Preparation of hepatocellular carcinoma mRNA vaccines based on potential tumor targets and immunophenotypes
Highlight box
Key findings
• Six genes (AURKA, CDC25C, KPNA2, MCM3, NEK2 and TUBG1) with high mutation and antigen-presenting cell infiltration can be utilized to develop messenger RNA (mRNA) vaccine for hepatocellular carcinoma (HCC).
• Immune-hot patients may exhibit characteristics of immune exhaustion, while immune-cold patients are potential beneficiaries of mRNA vaccines. However, high expression of blue and black immune gene modules is detrimental to the effectiveness of mRNA vaccines.
What is known and what is new?
• In the immune-hot phenotype, TP53 mutations are more prevalent, while in the immune-cold phenotype, CTNNB1 mutations are more predominant.
What is the implication, and what should change now?
• This research workflow provides insights into the preparation and application of mRNA vaccines for HCC.
• The differences in core mutation genes among different immunophenotypes suggest that exploratory studies related to them, such as TP53 and CTNNB1, may potentially enhance patients’ responsiveness to mRNA vaccines.
Introduction
Hepatocellular carcinoma (HCC) is the fourth most frequent cause of cancer-related death among all tumor-related deaths in China, accounting for 75–85% of primary liver cancer (1-3). It is widely known that hepatitis B virus-related and alcohol-related cirrhosis are the two major high-risk factors for HCC, and most cases of HCC have an insidious onset and progress rapidly (4,5). More than one-thirds of patients are in the middle and late stages at diagnosis, thus forfeiting the chance for immediate radical surgery (1,6). Comprehensive treatment for HCC has shown encouraging efficacy in early-stage liver cancer, but the efficacy for advanced HCC is still insignificant (7,8).
Immunotherapy for HCC has made great progress in recent years, bringing hope to many patients who cannot immediately undergo radical surgery, especially the immune checkpoint inhibitors (ICIs) therapy. It is often combined with other treatment modalities, such as targeted therapy and topical therapy, but its serious side effects limit the degree of benefit. With the continuous emergence of new therapeutic targets, tumor vaccines may be one of the development directions of HCC immunotherapy.
Tumor vaccine therapy often targets one or more tumor-associated antigens. It treats cancer by reprogramming the immune system to activate or enhance the body’s ability to attack cancer cells. Due to the advantages of relatively non-toxicity, high specificity, and the ability to stimulate lasting immune memory, tumor vaccines have the potential to overcome drug resistance and reduce adverse reactions, and ultimately achieve more ideal therapeutic effects (9). Peptides, cells, DNA, and RNA can be carrier of vaccines. Requiring customization based on individual patients and genetic analysis of the tumor, peptide vaccine is time-consuming and may postpone the patient’s treatment, thus they may not be suitable for treating HCC. Dendritic cells (DC), the most significant antigen-presenting cells (APCs), have shown satisfactory responses to stimulate T cell effect in pre-clinical trials as vaccines, but the outcomes in clinical trials have been disappointing. The limited immunogenicity and the risk of insertional mutations also limit the development of DNA vaccines (9-11).
In contrast, messenger RNA (mRNA)-based vaccines are tolerable and do not integrate into the host genome. Non-infectious mRNA molecules can induce both humoral and cellular immunity in mRNA vaccines without needing pathogenic viral preparations (11,12). Since tumor antigens are expressed at high levels in malignant tumor cells, mRNA vaccines can reach tumor cells through antigen presenting cells, triggering an immune waterfall response, and activated effector immune cells are like airborne troops targeting tumor cells, selectively killing them, but can reduce the killing of normal cells as much as possible. In addition, activated memory immune cells can become immune reserves, achieving long-lasting tumor killing (13). Combination therapy with mRNA vaccines and other immunotherapies are already used in lung cancer, pancreatic cancer and advanced melanoma, which promise even more encouraging therapeutic effects (14-16). The phase Ib clinical trial of Awad et al. showed that the personalized neoantigen vaccine NEO-PV-01 in combination with pemetrexed, carboplatin, and pembrolizumab has good safety and immunogenicity as a first-line treatment for advanced non-squamous non-small cell lung cancer (NSCLC) (16). In February 2023, the Food and Drug Administration (FDA) announced that the mRNA-4157 vaccine combined with pembrolizumab for treating high-risk melanoma has become a breakthrough treatment option, making it the first mRNA cancer vaccine to receive this designation worldwide.
Our research focuses on the selection and preliminary validation of tumor antigens for the preparation of mRNA vaccines for HCC, constructing the immune landscape of HCC to select potentially beneficial patients, and exploring genes that potentially suppress tumor antigen immunity. We selected 6 antigen targets associated with poor prognosis and APC enrichment scores among genes with overexpression and high mutation frequency in HCC. Based on the clustering analysis and immune landscape plotting with immune gene collections, the patients in The Cancer Genome Atlas - Liver Hepatocellular Carcinoma (TCGA-LIHC), International Cancer Genome Consortium - Liver Cancer (ICGC-LIRI) databases were divided into two phenotypes, immunocold and immunohot, and the two subtypes showed different clinical, molecular and cellular characteristics, patients with immunocold phenotypes were more likely to become beneficiaries of mRNA vaccines, while patients with immunothermal phenotypes showing autoimmune antagonism had inefficient response to mRNA vaccines, and the results of the two databases had a considerable degree of consistency. In conclusion, this study provides a detailed theoretical analysis for the development of mRNA vaccine for HCC, in anticipation of bringing more treatments to patients with intermediate and advanced stages and improving their survival time and quality of life.
