Comprehensive analysis of m6A RNA methylation regulators in esophageal carcinoma
Highlight box
Key findings
• This study established and validated a strong prognostic signature based on N6-methyladenosine (m6A) regulators.
What is known and what is new?
• m6A modification plays an important role in tumorigenesis, cell proliferation and the tumor microenvironment in various malignancies.
• This study constructed a m6A score system to improve the predictive value of a single m6A regulator, and evaluated the correlation of m6A regulators and immune cell infiltration in the tumor immune microenvironment.
What is the implication, and what should change now?
• m6A regulators can serve as potential prognostic biomarkers and promising therapeutic targets against immunotherapy.
Introduction
Esophageal carcinoma (ESCA) is a highly aggressive digestive malignancy, with the seventh highest incidence and sixth highest mortality rate worldwide (1,2). According to the National Central Cancer Registry of China (NCCR) statistics, there were approximately 478,000 newly diagnosed ESCA cases in China, which represents a threat to public health (3). The main histological subtypes can be classified into squamous cell carcinoma and adenocarcinoma based on the location of the tumor (4,5). It is challenging to choose an appropriate therapeutic schedule for curing the disease. Radical esophagectomy is the standard therapy for ESCA without metastatic disease. Neoadjuvant therapy with chemotherapy or chemoradiotherapy has been utilized as an effective supplemental treatment option to cure ESCA (6). Despite immense improvements in diagnosis and therapy, the prognosis of patients with ESCA remains pessimistic (7). The 5-year survival rate is between 20% and 30% (8,9). Although the TNM staging system (T: extent of the tumor; N: extent of spread to the lymph nodes; M: presence of metastasis) is the most common indicator for prognostic prediction, it is of great importance to develop additional powerful prognostic predictors for precise individualized treatment.
N6-methyladenosine (m6A) modification is one of the most pervasive modifications of RNA methylation in eukaryotic cells and is a dynamic and reversible process involved in RNA splicing, nuclear transport, stability and translation (10,11). It is well known that m6A modification relevant to messenger RNA (mRNA) and noncoding RNA (ncRNA) regulates basic biological processes (BP) and pathological functions, such as cell differentiation, development and tumorigenesis (12,13). Recently, with the continuous development of high-throughput sequencing and tumor epigenetics, m6A modification has gained increasing attention. The m6A modification is regulated by a variety of regulators, such as methyltransferases (writers), demethylases (erasers), and binding proteins (readers). The identification of these m6A regulators brings a new dawn for investigating RNA modification biology (11,14,15). m6A modification is installed enzymatically by a methyltransferase complex comprising writers, such as METTL3 and WTAP. It can be removed by two demethylases, ALKBH5 and FTO (16-18). m6A can be identified by various readers that implement regulatory functions by selectively recognizing methylated RNA (19). Increasing evidence has demonstrated that m6A modification plays an important role in tumorigenesis, cell proliferation and the tumor microenvironment in various malignancies, such as breast cancer, prostate cancer, hepatocellular cancer, thyroid cancer and lung cancer (20-26). A previous study reported that high expression of WTAP (one of the “writers”) leads to poor prognosis of gastric cancer by influencing T-lymphocyte infiltration, suggested that the m6A modification pattern was significantly associated with the tumor immune microenvironment (TIME) (27). Zhang et al. found that m6A modification patterns are strongly related to different TIME cell-infiltrating characteristics, and the evaluation of m6A modification patterns within individual tumors can predict immune infiltration and patient prognosis (28). Han et al. reported that YTHDF1 (one of the “readers”) inhibits cross-presentation by dendritic cells by recognizing and binding to m6A-tagged transcripts, thereby inhibiting anti-tumor immunity, blockade of YTHDF1 can enhance the therapeutic efficacy of immunotherapy (29). Thus, these findings suggest that the m6A modification pattern can affect prognosis and immunotherapeutic efficacy in various cancers. At present, little is known about the biological function of these m6A regulators. There are few studies on the molecular subtypes of m6A regulators in ESCA. The aim of this study was to investigate the landscape of m6A modification and establish a scoring system for accurately predicting the prognosis in ESCA, thereby prolonging the survival time.
In the present study, we downloaded transcriptomic data, clinical information and somatic mutations of ESCA from The Cancer Genome Atlas (TCGA). Copy number variations (CNVs) were obtained from the UCSC (University of California, Santa Cruz) Xena database. The differentially expressed m6A regulators between normal and tumor tissues were identified. Consensus clustering analysis was utilized to establish a scoring system based on these m6A regulators for predicting prognosis. Additionally, we investigated the correlation between m6A modification and immune cell infiltration and tumor mutation burden (TMB). Our observations indicated that m6A modification might exert an essential role in the progression of malignancy, and the prognostic signature may be considered a promising prognostic biomarker and potential therapeutic target in ESCA. We present this article in accordance with the TRIPOD reporting checklist (available at https://tcr.amegroups.com/article/view/10.21037/tcr-23-910/rc).
