Potential added value of computed tomography radiomics to multimodal prediction models for benign and malignant breast tumors
Highlight box
Key findings
• Computed tomography (CT) images have potential value in predicting benign and malignant breast diseases.
What is known and what is new?
• Significant advances have been made in the application of ultrasound radiomics made significant advances in identifying benign and malignant, predicting lymph node metastasis and predicting molecular typing.
• This study investigated whether the addition of CT radiomics information can improve the efficiency of breast cancer diagnosis.
What is the implication, and what should change now?
• The use of CT radiomics can improve the diagnosis of breast cancer and warrants greater research focus.
Introduction
Breast cancer, whose incidence has increased each year over the past few decades, has become the most common malignancy in the world, and in 2020, there were more than 2.3 million newly reported cases worldwide (1). Moreover, breast cancer consistently has a high death rate among female malignancies (2). However, some research suggests that although the incidence of breast cancer has been increasing, the mortality rate has been decreasing year by year, with a decrease of 2.7 deaths per 100,000 people in the European Union over the 2002–2012 period. This is particularly true among younger women aged 20–49 years, in whom the number of deaths has decreased by 21.8% (3). This phenomenon may be attributed to the gradual increase of women’s health awareness and the advancement in medical technology, as more women are undergoing regular medical checkups. This indicates that early detection, early diagnosis, and early treatment remain the overriding principles of breast cancer prevention and treatment.
Ultrasound (US) has long been an important means of breast disease screening. It has a high resolution on soft tissue and allows for the dynamic observation of suspicious masses through the use of different sections and angles. With the additional consideration of its relatively low cost, it is one of the best methods for initial screening. The effectiveness of US has been widely recognized, as evidenced by the establishment of the Breast Imaging Reporting and Data System (BI-RADS), the systematic diagnostic criteria for conventional diagnostic imaging (4). However, there remains controversy concerning the highly subjective nature of scanning and interpretation of US images and the inability of the human eye to recognize all differences in grayscale. The addition of artificial intelligence and radiomics technology has made US results more objective and reproduceable, and it has also improved the accuracy of breast mass classification and the differentiation between benign and malignant tumors (5,6). The use of computer technology to analyze multiple examination images provides more accurate diagnosis and can further improve the efficacy of diagnosis (7).
Computed tomography (CT) examinations are being increasingly used to examine a wider array of disease types. When scans are performed for pulmonary or cardiac disease, CT often generates the first image of a breast mass and provides a wealth of diagnostic information, such as preoperative diagnosis, tumor staging, and presence of metastases. Modern CT now has improved contrast resolution, a greater field of view, and a cross-sectional capability to characterize a mass in greater detail (8). A few studies reported there to be significant correlation between the CT presentation of breast images and histopathology, with CT being particularly useful in assessing suspicious changes in dense breast tissue (9,10). Currently, the National Comprehensive Cancer Network (NCCN) for breast cancer recommends CT or positron emission tomography- CT (PET/CT) imaging for patients with stage III to IV disease and chest CT for patients with early-stage disease and pulmonary symptoms. However, PET-CT is not recommended in guidelines for routine clinical breast screening, as CT is less costly and rapidly provides results after scanning (11). This makes it possible to obtain a large number of CT images of breast masses in the clinic for further analysis. Studies have demonstrated that radiomics CT analyses can assist in predicting sentinel lymph node metastasis and in the molecular subtyping of breast cancer (12,13). However, the role of CT radiomics features in predicting benign and malignant of breast cancers has not been thoroughly investigated.
Presently, the gold standard for determining the malignancy of tumors is pathological diagnosis via biopsy. However, this necessitates a large surgical wound, which may cause psychological trauma to patients. In contrast, puncture biopsies involve a smaller wound, but their accuracy is affected by the site of the puncture. Therefore, developing a noninvasive and simple examination method has been a critical goal of clinical research.