Methods
Data collection and preprocessing
Clinical information and RNA sequencing (RNASeq) data for patients and control cases were extracted from the TCGA-LIHC cohort (TCGA, https://www.cancer.gov/tcga) and IGCG-LIRI cohort (ICGC, https://www.icgc-argo.org), including 421 LIHCs samples and 260 LIRIs samples. Standard sample data and tumor sample data lacking clinical information were first eliminated. Samples with 0 transcripts per million (TPM) of more than 50% of genes were also eliminated. Ultimately, 330 LIHCs samples and 231 LIRIs samples were selected. Chip sequencing data for 14 pairs of tumor and normal tissues were obtained from GSE84402 (17). Chip sequencing data of 24 tumor tissues and 8 normal tissues were obtained from GSE101685. A total of 1,793 immune genes were obtained from the Immport database (https://www.immport.org/home) (18,19). R packages “DESeq2” was used to extract upregulated immune genes common to TCGA and ICGC cohorts (parameter: |log2FC| >1 and q-value <0.01) (20), resulting in a collection of immune genes including 129 genes for subsequent analysis (available online https://cdn.amegroups.cn/static/public/tcr-23-1237-1.xlsx). Surgical specimens and clinical data of 52 patients were recruited from Sun Yat-sen Memorial Hospital. The studies involving human participants were reviewed and approved by the Ethics Committee of Sun Yat-sen Memorial Hospital (ID: SYSKY-2023-1003-01) and were conducted in accordance with the Declaration of Helsinki (as revised in 2013). The patients/participants provided their written informed consent to participate in study.
Antigen targets exploration
GEPIA2.0 (http://gepia2.cancer-pku.cn) was employed to perform differential analysis (parameter: |log2FC| >1 and q-value <0.01) and survival analysis on LIHC samples. The Kaplan-Meier curve analysis explored overall survival (OS) and recurrence-free survival (RFS) with a log-rank test providing a P value (21). The cBioPortal (http://www.cbioportal.org) was employed to compare genetic mutation information from LIHC samples (22). The TIMER2.0 (https://cistrome.shinyapps.io/timer) was employed to visualize relationship of gene expression and immune cells in LIHC samples (23).
Immunohistochemistry (IHC)
Tissue microarrays (TMAs) were made using pathological specimens collected from 52 patients with HCC collected from Sun Yat-sen Memorial Hospital, including tumor tissue and adjacent tissues. AURKA, CDC25C, KPNA2, MCM3 and TUBG1 antibodies were purchased from Abcam (ab52973, ab32444, ab289858, ab128923, ab179503, CN, Shanghai) and diluted into 1:100, 1:1,250, 1:2,500, 1:100, 1:100, and NEK2 antibodies were purchased from Pygcn (NEK2 Polyclonal antibody, CN, Wuhan), and diluted into 1:400. The average optical density value calculated by IamgeJ was used as the observation index of IHC. Three fields of view for each tissue were selected, the average optical density value of its score was calculated, and the average of the three was taken as the result (mean). The top 26 patients with high expression scores were defined as high expression groups (available online https://cdn.amegroups.cn/static/public/tcr-23-1237-2.xlsx; https://cdn.amegroups.cn/static/public/tcr-23-1237-3.xlsx).
Discovery and validation of immunophenotypes
Immunophenotypes were obtained by clustering samples according to the expression matrix of 129 immune genes in LIHC samples and LIRI samples. R package “ConsensusClusterPlus” was responsible for clustering and selecting the optimal classification by cumulative distribution function (CDF) and delta area (DA) (24). The relevant parameters were set to a K value with a range of 2–10, 1,000 resamplings with 80% project resampling (pItem) and 100% gene resampling (pFeature).
Construction of immune landscape
R package Single sample gene set enrichment analysis (ssGSEA) was used to calculate the immune enrichment score of each sample, which could measure the immune infiltration status. R package “estimate” was used to assess each patient’s immune score and stromal score. R package “Monocle2” was used to visualize the distribution of immunophenotypes of LIHC samples (parameter: maximum component =3) (25,26). Finally, the immune landscape dimensionality reduction was visualized as a trajectory map composed of different immunophenotypes color-coded. The gene sets used in the enrichment analysis can be found in available online https://cdn.amegroups.cn/static/public/tcr-23-1237-4.xlsx; https://cdn.amegroups.cn/static/public/tcr-23-1237-5.xlsx.
Weighted immunogenic gene co-expression network of LIHC
R package weighted correlation network analysis (WGCNA) was used to construct a correlation network of 1,339 immune-related genes (soft threshold =5) and obtain different modules in the network. The characteristic genes of each module were calculated, and modules with prognostic values for OS were identified (P<0.05) (27). The correlation between genes within the predictive module and the characteristic genes was calculated, and genes with correlation >0.9 and P value <0.05 were identified in Cox regression analyses to construct a risk model. R package “DAVID” was used for functional annotation of each gene module through the Kyoto Encyclopedia of Genes and Genomes (KEGG) pathway enrichment analysis (28,29). The entire genes contained in each module can be found in available online https://cdn.amegroups.cn/static/public/tcr-23-1237-6.xlsx.
Statistical analysis
R software (version 4.1.0 and 4.2.2) was responsible for all statistical analyses and the Bonferroni corrected P value (P<0.05/n) was used to measure significant differences.