Methods
Data acquisition and processing
Transcriptomic data in the fragments per kilobase million (FPKM) format, somatic mutation and clinical information, including sex, depth of tumor invasion, lymphatic metastasis, distant metastasis and survival information, were downloaded from the TCGA-ESCA database (https://portal.gdc.cancer.gov/). A total of 10 normal samples and 159 tumor samples were enrolled for subsequent transcriptomic analysis. The pathological subtypes included squamous cell carcinoma (81 cases) and adenocarcinoma (78 cases). A total of 184 patients were enrolled for somatic mutation analysis. The CNV dataset was retrieved from the UCSC Xena database (https://xena.ucsc.edu/). Twenty-one well-acknowledged m6A regulators (writers including METTL3, METTL14, WTAP, VIRMA, ZC3H13, RBM15 and RBM15B; erasers including FTO and ALKBH5; readers including YTHDC1/2, YTHDF1/2/3, HNRNPC, FMR1, LRPPRC, HNRNPA2B1, IGF2BP1/2/3) were selected for subsequent research. This study was conducted in accordance with the Declaration of Helsinki (as revised in 2013).
Survival analysis
We utilized univariate Cox regression analysis and the Kaplan-Meier method to investigate the prognostic effect of candidate genes or prognostic signatures. Survival curves were plotted by the Kaplan-Meier method, and a log-rank P value of less than 0.05 was considered to be statistically significant.
Differential gene and somatic mutation analyses
The limma R package was used to identify m6A regulators that were differentially expressed between normal and tumor samples. The significance criteria were set at the cutoff value of P less than 0.05 and |fold change|=1. We employed the maftools R package to evaluate the differences in somatic mutations.
Consensus clustering analysis
Using the ConsensusClusterPlus R package, we clustered the patients with ESCA into distinct subgroups based on the profiles of 21 m6A regulators and m6A-related differentially expressed genes (DEGs). The K-means clustering method was utilized to decide the number of distinct subgroups. Additionally, we constructed a m6A score system to quantify the m6A modification pattern. All m6A-related DEGs with significant prognoses were dichotomized into two groups according to the hazard ratio with a cutoff value of 1. Then, all patients were divided into low and high m6A score groups on the basis of cumulative hazard ratios <1 or >1. The ggalluvial R package was employed to depict an alluvial diagram to show the correlation between distinct clusters.
Functional annotation
To evaluate the differences in BP between distinct m6A regulator subgroups, we performed gene set variation analysis (GSVA), gene ontology (GO) and Kyoto Encyclopedia of Genes and Genomes (KEGG) analyses. Selecting c2.cp.kegg.v7.2.symbols.gmt as the reference gene set, the GSVA R package was utilized to perform GSVA. Based on the coexpressed genes, we utilized the clusterProfiler R package to perform GO and KEGG analyses and utilized the ggplot2 R package to visualize the results.
Correlation analysis between m6A regulators and immune cell infiltration in the TIME
We detected the correlation between m6A regulators and immune cell infiltration (including B cells, CD4+ T cells, CD8+ T cells, macrophages, neutrophils, and dendritic cells) in ESCA by using the TIMER (Tumor Immune Estimation Resource) database (https://cistrome.shinyapps.io/timer/). The TISIDB (Tumor-Immune System Interactions Database; http://cis.hku.hk/TISIDB/) was employed to calculate the correlations between m6A regulators and immune regulators. Meanwhile, we employed the single-sample gene set enrichment analysis (ssGSEA) algorithm to evaluate the infiltration of 28 types of immune cells in the TIME. Additionally, we investigated the correlation between the m6A score and immune cell infiltration in the TIME.
Statistical analysis
The clinicopathological features between distinct subgroups were compared by using the chi-square test. The differences between distinct subgroups were compared by using Student’s t-test. We utilized univariate Cox regression analyses to evaluate the prognostic factors in patients with ESCA. Survival differences between distinct subgroups were compared by using the Kaplan-Meier method and log-rank test. SPSS version 21.0 (IBM Corp, Armonk, NY, USA) was employed to perform all statistical tests.
