A prognostic nomogram based on least absolute shrinkage and selection operator Cox regression in patients with pulmonary large-cell neuroendocrine carcinoma
Highlight box
Key findings
• Age, bone metastasis, liver metastasis, surgery, and chemotherapy were identified as independent risk factors for pulmonary large-cell neuroendocrine carcinoma (LCNEC).
What is known and what is new?
• Pulmonary LCNEC is a rare subtype of breast cancer.
• Our study is one of the largest population-based studies to estimate the prognosis of pulmonary LCNEC patients. Our prognostic nomogram may be very helpful in estimating the overall survival of patients with pulmonary LCNEC.
What is the implication, and what should change now?
• Surgery contributed to a better prognosis for pulmonary LCNEC patients. Importantly, prospective studies need to be conducted to explore tailored treatments and establish surveillance guidelines for pulmonary LCNEC patients at different stages.
Introduction
Large-cell neuroendocrine carcinoma (LCNEC) is an uncommon and extremely aggressive neuroendocrine tumor subtype with a poor prognosis (1). In 2010, it had an incidence of 1.8 per 100,000 people (2). It can affect any part of the body; however, the pulmonary and gastrointestinal tracts account for the majority of cases (3-6). Pulmonary LCNEC comprises approximately 3% of lung cancers (7). In 2015, the World Health Organization defined LCNEC as morphological non-small cell lung cancer (NSCLC) with the histopathological features of neuroendocrine cancer and the immunohistochemical expression of neuroendocrine markers. In addition, a subgroup of pulmonary LCNEC is described as “LCNEC with components of adenocarcinoma, squamous cell carcinoma, spindle cell carcinoma, and/or giant-cell carcinoma” (8,9).
The International Association for the Study of Lung Cancer introduced the 7th edition of the American Joint Committee on Cancer (AJCC) tumor, node, and metastasis (TNM) staging method of malignant tumors in 2010 and it is thought to provide the best staging approach for patients with LCNEC (10,11). However, this system was not specifically designed to predict outcomes for patients with pulmonary LCNEC; thus, new methods need to be developed to identify independent risk variables for the construction of prognostic prediction models.
In 1997, Tibshirani et al. presented the least absolute shrinkage and selection operator (LASSO) Cox regression method for variable factor selection, whereby a LASSO regression analysis and a multivariate Cox regression analysis were used to identify prognostic factors, and with which a more accurate model was constructed (12-14). A great deal of research that has employed the LASSO Cox regression method to identify risk genes has shown that it is essential in the study of cancer (15,16). However, few studies have used the LASSO Cox regression method to screen clinical indicators to establish prognostic prediction models (17). He et al. generated a nomogram model to predict overall survival (OS) in pulmonary LCNEC, in which univariate and multivariate Cox regression models were used to determine the demographic and clinicopathological features associated with OS (18). Similarly, Ma et al. generated a nomogram model for predicting cancer-specific survival in pulmonary LCNEC patients (19). Nomograms have demonstrated an ability to identify influencing factors and provide more accurate, individualized predictions. Additionally, a nomogram is a tool that combines numerous prognostic signs and provides a more convenient and straightforward way to predict the likelihood of an occurrence than traditional approaches (20,21).
Thus, we created a distinctive nomogram using a sizable patient cohort from the Surveillance, Epidemiology, and End Results (SEER) database, which collects and compiles data on cancer incidence, prevalence, treatment, and survival from diverse locations around the United States. This nomogram aims to offer more accurate predictions of OS for patients diagnosed with pulmonary LCNEC. In addition, the newly created nomogram was compared to the 7th edition of the AJCC TNM staging system to ascertain whether it was able to more accurately predict patient prognosis. We present this article in accordance with the TRIPOD reporting checklist (available at https://tcr.amegroups.com/article/view/10.21037/tcr-23-1061/rc).
Methods
Study population and data processing
In this comprehensive retrospective analysis based on a large population, data were extracted from the SEER database using SEER*Stat 8.4.01. The SEER database includes information about patients diagnosed with LCNEC. The National Cancer Institute’s 17 cancer registries provide information to the SEER database (22). The SEER database’s most recent follow-up and prognosis data were published in November 2021. We carried out this study in accordance with the guidelines of the Declaration of Helsinki (as revised in 2013).
Patients who were diagnosed with LCNEC between 2010 and 2019 were first identified in the SEER database. Patients were included in the study if they met the following inclusion criteria: (I) had at least one the following SEER primary site codes: C34.0, C34.1, C34.2, C34.3, C34.8, or C34.9; and (II) had the International Classification of Diseases for Oncology, 3rd Edition, histology code of 8013/3, which was used to diagnose LCNEC and to describe the condition. Patients were excluded from the study if they met any of the following exclusion criteria: (I) had a survival time of 0 months or less; (II) were death certificate-only cases; and/or (III) lacked or had insufficient information about their race, marital status, primary site, laterality, T stage, N stage, grade, surgery, or radiation treatment. Ultimately, 1,864 patients with pulmonary LCNEC were included in the primary cohort. The detailed screening process is displayed in Figure 1.

The following data were obtained from the SEER database: demographics (gender, race, age, and marital status at time of diagnosis); tumor characteristics (primary site, laterality, histologic grade, tumor size, AJCC stage, AJCC-T stage, AJCC-N stage, AJCC-M stage, brain metastasis, bone metastasis, liver metastasis, and lung metastasis); and treatment information (surgery to primary site, radiation, and chemotherapy); and follow-up information (survival time and survival status).
Retrospective reviews of the patients’ medical records were conducted to identify the demographic data and relevant clinicopathological features, such as age (0–59 years old or ≥60 years old), gender (male or female), race (white, black, or other; that is, American Indian/Alaska Native, Asian/Pacific Islander), marital status (married, single, widowed/divorced, or other/unknown), primary site (main bronchus, upper lobe, middle lobe, or lower lobe), tumor stage as per the 7th edition of the AJCC TNM staging system (occult, I, II, III, or IV), T stage as per the 7th edition of the AJCC TNM staging system (TX, T0, T1, T2, T3, or T4), N stage as per the 7th edition of the AJCC TNM staging system (NX, N0, N1, N2, or N3), M stage as per the 7th edition of the AJCC TNM staging system (M0 or M1). The following data were also collected in relation to metastasis status: brain (no/unknown or yes), bone (no/unknown or yes), liver (no/unknown, yes), and lung (no/unknown, yes). Additionally, the following treatment data were collected: surgery (no/unknown or yes), radiotherapy (no/unknown or yes), chemotherapy (no/unknown or yes). Data were also collected on patients’ OS. In this study, comprehensive data analyses were completed.