Results
Discovery of antigen targets for HCC
First of all, 1,482 overexpressed genes that may have the potential to be antigen targets were screened in LIHC (Figure 1A, available online https://cdn.amegroups.cn/static/public/tcr-23-1237-7.xlsx). Most patients exhibited a low overlap count in terms of fraction genome alteration and mutation count, which may indicate that HCC itself has low immunogenicity (Figure 1B,1C). Then, by analyzing gene expression of altered genome fragments and mutation counts in samples, 4,452 mutated genes that may express tumor-specific antigens were selected (Figure 1D). Mutation analysis showed that the highest ten in the altered genome fragments group were tumor protein p53 (TP53), titin (TTN), mucin 5B (MUC5B), pecanex 2 (PCNX2), obscurin (OBSCN), dystrobrevin beta (DTNB), solute carrier family 8 member A1 (SLC8A1), anoctamin 1 (ANO1), MYC binding protein 2 (MYCBP2) and serine protease 38 (PRSS38). The highest ten in the mutation counts group were titin (TTN), obscurin (OBSCN), tumor protein p53(TP53), mucin 16 (MUC16), AHNAK nucleoprotein 2 (AHNAK2), ryanodine receptor 2 (RYR2), dynein axonemal heavy chain 2 (DNAH2), piccolo presynaptic cytomatrix protein (PCLO), DExH-box helicase 9 (DHX9) and catenin beta 1 (CTNNB1), of which TP53, TTN, and OBSCN were in the top ten of both groups (Figure 1E,1F). Overall, 223 overexpressed and frequently mutated antigen targets were discovered (Figure 1C).
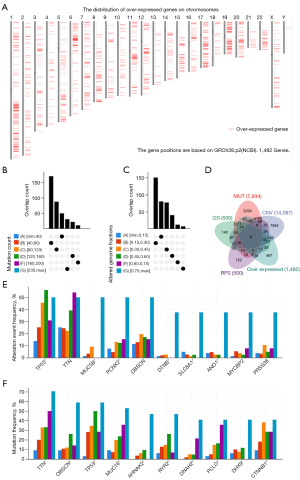
Identification of tumor antigens associated with HCC prognosis and antigen presenting cells
Screening for tumor antigens linked to a poor prognosis, six genes were found to be associated with shorter OS and RFS: AURKA, CDC25C, KPNA2, MCM3, NEK2 and TUBG1 (Figures 1C,2A). In summary, the six candidate genes are critical to the development and progress of LIHC. In addition, elevated expression levels of AURKA, CDC25C, KPNA2, MCM3, NEK2 and TUBG1 were positively associated with the infiltration of macrophages, dendritic cells, and B cells in tumor tissues (Figure 2B). It suggests that these tumor antigens can be delivered to T cells through APCs and trigger an immune response in vivo through mutual recognition with B cells. The elevated expression of the above six candidate genes in tumor tissues was verified in the two Gene Expression Omnibus (GEO) validation cohorts GSE84402 and GSE101685 (Figure S1A,S1B).
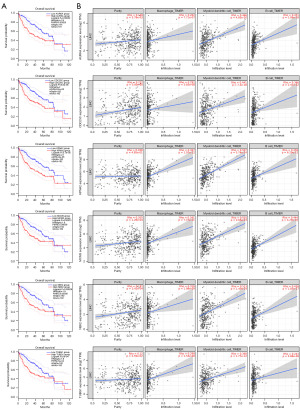
IHC
The feasibility of the six candidate genes were further verified by immunohistochemical analysis. We observed that the IHC scores of three genes were upregulated in tumorous tissues, among which AURKA staining concentrated in the cytoplasm, MCM3 staining concentrated in the nucleus, CDC25C staining concentrated in the cytoplasm and nucleus (Figure 3A,3B). Survival analysis was employed to evaluate the correlation between IHC scores and prognosis. High IHC scores of AURKA (P=0.038) and CDC25C (P=0.03) indicated poor prognosis (Figure 3C,3D).
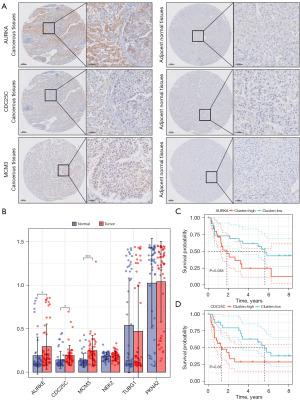
Identification of potential immunophenotypes of HCC
Immunophenotypes reflect the specific immune situation and clinical characteristics of HCC patients, helping to select potential beneficiaries. We analyzed the RNA-Seq data of 129 differential immune genes (Figure 4A) from 330 LIHC samples in LIHC cohort and 231 LIRI samples in ICGC cohort, and five stable immunophenotypes were obtained based on the CDF and DA result (k=5) of the two cohorts (Figure 4B-4H). The prognosis of IS2 and IS5 was better, while the prognosis of IS4 was worse (Figure 4E). Similarly, immunophenotypes were also associated with prognosis in the LIRI cohort (Figure 4I). The distribution relationship between immune subtypes and stage of LIHC and LIRI cohorts also showed certain similarities (Figure S2). In conclusion, immunophenotypes can assess the microenvironment of tumors and evaluate the prognosis of HCC and can be used as a complement to tumor behavior assessment.
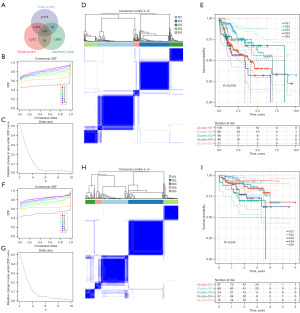
Cellular characteristics of immunophenotypes
The immune status of tumors often affects the effectiveness of mRNA vaccines, so we further used 28 previously reported immune cell genes to assess the immune situation of two cohorts of these subtypes with ssGSEA. Through the heatmap visualization of the ssGSEA score, we found that the distribution of immunity scores for IS1 and IS5 was similar, and IS2 and IS3 were identical, and interestingly this result was consistent across LIHC (Figure 5A) and LIRI (Figure 5B). Then we further compared the immune cell composition of each subtype and found a wide range of significant differences in expression. Therefore, IS1 and IS5, IS2 and IS3 were merged respectively. We discovered that Activated B cells, Activated CD4+ T cells, Activated CD8+ T cells, macrophage, myeloid-derived suppressor cells (MDSCs), and Regulatory T cells in IS1 and IS5 had higher enrichment scores, Type 1 T helper cells, Type 2 T helper cells, and Type 17 T helper cells had lower enrichment scores (Figure 5C,5D). Thus, IS1 and IS5 were immunologically hot accompanied by autoimmunosuppression, while IS2 and IS3 were immunologically cold. These results suggested that immunophenotypes reflect the immune status of HCC and can provide theoretical support for selecting appropriate beneficiaries. The mRNA vaccines can stimulate immune cell responses in immunologically cold IS2 and IS3 tumors for better therapeutic outcomes. In addition, the higher immune score and stromal score of IS1 and IS5 verified the reliability of our immunophenotypes, showing that the IS1 and IS5 subtypes may have a resistance to immunotherapy.