Results
Overview of m6A regulator expression in ESCA
First, we investigated the differences in 21 m6A regulators between normal and tumor tissues. The expression analysis revealed that METTL3, WTAP, VIRMA, RBM15, YTHDC1, YTHDF1/2/3, HNRNPC, FMR1, LRPPRC, HNRNPA2B1, IGF2BP1/2/3, and ALKBH5 were elevated in tumor tissues, and the remaining five m6A regulators showed no significant differences between normal and tumor tissues (Figure 1A). Using the GEPIA database by matching TCGA-ESCA normal and GTEx data, eight m6A regulators, including ZC3H13, RBM15, FTO, YTHDF1, YTHDF3, LRPPRC, IGF2BP2 and IGF2BP3, were differentially expressed in tumor tissues (Figure S1A-S1U). Compared with paired normal tissues, differentially expressed m6A regulators, including METTL3, YTHDF1, HNRNPC, FMR1, HNRNPA2B1, IGF2BP2 and IGF2BP3, were observed in tumor tissues (Figure S2A-S2U). The circle map shows the locations of CNV alterations of 21 m6A regulators on chromosomes (Figure 1B). Further analysis revealed that 21 m6A regulators in ESCA had prevalent CNV alterations. IGF2BP2, YTHDC1 and IGF2BP1 showed extensive CNV amplification. In contrast, RBM15, METTL3, HNRNPC, YTHDF2 and RBM15B had widespread CNV deletions (Figure 1C). A network map was constructed to illustrate the comprehensive landscape of the interactions between the m6A regulators and their prognostic significance in ESCA (Figure 1D). Additionally, we evaluated the prevalence of somatic mutations in 21 m6A regulators in ESCA. Among 184 samples, 23 samples (12.5%) experienced genetic mutations, mainly missense mutations and nonsense mutations. ZC3H13 had the highest mutation frequency, followed by LRPPRC (Figure 1E). We also employed correlation analysis to investigate the mutual regulation among these m6A regulators. METTL14 was significantly upregulated in ZC3H13 mutation samples (Figure 1F). However, other correlation analyses showed no apparent differences (data not shown). Survival analysis demonstrated that 9 m6A regulators were significantly associated with prognosis. Upregulation of FMR1 and YTHDF3 was remarkably related to shorter survival time, while ALKBH5, METTL14, RBM15B, YTHDC1, YTHDC2, YTHDF1 and YTHDF2 were significantly associated with prolonged survival time (Figure 2A-2I). These results revealed that m6A regulators, including writers, erasers and readers, were implicated in the m6A modification pattern, thereby simultaneously affecting the prognosis of ESCA.
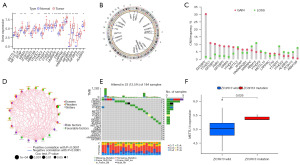
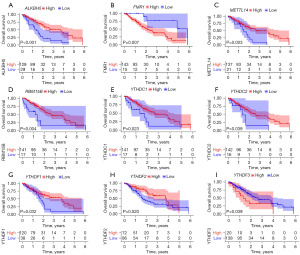
Identification of m6A modification patterns in ESCA
To evaluate the m6A modification pattern in ESCA, consensus clustering analysis was performed to stratify all samples. By using the K-means clustering method, two clusters with distinct m6A regulators were finally identified, including 47 cases in the m6A cluster A subgroup and 111 cases in the m6A cluster B subgroup (Figure 3A,3B). A heatmap of 21 m6A regulators revealed that there were apparent differences in distinct m6A cluster subgroups (Figure 3C). In addition, we performed GSVA enrichment analysis to evaluate the differences in biological function among distinct m6A cluster subgroups. The results showed that there were dramatic differences in tumor progression, such as in the PPAR signaling pathway and cell cycle (Figure 3D).
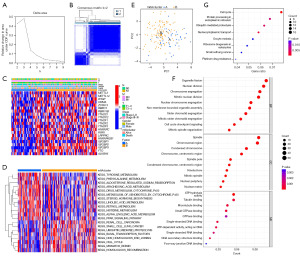
Functional annotation
Moreover, we utilized principal component analysis (PCA) to evaluate the differences among distinct m6A cluster subgroups and discovered that it was obvious to distinguish between the two m6A cluster subgroups (Figure 3E). By using univariate Cox analysis, a total of 519 DEGs between the two m6A cluster groups were identified. We performed GO enrichment and KEGG pathway analyses to evaluate the potential function. The top five BP, including organelle fission, nuclear division, chromosome segregation, mitotic nuclear division and nuclear chromosome segregation, were enriched. The category of cell components (CC) was enriched in spindle, chromosome region, condensed chromosome, centromeric region, and spindle pole. The top five enriched molecular functions (MFs) included ATP hydrolysis activity, tubulin binding, microtubule binding, small GTPase binding, and GTPase binding (Figure 3F). KEGG pathway analysis revealed that the top five processes, including the cell cycle, protein processing in the endoplasmic reticulum, ubiquitin-mediated proteolysis, nucleocytoplasmic transport and oocyte meiosis, were significantly enriched (Figure 3G).