In recent years, radiomics has been widely applied as more objective and reproducible method. In this approach, the regions of interest (ROIs) are segmented on the image pictures, and the high-throughput features of the ROIs are extracted via computer, which ultimately consist of the size, shape, texture, intensity, and other elements of the mass. These are then transformed into a high-dimensional dataset to be combined with the patient’s clinical information in the construction of a prediction model (14). Considerable progress has thus far been made in the differentiation of benign from malignant masses (5,6), the prediction of lymph node metastasis (15,16), the determination of molecular typing (17,18), and the evaluation of response to adjuvant chemotherapy (19,20). In addition to US, radiomics has also been used with magnetic resonance imaging (MRI) and X-rays in the development of various breast cancer predictive models, showing promising results in the noninvasive prediction of lesion malignancy (21,22). Based on this, the aim of this study was to investigate whether the establishment of a radiomics model composed of simultaneous US and CT images could help improve the efficacy of breast cancer diagnosis. We present this article in accordance with the TRIPOD reporting checklist (available at https://tcr.amegroups.com/article/view/10.21037/tcr-23-1042/rc).
Methods
Study population
The study was conducted in accordance with the Declaration of Helsinki (as revised in 2013) and was approved by the Institutional Review Board of the First Affiliated Hospital of Anhui Medical University (No. Quick-PJ 2023-05-35). Due to the retrospective nature of the study, informed consent was not required. Patients who were examined at our physical examination center from November 2020 to August 2021 were included and further screened based on selection criteria. The inclusion criteria were as follows: (I) definitive pathological results available; (II) simultaneous US and CT before biopsy or resection; and (III) no other surgery performed on the affected breast. The exclusion criteria were as follows: (I) incomplete pathological results; (II) anticancer therapy; and (III) image quality failing to meet the diagnostic criteria.
Finally, 420 lesions from 420 women were included, and the included patients were assigned to the training and validation groups in a 7:3 ratio.
Image acquisition
US examinations were performed using a Mindray Resona 7 (Mindray BioMedical Electronics Co., Ltd., Shenzhen, China) equipped with a high-frequency line array probe (frequency 5–12 MHz) and breast imaging condition. US focused on the center of the target mass and scanned the mass in multiple sections and angles. Grayscale images with the largest diameter and the richest vascularization were selected. A conventional chest CT scan was performed with 256-bar wide-body CT (Revolution CT, GE HealthCare, Chicago, IL, USA). The tube current was 175–545 mA, the tube voltage was 120 kVp, the noise index was 8, and the rotating speed was 0.5 s/r.
Image segmentation
The images were imported into MaZda software (version 4.6; http://www.eletel.p.lodz.pl/mazda/) for radiomics image processing. The process was as follows: (I) for image normalization, in order to ensure the repeatability of the results, the gray values of all images are normalized in the first step. In the MaZda software, µ±3σ was chosen as the standard processing method, with µ being the mean of the image gray values and σ being the standard deviation (SD) of the image gray values; (II) For ROI segmentation, the ROI segmentation was performed by two radiologists in consultation. Neither radiologist had prior knowledge of the pathology of the mass; (III) In radiomics feature extraction, automatic extraction of high-throughput features in the ROIs, such as the gray-level histogram, gray-level run-length matrix, gray-level co-occurrence matrix, and absolute gradient, was conducted using MaZda software.
Model construction
The obtained features were imported into the Dr.Wise™ scientific research platform (http://www.deepwise.com). In order to improve the performance of the prediction model and maintain its robustness, we first normalized the features to have a mean of zero, mapping from the original data to a distribution with mean of 0 and SD of 1; the normalization formula was as follows: z = (x − mean)/SD. Feature dimension reduction and screening were then completed using Pearson correlation coefficients to filter out the best features that were significantly related to the masses. The linear correlation coefficients between features and dependent variables were calculated, with the features with higher correlation coefficients being more likely to be retained. When the linear correlation coefficient between the two features was greater than a certain threshold, the one with less influence on the dependent variable was removed, and the threshold of the linear correlation coefficient was then set to 0.9. The optimal features were screened to establish the model and trained in the training group, the efficacy was tested in the validation group, and a receiver operating curve (ROC) was finally generated to evaluate the diagnostic efficacy. In this study, three machine learning algorithms, including random forest (RF), support vector machine (SVM), and K-nearest neighbor (KNN), were used to establish the diagnostic model. The best model was obtained by comparing the area under the curve (AUC) of the training group and the validation group. Finally, the calibration curve was plotted to determine the stability of the prediction model, and decision curve analysis (DCA) was applied to assess its clinical application value. A flowchart of the study process is included in Figure 1.
