0.74 |
Sex | 0.28 | ||
Male | 17 (23.9) | 17 (16.2) | |
Female | 54 (76.1) | 88 (83.8) | |
Tumor diameter (mm) | 16.61±13.59 | 29.15±13.35 | <0.001 |
Echogenicity | <0.001 | ||
Hypoechoic | 48 (67.6) | 35 (33.3) | |
Iso/hyperechoic | 7 (9.9) | 8 (7.6) | |
Mixed | 16 (22.5) | 62 (59.0) | |
Echotexture | 0.009 | ||
Homogeneous | 16 (22.5) | 9 (8.6) | |
Heterogeneous | 55 (77.5) | 96 (91.4) |
Data presentation: the number of patients is presented as n (%), while the age and tumor diameter in ultrasound images are displayed as mean ± standard deviation.
Establishment and evaluation of the radiomics model
A total of 1,477 handcrafted features were extracted across six categories, comprising 309 first-order features, 13 shape features, and the remaining texture features (Figure 3A) (table available at https://cdn.amegroups.cn/static/public/tcr-23-1375-1.xlsx) provides a detailed list of the handcrafted features. To extract all handcrafted features, we utilized an in-house feature analysis program implemented in Pyradiomics. For more information on Pyradiomics, please refer to its documentation at http://pyradiomics.readthedocs.io. Figure 3B displays all features and their corresponding P value results.
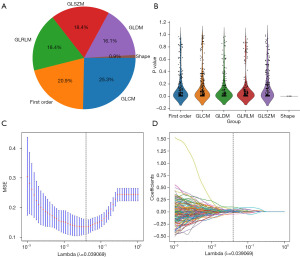
We used a LASSO logistic regression model to select the nonzero coefficients for establishing the Rad-score. Figure 3C,3D illustrates the coefficients and mean standard error (MSE) resulting from ten-fold validation. Following selection process, a total of fifteen features retained a nonzero coefficient value. The details of these features are shown in Figure 4.
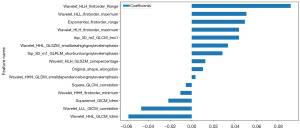
After selecting features with non-zero coefficients, we utilized the LightGBM model to analyze and construct a radiomics signature, also referred to as the radiomics score (Rad-score). Within the training cohort, the model exhibited an AUC of 0.974 [95% confidence interval (CI): 0.954–0.994], accompanied by a sensitivity of 0.901 and specificity of 0.962. In the test cohort, the AUC was 0.867 (95% CI: 0.757–0.977), and the sensitivity and specificity were 0.900 and 0.760, respectively (Figure 5, Table 3).
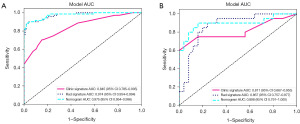
Table 3
Model | Training cohort | Test cohort | |||||
---|---|---|---|---|---|---|---|
AUC (95 % CI) | Sen | Spe | AUC (95 % CI) | Sen | Spe | ||
Clinical model | 0.845 (0.785–0.905) | 0.704 | 0.848 | 0.811 (0.667–0.955) | 0.600 | 1.000 | |
Radiomics model | 0.974 (0.954–0.994) | 0.901 | 0.962 | 0.867 (0.757–0.977) | 0.900 | 0.760 | |
Rad-clinic model | 0.975 (0.954–0.996) | 0.887 | 0.981 | 0.898 (0.791–1.000) | 0.900 | 0.840 |
Sensitivity and specificity were assessed at the cutoff value that yielded the maximum Youden index value. AUC, area under receiver operating curve; CI, confidence interval; Sen, sensitivity; Spe, specificity; Rad-clinic model, radiomics-clinical model.
Establishment and performance of the clinical model and radiomics-clinical model
We employed a significance level (P value ≤0.05) to identify the characteristics in the training cohort that would be used for constructing the clinical model. Only tumor dimension, echogenicity and echotexture met this condition, and therefore, these features were used to build the clinical model (Table 2).
In the training cohort, the clinical model demonstrated a balanced sensitivity of 0.704 and specificity of 0.848, resulting in an AUC of 0.845 (95% CI: 0.785–0.905). In the test cohort, the AUC was 0.811 (95% CI: 0.667–0.955), with a sensitivity of 0.600 and specificity of 1.000 (Figure 5, Table 3).
The radiomics-clinical model had an AUC of 0.975 (95% CI: 0.954–0.996) in the training cohort. The model exhibited a balanced sensitivity of 0.887 and a specificity of 0.981. In the test cohort, the AUC was 0.898 (95% CI: 0.791–1.000), with a sensitivity of 0.900 and a specificity of 0.840 (Figure 5, Table 3).