Follow-up
The primary outcome of the study was OS, which was defined as the interval between the diagnosis of pulmonary LCNEC until the final follow-up or death. Patients who participated in this study had a known survival status and a known survival period. The median follow-up time was 12 months (interquartile range: 5–33 months; range: 1–119 months) in the entire cohort.
Statistical analysis
In this study, 1864 eligible patients with pulmonary LCNEC were randomly divided into a training cohort and a validation cohort at a ratio of 7:3 using R (version 3.5.1) and the “caret” package. All the categorical variables were summarized as the count and percentage. The frequency distributions of the demographic, clinical, and pathologic characteristics of the eligible patients were compared between the training and validation sets using the chi-square test.
We converted the continuous variables into classification variables to increase the objectivity and simplicity of the model. In the training cohort, the covariates (e.g., age, gender, race, marital status, primary site, laterality, tumor size, stage, T stage, N stage, and M stage as per AJCC 7th edition, as well as bone metastasis, brain metastasis, liver metastasis, and lung metastasis, and surgery and chemotherapy) were entered into a LASSO regression using the R package “glmnet”, and “lambda.1se” was used to select the variables, and a 10-fold cross-validation was used to determine the optimal LASSO penalty. After testing for collinearity, a forward stepwise multivariate Cox proportional hazard regression model was developed to estimate the potential prognostic factors based on the LASSO regression results. Based on the results of the multivariable analysis, five significant variables (P<0.01) were selected and incorporated into the nomogram to provide visualized risk prediction; the nomogram adopted 3- and 5-year OS as the endpoints.
The accuracy of the nomogram for predicting visualized risk and survival outcomes was assessed using the Harrell’s concordance index (C-index) as an evaluation index (23). To establish the concordance between the predicted and observed 3- and 5-year OS outcomes and to evaluate the nomogram’s predictive accuracy in a well-calibrated model, we developed calibration curves for both the training and validation cohorts using 1,000 bootstrap re-samples. A decision curve analysis (DCA), which has specific advantages in evaluating the clinical value and utility of nomograms, was used to test the model’s reliability (24). We also calculated the area under the curve (AUC) of the time-dependent receiver operating characteristic (ROC) curve to evaluate the discrimination ability and calibration ability of the new nomogram and the 7th edition of the AJCC TNM staging system in the two cohorts. Finally, the net classification improvement (NRI) and integrated discrimination improvement (IDI) indexes were used to compare the clinical utility and net clinical benefits between the two new models and the 7th edition of the AJCC TNM staging system.
The survival curves for several categories of individual predictors were compared using the Kaplan-Meier (K-M) method, and the significance of these differences was assessed using the log-rank test. With the help of the “survivalROC” R package, a K-M curve for OS was also established. This package generated time-dependent ROC curves for the nomogram and all the independent prognostic variables at 3 and 5 years, and it then applied the corresponding time-dependent AUCs to demonstrate the discrimination ability of the model. All the LCNEC patients were split into high- and low-risk groups based on an appropriate cut-off value that was calculated based on the ROC analysis. Using the K-M analysis, survival curves for the low- and high-risk groups were plotted, and the log-rank test was used to demonstrate the difference in OS status between the two groups. A P value <0.05 was considered statistically significant.
The statistical analysis was conducted with SPSS 24.0 (IBM, Chicago, IL, USA), R software (version 3.6.3; http://www.Rproject.org), and MedCalc19.2.0. The categorical variables were classified based on the clinical findings. SPSS 24.0 was used to compare the clinicopathological characteristics between the two cohorts. “Glmnet” package was used to perform the LASSO Cox regression. The “foreign” package, “survival” package, and “rms” package were used to plot the nomograms and calibration plots and calculate the C-index. The “nricens” package for used to calculate the NRI index. “Stdca.R” was downloaded from Memorial Sloan Kettering Cancer Center (www.mskcc.org) and was used to conduct the DCA. The “IDI.INF” package was used to calculate the IDI index. The “time ROC” package was used to calculate the time-dependent ROC curves. The “survivalROC” package was used to generate the K-M curves for OS.
Results
Clinical characteristics of the participants
Based on the screening criteria, the data of 3,494 patients were initially collected from the SEER database. The data of 1,630 patients were then excluded. Ultimately, the final sample comprised 1,864 patients. The cohort was then divided into the following two groups at a 7:3 ratio: the training group (comprising 1,308 patients) and the validation group (comprising 556 cases). The clinicopathological and demographic characteristics of the patients are presented in Table 1. Among the patients, 868 were female and 996 were male, and 83.9% of the population was White, 11.9% was Black, and 4.2% were other. The upper lobe was the most common primary site, accounting for 55.0% of cases. The most common distant metastasis organ was the brain, accounting for 17.1% of cases, followed by bone (15.7%), liver (15.2%), and lung (10.0%). Additionally, 35.6%, 35.6%, and 56.6% of the patients received surgery, radiation, and chemotherapy, respectively. Finally, the results of the Chi-square test showed that except for radiation, there were no statistical differences between the two cohorts in terms of the clinicopathological characteristics (P<0.05).