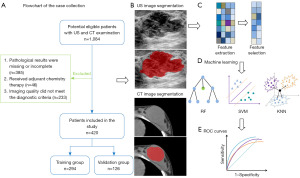
Statistical analysis
Statistical analysis was conducted using SPSS 25.0 (IBM Corp., Armonk, NY, USA). Quantitative data, such as patient, and age are expressed as the mean ± SD. Categorical variables are expressed as counts and percentages. If the quantitative data conformed to a normal distribution, the independent samples t-test was used to assess statistical significance (P<0.05). If the data did not meet the normality criteria, the Mann-Whitney test was applied. The data for the number of masses are expressed as counts and percentages, and the χ2 test was used for comparison between groups. The ROC curve, calibration curve, and decision analysis curve were drawn using R software (version 3.6.1; http://www.r.project.org).
Results
Basic information
A total of 420 patients with 420 biopsy-proven lesions were included in the study, with a mean age of 41.7±11.8 years (range, 19–82 years). Among them, 294 were benign cases (mean age 37.5±9.3 years; range, 19–67 years) and 126 were malignant cases (mean age 51.3±11.3 years; range, 28–82 years). The differences between the ages of the two groups were significant (P=0.047). The ratio between the training group and the validation group was 7:3, with 294 patients in the training group and 126 patients in the validation group. There was no statistical difference in age between these groups (P=0.741). In the training and validation groups, 29.9% (88/294) and 30.2% (38/126) of patients developed malignancy, respectively, with no significant difference in the presence of malignancy between the two groups (P=0.963) (Table 1).
Table 1
Characteristic | Training group (n=294) | Validation group (n=126) | P value |
---|---|---|---|
Age (years), mean ± SD | 41.8±11.5 | 43.6±11.6 | 0.741 |
Lesion pathology, n (%) | 0.963 | ||
Benign | 206 (70.1) | 88 (69.8) | |
Malignant | 88 (29.9) | 38 (30.2) |
SD, standard deviation.
Radiomics features
Each breast mass was manually segmented with MaZda software, resulting in a total of 379 features extracted from the US images and 371 features from the CT images, with the combined group consisting of 2 combinations of a total of 750 features. After screening, 8 optimal features eventually remained in the US group, while 12 features (6 US and 6 CT features) remained in the combined group. The feature importance of both groups is shown in Figure 2. The differences in radiomics features between benign and malignant lesions of the combined group are shown in Table 2 and the boxplot in Figure 3.
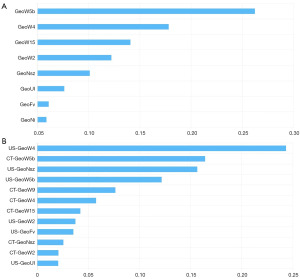
Table 2
Feature† | Classification of lesions | Mean ± SD | P value |
---|---|---|---|
US-GeoW4 | B | 2.837±0.881 | <0.001* |
M | 3.122±0.230 | ||
CT-GeoW5b | B | 0.139±0.057 | <0.001* |
M | 0.066±0.035 | ||
US-GeoNsz | B | 1,947.293±982.945 | <0.001* |
M | 3,950.912±1,333.810 | ||
US-GeoW5b | B | 0.082±0.040 | <0.001* |
M | 0.035±0.017 | ||
CT-GeoW9 | B | 1.306±0.071 | <0.001* |
M | 1.421±0.099 | ||
CT-GeoW4 | B | 2.733±0.062 | <0.001* |
M | 2.922±0.146 | ||
CT-GeoW15 | B | 0.947±0.049 | 0.001* |
M | 0.866±0.601 | ||
US-GeoW2 | B | 0.098±0.172 | 0.186 |
M | 0.078±0.015 | ||
US-GeoFv | B | 466.177±187.512 | 0.555 |
M | 676.476±198.121 | ||
CT-GeoNsz | B | 453.663±264.924 | <0.001* |
M | 881.405±478.908 | ||
CT-GeoW2 | B | 0.117±0.011 | 0.005 |
M | 0.095±0.014 | ||
US-GeoUl | B | 5,740.925±2,242.857 | 0.433 |
M | 8,506.049±2,126.892 |
*, P<0.05. †, feature ultimately selected in the combined group, in order of importance from highest to lowest. B and M represent benign and malignant labels, respectively. SD, standard deviation; US, ultrasound; CT, computed tomography.