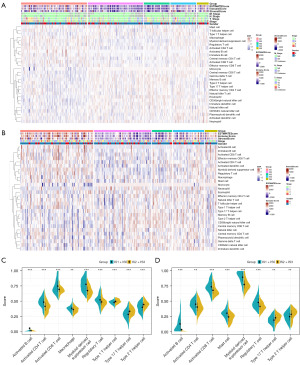
The immune landscape of HCC
We constructed the immune landscape of LIHC using the 129 immune gene sets previously built for immunophenotypes, and each point on the graph represented a patient with a unique immune status (Figure 6A). A significantly positive correlation was found between the horizontal axis (principal component one, PCA1) and ssGSEA scores of immune cell modules, such as MDSC, Activated DCs, Activated CD8+ T cells, Activated CD4+ T cells, Activated B cells, Regulatory T cells, Mast cells, and Macrophages, whereas the vertical axis (PCA2) was significantly negatively associated with them (Figure 6B). Based on the positions of patients, IS1, IS2, and IS3 were split into two subtypes (Figure 6C), and the new immunophenotypes also showed significant prognostic outcomes (Figure 6D). Compared to other sub-clusters, IS2a and IS3a had notably lower scores on activated immune cell modules. Thus, LIHC patients with IS2a and IS3a may be the most likely potential beneficiaries of mRNA vaccines. In summary, immunophenotyping-based immune landscapes can illustrate the infiltration of immune cells in each patient, forecast the prognosis of those patients, and facilitate the individualized selection of mRNA vaccines.
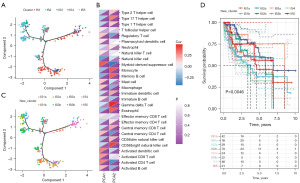
Association between immunophenotypes and immune modulators
Immune checkpoints (ICPs) and immunogenic cell death (ICD) regulators are related to tumor immunogenicity and responsiveness to immunotherapy, and we next analyzed the expression level of known ICP and ICD regulators in immunophenotypes of HCC. Expression levels of 45 previously reported ICPs genes and 26 ICD modulators genes were detected in different subtypes of HCC. In the LIHC cohort, 37/45 of the ICPs were higher in IS1 and IS5, and 16/26 of the ICD regulators were higher in IS3. In the LIRI cohort, 38/45 of the ICPs were more expressed in IS1 and IS5, and 19/26 of the ICD regulators were higher in IS3 (Figure S3A-S3D). Therefore, immunophenotypes can reflect the immunoreactivity of HCC patients to a certain extent and can provide a theoretical basis for selecting beneficiaries. Patients with IS1 and IS5 subtypes may have a stronger ability of tumor immune evasion, and patients with IS3 subtypes have greater immunoreactivity and may be potential beneficiaries of mRNA vaccines.
Association of immunophenotypes with mutational status
Higher tumor mutation burden (TMB) and somatic mutation rates often predict more substantial immune effects against tumors. Therefore, the MuTect2 Annotation mutation dataset from TCGA-LIHC was employed to assess the TMB and number of mutant genes for these five subtypes. In addition, the 10 most commonly genomically altered immune-related genes (Figure S4A,S4B) were demonstrated, and it showed that the high proportion of mutated genes in different immunophenotypes is different. TP53 mutations account for a higher proportion in IS1 and IS5, which means that these two subtypes have a lower probability of responding effectively to DNA damage, and thus have a higher risk of tumorigenesis. In IS2 and IS3, CTNNB1 mutations account for a high proportion, which can lead to the activation of the Wnt/β-catenin signaling pathway, inhibit apoptosis, thereby increasing the growth and spread capacity of tumors. In short, patients with different immunoisotypes had different tumor mutation landscapes, which provided new ideas and insights for tumor immunotherapy.
Association between immunophenotypes and tumor markers
Alpha-fetoprotein (AFP) is a clear prognostic and diagnostic marker for HCC and may indicate cancer progression, poor prognosis, and recurrence. The level of AFP expression in IS1 versus IS5 subtype expression is upregulated (Figure S4C), which is consistent with the poor prognosis of IS1 subtype in the LIHC cohort (Figure 4E). PDCD1 and CD274 encode two immunomodulatory proteins, programmed cell death protein 1 (PD1) and programmed cell death-ligand 1 (PD-L1), respectively, and their high expression is highly correlated with immune evasion by hepatoma cells, resulting in immunotherapy resistance. The upregulation of PDCD1 and CD274 expression levels in IS1 and IS5 subtypes reflects their potential rejection effect on immunotherapy, resulting in low immunogenicity of mRNA vaccines. The expression trends of AFP, PDCD1 and CD274 in the LIRI immunophenotype were similar to those of the LIHC cohort (Figure S4D). In summary, immunophenotypes have certain value in predicting HCC prognosis and immune response effects, which can supplement traditional predictive markers at the genetic level and guide immunotherapy.