We constructed a nomogram based on the radiomics-clinical model (Figure 6). In this study, each model was assessed and evaluated using DCA. Figure 7 displays the decision curve analysis for the clinical model, radiomics model, and radiomics-clinical nomogram. In comparison to scenarios where no prediction model was utilized (i.e., treat-all or treat-none scheme), both the radiomics-clinical model and the radiomics model consistently exhibited significant advantages in the majority of cases (Figure 7).
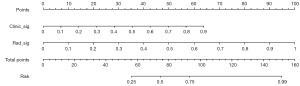
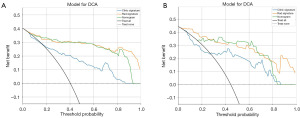
The AUC of the models was compared using the Delong test. In the test cohort, there was a statistically significant difference in AUC between the radiomics-clinical model and the clinical model (P=0.01). Nevertheless, the comparison of AUC between the radiomics model and the radiomics-clinical model in the test cohort did not yield any statistically significant difference (P=0.49).
Discussion
There has been a notable surge in the incidence of PTMC in recent years, resulting in a sharp increase in its morbidity rates. Although the surgical treatment of PTMC remains controversial, lymph node metastasis in PTMC is indisputable and the rate of metastasis is high. For instance, lymph node metastases contribute to as much as 24–64% of cases and are strongly linked to recurrence (26). The gravity of the situation demands utmost diagnostic accuracy. However, PTMC’s low diagnostic precision makes it vulnerable to frequent misdiagnosis or even overlooking (27,28). Many thyroid nodules with a TI-RADS score of 3 were pathologically confirmed as PTMC.
The rapid advancement of radiomics has created new opportunities for radiologists to evaluate tumor characteristics in a high-throughput manner. By detecting features that may not be visible to the human eye, radiomics offers the potential to provide non-invasive assessment and achieve more accurate tumor characterization. By quantifying images and analyzing the extracted information in-depth, radiomics could potentially address the diagnostic challenge of PTMC by identifying previously undetected features.
To the best of our knowledge, this is the first study to investigate the potential of CUS-based radiomics for predicting PTMC in patients with TI-RADS 3 nodules. According to univariate and multivariate analysis, this study observed associations between clinical variables, including tumor dimension, echotexture, and echogenicity, with PTMC. Subsequently, we proceeded to develop three predictive models utilizing clinical variables, radiomics features, and a fusion of both. In the validation datasets, we achieved an AUC of 0.867 with radiomics features alone in the differentiation of PTMC from TI-RADS 3 nodules. Nonetheless, the amalgamation of radiomics features with clinical variables led to enhanced performance of the radiomics-clinical model, resulting in an achieved AUC of 0.898. In contrast, the clinical model had a lower AUC value of 0.811, which was significantly different from the radiomics-clinical model. The predominant factors contributing to this phenomenon are primarily attributed to the subjective assessment and operator-dependent nature of interpreting CUS features, as well as the variation in ultrasound image quality across different machines. It is insufficient for clinical variables to distinguish PTMC from TI-RADS 3 nodules.
The study conducted by Zhang et al. revealed that ultrasound alone achieved an AUC of 0.728 in diagnosing PTMC, while Gao et al. examined the diagnostic capabilities of ultrasound-guided FNAB for PTMC, which exhibited remarkable performance with an AUC of 0.947 (29,30). Nevertheless, it entailed an invasive procedure. Our current study demonstrated that the radiomics-clinical model achieved an AUC of 0.898. These results suggest that the radiomics-clinical model could be a preferable alternative to ultrasonography alone or invasive FNAB examinations.
The aforementioned findings indicate that the radiomics-clinical model has the potential to serve as a valuable and quantitative tool for PTMC prediction. In a previous study, the diagnostic performance has been categorized into three levels based on the AUC value. These levels include low performance (AUC =0.5–0.7), moderate performance (AUC =0.7–0.9), and high performance (AUC >0.9) (31). Although both the radiomics-clinical model and radiomics model produced excellent predictive results, the combined model did not perform better than the radiomics model. It means that radiomics may be the best predictive factor for PTMC predicting. Furthermore, we constructed a nomogram derived from the radiomics-clinical model to facilitate clinical decision-making.
This research possesses several limitations that should be acknowledged. Firstly, being a retrospective study, there is a possibility of selection bias. Secondly, the study was conducted in a single center, thus warranting the need for multicenter studies with larger patient populations to validate the findings. Thirdly, in this retrospective study, we focused solely on the development of a CUS-based radiomics model. This was because the data pertaining to advanced CUS techniques like contrast enhanced ultrasound (CEUS) or ultrasound elastography were incomplete. We are confident that conducting additional studies that incorporate both radiomics and multi-modal ultrasound techniques may demonstrate enhanced diagnostic performance.