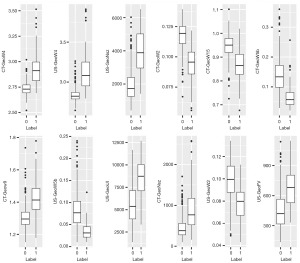
Diagnostic efficacy of the radiomics models
Three learners were used for the prediction models and the ROC curves for the US and combined groups: RF, KNN, and SVM (Figure 4). In the US group, SVM had the highest AUC value out of all the prediction models in the training group, while RF had the highest AUC value in the validation group. In the combined group, RF had the highest AUC values among all the models in the training and validation groups, so RF was selected as the optimal model. Tables 3,4 were plotted based on sensitivity, specificity, and other measures.
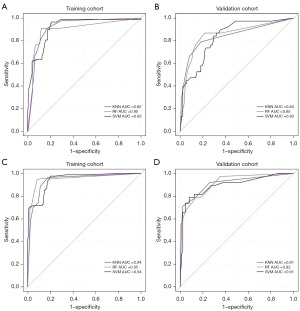
Table 3
Model | Group | AUC (95% CI) | Accuracy | Sensitivity | Specificity | PPV | NPV |
---|---|---|---|---|---|---|---|
RF | Training | 0.90 (0.852–0.942) | 0.85 | 0.77 | 0.89 | 0.75 | 0.90 |
Validation | 0.85 (0.775–0.930) | 0.82 | 0.58 | 0.92 | 0.76 | 0.84 | |
KNN | Training | 0.92 (0.885–0.952) | 0.87 | 0.82 | 0.89 | 0.76 | 0.92 |
Validation | 0.84 (0.760–0.925) | 0.84 | 0.66 | 0.92 | 0.78 | 0.86 | |
SVM | Training | 0.93 (0.907–0.959) | 0.79 | 0.65 | 0.85 | 0.65 | 0.85 |
Validation | 0.83 (0.757–0.906) | 0.73 | 0.53 | 0.82 | 0.56 | 0.80 |
US, ultrasound; AUC, area under the curve; CI, confidence interval; PPV, positive predictive value; NPV, negative predictive value; RF, random forest; KNN, K-nearest neighbor; SVM, support vector machine.
Table 4
Model | Group | AUC (95% CI) | Accuracy | Sensitivity | Specificity | PPV | NPV |
---|---|---|---|---|---|---|---|
RF | Training | 0.95 (0.918–0.981) | 0.91 | 0.85 | 0.94 | 0.85 | 0.94 |
Validation | 0.92 (0.866–0.969) | 0.86 | 0.71 | 0.92 | 0.79 | 0.88 | |
KNN | Training | 0.94 (0.909–0.970) | 0.85 | 0.73 | 0.91 | 0.77 | 0.89 |
Validation | 0.91 (0.852–0.971) | 0.88 | 0.74 | 0.94 | 0.85 | 0.89 | |
SVM | Training | 0.94 (0.918–0.970) | 0.81 | 0.72 | 0.85 | 0.68 | 0.88 |
Validation | 0.91 (0.850–0.966) | 0.87 | 0.74 | 0.93 | 0.82 | 0.89 |
AUC, area under the curve; PPV, positive predictive value; NPV, negative predictive value; RF, random forest; KNN, K-nearest neighbor; SVM, support vector machine.
When only US image information was used, the optimal model RF had an AUC of 0.90 [95% confidence interval (CI): 0.852–0.942], an accuracy of 0.85, a sensitivity of 0.77, and a specificity of 0.89 for the training group; and an AUC of 0.85 (95% CI: 0.775–0.930), an accuracy of 0.82, a sensitivity of 0.58, and a specificity of 0.92 for the validation group. After the addition of CT image information, the AUC of the combined optimal RF model increased to 0.95 (95% CI: 0.918–0.981) in the training group, with an accuracy of 0.91, a sensitivity of 0.85, and a specificity of 0.94; meanwhile, in the validation group, the AUC was 0.92 (95% CI: 0.866–0.969), with an accuracy of 0.86, a sensitivity of 0.71, and a specificity of 0.92. CT radiomics features provided more valuable information, allowing for a substantial increase in the accuracy, specificity, and especially the sensitivity of the prediction model. Figure 5 shows the waterfall plot for distinguishing benign and malignant masses based on the radiomics score of US and the combined group, which visually demonstrates the good discrimination performance of the prediction model.