Table 1
Characteristics | All patients (n=1,864) | Training set (n=1,308) | Validation set (n=556) | P value |
---|---|---|---|---|
Age, years | 0.389 | |||
0–59 | 544 (29.2) | 374 (28.6) | 170 (30.6) | |
≥60 | 1,320 (70.8) | 934 (71.4) | 386 (69.4) | |
Gender | 0.168 | |||
Male | 996 (53.4) | 713 (54.5) | 283 (50.9) | |
Female | 868 (46.6) | 595 (45.5) | 273 (49.1) | |
Race | 0.471 | |||
White | 1,563 (83.9) | 1,090 (83.3) | 473 (85.1) | |
Black | 222 (11.9) | 158 (12.1) | 64 (11.5) | |
Other | 79 (4.2) | 60 (4.6) | 19 (3.4) | |
Marital status | 0.106 | |||
Married | 964 (51.7) | 694 (53.1) | 270 (48.6) | |
Single | 281 (15.1) | 199 (15.2) | 82 (14.7) | |
Widowed/divorced | 497 (26.7) | 339 (25.9) | 158 (28.4) | |
Other/unknown | 122 (6.5) | 76 (5.8) | 46 (8.3) | |
Primary site | 0.623 | |||
Main bronchus | 75 (4.0) | 48 (3.7) | 27 (4.9) | |
Upper lobe | 1,025 (55.0) | 732 (56.0) | 293 (52.7) | |
Middle lobe | 75 (4.0) | 54 (4.1) | 21 (3.8) | |
Lower lobe | 450 (24.1) | 310 (23.7) | 140 (25.2) | |
Overlapping lesion of lung | 21 (1.1) | 16 (1.2) | 5 (0.9) | |
Lung, NOS | 218 (11.7) | 148 (11.3) | 70 (12.6) | |
Laterality | 0.345 | |||
Right | 1,046 (56.1) | 736 (56.3) | 310 (55.8) | |
Left | 734 (39.4) | 519 (39.7) | 215 (38.7) | |
Other | 84 (4.5) | 53 (4.1) | 31 (5.6) | |
Tumor size (mm) | 0.057 | |||
0–19 | 344 (18.5) | 223 (17.0) | 121 (21.8) | |
20–39 | 562 (30.2) | 411 (31.4) | 151 (27.2) | |
40–79 | 520 (27.9) | 361 (27.6) | 159 (28.6) | |
≥80 | 438 (23.5) | 313 (23.9) | 125 (22.5) | |
AJCC 7th edition | 0.204 | |||
Occult | 6 (0.3) | 4 (0.3) | 2 (0.4) | |
I | 407 (21.8) | 281 (21.5) | 126 (22.7) | |
II | 190 (10.2) | 135 (10.3) | 55 (9.9) | |
III | 364 (19.5) | 239 (18.3) | 125 (22.5) | |
IV | 897 (48.1) | 649 (49.6) | 248 (44.6) | |
T stage | 0.347 | |||
TX | 155 (8.3) | 105 (8.0) | 50 (9.0) | |
T0 | 16 (0.9) | 11 (0.8) | 5 (0.9) | |
T1 | 454 (24.4) | 305 (23.3) | 149 (26.8) | |
T2 | 521 (28.0) | 375 (28.7) | 146 (26.3) | |
T3 | 364 (19.5) | 251 (19.2) | 113 (20.3) | |
T4 | 354 (19.0) | 261 (20.0) | 93 (16.7) | |
N stage | 0.643 | |||
NX | 49 (2.6) | 30 (2.3) | 19 (3.4) | |
N0 | 768 (41.2) | 536 (41.0) | 232 (41.7) | |
N1 | 175 (9.4) | 121 (9.3) | 54 (9.7) | |
N2 | 621 (33.3) | 441 (33.7) | 180 (32.4) | |
N3 | 251 (13.5) | 180 (13.8) | 71 (12.8) | |
M stage | 0.053 | |||
M0 | 967 (51.9) | 659 (50.4) | 308 (55.4) | |
M1 | 897 (48.1) | 649 (49.6) | 248 (44.6) | |
Bone metastasis | 0.478 | |||
No/unknown | 1,572 (84.3) | 1,098 (83.9) | 474 (85.3) | |
Yes | 292 (15.7) | 210 (16.1) | 82 (14.7) | |
Brain metastasis | 0.185 | |||
No/unknown | 1,546 (82.9) | 1,075 (82.2) | 471 (84.7) | |
Yes | 318 (17.1) | 233 (17.8) | 85 (15.3) | |
Liver metastasis | 0.080 | |||
No/unknown | 1,581 (84.8) | 1,097 (83.9) | 484 (87.1) | |
Yes | 283 (15.2) | 211 (16.1) | 72 (12.9) | |
Lung metastasis | 0.524 | |||
No/unknown | 1,677 (90.0) | 1,173 (89.7) | 504 (90.6) | |
Yes | 187 (10.0) | 135 (10.3) | 52 (9.4) | |
Surgery of primary site | 0.602 | |||
No/unknown | 1,200 (64.4) | 847 (64.8) | 353 (63.5) | |
Yes | 664 (35.6) | 461 (35.2) | 203 (36.5) | |
Radiation | 0.001 | |||
No/unknown | 1,200 (64.4) | 719 (55.0) | 353 (63.5) | |
Yes | 664 (35.6) | 589 (45.0) | 203 (36.5) | |
Chemotherapy | 0.561 | |||
No/unknown | 809 (43.4) | 562 (43.0) | 247 (44.4) | |
Yes | 1,055 (56.6) | 746 (57.0) | 309 (55.6) |
Data are presented as n (%). LCLEC, large-cell neuroendocrine carcinoma; NOS, not otherwise specified; AJCC, American Joint Committee on Cancer.
Nomogram construction
We used LASSO regression and multivariate Cox regression analyses to select the optimal variables for the predictive model construction. The LASSO regression was applied to analyze the correlation between the 18 variables and OS; “lambda.1se” was used to select the variables; the value of log (λ) was –2.506296. All the eight variables with non-zero coefficients (i.e., age, tumor stage, N stage, M stage, bone metastasis, liver metastasis, surgery, and chemotherapy) were selected for the multivariate analysis (Figure 2). After the multivariate Cox analysis of the eight variable factors, five clinical variable factors were found to be associated with OS (P≤0.05). The results of the multivariate Cox analysis are summarized in Table 2. Finally, based on the results, age [≥60 years, hazard ratio (HR) =1.21, 95% confidence interval (CI): 1.06–1.38], bone metastasis (yes, HR =1.20, 95% CI: 1.01–1.43), liver metastasis (yes, HR =1.70, 95% CI: 1.43–2.03), surgery (yes, HR =0.56, 95% CI: 0.46–0.69), and chemotherapy (yes, HR =0.49, 95% CI: 0.42–0.56) were identified as independent risk factors for OS. We used these five independent prognostic factors for OS to construct 3- and 5-year OS nomograms (Figure 3), and each subgroup within these variables was allocated a score (Table 3).
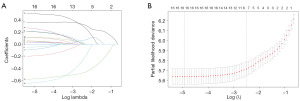
Table 2
Characteristic | HR | 95% CI | P value |
---|---|---|---|
Age, years | |||
0–59 | Reference | ||
≥60 | 1.21 | 1.06–1.38 | 0.01 |
AJCC 7th edition | |||
Occult | Reference | ||
I | 0.69 | 0.22–2.18 | 0.53 |
II | 1.33 | 0.42–4.27 | 0.63 |
III | 1.61 | 0.51–5.10 | 0.42 |
IV | 2.64 | 0.47–1.03 | 0.10 |
N stage | |||
NX | Reference | ||
N0 | 0.69 | 0.7–1.03 | 0.07 |
N1 | 0.84 | 0.55–1.29 | 0.43 |
N2 | 0.92 | 0.63–1.35 | 0.67 |
N3 | 1.19 | 0.80–1.75 | 0.39 |
M stage | |||
M0 | Reference | ||
M1 | NA | NA | NA |
Bone metastasis | |||
No/unknown | Reference | ||
Yes | 1.20 | 1.01–1.43 | 0.04 |
Liver metastasis | |||
No/unknown | Reference | ||
Yes | 1.70 | 1.43–2.03 | <0.01 |
Surgery | |||
No/unknown | Reference | ||
Yes | 0.56 | 0.46–0.69 | <0.01 |
Chemotherapy | |||
No/unknown | Reference | ||
Yes | 0.49 | 0.42–0.56 | <0.01 |
OS, overall survival; HR, hazard ratio; CI, confidence interval; AJCC, American Joint Committee on Cancer; NA, not applicable.