Construction of the m6A score system
On the basis of the DEGs, we also performed consensus clustering analysis to further investigate m6A modification. By using the K-means method, two gene cluster subgroups were finally identified (Figure 4A,4B). The gene cluster A subgroup was remarkably related to m6A cluster A (Figure 4C). There were no significant differences in RBM15B and YTHDF2 among distinct gene cluster subgroups. The remaining 19 m6A regulators in the gene cluster B subgroup were significantly expressed in tumor tissues compared with normal tissues (Figure 4D). We constructed a m6A score system to subsequently evaluate the prognosis of ESCA. All patients were dichotomized into low and high m6A score subgroups. The alluvial diagram was utilized to show the correlation of distinct clusters (Figure 4E). Additionally, correlation analysis indicated that the m6A cluster A and gene cluster A subgroups were significantly associated with high m6A score (Figure 4F,4G). Between the high and low m6A score subgroups, we compared somatic tumor mutation profiles to investigate the relationship between m6A score and TMB. Somatic mutation analysis revealed that MUC16, CSMD3, ZNF804B and DYNC2H1 had more mutations in the high m6A score subgroups, while FLG and PCLO had more mutations in the low m6A score subgroups (Figure 4H,4I). Further analysis indicated that patients who died had higher m6A score (Figure 4J). On the other hand, we found that surviving patients accounted for 79% of the low m6A score subgroup (Figure 5A). Survival analysis indicated that patients in the high m6A score or high TMB subgroup experienced unfavorable prognoses (Figure 5B,5C). Survival analysis combined with TMB and m6A score demonstrated that patients in the high TMB and high m6A score subgroup had the worst prognosis (median survival time 12 months), while patients in the low TMB and low m6A score subgroup had the best prognosis (median survival time 48 months) (Figure 5D).
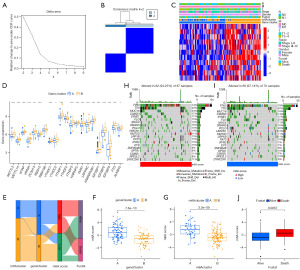
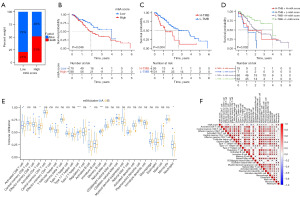
Correlation analysis between m6A regulators and immune cell infiltration
First, we investigated the correlation between m6A regulators and six types of immune cells by using the TIMER database. Our results revealed that B cells were positively associated with METTL3, METTL14, ZC3H13, RBM15, RBM15B, YTHDC1, YTHDF1 and LRPPRC. CD8+ T cells were positively related to WTAP and negatively related to RBM15B. Macrophages were positively correlated with METTL3, ZC3H13, FTO, YTHDC1, YTHDF1 and FMR1. Neutrophils were positively associated with WTAP and negatively related to LRPPRC. Dendritic cells were negatively related to VIRMA, ZC3H13, RBM15, RBM15B, YTHDF1, YTHDF3, HNRNPA2B1 and LRPPRC (Figures S3-S9). Then, we utilized the TIMER database to analyze the influence of somatic CNVs on immune cell infiltration and demonstrated that CNVs significantly affected the abundance of six types of infiltrating immune cells (Figure S10). Based on distinct m6A cluster subgroups, ssGSEA revealed that activated B cells, activated CD8 T cells and neutrophils were upregulated in the m6A cluster A subgroup. In contrast, activated CD4 T cells, type 2 T helper cells and memory B cells were reduced in the m6A cluster A group (Figure 5E). In terms of the m6A score, neutrophils and monocytes were positively correlated with the m6A score, while CD4 T cells, type 2 T helper cells and memory B cells were negatively associated with the m6A score (Figure 5F). Meanwhile, we evaluated the relationships between the abundance of 28 types of tumor-infiltrating immune cells and m6A regulators by using the TISIDB database. This observation showed that m6A regulators were significantly associated with distinct tumor-infiltrating immune cells (Figure S11A). Correlation analysis indicated that most m6A regulators were significantly related to immunoinhibitors and immunostimulators (Figure S11B,S11C). To further evaluate the effects of m6A regulators on the tumor immune response, we also analyzed the correlation between m6A regulators and major histocompatibility complex (MHC) molecules and found that WTAP and ZC3H13 were positively related to MHC molecules, and the remaining m6A regulators were negatively associated with MHC molecules (Figure S11D). A previous study conducted by Thorsson et al. revealed that tumor tissues can be divided into six immune subtypes: wound healing, IFN-gamma dominant, inflammatory, lymphocyte depleted, immunologically quiet, and TGF-b dominant (30). Our results demonstrated that HNRNPC, FMR1, LRPPRC, HNRNPA2B1, IGF2BP1 and IGF2BP2 were implicated in immune subtypes (Figure S12). METTL3, WTAP, RBM15, FTO, ALKBH5, YTHDF1, YTHDF2 and HNRNPC were significantly associated with molecular subtypes of ESCA (Figure S13). In conclusion, our studies revealed that the m6A regulators were closely related to immune cell infiltration in the tumor immune microenvironment.