Conclusions
Building an effective machine learning model based on radiomics and clinical information for distinguishing between benign thyroid nodules and PTMC in cases with a TI-RADS score of 3 is crucial for clinical practice. Based on our findings, it can be concluded that the utilization of a radiomics approach applied to ultrasound images provides an effective means of predicting the presence of PTMC in patients presenting with thyroid nodules having a TI-RADS score of 3. Incorporating radiomics features into clinical variables can improve the accuracy of PTMC prediction compared to using clinical variables alone. To validate the significance of our findings, it is imperative to pursue further investigations on a larger and more diverse patient sample, encompassing multiple centers.
Acknowledgments
Funding: This work was supported by
Footnote
Reporting Checklist: The authors have completed the TRIPOD reporting checklist. Available at https://tcr.amegroups.com/article/view/10.21037/tcr-23-1375/rc
Data Sharing Statement: Available at https://tcr.amegroups.com/article/view/10.21037/tcr-23-1375/dss
Peer Review File: Available at https://tcr.amegroups.com/article/view/10.21037/tcr-23-1375/prf
Conflicts of Interest: All authors have completed the ICMJE uniform disclosure form (available at https://tcr.amegroups.com/article/view/10.21037/tcr-23-1375/coif). The authors have no conflicts of interest to declare.
Ethical Statement: The authors are accountable for all aspects of the work in ensuring that questions related to the accuracy or integrity of any part of the work are appropriately investigated and resolved. The study was conducted in accordance with the Declaration of Helsinki (as revised in 2013). This study was approved by institutional ethics committee of the Second Affiliated Hospital of Wenzhou Medical University (No. 2023-K-43-01) and individual consent for this retrospective analysis was waived. However, written consent was obtained from each patient before surgery or biopsy.
Open Access Statement: This is an Open Access article distributed in accordance with the Creative Commons Attribution-NonCommercial-NoDerivs 4.0 International License (CC BY-NC-ND 4.0), which permits the non-commercial replication and distribution of the article with the strict proviso that no changes or edits are made and the original work is properly cited (including links to both the formal publication through the relevant DOI and the license). See: https://creativecommons.org/licenses/by-nc-nd/4.0/.
References
- Xu D, Lv X, Wang S, et al. Risk factors for predicting central lymph node metastasis in papillary thyroid microcarcinoma. Int J Clin Exp Pathol 2014;7:6199-205. [PubMed]
- Chen AY, Jemal A, Ward EM. Increasing incidence of differentiated thyroid cancer in the United States, 1988-2005. Cancer 2009;115:3801-7. [Crossref] [PubMed]
- Ito Y, Miyauchi A, Inoue H, et al. An observational trial for papillary thyroid microcarcinoma in Japanese patients. World J Surg 2010;34:28-35. [Crossref] [PubMed]
- Tessler FN, Middleton WD, Grant EG, et al. ACR Thyroid Imaging, Reporting and Data System (TI-RADS): White Paper of the ACR TI-RADS Committee. J Am Coll Radiol 2017;14:587-95. [Crossref] [PubMed]
- Hong YJ, Son EJ, Kim EK, et al. Positive predictive values of sonographic features of solid thyroid nodule. Clin Imaging 2010;34:127-33. [Crossref] [PubMed]
- Moon WJ, Jung SL, Lee JH, et al. Benign and malignant thyroid nodules: US differentiation--multicenter retrospective study. Radiology 2008;247:762-70. [Crossref] [PubMed]
- Singh Ospina N, Brito JP, Maraka S, et al. Diagnostic accuracy of ultrasound-guided fine needle aspiration biopsy for thyroid malignancy: systematic review and meta-analysis. Endocrine 2016;53:651-61. [Crossref] [PubMed]
- Cibas ES, Alexander EK, Benson CB, et al. Indications for thyroid FNA and pre-FNA requirements: a synopsis of the National Cancer Institute Thyroid Fine-Needle Aspiration State of the Science Conference. Diagn Cytopathol 2008;36:390-9. [Crossref] [PubMed]
- Itani M, Assaker R, Moshiri M, et al. Inter-observer Variability in the American College of Radiology Thyroid Imaging Reporting and Data System: In-Depth Analysis and Areas for Improvement. Ultrasound Med Biol 2019;45:461-70. [Crossref] [PubMed]
- Araruna Bezerra de Melo R, Menis F, Calsavara VF, et al. The impact of the use of the ACR-TIRADS as a screening tool for thyroid nodules in a cancer center. Diagn Cytopathol 2022;50:18-23. [Crossref] [PubMed]