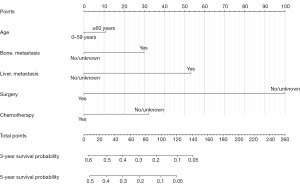
Table 3
Characteristics | Points |
---|---|
Age, years | |
0–59 | 0 |
≥60 | 11 |
Bone metastasis | |
No/unknown | 0 |
Yes | 30 |
Liver metastasis | |
No/unknown | 0 |
Yes | 53 |
Surgery | |
No/unknown | 100 |
Yes | 0 |
Chemotherapy | |
No/unknown | 32 |
Yes | 0 |
Validation of nomogram
The C-indexes of the nomogram were 0.716 (95% CI: 0.687–0.745) and 0.708 (95% CI: 0.661–0.755) in the training cohort and validation cohort, respectively. While the C-indexes of the 7th edition of the AJCC TNM staging system were 0.685 (95% CI: 0.668–0.722) and 0.705 (95% CI: 0.660–0.750) in the training and validation cohorts, respectively. These results indicated that the new model had a great ability to predict for LCNEC.
We also generated calibration curves to assess the calibration ability of the nomogram in the two cohorts. Calibration plots of the nomogram’s prediction accuracy are shown in Figure 4. There was good agreement between actual and predicted 3- and 5-year OS. Thus, we performed a DCA to compare the clinical usability and benefits between the nomogram and the 7th edition of the AJCC TNM staging system. We found that the nomogram could better predict 3-year OS and had more clinical net benefits than the 7th edition of the AJCC TNM staging system for a specific range of threshold probabilities in both the training and validation cohorts (Figure 5A,5B). Similar result was also observed for the 5-year OS prediction (Figure 5C,5D). Finally, the NRI and IDI indexes were also calculated to evaluate the accuracy of the nomogram for predicting OS compared with the 7th edition of the AJCC TNM staging system. In the training cohort, the NRI values were 0.296 (95% CI: −0.142–0.349) and 0.161 (95% CI: 0.032–0.252), and the IDI values were 0.054 (95% CI: 0.030–0.082, P<0.01) and 0.055 (95% CI: 0.031–0.089, P<0.01) for 3- and 5-year OS, respectively. In the validation cohort, the NRI values were 0.289 (95% CI: −0.177–0.409) and –0.009 (95% CI: −0.144–0.209), and the IDI values were 0.053 (95% CI: 0.006–0.119, P=0.030) and 0.041 (95% CI: −0.004–0.094, P=0.090) for 3- and 5-year OS, respectively.
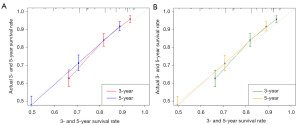
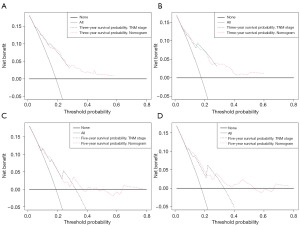
We applied the time-dependent ROC curves to compare the sensitivity and specificity of our new nomogram and the 7th edition of the AJCC TNM staging system at 3 and 5 years. We calculated the AUCs for the new nomogram and the 7th edition of the AJCC TNM staging system. In the training cohort, the AUCs for predicting 3-year OS were 0.811 and 0.820, and the AUCs for predicting 5-year OS were 0.821 and 0.828 for the nomogram and the 7th edition of the AJCC TNM staging system, respectively. While in the validation cohort, the AUCs for predicting 3-year OS were 0.791 and 0.822, and the AUCs for 5-year OS were 0.806 and 0.792 for the nomogram and the 7th edition of the AJCC TNM staging system, respectively (Figure 6).
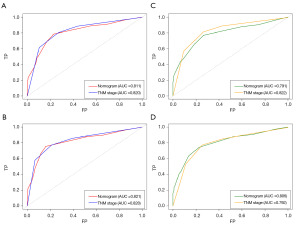
K-M curves of significant features
In our study, all five potential predictors of survival were found to be useful in predicting OS in the training cohort (Figure 7A-7E). Patients aged over 60 years had a shorter OS than those aged 0–59 years. Patients without bone and liver metastases had better OS than those with bone and liver metastases. Patients who received surgery had a better OS than those who did not receive surgery.Patients who received chemotherapy had a shorter OS than those who did not receive chemotherapy, but there is no statistically significant difference (P=0.12). In addition, the K-M curves showed that pulmonary LCNEC patients with an advanced tumor stage as per the 7th edition of the AJCC TNM staging system had worse OS than those with an earlier stage. According to the K-M curves used in our study for the 7th edition of the AJCC TNM staging system, patients with stage III and IV tumors had a noticeably worse prognosis than those with stage I and II tumors (Figure 7F).
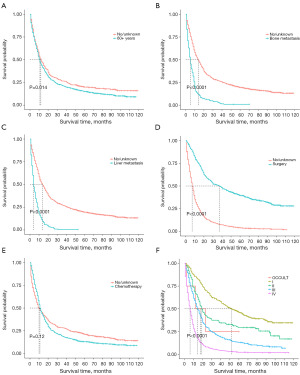
We used time-dependent ROC curves to assess the discrimination ability of the nomogram in the two cohorts. We calculated the AUCs of the new nomogram in predicting 3-year OS (0.811 vs. 0.791) and 5-year OS (0.821 vs. 0.806) for the training cohort (Figure 8A) and the validation cohort (Figure 8B). Further, the patients were divided into low- and high-risk groups based on the cut-off values of the time-dependent ROC curve at a survival time of 5 years in the training cohort (AUC =0.811, cut-off =0.668) and validation cohort (AUC =0.821, cut-off =0.626. Survival curves for the low- and high-risk groups were plotted using a K-M analysis. Patients in the high-risk groups had significantly lower chances of surviving than those in the low-risk groups (Figure 8C,8D), which suggests that the developed nomogram can successfully identify high-risk patients.
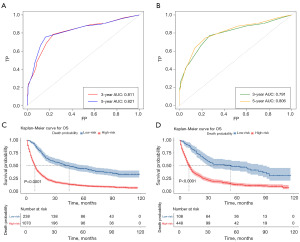
Discussion
This was the first time that we used the LASSO Cox regression method for variable selection and shrinkage in Cox proportional hazards models. The simulations showed that the LASSO selection was more accurate than the stepwise selection, as it compressed coefficients and produced some coefficients that were precisely zero. By experimentally expanding the LASSO penalty, it generated an understandable final model and decreased the false discovery rate in the univariate and multivariate Cox regression (13,25). After using the LASSO regression to examine the relationship between OS and the 18 other variables, eight variables with non-zero coefficients were chosen for the multivariate analysis. The nomogram included five parameters linked with OS that were found to be independent predictive factors of pulmonary LCNEC in the multivariate Cox analysis. Finally, we created 3- and 5-year OS nomograms using these five independent prognostic markers for OS.