Discussion
Tumorigenesis is a multistep and multifactor process that involves genetic alteration, epigenetics, posttranscriptional regulation and immune evasion (31,32). m6A is the most pervasive modification of human RNA and is dramatically correlated with various physiological and pathological processes, such as obesity and cancer (33,34). Growing evidence has revealed that abnormal expression of m6A regulators is remarkably implicated in cell proliferation, migration and invasion, thereby leading to tumorigenesis and progression (9). Until now, very few studies have focused on ESCA. Therefore, it is imperative to further investigate the underlying functional role of m6A regulators in ESCA and the relationship between m6A regulators and immune cell infiltration in the TIME. With the development of high-throughput sequencing and other biological techniques, the biological function of m6A modification in ESCA has been gradually disclosed.
In this study, we evaluated the expression pattern and prognostic significance of 21 m6A regulators and found that most m6A regulators were upregulated in ESCA tumor tissues compared with normal tissues. Survival analysis of a single m6A regulator revealed that nine genes were closely related to survival time. By using consensus clustering analysis, all included patients with ESCA were divided into two subgroups. Patients in the low TMB and low m6A score subgroup had the best prognosis. Moreover, we constructed a human m6A score system to further evaluate prognosis and immune cell infiltration. Based on the m6A score system, distinct subgroups can be completely distinguished. Additionally, we also investigated the correlations of m6A regulators and immune cell infiltration in the TIME. Dendritic cells were negatively associated with most m6A regulators. Neutrophils and monocytes were positively related to the m6A score. CD4+ T cells and type 2 T helper cells were negatively associated with the m6A score.
An increasing number of studies have demonstrated that m6A regulators have an important regulatory effect on immunity (35). Previously, the therapeutic schedule was mainly based on the TNM staging system and pathological classification. To date, with the development of research, it has been found that the status of the host immune system is closely related to the prognosis of various cancers, such as colorectal cancer, lung cancer and ESCA. The inherent aptitude of the immune system is to distinguish it from healthy self-tissues or foreign substances and to recognize and eradicate tumor cells. Nevertheless, tumor cells can escape immune surveillance by cancer immunoediting, where the immune system can hold back or accelerate tumor growth (36,37). Accumulating evidence has revealed that the TIME plays a crucial role in mediating tumor proliferation and affecting the response to immunotherapy (38). Recently, immunotherapies, including PD-1 antibodies and dendritic cell vaccines, have been used in clinical trials for patients with advanced malignancy for whom first-line chemotherapy failed (39,40). Our study demonstrated that most m6A regulators were negatively related to dendritic cells, indicating that m6A regulators contribute to dysfunction of the immune system. Dendritic cells, as robust antigen-presenting cells, take part in mediating the initiation of adaptive immune responses and innate immune responses (41).
