Multiple databases analyzed the prognosis prediction of renin secretion pathway-related genes in renal clear cell carcinoma and immunotherapy
Highlight box
Key findings
• A prognostic model based on renin secretion pathway-related genes was established, which can stably predict the prognosis of clear cell renal cell carcinoma (ccRCC) patients.
What is known and what is new?
• The renin secretion pathway affects the various processes of tumor progression, but few studies have explored the effect of renin secretion pathway-related genes on prognosis in patients with ccRCC.
• Based on renin secretion pathway-related genes, a model for predicting the prognosis of ccRCC patients was developed and validated.
What is the implication, and what should change now?
• The model can predict the prognosis of patients with ccRCC. In addition, immuno-related analysis, drug susceptibility analysis, and subtype identification can help personalize the treatment of ccRCC patients.
Introduction
Renal cell carcinoma (RCC) accounts for about 2% to 3% of all adult malignancies (1). The most common histological RCC subtype is clear cell renal cell carcinoma (ccRCC), accounting for ~80% of all RCC cases (2). Diagnostic methods include computed tomography (CT) and magnetic resonance imaging (MRI) imaging but ccRCC is highly aggressive and has a poor prognosis if left untreated. ccRCC management typically involves surgical tumour removal which is most effective when the disease is diagnosed early (3). However, in cases where the disease has spread beyond the kidneys, systemic therapy including targeted agents and immunotherapy may be required. With the development of bioinformatics technology, increasingly more genes are being used as biomarkers for the prognosis prediction of ccRCC (4,5).
The renin-angiotensin-aldosterone system (RAAS) is a crucial physiological process in extracellular volume homeostasis control and kidney disease pathogenesis (6). Renin is released by the glomerular stromal cells and cleaves angiotensinogen to produce angiotensin to regulate blood pressure (7,8). Also, hypertension is an established risk factor for ccRCC development (9) with renin closely associated with the occurrence and progression of malignant tumours (10-12).
This study established a prognostic model containing genes associated with the renin secretion pathway to determine the prognostic significance and mechanisms of renin secretion-related genes in ccRCC. We present this article in accordance with the TRIPOD reporting checklist (available at https://tcr.amegroups.com/article/view/10.21037/tcr-23-1254/rc).
Methods
Data acquisition
ccRCC raw information was downloaded from The Cancer Genome Atlas (TCGA) and International Cancer Genome Consortium (ICGC) databases. TCGA data included 542 kidney cancer samples and 72 normal samples, and the external validation dataset was from the ICGC database. The data were organised through R (4.2.1) and Perl (Strawberry Edition). The GeneCards website was used to identify genes associated with the renin secretion pathway (https://www.genecards.org/). The results of the single-cell analysis of model-related genes are available through our website (http://tisch.comp-genomics.org/). The study was conducted in accordance with the Declaration of Helsinki (as revised in 2013).
Selection of differentially expressed genes (DEGs)
In total, 2,920 renin secretion-related genes were retrieved from the GeneCards website. The ‘limma’ R package identified 453 DEGs including 201 downregulated genes and 252 up-regulated genes [|log fold change (FC)| >1.5, false discovery rate (FDR) <0.05]. DEG expression was then extracted from the TCGA and ICGC cohorts for subsequent analysis.
Model validation
Univariate Cox analysis of the TCGA cohort data identified 188 prognosis-related genes (P<0.05) which were subjected to least absolute shrinkage and selection operator (LASSO) regression and penalty maximum likelihood estimation was performed with 10,000 bootstrap replicates. The optimal weighting coefficient for the gene was determined using the regularisation coefficient of the 1-standard error (SE) standard. Six DEGs (IGFBP3, PLAUR, CHKB-CPT1B, HOXA13, CDH13, and CDC20) were then used to build a model to estimate prognosis. R packages “survival” and “timeROC” were utilised for survival analysis and receiver operating characteristic (ROC) curves were drawn to verify the effectiveness and accuracy of the prognostic model. The ccRCC patients were then classified as low or high risk based on the prognostic model.
Single-cell data analysis of model-associated genes
Single-cell data analysis was applied to verify the model-related gene expression profile in tissues and organs to determine differences between gene expression in different cell types and their role in development, disease and treatment (http://tisch.comp-genomics.org/).
Validation of prognostic features
To assess whether the risk score can be used as an independent predictor of prognosis, Cox analysis was implemented by combining clinical variables. For the purpose of a more accurate forecast of the survival rate of ccRCC patients, while making the model more readable, a nomogram was constructed based on clinical indicators and risk scores. A consistency indicatrix and calibration curve were constructed to validate the prediction accuracy of the nomograms.