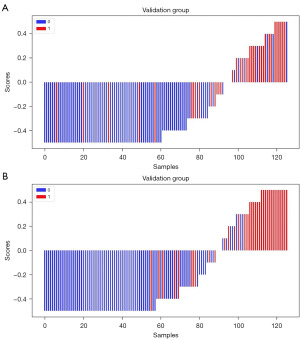
Evaluation of the radiomics models
The ROC calibration curves of the optimal learner for the training and validation cohorts in the combined group are shown in Figure 6. The calibration curve shows that the combined model fit well and that there was good concordance between the prediction of malignant mass and the actual pathology.
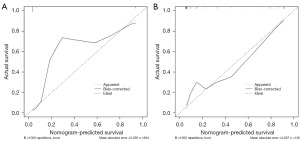
The decision analysis curves of CT, US, and the combined method are shown in Figure 7. The black solid line represents the assumption that all patients are included in the malignant group, and the gray solid line represents the assumption that all patients are included in the benign group. The decision curve shows that the net clinical benefit of the combined method is much greater than that of any single examination and that the prediction model of the combined group has certain clinical value.
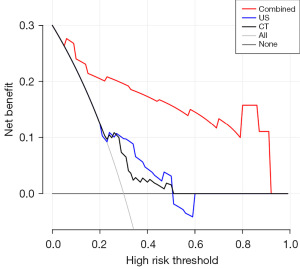
Discussion
In this study, the combined diagnostic model composed of US and CT images was shown capable of differentiating between benign and malignant breast masses. We established a radiomics model with 420 cases, the ROC curve showed its excellent diagnostic efficiency—which was corroborated by the measures such as sensitivity and specificity—and its clinical application was also proven.
In recent years, the study of breast radiomics has made rapid progress. Radiomics features reproduce the texture features of the mass based on the assumption that the extracted features are derived from products at the molecular genetic level, which in turn correlates with the phenotypic characteristics of the tissue (23). Previous studies have revealed that image information is highly correlated with the biological behavior of the mass (17,18,24). The high heterogeneity of breast cancer mass provides the possibility for the study of radiomics, which has the advantages of stability, objectivity, and repeatability that many clinicians do not have and thus can be used as a supplementary diagnostic method to improve their diagnostic capability. Our study population was relatively large, and the study images were all from the same modality on the same type of machine, thus ensuring the stability of the imaging data. We then performed normalized preprocessing on the gray values of all the images to reduce the differences between different images and make it easier for the machine to learn the features, thus improving the robustness and accuracy of the model (14). Eventually, we filtered out the stable, relevant, and non-redundant features in the model for final validation.
US is one of the most important means of diagnosing breast benign and malignant masses, and the BI-RADS score is currently the most widely used diagnostic guideline. Youk et al. investigated the diagnostic efficacy of the human eye for breast US using the fifth edition of BI-RADS as a standard, achieving an AUC of 0.80 (25). The diagnostic efficacy of the human eye is low. Luo et al. extracted 315 features of US images of breast masses using radiomics and performed logistic regression analysis. The AUC value of the benign and malignant prediction model in the training group was 0.83, and when combined with BI-RADS classification and plotted with a nomogram, the predictive performance improved to 0.93 (26). Sultan et al. studied the grayscale and Doppler US images of 160 breast masses and established a prediction model by using a logistic regression classifier. The ROC curve area for grayscale image analysis was 0.85 and increased to 0.89 with the addition of Doppler image feature information (7). Romeo et al. built a benign and malignant diagnostic model by applying US radiomics; in this study, 135 lesions from institution 1 were placed into the training and test groups, 66 lesions from institution 2 were used as external validation, and RF classifiers were used to build the model and plot ROC curves; the AUC values for the training and validation group were 0.90 and 0.82, respectively, and the accuracy of the validation group was 0.82 (27). This fits well with our US radiomics–only results (AUCs of 0.90 and 0.85 for the training and validation groups, respectively, and an accuracy of 0.82 for the validation group). In our study, we used radiomics to establish a diagnostic model based on CT and US features. The combined model greatly improved the diagnostic efficacy as compared to direct use of the BI-RADS by clinicians. Caballo et al. used 202 cases to develop and evaluate multimarker quantitative radiomics, quantified the morphological characteristics of breast masses, extracted various features of the edges of the tumors, and built a prediction model with a linear discriminant analysis classifier, which obtained high CT-based diagnostic efficacy of benign and malignant breast masses, with a final prediction model AUC of 0.90 (28). This suggests that CT images contain a wealth of valuable diagnostic information that should be utilized. In our RF model for the US method, the sensitivity of the training and validation groups was low at 0.77 and 0.58, respectively, but improved to 0.85 and 0.71 after the addition of CT features. The specificity and accuracy also improved to a relatively satisfactory degree, ensuring higher diagnostic efficacy of the combined method. Increased sensitivity allows for a greater of number of malignant masses to be identified at an early stage, while increased specificity reduces the probability of biopsy in healthy patients.