In the training cohort, the nomogram model demonstrated strong discriminative accuracy (C-index =0.716), which was verified in the validation cohort (C-index =0.708). However, the C-indexes for the 7th edition AJCC TNM staging system in the training set and validation set were 0.685 and 0.705, respectively. These results demonstrated the excellent ability of the proposed model to predict pulmonary LCNEC. Further, the calibration plots showed that this nomogram performed exceptionally well in calculating the prognosis of pulmonary LCNEC patients, with good agreement for the prediction of OS at 3 and 5 years in both cohorts. The DCA results showed that the nomogram also performed well compared to the 7th edition of the AJCC TNM staging system, and thus the nomogram might improve the precision of customized clinical decisions. Our findings clarified the features of pulmonary LCNEC and were consistent with another SEER database-based LCNEC study (19).
Our study demonstrated that an absence of bone and liver metastases was associated with longer OS in patients with pulmonary LCNEC. Zhao et al. conducted a study to examine the survival outcomes, prevalence of brain metastasis, and recurrence patterns of LCNEC patients, and found that disease relapse occurred in 73% of patients who had received curative treatment, most frequently in the local lymph nodes, brain, bones, and liver. The incidence of brain metastasis in LCNEC was higher than that observed in other types of NSCLCs (26,27). Fisch et al. retrospectively examined 191 patients with metastatic pulmonary LCNEC in the data from German, and the type of systemic treatment was the single factor most significantly associated with patients’ outcomes. Fisch et al. reported that patients who received immune checkpoint inhibitors (ICIs) (n=13) had a median OS of 26.4 months, while those who received first-line platinum doublets (n=129) had a median OS of 9.0 months, and those who received non-platinum chemotherapies had a median OS of 4.0 months (n=17, P=0.01) (28). We observed that the brain was the most common distant metastasis organ (17.1% of the patients had distant brain metastasis). Stereotactic radiosurgery may be an option for LCNEC patients who are receiving upfront treatment for localized brain metastasis, according to several studies. A multivariate Cox regression analysis revealed that bone metastasis and liver metastasis were independent prognostic factors in a training group, and the 3-year survival rates in the bone metastasis and liver metastasis groups were 2.86% (95% CI: 1.30–6.29%) and 0.474% (95% CI: 0.067–3.35%), respectively (29,30). Thus, there is an urgent need for additional safe and effective therapy choices.
Radiotherapy had no discernible effect on patient prognosis; however, our investigation verified the prognostic usefulness of surgery and chemotherapy for OS in patients with pulmonary LCNEC. Surgery has been linked to an increase in OS. A previous study found that patients with pulmonary LCNEC had a median OS of 66.0 months and a 5-year OS rate of 52.5% (31). In our analysis, the 3- and 5-year OS rates for surgical patients were 50.35% (95% CI: 45.97–55.14%) and 39.57% (95% CI: 35.29–44.36%), respectively. All patients with early stage (I–III) pulmonary LCNEC should be treated with surgery as a first option (32). Further, retrospective studies indicate that surgical resection of pulmonary LCNEC at pathologic stages I and II, most frequently lobectomy and pneumonectomy, was linked to a 5-year OS of 49.2% (33,34). However, a different study reported that sublobar lung resection was linked to a lower survival rate for individuals with early (stage I) pulmonary LCNEC (35).
In addition, certain studies have found evidence of the value of adjuvant treatment for pulmonary LCNEC, particularly at pathologic stage II or higher (36,37). Upfront surgery is suggested in the early stages (I to IIB), and adjuvant chemotherapy should also be administered in accordance with the small-cell lung cancer (SCLC) protocol, according to current treatment recommendations. Results of pertinent prospective clinical studies evaluating adjuvant therapy for pulmonary LCNEC have also been published (38,39). However, adjuvant therapy following surgery has been shown to be essential to prevent tumor recurrence, and in locally advanced stages (III–IV), a multimodality approach was suggested (40-42). According to research by Jiang et al., lobectomy might considerably increase OS and lung cancer-specific survival for patients with stage I to II pulmonary LCNEC, while radiation could dramatically increase the survival time of patients with stage III LCNEC.
Conversely, radiation might shorten the period that patients with LCNEC survive surgery (43). Zhang et al. performed a multicenter retrospective study and showed that the median disease-free survival time of the SCLC-regimen group was not significantly longer than that of the NSCLC-regimen group in the adjuvant cohort. The median progression-free survival (PFS) time of the SCLC-regimen group in the first-line cohort was 11.5 months, which was longer than the PFS time of the NSCLC-regimen group. Thus, the SCLC treatment was found to be a more effective choice as either a first-line treatment or adjuvant chemotherapy for LCNEC patients (44). According to Cao et al., radiotherapy may increase the lifespan of patients with pulmonary LCNEC, particularly those who did not have surgery or chemotherapy (45). Conversely, another study found that postoperative chemotherapy, radiation, or combination therapy had no effect on the survival of patients with pulmonary LCNEC who had had surgery (46). Interestingly, our results showed that radiotherapy and chemotherapy had a significant detrimental effect on OS, as the patients receiving radiotherapy and chemotherapy had shorter OS than those receiving neither treatment. Possible explanations for this contentious outcome include the fact that these two trials did not provide many details on the treatment regimens.
Numerous studies have demonstrated that platinum-based and SCLC-based chemotherapy results in longer OS for patients with pulmonary LCNEC (47,48). Recent research suggests that pulmonary LCNEC patients were significantly less chemo-responsive to this regimen; however, prospective studies using platinum-based etoposide in patients with extensive-stage SCLC have reported response rates of around 50% and up to 80% (49,50). However, the clinical course and treatment of patients with advanced LCNEC are limited (51). These studies revealed that for advanced-stage LCNEC patients who received a combination of cisplatin-etoposide or cisplatin-irinotecan, the median OS was 7.7 (95% CI: 6–9.6) vs. 15.1 (95% CI: 11.2–19) months (52,53). Additionally, four to six cycles of chemotherapy that included etoposide in addition to cisplatin or carboplatin were typically shown to improve the prognosis of the advanced-stage patients (48,54). Certain studies also noted that patients with unresectable pulmonary LCNEC (including stage I and II) who received treatment with a variety of modalities had higher OS results than those who received chemotherapy alone (10,55). Thus, we must create novel treatments for patients with pulmonary LCNEC.