Functional enrichment analysis
The DEG data were analysed by Gene Ontology (GO) and Kyoto Encyclopedia of Genes and Genomes (KEGG) (P<0.05) using the “clusterProfiler” package.
Immune cell infiltration analysis
Immune cell infiltration was compared by diverse analytical platforms. Immune-related functions, immune checkpoints between risk categories, and the tumour microenvironment (TME) scores were investigated to score immune status in ccRCC patients.
Drug susceptibility analysis
The correlation of different drug susceptibility analysis results in cell line progenitors was used to assess the response of ccRCC patients to treatment through the pRRophetic software package.
Subtype analysis
The R package “ConsensusClusterPlus” was used for consistency cluster analysis, to define molecular subsets by six renin secretion pathway-related genes, and compare the relationship between renin secretion pathway-related gene molecular subtypes and overall survival (OS). The characteristics of TME, immune checkpoint differences and drug susceptibility analysis were compared between subtypes to provide some basis for immunotherapy.
Statistical analysis
The statistical analysis was performed using R software (version 4.1.2). The chi-square test was used to analyse differences in patients, with Kaplan-Meier analysis and log-rank analysis used to assess patients’ OS and progression-free survival (PFS). The Wilcoxon test was used to check the deviation between the components. A P value <0.05 was considered statistically significant.
Results
Selection and identification of DEG
The study flow diagram is presented in Figure 1. Gene expression in tumour and normal tissues were compared to identify 453 DEGs (Figure 2). Univariate Cox analysis (P<0.05) was performed to obtain 188 DEGs associated with ccRCC.
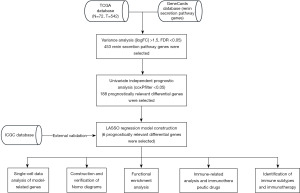
Construction and validation of the prognostic model
LASSO regression analysis using the “glmnet” R package yielded six (IGFBP3, PLAUR, CHKB-CPT1B, HOXA13, CDH13, and CDC20) key renin secretion pathway-related genes (Figure 3A). The risk score was calculated as follows: (IGFBP3*−0.0062) + (PLAUR*0.1201) + (CHKB-CPT1B*6.0671) + (HOXA13*0.3239) + (CDH13*−0.1375) + (CDC20*0.2060) (risk factor results retain 4 decimal places). ccRCC patients were then scored using this formula and grouped using the med-value patient risk score of the TCGA dataset. The outcomes revealed that the risk score is a speciality factor (Figure 3B).
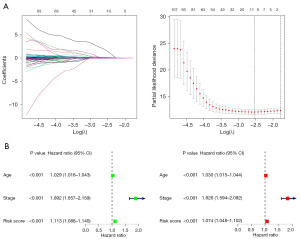
The model accuracy was evaluated using survival and ROC curves. The survival analysis of the TCGA dataset indicated a difference in survival between the low- and high-risk groups (P<0.001), with a ROC area >0.65 (Figure 4A). The model accuracy was then confirmed using the ICGC dataset for external testing, revealing that there was a significant difference in the survival rate between the two groups (P<0.001). The 1-, 3- and 5-year area under the curve (AUC) values were 0.739, 0.734 and 0.678 respectively (Figure 4B). A PFS analysis model was then constructed showing that PFS was different between risk groups (Figure 4C). Among the multivariate ROC curves, the risk score had the highest fatidic potency (Figure 4C). Analysis of all cohorts showed an increase in deaths as risk scores increased, indicating that patients with higher risk scores had a worse prognosis.
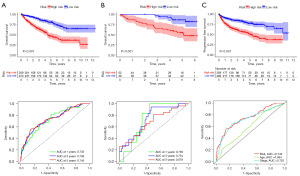
Single-cell data analysis of model-associated genes
The differences between model genes (IGFBP3, PLAUR, CDH13, CDC20, CHKB-CPT1B, and HOXA13) in different cell types and their role in development, disease and treatment were investigated using the http://tisch.comp-genomics.org/ website. There was insufficient information regarding CHKB-CPT1B and HOXA13, so IGFBP3, PLAUR, CDH13 and CDC20 were analysed to provide important help for clinical application (Figure 5).