Identification of immune gene co-expression modules
WGCNA was used to perform clustering analysis (Figure 7A) and construct an immune gene co-expression network for LIHC cohort, with a soft threshold of 4 (Figure 7B,7C). We used the average linkage hierarchical clustering method to merge closed modules into new modules, with criteria including a height cut-off of 0.20, a deep split distance of 5, and a minimum module size of 30, and then we obtained 7 co-expression modules and 1,339 transcripts (Figure 7D). The Eigengenes of the black module were strongly correlated with those of the blue, brown, and red modules (Figure 7E). Further analysis of the distribution of 5 immunophenotypes in the 6 module genes revealed significant differences in all modules, with IS2 showing the lowest feature genes in the black, blue, brown, blue, red, and yellow modules, and IS1 showing the highest feature genes in the black, blue, brown, and red modules (Figure 7F). Therefore, we observed that IS2 corresponds to the immunologically cold subtype and IS1 corresponds to the immunologically hot subtype, which is consistent with the results of previous immune infiltration analyses.
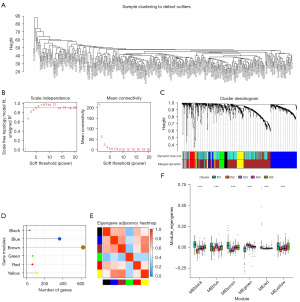
Further prognosis-related analysis showed that the black and blue modules were significantly associated with the prognosis of LIHC (Figure 8A). In addition, the blue and black modules were enriched in immune cell receptor pathways and malignant tumor regulatory pathways such as MAPK signaling and T-cell receptor signaling and showed consistent negative correlations with PCA2 of the immune landscape (Figure 8B-8E). Analysis of prognosis-related genes in the black and blue modules showed that higher expression scores correlated with poor LIHC prognosis (Figure 8F,8G).
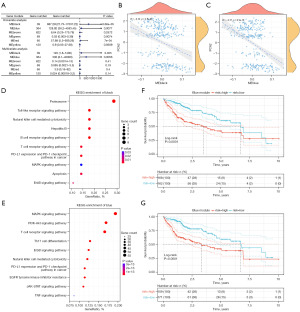
Discussion
The mRNA vaccine is deemed as an epoch-making innovation. It does not need to inject inactivated or deactivated virus into the human body, but through artificial gene fragments to mimic viral attack, mobilizing the body’s immune response, and its effect depends on the immunogenicity of the antigen (30,31). In order to obtain ideal targets for HCC, we first considered the following factors. First, we screened for neoantigens with upregulated expression and poor prognosis to ensure that the vaccine targeted the chance of hits. Second, candidate genes should be distributed in patients with high variability to ensure that more potential beneficiaries are identified. Third, it needs to correlate with APC abundance to ensure that antigen entry into major histocompatibility complex (MHC) triggers an immune cascade that elicits an immune response in T cells (12). Finally, we screened six tumor-specific antigens, AURKA, CDC25C, KPNA2, MCM3, NEK2 and TUBG1, and we also observed upregulation of their expression in tumor tissues in two GEO databases.
These six candidate genes had been previously reported to have potential in the preparation of mRNA vaccines. Overexpression of AURKA is associated with distant metastasis and chemotherapy resistance in HCC (32-34). In addition, its capacity to induce HCC metastasis is demonstrated by its ability to control epithelial-mesenchymal transition and cancer stem cell-like characteristics (32). CDC25C is a gene encoding a cell cycle regulatory protein, and the protein it encodes is a protein kinase phosphatase that primarily regulates the progress of the cell cycle (35). It may participate in CHD1L/TCTP/CDC25C/CDK1-pathway leading to canceration of liver cells, which accelerates mitotic progression and generates a phenotype of aneuploidy (36). Drug experiments have shown that aloperine can induce G2/M cell cycle arrest and produce anti-tumor effects in vitro by downregulating CDC25C (37). KPNA2 encodes a nuclear transport protein, also known as transportin alpha-1 (Trnα1), which regulates nucleocytoplasmic transport (38). A KPNA2-miR139 axis exists in the development of HCC, and overexpression of KPNA2 inhibits the therapeutic effect of miR139, leading to tumor progression (39). The protein expressed by the MCM3 gene plays a key role in the process of DNA replication, participating in the regulation of the cell cycle. Its abnormal expression is related to the poor prognosis of HCC and may become a new diagnostic marker (40). Nude mouse experiments have found that MCM3 promotes radioresistance by activating the NF-κB pathway, enhancing the role of MCM subunits in tumor progression, which may provide a new target for treating HCC (41). NEK2 is upregulated in HCC, especially in late-stage HCC (42). It can activate PAKT/NF-κB signaling pathway to mediate tumor growth, drug resistance, angiogenesis, and metastasis (43). Cell experiments have found that NEK2 induces sorafenib resistance through β-Catenin (44). Interestingly, we found that NEK2 has similar biological functions with AURKA, and together with FOXM1, they lead to the progression and poor prognosis of HCC (43,45). TUBG1 encodes γ-microtubule-associated protein (GMAP), which is essential for cell division and morphological maintenance. TUBG1 plays an important role in a variety of malignancies, but its relationship with HCC has not been widely studied (46). In our experiments, we found that AURKA and CDC25C had higher expression in tumor tissues and suggested a worse prognosis, which validates bioinformatics analysis to some extent, but it requires deep validation to further support. In addition, some studies have shown that high expression of candidate antigen targets is associated with disease progression and poor prognosis in other malignancies, such as prostate cancer (47), lung cancer (48), and breast cancer (49,50). The findings of pan-carcinoma-related research further support the feasibility of antigen targets.