With the development of new techniques, tyrosine kinase inhibitors (TKIs) and other targeted medications may improve the quality of life and survival of patients with advanced or relapsed high-grade neuroendocrine carcinoma. However, only a few studies have described cases of LCNEC with epidermal growth factor receptor (EGFR) mutations or anaplastic lymphoma kinase (ALK) translocations (56-59). Currently, the clinical value of TKI in pulmonary LCNEC is relatively restricted; thus, it is crucial to identify other treatment targets (60,61). An inescapable question in the current treatment is whether LCNEC could be vulnerable to treatment with ICIs. According to certain published case reports and research, individuals with pulmonary LCNEC appear to live longer after receiving ICIs (62-65). Naganuma et al. performed a retrospective study and showed that the overall response rate, median PFS, and median OS of previously treated advanced LCNEC patients who then received anti-programed death-blockade monotherapy were 9.1%, 2.7 months, and 4.6 months, respectively, and observed adverse events in 9 patients (82%) (66). Levra et al. showed that pulmonary LCNEC patients with stages IIIB to IV could gain a survival benefit from nivolumab or pembrolizumab after platinum-based first-line therapy (63). However, there were no data on immunotherapy in the SEER database, and as there is no prospective evidence, we cannot yet determine if immunotherapy is effective in treating pulmonary LCNEC. Future clinical trials should include numerous sizable multicenter studies to confirm the findings. It may be that this divergence in findings was caused by the limited sample sizes and the potential for follow-up bias.
Conclusions
In conclusion, our results demonstrated that this newly designed nomogram has remarkable power in predicting the likelihood of survival of patients with LCNEC compared to the 7th edition of the AJCC TNM staging system. The key feature of the study is that we were able to decrease the selection and surveillance biases related to specific institutions by employing one of the largest population-based cohorts of patients with pulmonary LCNEC across 17 United States registries. However, some of the significant limitations of this study must be acknowledged. First, due to the small sample size and potential for follow-up bias, every retrospective study inevitably contains bias. Second, specific details about chemotherapy, surgery, radiotherapy, or other therapies were not provided, nor were the reasons as to why patients preferred some therapy methods over others. Finally, the SEER database lacked data on tumor recurrence trends and post-recurrence treatment histories. Thus, real-world data needs to be gathered to externally verify our results in the future.
Acknowledgments
Funding: This work was supported by
Footnote
Reporting Checklist: The authors have completed the TRIPOD reporting checklist. Available at https://tcr.amegroups.com/article/view/10.21037/tcr-23-1061/rc
Peer Review File: Available at https://tcr.amegroups.com/article/view/10.21037/tcr-23-1061/prf
Conflicts of Interest: All authors have completed the ICMJE uniform disclosure form (available at https://tcr.amegroups.com/article/view/10.21037/tcr-23-1061/coif). The authors have no conflicts of interest to declare.
Ethical Statement: The authors are accountable for all aspects of the work in ensuring that questions related to the accuracy or integrity of any part of the work are appropriately investigated and resolved. This study was conducted in accordance with the Declaration of Helsinki (as revised in 2013).
Open Access Statement: This is an Open Access article distributed in accordance with the Creative Commons Attribution-NonCommercial-NoDerivs 4.0 International License (CC BY-NC-ND 4.0), which permits the non-commercial replication and distribution of the article with the strict proviso that no changes or edits are made and the original work is properly cited (including links to both the formal publication through the relevant DOI and the license). See: https://creativecommons.org/licenses/by-nc-nd/4.0/.
References
- Rindi G, Klimstra DS, Abedi-Ardekani B, et al. A common classification framework for neuroendocrine neoplasms: an International Agency for Research on Cancer (IARC) and World Health Organization (WHO) expert consensus proposal. Mod Pathol 2018;31:1770-86. [Crossref] [PubMed]
- Korse CM, Taal BG, van Velthuysen ML, et al. Incidence and survival of neuroendocrine tumours in the Netherlands according to histological grade: experience of two decades of cancer registry. Eur J Cancer 2013;49:1975-83. [Crossref] [PubMed]
- Strosberg JR, Coppola D, Klimstra DS, et al. The NANETS consensus guidelines for the diagnosis and management of poorly differentiated (high-grade) extrapulmonary neuroendocrine carcinomas. Pancreas 2010;39:799-800. [Crossref] [PubMed]
- Xia K, Zhong W, Chen J, et al. Clinical Characteristics, Treatment Strategy, and Outcomes of Primary Large Cell Neuroendocrine Carcinoma of the Bladder: A Case Report and Systematic Review of the Literature. Front Oncol 2020;10:1291. [Crossref] [PubMed]
- Tu X, Chang T, Nie L, et al. Large Cell Neuroendocrine Carcinoma of the Prostate: A Systematic Review and Pooled Analysis. Urol Int 2019;103:383-90. [Crossref] [PubMed]
- Yang X, Chen J, Dong R. Pathological features, clinical presentations and prognostic factors of ovarian large cell neuroendocrine carcinoma: a case report and review of published literature. J Ovarian Res 2019;12:69. [Crossref] [PubMed]
- Lázaro S, Pérez-Crespo M, Lorz C, et al. Differential development of large-cell neuroendocrine or small-cell lung carcinoma upon inactivation of 4 tumor suppressor genes. Proc Natl Acad Sci U S A 2019;116:22300-6. [Crossref] [PubMed]
- Schnabel PA, Junker K. Pulmonary neuroendocrine tumors in the new WHO 2015 classification: Start of breaking new grounds? Pathologe 2015;36:283-92. [Crossref] [PubMed]