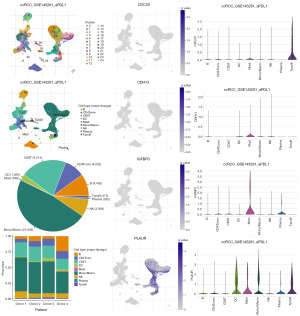
Nomogram construction and verification
A nomogram was constructed to improve the accuracy and readability and evaluated using calibration curves (Figure 6A,6B). Cox analysis showed that the predictive power of the nomogram was not affected by other factors (Figure 6C,6D).
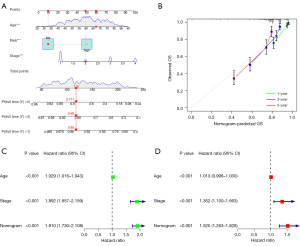
Functional enrichment analysis
Gene Set Variation Analysis (GSVA) was conducted to study the differences in the biological functions of the DEGs, showing that the phosphokinase metabolic pathway, neurotrophic factor signalling pathway and TGFβ signalling pathways were primarily enriched in the low-risk groups, whereas the P53 signalling pathway, myocardial contraction pathway and other signalling pathways were mainly enriched in the high-risk group (Figure 7A). The DEGs were then analysed using the “limma” package, GO and KEGG (Figure 7B,7C).
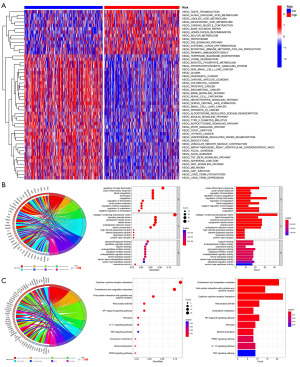
Analysis of immune cell infiltration and drug susceptibility
The correlation of immune-related functions was assessed showing that the immune pathway activity in the high-risk group was much higher than that of the type II interferon (IFN) response pathway (Figure 8A). In combination with immune checkpoint analysis, most immune checkpoints were activated in the high-risk group (Figure 8B), suggesting that we can block the suppression of immune cells by tumour cells by customising individualised immunosuppression. Comparison of the immune function between groups with the ESTIMATE package revealed a higher ImmuneScore and ESTIMATEScore in the high-risk group (Figure 8C). In summary, immunotherapy is more effective for high-risk ccRCC. Drug susceptibility analysis results showed that patients with higher risk scores were more sensitive to common chemotherapy drugs (vinorelbine, sorafenib, pyrimethamine, and salubrinal) (Figure 8D), therefore allowing more precise treatment of ccRCC patients with different risk stratifications.
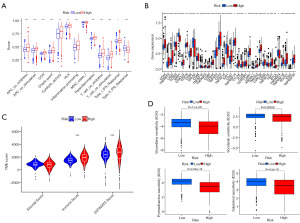
Determination of the immune subtype
Consistent clustering analysis was performed using the six renin secretion pathway-related genes closely related to OS. Principal component analysis (PCA) divided C1 and C2 into two different regions, with T-distributed stochastic neighbor embedding (t-SNE) showing tumour heterogeneity in ccRCC. The Sankey plot showed that C1 is mostly pooled in the low-risk group and C2 has more overlapping areas in the high-risk group (Figure 9).
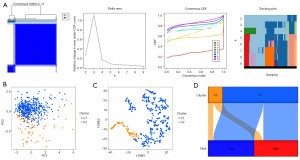
Immune subtype and immunotherapy
All immune checkpoints are upregulated at C1 (Figure 10A). The drug sensitivity analysis revealed that the half maximal inhibitory concentration (IC50) of C2 was lower than that of C1, indicating that immunotherapy is more effective in C2 (Figure 10B).
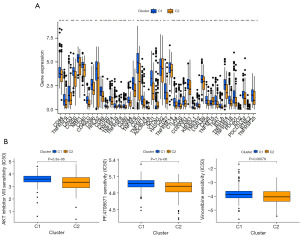
Discussion
ccRCC progression is associated with the renin secretion pathway, so bioinformatics analyses were performed to evaluate the potential of renin secretion pathway-related genes as ccRCC prognostic biomarkers. Six renin secretion genes were identified (IGFBP3, PLAUR, CHKB-CPT1B, HOXA13, CDH13, and CDC20) and used to construct the prognostic model.