Due to the limited population benefiting from mRNA vaccines, we divided HCC into 5 immunophenotypes based on the specific immune gene collection to select appropriate recipients. In the LIHC and LIRI cohorts, patients with IS2 and IS5 tumors had better prognoses, indicating that immunophenotypes can be used to forecast the prognosis of HCC patients. The significant upregulation of AFP in IS1 and IS5 tumor patients corresponds to poor prognosis in IS1 subtypes, and our immunophenotype can complement traditional tumor markers to predict tumor progression and prognosis more accurately. Programmed cell death 1 (PD-1) is an immunomodulatory protein encoded by the PDCD1 gene. In the immune system, PD-1 binds to its ligand, PD-L1 to inhibit the activity of T cells, thereby regulating the immune response and preventing excessive immune responses from causing damage to their own tissues (51). The expression levels of PDCD1 and CD274 in the IS1 and IS5 subtypes were up-regulated, reflecting their potential rejection effect on immunotherapy, which in turn led to low immunogenicity of mRNA vaccines, reducing vaccine efficacy.
Patients with higher TMB and somatic mutations may be more responsive to mRNA vaccines. Although TMB did not show significant differences between the two combined immunophenotypes (Figure S4B), the proportion of distinct mutant genes also revealed different mutational characteristics of different immunophenotypes. In IS1 and IS5, TP53 mutations account for a higher proportion. In IS2 and IS3, CTNNB1 mutations account for a higher proportion. Calderaro et al. explained that CTNNB1 and TP53 mutations were mutually exclusive from a pathological point of view and defined two groups of HCCs with different characteristics according to it (52). A previous study has shown that when the CTNNB1 mutated, the β-catenin protein might escape degradation, accumulate in the cell, and enter the nucleus, activate the Wnt/β-catenin signaling pathway, promote the proliferation of tumor cells, inhibit apoptosis, and thus increase tumor growth and spread (53). However, the effect of different case subtypes on tumor immunotherapy is still unclear, especially in the field of immunogenicity and immune reactivity of mRNA vaccines.
It is worth noting that immunophenotypes can predict the therapeutic effect of mRNA vaccines. For the expression of immune modulators in different types, we speculate that IS1 and IS5 have inhibitory tumor microenvironments, which may lead to low responsiveness of mRNA vaccines. In addition, the immune landscape shows significant differences in immune composition among different patients, which is detrimental to the development of mRNA vaccines that requires large-scale production. Interestingly, IS1 and IS5 patients are enriched with effector T cells and immunosuppressive cells, and this antagonistic cellular action may cause patients to form a depleted state of effector T cells, making mRNA vaccination less effective. A negative correlation between the upregulation of gene expression in the blue and black module and PCA2 in the immune landscape implies that high expression of these genes may lead to inadequate responsiveness of mRNA vaccines.
In conclusion, our research discovered potential antigens for mRNA vaccines at first, and AURKA and CDC25C were identified as the most promising targets. In order to find potential beneficiaries, we divided HCC into five stable immunophenotypes, and IS2 and IS3 patients are considered to be immunologically cold according to the degree of immune cell enrichment, who are more likely to be potential beneficiaries of mRNA vaccines. Finally, we explored the influencing factors of mRNA vaccine effectiveness, WGCNA results showed that high expression of blue and black module genes may have an inhibitory effect on mRNA vaccine reactivity. This study aims to explore a new way out of immunotherapy for HCC. Its shortcomings are mostly based on theoretical exploration and the validation cohort of relatively small sample size. The follow-up experimental analysis of this study will be continued in the future.
Conclusions
AURKA, CDC25C, KPNA2, MCM3, NEK2 and TUBG1 may be potential targets for mRNA vaccine development for HCC, especially AURKA and CDC25C. HCC patients with IS1 and IS5 subtypes perhaps presents an autoimmunosuppressed state, then IS2 and IS3 subtypes perhaps potential beneficiaries.
Acknowledgments
We thank Dr. Xiaolin Chen from the Department of Breast Surgery of Sun Yat-sen Memorial Hospital for her guidance on data statistics, and we also thank Professor Lehang Lin from the Scientific Research Center of Sun Yat-sen Memorial Hospital for his guidance of the experimental part.
Funding: This research was supported by
Footnote
Data Sharing Statement: Available at https://tcr.amegroups.com/article/view/10.21037/tcr-23-1237/dss
Peer Review File: Available at https://tcr.amegroups.com/article/view/10.21037/tcr-23-1237/prf
Conflicts of Interest: All authors have completed the ICMJE uniform disclosure form (available at https://tcr.amegroups.com/article/view/10.21037/tcr-23-1237/coif) and report that the fund supported the experimental parts (reagent purchase, experimental materials, etc.) involved in this manuscript was Basic and Applied Basic Research Foundation of Guangdong Province (Grant No. 2020A1515011379 to He-Yun Zhang). The authors have no other conflicts of interest to declare.
Ethical Statement: The authors are accountable for all aspects of the work in ensuring that questions related to the accuracy or integrity of any part of the work are appropriately investigated and resolved. The studies involving human participants were reviewed and approved by the Ethics Committee of Sun Yat-sen Memorial Hospital (ID: SYSKY-2023-1003-01) and were conducted in accordance with the Declaration of Helsinki (as revised in 2013). The patients/participants provided their written informed consent to participate in study.
Open Access Statement: This is an Open Access article distributed in accordance with the Creative Commons Attribution-NonCommercial-NoDerivs 4.0 International License (CC BY-NC-ND 4.0), which permits the non-commercial replication and distribution of the article with the strict proviso that no changes or edits are made and the original work is properly cited (including links to both the formal publication through the relevant DOI and the license). See: https://creativecommons.org/licenses/by-nc-nd/4.0/.