- Carmeci C, Jeffrey RB, McDougall IR, et al. Ultrasound-guided fine-needle aspiration biopsy of thyroid masses. Thyroid 1998;8:283-9. [Crossref] [PubMed]
- Hagag P, Strauss S, Weiss M. Role of ultrasound-guided fine-needle aspiration biopsy in evaluation of nonpalpable thyroid nodules. Thyroid 1998;8:989-95. [Crossref] [PubMed]
- Mittendorf EA, Tamarkin SW, McHenry CR. The results of ultrasound-guided fine-needle aspiration biopsy for evaluation of nodular thyroid disease. Surgery 2002;132:648-53; discussion 653-4. [Crossref] [PubMed]
- Kim DW, Lee EJ, Kim SH, et al. Ultrasound-guided fine-needle aspiration biopsy of thyroid nodules: comparison in efficacy according to nodule size. Thyroid 2009;19:27-31. [Crossref] [PubMed]
- Leenhardt L, Hejblum G, Franc B, et al. Indications and limits of ultrasound-guided cytology in the management of nonpalpable thyroid nodules. J Clin Endocrinol Metab 1999;84:24-8. [Crossref] [PubMed]
- Lim YC, Choi EC, Yoon YH, et al. Central lymph node metastases in unilateral papillary thyroid microcarcinoma. Br J Surg 2009;96:253-7. [Crossref] [PubMed]
- Lombardi CP, Bellantone R, De Crea C, et al. Papillary thyroid microcarcinoma: extrathyroidal extension, lymph node metastases, and risk factors for recurrence in a high prevalence of goiter area. World J Surg 2010;34:1214-21. [Crossref] [PubMed]
- Mercante G, Frasoldati A, Pedroni C, et al. Prognostic factors affecting neck lymph node recurrence and distant metastasis in papillary microcarcinoma of the thyroid: results of a study in 445 patients. Thyroid 2009;19:707-16. [Crossref] [PubMed]
- Varsavsky M, Cortés Berdonces M, Alonso G, et al. Metastatic adenopathy from a thyroid microcarcinoma: final diagnosis of a presumed paraganglioma. Endocrinol Nutr 2011;58:143-4. [Crossref] [PubMed]
- Chen J, Jin P, Song Y, et al. Auto-Segmentation Ultrasound-Based Radiomics Technology to Stratify Patient With Diabetic Kidney Disease: A Multi-Center Retrospective Study. Front Oncol 2022;12:876967. [Crossref] [PubMed]
- Limkin EJ, Sun R, Dercle L, et al. Promises and challenges for the implementation of computational medical imaging (radiomics) in oncology. Ann Oncol 2017;28:1191-206. [Crossref] [PubMed]
- Rigiroli F, Hoye J, Lerebours R, et al. CT Radiomic Features of Superior Mesenteric Artery Involvement in Pancreatic Ductal Adenocarcinoma: A Pilot Study. Radiology 2021;301:610-22. [Crossref] [PubMed]
- Jiang M, Li CL, Luo XM, et al. Radiomics model based on shear-wave elastography in the assessment of axillary lymph node status in early-stage breast cancer. Eur Radiol 2022;32:2313-25. [Crossref] [PubMed]
- Li MD, Lu XZ, Liu JF, et al. Preoperative Survival Prediction in Intrahepatic Cholangiocarcinoma Using an Ultrasound-Based Radiographic-Radiomics Signature. J Ultrasound Med 2022;41:1483-95. [Crossref] [PubMed]
- Yao F, Ding J, Hu Z, et al. Ultrasound-based radiomics score: a potential biomarker for the prediction of progression-free survival in ovarian epithelial cancer. Abdom Radiol (NY) 2021;46:4936-45. [Crossref] [PubMed]
- Cho SY, Lee TH, Ku YH, et al. Central lymph node metastasis in papillary thyroid microcarcinoma can be stratified according to the number, the size of metastatic foci, and the presence of desmoplasia. Surgery 2015;157:111-8. [Crossref] [PubMed]
- Ito Y, Tomoda C, Uruno T, et al. Papillary microcarcinoma of the thyroid: how should it be treated? World J Surg 2004;28:1115-21. [Crossref] [PubMed]
- Li B, Zhang Y, Yin P, et al. Ultrasonic features of papillary thyroid microcarcinoma coexisting with a thyroid abnormality. Oncol Lett 2016;12:2451-6. [Crossref] [PubMed]
- Zhang Y, Pan J, Xu D, et al. Combination of serum microRNAs and ultrasound profile as predictive biomarkers of diagnosis and prognosis for papillary thyroid microcarcinoma. Oncol Rep 2018;40:3611-24. [Crossref] [PubMed]
- Gao L, Ma B, Zhou L, et al. The impact of presence of Hashimoto's thyroiditis on diagnostic accuracy of ultrasound-guided fine-needle aspiration biopsy in subcentimeter thyroid nodules: A retrospective study from FUSCC. Cancer Med 2017;6:1014-22. [Crossref] [PubMed]
- Xu JM, Xu XH, Xu HX, et al. Conventional US, US elasticity imaging, and acoustic radiation force impulse imaging for prediction of malignancy in thyroid nodules. Radiology 2014;272:577-86. [Crossref] [PubMed]