The IGF1 signalling pathway participates in cell proliferation and differentiation and is closely associated with tumour progression (13). IGFBP3 regulates IGF1 activity by binding to IGF1 and IGFBP3 leads to the recurrence and metastasis of lung cancer (14). High IGFBP3 expression has been observed in endometrial cancer, stomach cancer, and advanced prostate cancer (15-17) and increases the risk of premenopausal breast and prostate cancers (17,18). Transcription factor 7 like 2 (TCF7L2) is a key regulator of cell development and growth (19,20) and is associated with malignant tumours such as colon cancer and prostate cancer (21,22). As a transcription factor for PLAUR, TCF7L2 is associated with the poor prognosis of gastric cancer (23). Wang et al. reported that high PLAUR expression decreased the prognostic level of ccRCC and PLAUR could be key to the treatment of ccRCC (24). HOXA13 is a member of the homeobox (HOX) family (25,26) and is abnormally expressed in a variety of tumours (26-29); the higher the HOXA13 expression, the worse the prognosis in line with our research. Lin et al. found that CDH13 was positively correlated with the metastasis and progression of bladder cancer (30). Wang et al. and Zheng et al. also reported that CDH13 can enhance tumour invasion ability and regulate tumour progression (31,32). This study confirms the conclusions of Kidokoro et al. and Wang et al. that CDC20 is a carcinogen that promotes tumour progression (33,34). Consistent with our study, increased CDC20 expression in ccRCC patients is associated with a poor prognosis (35). Carnitine palmitoyl transferase 1B (CPT1B) is closely related to choline kinase β (CHKB). The CHKB gene is transcribed from the same DNA strand and terminates upstream of CPT1B (36). The tight combination of CHKB-CPT1B may overlap the cis-action regulatory domains and realise a common regulatory mechanism (37). Mierxiati Abudurexiti’s study has confirmed that CTP1B expression is upregulated in prostate cancer (38). Our study also confirms the upregulation mechanism of CHKB-CPT1B in tumours, so the gene could be a potential biomarker for bladder cancer.
GO enrichment analysis demonstrated metabolic pathways involving genes associated with the renin secretion pathway. KEGG’s main enriched pathway is involved in promoting metabolism, cell proliferation, differentiation and death. Genes related to the renin secretion pathway are irreplaceable in the metabolism of cancer cells and the formation of the TME.
Those ccRCC patients who cannot tolerate surgery may be suitable for immunotherapy. We identified two tumour subtypes, showing that C1 mostly overlapped in the low-risk group and C2 mostly pooled in the high-risk group. The IC50 value measures the drug’s ability to induce apoptosis, thus lower IC50 values increase inductive power and reflect the cell’s tolerance to the drug. The IC50 of C2 was significantly lower than that of C1 indicating that drug subtype C2 was less tolerant to immunotherapy, suggesting that the C2 group is more likely to benefit from immunotherapy.
This study has some limitations. Although we screened and identified six genes associated with ccRCC prognosis, the accuracy of the modelling was determined by an external validation set. However, we could only answer predictions about ccRCC prognosis with limited data but this preliminary research provides a solid basis for further research.
Conclusions
This study provides a crucial foundation for ccRCC therapy and can help to individualize the treatment of ccRCC in the early stage.
Acknowledgments
We sincerely thank all members for their contributions to this study. We would like to express our gratitude to the TCGA database for providing the platform and meaningful data.
Funding: None.
Footnote
Reporting Checklist: The authors have completed the TRIPOD reporting checklist. Available at https://tcr.amegroups.com/article/view/10.21037/tcr-23-1254/rc
Peer Review File: Available at https://tcr.amegroups.com/article/view/10.21037/tcr-23-1254/prf
Conflicts of Interest: All authors have completed the ICMJE uniform disclosure form (available at https://tcr.amegroups.com/article/view/10.21037/tcr-23-1254/coif). The authors have no conflicts of interest to declare.
Ethical Statement: The authors are accountable for all aspects of the work in ensuring that questions related to the accuracy or integrity of any part of the work are appropriately investigated and resolved. The study was conducted in accordance with the Declaration of Helsinki (as revised in 2013).
Open Access Statement: This is an Open Access article distributed in accordance with the Creative Commons Attribution-NonCommercial-NoDerivs 4.0 International License (CC BY-NC-ND 4.0), which permits the non-commercial replication and distribution of the article with the strict proviso that no changes or edits are made and the original work is properly cited (including links to both the formal publication through the relevant DOI and the license). See: https://creativecommons.org/licenses/by-nc-nd/4.0/.