References
- Yang JD, Hainaut P, Gores GJ, et al. A global view of hepatocellular carcinoma: trends, risk, prevention and management. Nat Rev Gastroenterol Hepatol 2019;16:589-604. [Crossref] [PubMed]
- Zhou M, Wang H, Zeng X, et al. Mortality, morbidity, and risk factors in China and its provinces, 1990-2017: a systematic analysis for the Global Burden of Disease Study 2017. Lancet 2019;394:1145-58. [Crossref] [PubMed]
- Sung H, Ferlay J, Siegel RL, et al. Global Cancer Statistics 2020: GLOBOCAN Estimates of Incidence and Mortality Worldwide for 36 Cancers in 185 Countries. CA Cancer J Clin 2021;71:209-49. [Crossref] [PubMed]
- Huang DQ, Mathurin P, Cortez-Pinto H, et al. Global epidemiology of alcohol-associated cirrhosis and HCC: trends, projections and risk factors. Nat Rev Gastroenterol Hepatol 2023;20:37-49. [Crossref] [PubMed]
- Shukla SK, Kumar V. Hepatitis B virus X protein and c-Myc cooperate in the upregulation of ribosome biogenesis and in cellular transformation. FEBS J 2012;279:3859-71. [Crossref] [PubMed]
- Rimassa L, Pressiani T, Merle P. Systemic Treatment Options in Hepatocellular Carcinoma. Liver Cancer 2019;8:427-46. [Crossref] [PubMed]
- Mizukoshi E, Kaneko S. Immune cell therapy for hepatocellular carcinoma. J Hematol Oncol 2019;12:52. [Crossref] [PubMed]
- Heimbach JK, Kulik LM, Finn RS, et al. AASLD guidelines for the treatment of hepatocellular carcinoma. Hepatology 2018;67:358-80. [Crossref] [PubMed]
- Emens LA. Roadmap to a better therapeutic tumor vaccine. Int Rev Immunol 2006;25:415-43. [Crossref] [PubMed]
- Bouzid R, Peppelenbosch M, Buschow SI. Opportunities for Conventional and in Situ Cancer Vaccine Strategies and Combination with Immunotherapy for Gastrointestinal Cancers, A Review. Cancers (Basel) 2020;12:1121. [Crossref] [PubMed]
- Sun K, Wang L, Zhang Y. Dendritic cell as therapeutic vaccines against tumors and its role in therapy for hepatocellular carcinoma. Cell Mol Immunol 2006;3:197-203. [PubMed]
- Pardi N, Hogan MJ, Porter FW, et al. mRNA vaccines - a new era in vaccinology. Nat Rev Drug Discov 2018;17:261-79. [Crossref] [PubMed]
- Lorentzen CL, Haanen JB, Met Ö, et al. Clinical advances and ongoing trials on mRNA vaccines for cancer treatment. Lancet Oncol 2022;23:e450-8. [Crossref] [PubMed]
- Moderna. Moderna research product pipeline. Available online: https://www.modernatx.com/research/product-pipeline? (accessed March 31, 2022).
- King G, Green S, Chiorean EG. Finding a role for cancer vaccines in pancreatic cancer: a model of resilience. Hepatobiliary Surg Nutr 2022;11:115-8. [Crossref] [PubMed]
- Awad MM, Govindan R, Balogh KN, et al. Personalized neoantigen vaccine NEO-PV-01 with chemotherapy and anti-PD-1 as first-line treatment for non-squamous non-small cell lung cancer. Cancer Cell 2022;40:1010-1026.e11. [Crossref] [PubMed]
- Wang H, Huo X, Yang XR, et al. STAT3-mediated upregulation of lncRNA HOXD-AS1 as a ceRNA facilitates liver cancer metastasis by regulating SOX4. Mol Cancer 2017;16:136. [Crossref] [PubMed]
- Chaussabel D, Baldwin N. Democratizing systems immunology with modular transcriptional repertoire analyses. Nat Rev Immunol 2014;14:271-80. [Crossref] [PubMed]
- Li S, Rouphael N, Duraisingham S, et al. Molecular signatures of antibody responses derived from a systems biology study of five human vaccines. Nat Immunol 2014;15:195-204. [Crossref] [PubMed]
- Love MI, Huber W, Anders S. Moderated estimation of fold change and dispersion for RNA-seq data with DESeq2. Genome Biol 2014;15:550. [Crossref] [PubMed]
- Tang Z, Kang B, Li C, et al. GEPIA2: an enhanced web server for large-scale expression profiling and interactive analysis. Nucleic Acids Res 2019;47:W556-60. [Crossref] [PubMed]
- Gao J, Aksoy BA, Dogrusoz U, et al. Integrative analysis of complex cancer genomics and clinical profiles using the cBioPortal. Sci Signal 2013;6:pl1. [Crossref] [PubMed]
- Cerami E, Gao J, Dogrusoz U, et al. The cBio cancer genomics portal: an open platform for exploring multidimensional cancer genomics data. Cancer Discov 2012;2:401-4. [Crossref] [PubMed]
- Wilkerson MD, Hayes DN. ConsensusClusterPlus: a class discovery tool with confidence assessments and item tracking. Bioinformatics 2010;26:1572-3. [Crossref] [PubMed]
- Trapnell C, Cacchiarelli D, Grimsby J, et al. The dynamics and regulators of cell fate decisions are revealed by pseudotemporal ordering of single cells. Nat Biotechnol 2014;32:381-6. [Crossref] [PubMed]
- Qiu X, Hill A, Packer J, et al. Single-cell mRNA quantification and differential analysis with Census. Nat Methods 2017;14:309-15. [Crossref] [PubMed]
- Langfelder P, Horvath S. WGCNA: an R package for weighted correlation network analysis. BMC Bioinformatics 2008;9:559. [Crossref] [PubMed]
- Kanehisa M, Goto S. KEGG: kyoto encyclopedia of genes and genomes. Nucleic Acids Res 2000;28:27-30. [Crossref] [PubMed]