The AUC value in the ROC curve only represents on the overall accuracy of the model, while the decision curve represents the relationship between benefits and risks brought by the model. In our study, the decision curve evaluated the clinical value of the CT, US, and the combined method separately and showed that the net benefit of the combined method was significantly higher than that of any single test. This further confirms that combining existing CT images can improve the effectiveness of individual clinical decision-making.
We further analyzed the role of radiomics features in the predictive model in this study and examined them in the context of clinical application, allowing us to interpret the clinical significance of the model. US-GeoW4 is one of the most important and discriminative features of the combined group, and its mathematical definition is calculated as follows: US-GeoW4 = US-GeoUl/US-GeoUw, where GeoUl represents the profile specific perimeter, which is the length of the contour of the ROI of the mass segmented by the clinician, and GeoUw represents the perimeter of the convex hull. Thus, we first need to clarify the simplified concept of the convex hull: in a given two-dimensional planar point set, a convex hull is a convex polygon formed by the straight lines connecting the outermost points, which can contain all the points in the point set, and the perimeter of this convex polygon is the perimeter of the convex hull. In two-dimensional Euclidean space, the convex hull can be imagined as a rubber band that just wraps around all the points. The ratio between the two was calculated, and finally it was found that high values of US-GeoW4 tended toward the “malignant” class, while low US-GeoW4 values tended toward the “benign” class. We hypothesized that for the same GeoUw, the more zigzag there is in the contour of the figure within the convex hull, the longer the GeoUl, leading to a tendency for US-GeoW4 to be high (malignant class); conversely, the smoother the contour is, the shorter the GeoUl, leading to a tendency for US-GeoW4 to be low (benign class). Indeed, the zigzagging contours, such as the burr sign, correspond precisely to the irregular morphology of malignant masses in clinical decision-making (29).
In this study, we analyzed US and CT images taken concurrently at the time of physical examination for the noninvasive assessment of benign and malignant breast masses without the need for additional radiation exposure and financial cost to the patients. CT images of breast patients could also be used in the future as a complementary tool for wider use in clinical or physical examination.
The advantages of this study are that, first, we included a relatively large number of cases and more consistent results. Second, this study is novel in that no other research has yet focused on the combined analysis of CT and US images to improve the diagnostic efficacy of breast cancer.
There are several limitations to this study. First, as a retrospective study, bias is inevitable. Second, this is a single-center study and lacks external data for verification. Third, the images obtained in this retrospective study were from different operators, which might have introduced a personal subjective element. Fourth, the delineation methods in this study were all manual segmentation by physicians, which was time-consuming and labor-intensive. In future studies, semiautomatic segmentation can be adopted to delineate ROIs, as it has greater repeatability and stability than do features extracted by manual segmentation (30).
Conclusions
The combined predictive model composed of US and CT images can be used as a personalized predictive method for the diagnosis of benign and malignant breast diseases, and this noninvasive predictive method can be an important supplement to biopsy. This suggests that we should maximize use of the patients’ imaging information and should not overlook the potential analytical value of CT images for early medical intervention to prolong the patient’s survival time.
Acknowledgments
Funding: None.