References
- Znaor A, Lortet-Tieulent J, Laversanne M, et al. International variations and trends in renal cell carcinoma incidence and mortality. Eur Urol 2015;67:519-30. [Crossref] [PubMed]
- Wei JH, Feng ZH, Cao Y, et al. Predictive value of single-nucleotide polymorphism signature for recurrence in localised renal cell carcinoma: a retrospective analysis and multicentre validation study. Lancet Oncol 2019;20:591-600. [Crossref] [PubMed]
- Ljungberg B, Bensalah K, Canfield S, et al. EAU guidelines on renal cell carcinoma: 2014 update. Eur Urol 2015;67:913-24. [Crossref] [PubMed]
- Sun Z, Tao W, Guo X, et al. Construction of a Lactate-Related Prognostic Signature for Predicting Prognosis, Tumor Microenvironment, and Immune Response in Kidney Renal Clear Cell Carcinoma. Front Immunol 2022;13:818984. [Crossref] [PubMed]
- Huili Y, Nie S, Zhang L, et al. Cuproptosis-related lncRNA: Prediction of prognosis and subtype determination in clear cell renal cell carcinoma. Front Genet 2022;13:958547. [Crossref] [PubMed]
- Atlas SA. The renin-angiotensin aldosterone system: pathophysiological role and pharmacologic inhibition. J Manag Care Pharm 2007;13:9-20. [Crossref] [PubMed]
- Gonzalez AA, Prieto MC. Renin and the (pro)renin receptor in the renal collecting duct: Role in the pathogenesis of hypertension. Clin Exp Pharmacol Physiol 2015;42:14-21. [Crossref] [PubMed]
- Gonzalez AA, Lara LS, Prieto MC. Role of Collecting Duct Renin in the Pathogenesis of Hypertension. Curr Hypertens Rep 2017;19:62. [Crossref] [PubMed]
- Zheng Q, Wang Y, Zhao R, et al. Inactivation of epithelial sodium ion channel molecules serves as effective diagnostic biomarkers in clear cell renal cell carcinoma. Genes Genomics 2023;45:855-66. [Crossref] [PubMed]
- Citron M, Decker R, Chen S, et al. O6-methylguanine-DNA methyltransferase in human normal and tumor tissue from brain, lung, and ovary. Cancer Res 1991;51:4131-4. [PubMed]
- Deckers IA, van den Brandt PA, van Engeland M, et al. Polymorphisms in genes of the renin-angiotensin-aldosterone system and renal cell cancer risk: interplay with hypertension and intakes of sodium, potassium and fluid. Int J Cancer 2015;136:1104-16. [Crossref] [PubMed]
- Wu B, Dou G, Zhang Y, et al. Identification of key pathways and genes in vestibular schwannoma using bioinformatics analysis. Exp Ther Med 2022;23:217. [Crossref] [PubMed]
- Pollak M. Insulin and insulin-like growth factor signalling in neoplasia. Nat Rev Cancer 2008;8:915-28. [Crossref] [PubMed]
- Chen B, Liu S, Xu W, et al. IGF-I and IGFBP-3 and the risk of lung cancer: a meta-analysis based on nested case-control studies. J Exp Clin Cancer Res 2009;28:89. [Crossref] [PubMed]
- Lacey JV Jr, Potischman N, Madigan MP, et al. Insulin-like growth factors, insulin-like growth factor-binding proteins, and endometrial cancer in postmenopausal women: results from a U.S. case-control study. Cancer Epidemiol Biomarkers Prev 2004;13:607-12. [Crossref] [PubMed]
- Xue M, Fang Y, Sun G, et al. IGFBP3, a transcriptional target of homeobox D10, is correlated with the prognosis of gastric cancer. PLoS One 2013;8:e81423. [Crossref] [PubMed]
- Chen W, Wang S, Tian T, et al. Phenotypes and genotypes of insulin-like growth factor 1, IGF-binding protein-3 and cancer risk: evidence from 96 studies. Eur J Hum Genet 2009;17:1668-75. [Crossref] [PubMed]
- Renehan AG, Zwahlen M, Minder C, et al. Insulin-like growth factor (IGF)-I, IGF binding protein-3, and cancer risk: systematic review and meta-regression analysis. Lancet 2004;363:1346-53. [Crossref] [PubMed]
- Dias C, Pfundt R, Kleefstra T, et al. De novo variants in TCF7L2 are associated with a syndromic neurodevelopmental disorder. Am J Med Genet A 2021;185:2384-90. [Crossref] [PubMed]