- Sherman BT, Hao M, Qiu J, et al. DAVID: a web server for functional enrichment analysis and functional annotation of gene lists (2021 update). Nucleic Acids Res 2022;50:W216-21. [Crossref] [PubMed]
- Thorsson V, Gibbs DL, Brown SD, et al. The Immune Landscape of Cancer. Immunity 2018;48:812-830.e14. [Crossref] [PubMed]
- Miao L, Zhang Y, Huang L. mRNA vaccine for cancer immunotherapy. Mol Cancer 2021;20:41. [Crossref] [PubMed]
- Jeng YM, Peng SY, Lin CY, et al. Overexpression and amplification of Aurora-A in hepatocellular carcinoma. Clin Cancer Res 2004;10:2065-71. [Crossref] [PubMed]
- Chen C, Song G, Xiang J, et al. AURKA promotes cancer metastasis by regulating epithelial-mesenchymal transition and cancer stem cell properties in hepatocellular carcinoma. Biochem Biophys Res Commun 2017;486:514-20. [Crossref] [PubMed]
- Zhang K, Chen J, Chen D, et al. Aurora-A promotes chemoresistance in hepatocelluar carcinoma by targeting NF-kappaB/microRNA-21/PTEN signaling pathway. Oncotarget 2014;5:12916-35. [Crossref] [PubMed]
- Boutros R, Lobjois V, Ducommun B. CDC25 phosphatases in cancer cells: key players? Good targets? Nat Rev Cancer 2007;7:495-507. [Crossref] [PubMed]
- Chan TH, Chen L, Liu M, et al. Translationally controlled tumor protein induces mitotic defects and chromosome missegregation in hepatocellular carcinoma development. Hepatology 2012;55:491-505. [Crossref] [PubMed]
- Liu JS, Huo CY, Cao HH, et al. Aloperine induces apoptosis and G2/M cell cycle arrest in hepatocellular carcinoma cells through the PI3K/Akt signaling pathway. Phytomedicine 2019;61:152843. [Crossref] [PubMed]
- Guo X, Wang Z, Zhang J, et al. Upregulated KPNA2 promotes hepatocellular carcinoma progression and indicates prognostic significance across human cancer types. Acta Biochim Biophys Sin (Shanghai) 2019;51:285-92. [Crossref] [PubMed]
- Zan Y, Wang B, Liang L, et al. MicroRNA-139 inhibits hepatocellular carcinoma cell growth through down-regulating karyopherin alpha 2. J Exp Clin Cancer Res 2019;38:182. [Crossref] [PubMed]
- Li HT, Wei B, Li ZQ, et al. Diagnostic and prognostic value of MCM3 and its interacting proteins in hepatocellular carcinoma. Oncol Lett 2020;20:308. [Crossref] [PubMed]
- Yang Q, Xie B, Tang H, et al. Minichromosome maintenance 3 promotes hepatocellular carcinoma radioresistance by activating the NF-κB pathway. J Exp Clin Cancer Res 2019;38:263. Erratum in: J Exp Clin Cancer Res 2019;38:336 Erratum in: J Exp Clin Cancer Res 2019;38:387. [Crossref] [PubMed]
- Wonsey DR, Follettie MT. Loss of the forkhead transcription factor FoxM1 causes centrosome amplification and mitotic catastrophe. Cancer Res 2005;65:5181-9. [Crossref] [PubMed]
- Wu SM, Lin SL, Lee KY, et al. Hepatoma cell functions modulated by NEK2 are associated with liver cancer progression. Int J Cancer 2017;140:1581-96. [Crossref] [PubMed]
- Deng L, Sun J, Chen X, et al. Nek2 augments sorafenib resistance by regulating the ubiquitination and localization of β-catenin in hepatocellular carcinoma. J Exp Clin Cancer Res 2019;38:316. [Crossref] [PubMed]
- Calvisi DF, Pinna F, Ladu S, et al. Forkhead box M1B is a determinant of rat susceptibility to hepatocarcinogenesis and sustains ERK activity in human HCC. Gut 2009;58:679-87. [Crossref] [PubMed]
- Alvarado-Kristensson M. γ-tubulin as a signal-transducing molecule and meshwork with therapeutic potential. Signal Transduct Target Ther 2018;3:24. [Crossref] [PubMed]
- Iglesias-Gato D, Thysell E, Tyanova S, et al. The Proteome of Prostate Cancer Bone Metastasis Reveals Heterogeneity with Prognostic Implications. Clin Cancer Res 2018;24:5433-44. [Crossref] [PubMed]
- Stern E, Pines G, Lazar LO, et al. CDC25C Protein Expression Correlates with Tumor Differentiation and Clinical Outcomes in Lung Adenocarcinoma. Biomedicines 2023;11:362. [Crossref] [PubMed]
- Zeng X, Shi G, He Q, et al. Screening and predicted value of potential biomarkers for breast cancer using bioinformatics analysis. Sci Rep 2021;11:20799. [Crossref] [PubMed]
- Løkkegaard S, Elias D, Alves CL, et al. MCM3 upregulation confers endocrine resistance in breast cancer and is a predictive marker of diminished tamoxifen benefit. NPJ Breast Cancer 2021;7:2. [Crossref] [PubMed]
- Dammeijer F, van Gulijk M, Mulder EE, et al. The PD-1/PD-L1-Checkpoint Restrains T cell Immunity in Tumor-Draining Lymph Nodes. Cancer Cell 2020;38:685-700.e8. [Crossref] [PubMed]
- Calderaro J, Couchy G, Imbeaud S, et al. Histological subtypes of hepatocellular carcinoma are related to gene mutations and molecular tumour classification. J Hepatol 2017;67:727-38. [Crossref] [PubMed]
- Liu J, Xiao Q, Xiao J, et al. Wnt/β-catenin signalling: function, biological mechanisms, and therapeutic opportunities. Signal Transduct Target Ther 2022;7:3. [Crossref] [PubMed]