Footnote
Reporting Checklist: The authors have completed the TRIPOD reporting checklist. Available at https://tcr.amegroups.com/article/view/10.21037/tcr-23-1042/rc
Data Sharing Statement: Available at https://tcr.amegroups.com/article/view/10.21037/tcr-23-1042/dss
Peer Review File: Available at https://tcr.amegroups.com/article/view/10.21037/tcr-23-1042/prf
Conflicts of Interest: All authors have completed the ICMJE uniform disclosure form (available at https://tcr.amegroups.com/article/view/10.21037/tcr-23-1042/coif). The authors have no conflicts of interest to declare.
Ethical Statement: The authors are accountable for all aspects of the work in ensuring that questions related to the accuracy or integrity of any part of the work are appropriately investigated and resolved. The study was conducted in accordance with the Declaration of Helsinki (as revised in 2013) and was approved by the Institutional Review Board of the First Affiliated Hospital of Anhui Medical University (No. Quick-PJ 2023-05-35). Due to the retrospective nature of the study, informed consent was not required.
Open Access Statement: This is an Open Access article distributed in accordance with the Creative Commons Attribution-NonCommercial-NoDerivs 4.0 International License (CC BY-NC-ND 4.0), which permits the non-commercial replication and distribution of the article with the strict proviso that no changes or edits are made and the original work is properly cited (including links to both the formal publication through the relevant DOI and the license). See: https://creativecommons.org/licenses/by-nc-nd/4.0/.
References
- Sung H, Ferlay J, Siegel RL, et al. Global Cancer Statistics 2020: GLOBOCAN Estimates of Incidence and Mortality Worldwide for 36 Cancers in 185 Countries. CA Cancer J Clin 2021;71:209-49. [Crossref] [PubMed]
- Siegel RL, Miller KD, Jemal A. Cancer statistics, 2020. CA Cancer J Clin 2020;70:7-30. [Crossref] [PubMed]
- Carioli G, Malvezzi M, Rodriguez T, et al. Trends and predictions to 2020 in breast cancer mortality in Europe. Breast 2017;36:89-95. [Crossref] [PubMed]
- Burnside ES, Sickles EA, Bassett LW, et al. The ACR BI-RADS experience: learning from history. J Am Coll Radiol 2009;6:851-60. [Crossref] [PubMed]
- Xu Z, Wang Y, Chen M, et al. Multi-region radiomics for artificially intelligent diagnosis of breast cancer using multimodal ultrasound. Comput Biol Med 2022;149:105920. [Crossref] [PubMed]
- Youk JH, Kwak JY, Lee E, et al. Grayscale Ultrasound Radiomic Features and Shear-Wave Elastography Radiomic Features in Benign and Malignant Breast Masses. Ultraschall Med 2020;41:390-6. [Crossref] [PubMed]
- Sultan LR, Schultz SM, Cary TW, et al. Machine learning to improve breast cancer diagnosis by multimodal ultrasound. IEEE Int Ultrason Symp 2018; [Crossref] [PubMed]
- Harish MG, Konda SD, MacMahon H, et al. Breast lesions incidentally detected with CT: what the general radiologist needs to know. Radiographics 2007;27:S37-51. [Crossref] [PubMed]
- Fujita T, Doihara H, Takabatake D, et al. Multidetector row computed tomography for diagnosing intraductal extension of breast carcinoma. J Surg Oncol 2005;91:10-6. [Crossref] [PubMed]
- Georgieva M, Rennert J, Brochhausen C, et al. Suspicious breast lesions incidentally detected on chest computer tomography with histopathological correlation. Breast J 2021;27:715-22. [Crossref] [PubMed]
- Gradishar WJ, Anderson BO, Balassanian R, et al. NCCN Guidelines Insights Breast Cancer, Version 1.2016. J Natl Compr Canc Netw 2015;13:1475-85. [Crossref] [PubMed]
- Yang X, Wu L, Ye W, et al. Deep Learning Signature Based on Staging CT for Preoperative Prediction of Sentinel Lymph Node Metastasis in Breast Cancer. Acad Radiol 2020;27:1226-33. [Crossref] [PubMed]