- Travis WD, Brambilla E, Burke AP, et al. Introduction to The 2015 World Health Organization Classification of Tumors of the Lung, Pleura, Thymus, and Heart. J Thorac Oncol 2015;10:1240-2. [Crossref] [PubMed]
- Gu J, Gong D, Wang Y, et al. The demographic and treatment options for patients with large cell neuroendocrine carcinoma of the lung. Cancer Med 2019;8:2979-93. [Crossref] [PubMed]
- Goldstraw P, Crowley J, Chansky K, et al. The IASLC Lung Cancer Staging Project: proposals for the revision of the TNM stage groupings in the forthcoming (seventh) edition of the TNM Classification of malignant tumours. J Thorac Oncol 2007;2:706-14. [Crossref] [PubMed]
- Liao F, Guo X, Lu X, et al. A validated survival nomogram for early-onset diffuse gastric cancer. Aging (Albany NY) 2020;12:13160-71. [Crossref] [PubMed]
- Tibshirani R. The lasso method for variable selection in the Cox model. Stat Med 1997;16:385-95. [Crossref] [PubMed]
- Van Belle V, Van Calster B. Visualizing Risk Prediction Models. PLoS One 2015;10:e0132614. [Crossref] [PubMed]
- Xiong Y, Yuan L, Xiong J, et al. An outcome model for human bladder cancer: A comprehensive study based on weighted gene co-expression network analysis. J Cell Mol Med 2020;24:2342-55. [Crossref] [PubMed]
- Liu GM, Xie WX, Zhang CY, et al. Identification of a four-gene metabolic signature predicting overall survival for hepatocellular carcinoma. J Cell Physiol 2020;235:1624-36. [Crossref] [PubMed]
- Sheng X, Lu X, Wu J, et al. A Nomogram Predicting the Prognosis of Renal Cell Carcinoma Patients with Lung Metastases. Biomed Res Int 2021;2021:6627562. [Crossref] [PubMed]
- He Y, Liu H, Wang S, et al. Prognostic nomogram predicts overall survival in pulmonary large cell neuroendocrine carcinoma. PLoS One 2019;14:e0223275. [Crossref] [PubMed]
- Ma H, Xu Z, Zhou R, et al. A Clinical Nomogram for Predicting Cancer-Specific Survival in Pulmonary Large-Cell Neuroendocrine Carcinoma Patients: A Population-Based Study. Int J Gen Med 2021;14:7299-310. [Crossref] [PubMed]
- Iasonos A, Schrag D, Raj GV, et al. How to build and interpret a nomogram for cancer prognosis. J Clin Oncol 2008;26:1364-70. [Crossref] [PubMed]
- Balachandran VP, Gonen M, Smith JJ, et al. Nomograms in oncology: more than meets the eye. Lancet Oncol 2015;16:e173-80. [Crossref] [PubMed]
- Cronin KA, Ries LA, Edwards BK. The Surveillance, Epidemiology, and End Results (SEER) Program of the National Cancer Institute. Cancer 2014;120:3755-7. [Crossref] [PubMed]
- Kattan MW, Gerds TA. A Framework for the Evaluation of Statistical Prediction Models. Chest 2020;158:S29-38. [Crossref] [PubMed]
- Vickers AJ, Elkin EB. Decision curve analysis: a novel method for evaluating prediction models. Med Decis Making 2006;26:565-74. [Crossref] [PubMed]
- Ternès N, Rotolo F, Michiels S. Empirical extensions of the lasso penalty to reduce the false discovery rate in high-dimensional Cox regression models. Stat Med 2016;35:2561-73. [Crossref] [PubMed]
- Zhao Y, Castonguay M, Wilke D, et al. Treatment outcomes and incidence of brain metastases in pulmonary large cell neuroendocrine carcinoma. Curr Probl Cancer 2019;43:54-65. [Crossref] [PubMed]
- Naidoo J, Santos-Zabala ML, Iyriboz T, et al. Large Cell Neuroendocrine Carcinoma of the Lung: Clinico-Pathologic Features, Treatment, and Outcomes. Clin Lung Cancer 2016;17:e121-9. [Crossref] [PubMed]
- Fisch D, Bozorgmehr F, Kazdal D, et al. Comprehensive Dissection of Treatment Patterns and Outcome for Patients With Metastatic Large-Cell Neuroendocrine Lung Carcinoma. Front Oncol 2021;11:673901. [Crossref] [PubMed]
- Wegner RE, Hasan S, Williamson RW, et al. Management of brain metastases from large cell neuroendocrine carcinoma of the lung: improved outcomes with radiosurgery. Acta Oncol 2019;58:499-504. [Crossref] [PubMed]
- Kawabe T, Yamamoto M, Sato Y, et al. Gamma Knife radiosurgery for brain metastases from pulmonary large cell neuroendocrine carcinoma: a Japanese multi-institutional cooperative study (JLGK1401). J Neurosurg 2016;125:11-7. [Crossref] [PubMed]
- Zou L, Guo T, Ye L, et al. Outcomes for Surgery in Stage IA Large Cell Lung Neuroendocrine Compared With Other Types of Non-Small Cell Lung Cancer: A Propensity Score Matching Study Based on the Surveillance, Epidemiology, and End Results (SEER) Database. Front Oncol 2020;10:572462. [Crossref] [PubMed]
- Corbett V, Arnold S, Anthony L, et al. Management of Large Cell Neuroendocrine Carcinoma. Front Oncol 2021;11:653162. [Crossref] [PubMed]
- Zacharias J, Nicholson AG, Ladas GP, et al. Large cell neuroendocrine carcinoma and large cell carcinomas with neuroendocrine morphology of the lung: prognosis after complete resection and systematic nodal dissection. Ann Thorac Surg 2003;75:348-52. [Crossref] [PubMed]
- Fournel L, Falcoz PE, Alifano M, et al. Surgical management of pulmonary large cell neuroendocrine carcinomas: a 10-year experience. Eur J Cardiothorac Surg 2013;43:111-4. [Crossref] [PubMed]
- Lutfi W, Schuchert MJ, Dhupar R, et al. Sublobar resection is associated with decreased survival for patients with early stage large-cell neuroendocrine carcinoma of the lung. Interact Cardiovasc Thorac Surg 2019;29:517-24. [Crossref] [PubMed]
- Filosso PL, Guerrera F, Evangelista A, et al. Adjuvant chemotherapy for large-cell neuroendocrine lung carcinoma: results from the European Society for Thoracic Surgeons Lung Neuroendocrine Tumours Retrospective Database. Eur J Cardiothorac Surg 2017;52:339-45. [Crossref] [PubMed]
- Kim KW, Kim HK, Kim J, et al. Outcomes of Curative-Intent Surgery and Adjuvant Treatment for Pulmonary Large Cell Neuroendocrine Carcinoma. World J Surg 2017;41:1820-7. [Crossref] [PubMed]
- Saji H, Tsuboi M, Matsubayashi J, et al. Clinical response of large cell neuroendocrine carcinoma of the lung to perioperative adjuvant chemotherapy. Anticancer Drugs 2010;21:89-93. [Crossref] [PubMed]