At present, the relationship between m6A regulators and immune cell infiltration in the tumor microenvironment remains obscure. In this study, we also found that TP53 and TTN displayed the highest incidences of somatic mutations, and IGF2BP2 showed the most extensive CNV amplification. IGF2BP2, as a reader, plays a pivotal role in mRNA localization, stability and translation. A previous study demonstrated that higher expression of IGF2BP2 was observed in hepatocellular carcinoma and significantly related to unfavorable prognosis, indicating that IGF2BP2 serves as an oncogene and could promote tumor progression via a m6A-FEN1-dependent mechanism (42). Another study revealed that upregulation of IGF2BP2 facilitates lung cancer proliferation and angiogenesis (43). Meanwhile, our study found that IGF2BP2 was overexpressed in ESCA tumor tissues and closely related to immune subtypes, thereby hinting that IGF2BP2 is involved in the prognosis of ESCA. A previous study revealed that the mRNA and protein levels of METTL3 were significantly upregulated in esophageal squamous cell carcinoma (ESCC) tissues, and inhibition of METTL3 could impede tumor proliferation (44). In our study, we confirmed that METTL3 was upregulated in tumor tissue, indicating that it acts as an oncogene. Growing evidence has reported that YTHDF1 has an important role in the antitumor immune response. A study conducted by Han et al. reported that knockdown of YTHDF1 can elevate the antitumor response of CD8+ T cells and PD-L1, implicating YTHDF1 as a candidate therapeutic target in antitumor immunotherapy (29). Our research revealed that CNV of m6A regulators, including arm level again, high amplification and arm level deletion, could affect the abundance of immune cell infiltration in ESCA. This observation confirmed that m6A regulators are likely to display a vital regulatory effect on immune cell infiltration in ESCA. The role of the m6A demethylase ALKBH5 in ESCA is controversial (9). A previous study investigated the functional role of ALKBH5 in ESCC and found that upregulation of ALKBH5 was dramatically related to unfavorable prognosis. Knockdown of ALKBH5 impeded tumor cell proliferation and migration in vitro and in vivo and accumulated tumor cells in the G0/G1 phase (45). In contrast, another study reported that overexpression of ALKBH5 reduced tumor cell migration and invasion in ESCC (46). Similar to this research, other studies reported that ALKBH5 can suppress the malignancy of esophageal cancer in vivo and in vitro, indicating that ALKBH5 takes part in an inhibitory effect in ESCA (47,48).
It is noteworthy that there are several limitations in this study. First, we did not separately investigate the distinct histological types due to the small sample size. The difference between squamous cell carcinoma and adenocarcinoma could affect the consistency of our results. In addition, these results were obtained by integrated bioinformatics analyses, lacking validation in vivo and in vitro. To further verify these conclusions, subsequent studies in cells, animal models and clinical experiments need to be implemented.
Conclusions
In summary, the present study systematically investigated the expression pattern of m6A regulators, constructed a m6A score system to improve the predictive value of a single m6A regulator, and evaluated the correlation of m6A regulators and immune cell infiltration in the TIME. Our results provide important insights into the underlying role of m6A regulators and suggest that m6A regulators can serve as potential prognostic biomarkers and promising therapeutic targets against immunotherapy.
Acknowledgments
Funding: None.
Footnote
Reporting Checklist: The authors have completed the TRIPOD reporting checklist. Available at https://tcr.amegroups.com/article/view/10.21037/tcr-23-910/rc
Peer Review File: Available at https://tcr.amegroups.com/article/view/10.21037/tcr-23-910/prf
Conflicts of Interest: All authors have completed the ICMJE uniform disclosure form (available at https://tcr.amegroups.com/article/view/10.21037/tcr-23-910/coif). The authors have no conflicts of interest to declare.
Ethical Statement: The authors are accountable for all aspects of the work in ensuring that questions related to the accuracy or integrity of any part of the work are appropriately investigated and resolved. The study was conducted in accordance with the Declaration of Helsinki (as revised in 2013).