- Fan Y, Pan X, Yang F, et al. Preoperative Computed Tomography Radiomics Analysis for Predicting Receptors Status and Ki-67 Levels in Breast Cancer. Am J Clin Oncol 2022;45:526-33. [Crossref] [PubMed]
- Papanikolaou N, Matos C, Koh DM. How to develop a meaningful radiomic signature for clinical use in oncologic patients. Cancer Imaging 2020;20:33. [Crossref] [PubMed]
- Zhou WJ, Zhang YD, Kong WT, et al. Preoperative prediction of axillary lymph node metastasis in patients with breast cancer based on radiomics of gray-scale ultrasonography. Gland Surg 2021;10:1989-2001. [Crossref] [PubMed]
- Zha HL, Zong M, Liu XP, et al. Preoperative ultrasound-based radiomics score can improve the accuracy of the Memorial Sloan Kettering Cancer Center nomogram for predicting sentinel lymph node metastasis in breast cancer. Eur J Radiol 2021;135:109512. [Crossref] [PubMed]
- Liu J, Wang X, Hu M, et al. Development of an ultrasound-based radiomics nomogram to preoperatively predict Ki-67 expression level in patients with breast cancer. Front Oncol 2022;12:963925. [Crossref] [PubMed]
- Xu A, Chu X, Zhang S, et al. Development and validation of a clinicoradiomic nomogram to assess the HER2 status of patients with invasive ductal carcinoma. BMC Cancer 2022;22:872. [Crossref] [PubMed]
- Bhardwaj D, Dasgupta A, DiCenzo D, et al. Early Changes in Quantitative Ultrasound Imaging Parameters during Neoadjuvant Chemotherapy to Predict Recurrence in Patients with Locally Advanced Breast Cancer. Cancers (Basel) 2022;14:1247. [Crossref] [PubMed]
- Yang M, Liu H, Dai Q, et al. Treatment Response Prediction Using Ultrasound-Based Pre-, Post-Early, and Delta Radiomics in Neoadjuvant Chemotherapy in Breast Cancer. Front Oncol 2022;12:748008. [Crossref] [PubMed]
- Militello C, Rundo L, Dimarco M, et al. 3D DCE-MRI Radiomic Analysis for Malignant Lesion Prediction in Breast Cancer Patients. Acad Radiol 2022;29:830-40. [Crossref] [PubMed]
- Lei C, Wei W, Liu Z, et al. Mammography-based radiomic analysis for predicting benign BI-RADS category 4 calcifications. Eur J Radiol 2019;121:108711. [Crossref] [PubMed]
- Limkin EJ, Sun R, Dercle L, et al. Promises and challenges for the implementation of computational medical imaging (radiomics) in oncology. Ann Oncol 2017;28:1191-206. [Crossref] [PubMed]
- Bene I, Ciurea AI, Ciortea CA, et al. Radiomic Signatures Derived from Hybrid Contrast-Enhanced Ultrasound Images (CEUS) for the Assessment of Histological Characteristics of Breast Cancer: A Pilot Study. Cancers (Basel) 2022;14:3905. [Crossref] [PubMed]
- Youk JH, Jung I, Yoon JH, et al. Comparison of Inter-Observer Variability and Diagnostic Performance of the Fifth Edition of BI-RADS for Breast Ultrasound of Static versus Video Images. Ultrasound Med Biol 2016;42:2083-8.
- Luo WQ, Huang QX, Huang XW, et al. Predicting Breast Cancer in Breast Imaging Reporting and Data System (BI-RADS) Ultrasound Category 4 or 5 Lesions: A Nomogram Combining Radiomics and BI-RADS. Sci Rep 2019;9:11921. [Crossref] [PubMed]
- Romeo V, Cuocolo R, Apolito R, et al. Clinical value of radiomics and machine learning in breast ultrasound: a multicenter study for differential diagnosis of benign and malignant lesions. Eur Radiol 2021;31:9511-9. [Crossref] [PubMed]
- Caballo M, Pangallo DR, Sanderink W, et al. Multi-marker quantitative radiomics for mass characterization in dedicated breast CT imaging. Med Phys 2021;48:313-28. [Crossref] [PubMed]
- Spak DA, Plaxco JS, Santiago L, et al. BI-RADS(®) fifth edition: A summary of changes. Diagn Interv Imaging 2017;98:179-90.
- Parmar C, Rios Velazquez E, Leijenaar R, et al. Robust Radiomics feature quantification using semiautomatic volumetric segmentation. PLoS One 2014;9:e102107. [Crossref] [PubMed]