- Sarkaria IS, Iyoda A, Roh MS, et al. Neoadjuvant and adjuvant chemotherapy in resected pulmonary large cell neuroendocrine carcinomas: a single institution experience. Ann Thorac Surg 2011;92:1180-6; discussion 1186-7. [Crossref] [PubMed]
- Iyoda A, Hiroshima K, Moriya Y, et al. Prospective study of adjuvant chemotherapy for pulmonary large cell neuroendocrine carcinoma. Ann Thorac Surg 2006;82:1802-7. [Crossref] [PubMed]
- Kenmotsu H, Niho S, Tsuboi M, et al. Randomized Phase III Study of Irinotecan Plus Cisplatin Versus Etoposide Plus Cisplatin for Completely Resected High-Grade Neuroendocrine Carcinoma of the Lung: JCOG1205/1206. J Clin Oncol 2020;38:4292-301. [Crossref] [PubMed]
- Eba J, Kenmotsu H, Tsuboi M, et al. A Phase III trial comparing irinotecan and cisplatin with etoposide and cisplatin in adjuvant chemotherapy for completely resected pulmonary high-grade neuroendocrine carcinoma (JCOG1205/1206). Jpn J Clin Oncol 2014;44:379-82. [Crossref] [PubMed]
- Jiang Y, Lei C, Zhang X, et al. Double-edged role of radiotherapy in patients with pulmonary large-cell neuroendocrine carcinoma. J Cancer 2019;10:6422-30. [Crossref] [PubMed]
- Zhang JT, Li Y, Yan LX, et al. Disparity in clinical outcomes between pure and combined pulmonary large-cell neuroendocrine carcinoma: A multi-center retrospective study. Lung Cancer 2020;139:118-23. [Crossref] [PubMed]
- Cao L, Wu HF, Zhao L, et al. The role of radiotherapy in pulmonary large cell neuroendocrine carcinoma: propensity score matching analysis. J Radiat Res 2020;61:594-601. [Crossref] [PubMed]
- Dresler CM, Ritter JH, Patterson GA, et al. Clinical-pathologic analysis of 40 patients with large cell neuroendocrine carcinoma of the lung. Ann Thorac Surg 1997;63:180-5. [Crossref] [PubMed]
- Derks JL, van Suylen RJ, Thunnissen E, et al. Chemotherapy for pulmonary large cell neuroendocrine carcinomas: does the regimen matter? Eur Respir J 2017;49:1601838. [Crossref] [PubMed]
- Andrini E, Marchese PV, De Biase D, et al. Large Cell Neuroendocrine Carcinoma of the Lung: Current Understanding and Challenges. J Clin Med 2022;11:1461. [Crossref] [PubMed]
- Lowczak A, Kolasinska-Cwikla A, Osowiecka K, et al. Outcomes of Patients with Pulmonary Large Cell Neuroendocrine Carcinoma in I-IV Stage. Medicina (Kaunas) 2021;57:118. [Crossref] [PubMed]
- Noda K, Nishiwaki Y, Kawahara M, et al. Irinotecan plus cisplatin compared with etoposide plus cisplatin for extensive small-cell lung cancer. N Engl J Med 2002;346:85-91. [Crossref] [PubMed]
- Sun JM, Ahn MJ, Ahn JS, et al. Chemotherapy for pulmonary large cell neuroendocrine carcinoma: similar to that for small cell lung cancer or non-small cell lung cancer? Lung Cancer 2012;77:365-70. [Crossref] [PubMed]
- Le Treut J, Sault MC, Lena H, et al. Multicentre phase II study of cisplatin-etoposide chemotherapy for advanced large-cell neuroendocrine lung carcinoma: the GFPC 0302 study. Ann Oncol 2013;24:1548-52. [Crossref] [PubMed]
- Yamazaki S, Sekine I, Matsuno Y, et al. Clinical responses of large cell neuroendocrine carcinoma of the lung to cisplatin-based chemotherapy. Lung Cancer 2005;49:217-23. [Crossref] [PubMed]
- Iyoda A, Makino T, Koezuka S, et al. Treatment options for patients with large cell neuroendocrine carcinoma of the lung. Gen Thorac Cardiovasc Surg 2014;62:351-6. [Crossref] [PubMed]
- Limonnik V, Abel S, Finley GG, et al. Factors associated with treatment receipt and overall survival for patients with locally advanced large cell neuroendocrine carcinoma of the lung: A National Cancer Database analysis. Lung Cancer 2020;150:107-13. [Crossref] [PubMed]
- Yoshida Y, Ota S, Murakawa T, et al. Combined large cell neuroendocrine carcinoma and adenocarcinoma with epidermal growth factor receptor mutation in a female patient who never smoked. Ann Thorac Cardiovasc Surg 2014;20:582-4. [Crossref] [PubMed]
- Sakai Y, Yamasaki T, Kusakabe Y, et al. Large-cell neuroendocrine carcinoma of lung with epidermal growth factor receptor (EGFR) gene mutation and co-expression of adenocarcinoma markers: a case report and review of the literature. Multidiscip Respir Med 2013;8:47. [Crossref] [PubMed]
- George J, Walter V, Peifer M, et al. Integrative genomic profiling of large-cell neuroendocrine carcinomas reveals distinct subtypes of high-grade neuroendocrine lung tumors. Nat Commun 2018;9:1048. [Crossref] [PubMed]
- Leblanc A, Owen S, Fiset PO, et al. Metastatic Large-Cell Neuroendocrine Lung Carcinoma With ALK Fusion Oncogene With Partial Response to Alectinib. JCO Precis Oncol 2021;5:802-7. [Crossref] [PubMed]
- Odate S, Nakamura K, Onishi H, et al. TrkB/BDNF signaling pathway is a potential therapeutic target for pulmonary large cell neuroendocrine carcinoma. Lung Cancer 2013;79:205-14. [Crossref] [PubMed]
- Iyoda A, Travis WD, Sarkaria IS, et al. Expression profiling and identification of potential molecular targets for therapy in pulmonary large-cell neuroendocrine carcinoma. Exp Ther Med 2011;2:1041-5. [Crossref] [PubMed]
- Daido W, Yamasaki M, Saito N, et al. Effectiveness of Nivolumab in Large-Cell Neuroendocrine Carcinoma of the Lung - A Report of Two Cases. Gan To Kagaku Ryoho 2017;44:59-62. [PubMed]
- Levra MG, Mazieres J, Valette CA, et al. P1.07-012 Efficacy of Immune Checkpoint Inhibitors in Large Cell Neuroendocrine Lung Cancer: Results from a French Retrospective Cohort: Topic: Drug Treatment Alone and in Combination with Radiotherapy. J Thorac Oncol 2017;12:S702-3. [Crossref]
- Dudnik E, Kareff S, Moskovitz M, et al. Real-world survival outcomes with immune checkpoint inhibitors in large-cell neuroendocrine tumors of lung. J Immunother Cancer 2021;9:e001999. [Crossref] [PubMed]
- Komiya T, Ravindra N, Powell E. Role of Immunotherapy in Stage IV Large Cell Neuroendocrine Carcinoma of the Lung. Asian Pac J Cancer Prev 2021;22:365-70. [Crossref] [PubMed]
- Naganuma K, Imai H, Yamaguchi O, et al. Efficacy and Safety of Anti-Programed Death-1 Blockade in Previously Treated Large-Cell Neuroendocrine Carcinoma. Chemotherapy 2021;66:65-71. [Crossref] [PubMed]