- Savic D, Ye H, Aneas I, et al. Alterations in TCF7L2 expression define its role as a key regulator of glucose metabolism. Genome Res 2011;21:1417-25. [Crossref] [PubMed]
- Chen J, Yuan T, Liu M, et al. Association between TCF7L2 gene polymorphism and cancer risk: a meta-analysis. PLoS One 2013;8:e71730. [Crossref] [PubMed]
- Drake I, Wallström P, Hindy G, et al. TCF7L2 type 2 diabetes risk variant, lifestyle factors, and incidence of prostate cancer. Prostate 2014;74:1161-70. [Crossref] [PubMed]
- Zhang T, Wang B, Su F, et al. TCF7L2 promotes anoikis resistance and metastasis of gastric cancer by transcriptionally activating PLAUR. Int J Biol Sci 2022;18:4560-77. [Crossref] [PubMed]
- Wang Y, Sun Z, Lu S, et al. Identification of PLAUR-related ceRNA and immune prognostic signature for kidney renal clear cell carcinoma. Front Oncol 2022;12:834524. [Crossref] [PubMed]
- Quagliata L, Matter MS, Piscuoglio S, et al. Long noncoding RNA HOTTIP/HOXA13 expression is associated with disease progression and predicts outcome in hepatocellular carcinoma patients. Hepatology 2014;59:911-23. [Crossref] [PubMed]
- Qiao C, Huang W, Chen J, et al. IGF1-mediated HOXA13 overexpression promotes colorectal cancer metastasis through upregulating ACLY and IGF1R. Cell Death Dis 2021;12:564. [Crossref] [PubMed]
- Qin Z, Chen Z, Weng J, et al. Elevated HOXA13 expression promotes the proliferation and metastasis of gastric cancer partly via activating Erk1/2. Onco Targets Ther 2019;12:1803-13. [Crossref] [PubMed]
- Gu ZD, Shen LY, Wang H, et al. HOXA13 promotes cancer cell growth and predicts poor survival of patients with esophageal squamous cell carcinoma. Cancer Res 2009;69:4969-73. [Crossref] [PubMed]
- Liu C, Tian X, Zhang J, et al. Long Non-coding RNA DLEU1 Promotes Proliferation and Invasion by Interacting With miR-381 and Enhancing HOXA13 Expression in Cervical Cancer. Front Genet 2018;9:629. [Crossref] [PubMed]
- Lin YL, Xie PG, Ma JG. Aberrant methylation of CDH13 is a potential biomarker for predicting the recurrence and progression of non muscle invasive bladder cancer. Med Sci Monit 2014;20:1572-7. [Crossref] [PubMed]
- Wang M, Long K, Li E, et al. DNA polymerase beta modulates cancer progression via enhancing CDH13 expression by promoter demethylation. Oncogene 2020;39:5507-19. [Crossref] [PubMed]
- Zheng W, Zhang S, Guo H, et al. Multi-omics analysis of tumor angiogenesis characteristics and potential epigenetic regulation mechanisms in renal clear cell carcinoma. Cell Commun Signal 2021;19:39. [Crossref] [PubMed]
- Kidokoro T, Tanikawa C, Furukawa Y, et al. CDC20, a potential cancer therapeutic target, is negatively regulated by p53. Oncogene 2008;27:1562-71. [Crossref] [PubMed]
- Wang Z, Wan L, Zhong J, et al. Cdc20: a potential novel therapeutic target for cancer treatment. Curr Pharm Des 2013;19:3210-4. [Crossref] [PubMed]
- Shi J, Chen Y, Gu X, et al. The Prognostic Assessment of CDC20 in Patients with Renal Clear Cell Carcinoma and Its Relationship with Body Immunity. Contrast Media Mol Imaging 2022;2022:7727539. [Crossref] [PubMed]
- Patel BV, Yao F, Howenstine A, et al. Emergent Coordination of the CHKB and CPT1B Genes in Eutherian Mammals: Implications for the Origin of Brown Adipose Tissue. J Mol Biol 2020;432:6127-45. [Crossref] [PubMed]
- Cingoz S, Agilkaya S, Oztura I, et al. Identification of the variations in the CPT1B and CHKB genes along with the HLA-DQB1*06:02 allele in Turkish narcolepsy patients and healthy persons. Genet Test Mol Biomarkers 2014;18:261-8. [Crossref] [PubMed]
- Abudurexiti M, Zhu W, Wang Y, et al. Targeting CPT1B as a potential therapeutic strategy in castration-resistant and enzalutamide-resistant prostate cancer. Prostate 2020;80:950-61. [Crossref] [PubMed]