Open Access Statement: This is an Open Access article distributed in accordance with the Creative Commons Attribution-NonCommercial-NoDerivs 4.0 International License (CC BY-NC-ND 4.0), which permits the non-commercial replication and distribution of the article with the strict proviso that no changes or edits are made and the original work is properly cited (including links to both the formal publication through the relevant DOI and the license). See: https://creativecommons.org/licenses/by-nc-nd/4.0/.
References
- Sung H, Ferlay J, Siegel RL, et al. Global Cancer Statistics 2020: GLOBOCAN Estimates of Incidence and Mortality Worldwide for 36 Cancers in 185 Countries. CA Cancer J Clin 2021;71:209-49. [Crossref] [PubMed]
- Gupta B, Kumar N. Worldwide incidence, mortality and time trends for cancer of the oesophagus. Eur J Cancer Prev 2017;26:107-18. [Crossref] [PubMed]
- Chen W, Zheng R, Baade PD, et al. Cancer statistics in China, 2015. CA Cancer J Clin 2016;66:115-32. [Crossref] [PubMed]
- Abnet CC, Arnold M, Wei WQ. Epidemiology of Esophageal Squamous Cell Carcinoma. Gastroenterology 2018;154:360-73. [Crossref] [PubMed]
- Yuan Z, Wang X, Geng X, et al. Liquid biopsy for esophageal cancer: Is detection of circulating cell-free DNA as a biomarker feasible? Cancer Commun (Lond) 2021;41:3-15. [Crossref] [PubMed]
- de Gouw DJJM, Klarenbeek BR, Driessen M, et al. Detecting Pathological Complete Response in Esophageal Cancer after Neoadjuvant Therapy Based on Imaging Techniques: A Diagnostic Systematic Review and Meta-Analysis. J Thorac Oncol 2019;14:1156-71. [Crossref] [PubMed]
- van Rossum PSN, Mohammad NH, Vleggaar FP, et al. Treatment for unresectable or metastatic oesophageal cancer: current evidence and trends. Nat Rev Gastroenterol Hepatol 2018;15:235-49. [Crossref] [PubMed]
- Abbas G, Krasna M. Overview of esophageal cancer. Ann Cardiothorac Surg 2017;6:131-6. [Crossref] [PubMed]
- Zhang X, Lu N, Wang L, et al. Recent advances of m(6)A methylation modification in esophageal squamous cell carcinoma. Cancer Cell Int 2021;21:421. [Crossref] [PubMed]
- Zhang C, Fu J, Zhou Y. A Review in Research Progress Concerning m6A Methylation and Immunoregulation. Front Immunol 2019;10:922. [Crossref] [PubMed]
- Gu C, Shi X, Dai C, et al. RNA m(6)A Modification in Cancers: Molecular Mechanisms and Potential Clinical Applications. Innovation (Camb) 2020;1:100066. [Crossref] [PubMed]
- Dominissini D, Moshitch-Moshkovitz S, Schwartz S, et al. Topology of the human and mouse m6A RNA methylomes revealed by m6A-seq. Nature 2012;485:201-6. [Crossref] [PubMed]
- Yang L, Liu X, Song L, et al. Melatonin restores the pluripotency of long-term-cultured embryonic stem cells through melatonin receptor-dependent m6A RNA regulation. J Pineal Res 2020;69:e12669. [Crossref] [PubMed]
- Meyer KD, Saletore Y, Zumbo P, et al. Comprehensive analysis of mRNA methylation reveals enrichment in 3' UTRs and near stop codons. Cell 2012;149:1635-46. [Crossref] [PubMed]
- Shi H, Wei J, He C. Where, When, and How: Context-Dependent Functions of RNA Methylation Writers, Readers, and Erasers. Mol Cell 2019;74:640-50. [Crossref] [PubMed]
- Li XC, Jin F, Wang BY, et al. The m6A demethylase ALKBH5 controls trophoblast invasion at the maternal-fetal interface by regulating the stability of CYR61 mRNA. Theranostics 2019;9:3853-65. [Crossref] [PubMed]
- Zhao BS, Roundtree IA, He C. Post-transcriptional gene regulation by mRNA modifications. Nat Rev Mol Cell Biol 2017;18:31-42. [Crossref] [PubMed]
- Wang T, Kong S, Tao M, et al. The potential role of RNA N6-methyladenosine in Cancer progression. Mol Cancer 2020;19:88. [Crossref] [PubMed]
- Luo J, Liu H, Luan S, et al. Aberrant Regulation of mRNA m6A Modification in Cancer Development. Int J Mol Sci 2018;19:2515. [Crossref] [PubMed]
- Wei J, Fang DL, Zhou W, et al. N6-methyladenosine (m6A) regulatory gene divides hepatocellular carcinoma into three subtypes. J Gastrointest Oncol 2021;12:1860-72. [Crossref] [PubMed]
- Zhang Z, Zhang C, Luo Y, et al. m(6)A regulator expression profile predicts the prognosis, benefit of adjuvant chemotherapy, and response to anti-PD-1 immunotherapy in patients with small-cell lung cancer. BMC Med 2021;19:284. [Crossref] [PubMed]
- Liu F, Yu X, He G. m6A-Mediated Tumor Invasion and Methylation Modification in Breast Cancer Microenvironment. J Oncol 2021;2021:9987376. [Crossref] [PubMed]
- Xu N, Chen J, He G, et al. Prognostic values of m6A RNA methylation regulators in differentiated Thyroid Carcinoma. J Cancer 2020;11:5187-97. [Crossref] [PubMed]
- Yu ZH, Feng ST, Zhang D, et al. The functions and prognostic values of m6A RNA methylation regulators in thyroid carcinoma. Cancer Cell Int 2021;21:385. [Crossref] [PubMed]
- Zhang Q, Luan J, Song L, et al. Malignant Evaluation and Clinical Prognostic Values of M6A RNA Methylation Regulators in Prostate Cancer. J Cancer 2021;12:3575-86. [Crossref] [PubMed]
- Dai F, Wu Y, Lu Y, et al. Crosstalk between RNA m(6)A Modification and Non-coding RNA Contributes to Cancer Growth and Progression. Mol Ther Nucleic Acids 2020; Epub ahead of print. [Crossref] [PubMed]
- Li H, Su Q, Li B, et al. High expression of WTAP leads to poor prognosis of gastric cancer by influencing tumour-associated T lymphocyte infiltration. J Cell Mol Med 2020;24:4452-65. [Crossref] [PubMed]
- Zhang B, Wu Q, Li B, et al. m(6)A regulator-mediated methylation modification patterns and tumor microenvironment infiltration characterization in gastric cancer. Mol Cancer 2020;19:53. [Crossref] [PubMed]
- Han D, Liu J, Chen C, et al. Anti-tumour immunity controlled through mRNA m(6)A methylation and YTHDF1 in dendritic cells. Nature 2019;566:270-4. [Crossref] [PubMed]
- Thorsson V, Gibbs DL, Brown SD, et al. The Immune Landscape of Cancer. Immunity 2018;48:812-830.e14. [Crossref] [PubMed]
- Lagergren J, Smyth E, Cunningham D, et al. Oesophageal cancer. Lancet 2017;390:2383-96. [Crossref] [PubMed]
- Tauriello DVF, Sancho E, Batlle E. Overcoming TGFβ-mediated immune evasion in cancer. Nat Rev Cancer 2022;22:25-44. [Crossref] [PubMed]
- Cui X, Wang Z, Li J, et al. Cross talk between RNA N6-methyladenosine methyltransferase-like 3 and miR-186 regulates hepatoblastoma progression through Wnt/β-catenin signalling pathway. Cell Prolif 2020;53:e12768. [Crossref] [PubMed]
- Chen J, Du B. Novel positioning from obesity to cancer: FTO, an m(6)A RNA demethylase, regulates tumour progression. J Cancer Res Clin Oncol 2019;145:19-29. [Crossref] [PubMed]
- Shulman Z, Stern-Ginossar N. The RNA modification N(6)-methyladenosine as a novel regulator of the immune system. Nat Immunol 2020;21:501-12. [Crossref] [PubMed]
- Schreiber RD, Old LJ, Smyth MJ. Cancer immunoediting: integrating immunity's roles in cancer suppression and promotion. Science 2011;331:1565-70. [Crossref] [PubMed]
- Nakamura K, Smyth MJ, Martinet L. Cancer immunoediting and immune dysregulation in multiple myeloma. Blood 2020;136:2731-40. [Crossref] [PubMed]
- Galon J, Bruni D. Approaches to treat immune hot, altered and cold tumours with combination immunotherapies. Nat Rev Drug Discov 2019;18:197-218. [Crossref] [PubMed]
- Saadeldin MK, Abdel-Aziz AK, Abdellatif A. Dendritic cell vaccine immunotherapy; the beginning of the end of cancer and COVID-19. A hypothesis. Med Hypotheses 2021;146:110365. [Crossref] [PubMed]
- Zheng Y, Chen Z, Han Y, et al. Immune suppressive landscape in the human esophageal squamous cell carcinoma microenvironment. Nat Commun 2020;11:6268. [Crossref] [PubMed]
- Zhang X, He T, Li Y, et al. Dendritic Cell Vaccines in Ovarian Cancer. Front Immunol 2021;11:613773. [Crossref] [PubMed]
- Pu J, Wang J, Qin Z, et al. IGF2BP2 Promotes Liver Cancer Growth Through an m6A-FEN1-Dependent Mechanism. Front Oncol 2020;10:578816. [Crossref] [PubMed]
- Ma YS, Shi BW, Guo JH, et al. microRNA-320b suppresses HNF4G and IGF2BP2 expression to inhibit angiogenesis and tumor growth of lung cancer. Carcinogenesis 2021;42:762-71. [Crossref] [PubMed]
- Zou J, Zhong X, Zhou X, et al. The M6A methyltransferase METTL3 regulates proliferation in esophageal squamous cell carcinoma. Biochem Biophys Res Commun 2021;580:48-55. [Crossref] [PubMed]
- Nagaki Y, Motoyama S, Yamaguchi T, et al. m(6) A demethylase ALKBH5 promotes proliferation of esophageal squamous cell carcinoma associated with poor prognosis. Genes Cells 2020;25:547-61. [Crossref] [PubMed]
- Xiao D, Fang TX, Lei Y, et al. m(6)A demethylase ALKBH5 suppression contributes to esophageal squamous cell carcinoma progression. Aging (Albany NY) 2021;13:21497-512. [Crossref] [PubMed]
- Chen P, Li S, Zhang K, et al. N(6)-methyladenosine demethylase ALKBH5 suppresses malignancy of esophageal cancer by regulating microRNA biogenesis and RAI1 expression. Oncogene 2021;40:5600-12. [Crossref] [PubMed]
- Xue J, Xiao P, Yu X, et al. A positive feedback loop between AlkB homolog 5 and miR-193a-3p promotes growth and metastasis in esophageal squamous cell carcinoma. Hum Cell 2021;34:502-14. [Crossref] [PubMed]