The value of intratumoral and peritumoral radiomics features in differentiating early-stage lung invasive adenocarcinoma (≤3 cm) subtypes
Highlight box
Key findings
• The intratumoral and peritumoral radiomics features could improve the predictive ability to differentiate early-stage lung invasive adenocarcinoma (≤3 cm) subtypes.
What is known and what is new?
• Radiomics is an emerging and reliable research approach that is widely used to non-invasively predict the classification and extent of cancer. However, few radiomics studies have investigated or discussed the subtypes of early-stage lung invasive adenocarcinoma. This manuscript demonstrates the value of peritumoral radiomics features in differentiating early-stage lung invasive adenocarcinoma.
What is the implication, and what should change now?
• The diagnostic efficacy of the intra-peritumoral radiomics combined clinical model was further improved, with particular reference significance for the preoperative staging and treatment decision-making.
Introduction
Pathologically, invasive lung adenocarcinoma comprises the following five types: lepidic-predominant adenocarcinoma (LPA), acinar-predominant adenocarcinoma (APA), papillary-predominant adenocarcinoma (PPA), micropapillary-predominant adenocarcinoma (MPA) and solid-predominant adenocarcinoma (SPA) (1). Although lobectomy is the traditional clinical treatment for lung adenocarcinoma, wedge or segmental lung resection is probably more useful for the lepidic-dominanted subtype (2-4). Patients who choose sublobar resection have less surgical trauma and loss of lung function than with lobectomy. They have a substantially better quality of life after surgery. Moreover, the 5-year recurrence-free survival (RFS) and disease-free survival (DFS) rates for lepidic lung adenocarcinoma are significantly higher than those for the other subtypes (5,6). However, early-stage invasive lung adenocarcinoma always appears as a ground glass nodule on computed tomography (CT) with a low operative component. It is challenging to determine the lung adenocarcinoma subtype of patients by traditional methods such as imaging, blood markers, and needle biopsy before surgery (7-9). Therefore, clinical decision-making desperately needs more effective and non-invasive methods to bail out the phenomenon of overtreatment.
Radiomics is an emerging and reliable research approach that is widely used to non-invasively predict the classification and extent of cancer, resolve genetic phenotypes, and predict disease onset and regression (10-13). It is a data-driven method that screens extensive features from medical images for reproducibility to excavate the research object of potential information.
The changes in lymphatic vessels, blood vessels, inflammation and immune cell infiltration in the microenvironment around tumors are conditions that support the occurrence and progression of tumors (14-16). Many studies of the peritumoral region have yielded promising results in recent years. Zhang et al. revealed the added value of peritumoral radiomics in terms of tumor heterogeneity (17). Chen et al. extracted the radiomics features of the 9 mm area surrounding the lesions to improve the prediction of lymphovascular invasion and overall survival in non-small cell lung cancer (18). Yamazaki et al.’s studies have shown the combined use of intra- and peritumoral radiomic features improved the performance for epidermal growth factor receptor (EGFR) mutation prediction in primary lung cancer (19). The above studies provided methodological feasibility. Few studies on radiomics have investigated or discussed the subtypes of early-stage lung invasive adenocarcinoma.
Therefore, this study aimed to investigate the potentially valuable information in the intratumoral and peritumoral regions of early invasive lung adenocarcinoma through radiomics methods and construct the effective model to distinguish between the LPA and the non-lepidic-predominant subtype (non-LPA) before the clinical operation. We present this article in accordance with the TRIPOD reporting checklist (available at https://tcr.amegroups.com/article/view/10.21037/tcr-23-1324/rc)
Methods
Study population
This study retrospectively analysed patients with pathologically confirmed lung adenocarcinoma in Shaoxing People’s Hospital, Shaoxing Hospital, Zhejiang University School of Medicine from January 2016 to September 2021. Clinical and imaging data were collected from 856 patients with 937 lesions after evaluation. Inclusion criteria: (I) pulmonary nodules underwent complete surgical resection with pathologically confirmed early invasive lung adenocarcinoma; (II) the interval between CT examination and surgery of less than 30 days; (III) patients with complete clinical data. Exclusion criteria: (I) patients with a history of extra-pulmonary malignancy (n=9); (II) poor CT image quality due to severe respiratory artefacts or inflammation affecting the assessment (n=46); (III) patients with previous neoadjuvant treatment for malignancy (n=10); (IV) lesions larger than 3 cm in length on imaging (n=29). The blood biomarkers criteria: the normal upper limit for carcinoembryonic antigen (CEA), cancer antigen 125 (CA125), CA50, CA242, and neuron-specific enolase (NSE) was 5, 35 ng/mL, 25, 35 IU/mL, and 15.7 ng/mL, respectively. All patients underwent blood testing at our institution. The study was conducted in accordance with the Declaration of Helsinki (as revised in 2013). The study was approved by the Ethics Committee of the Shaoxing People’s Hospital, Shaoxing Hospital, Zhejiang University School of Medicine (No. 2021-K-Y-363-01), and the patient consent requirement was waived because of its retrospective nature.
CT image acquisition
All patients were scanned with a Brilliance 64 scanner (Philips Healthcare, Eindhoven, The Netherlands) and a Brightspeed 16 scanner (General Electric Healthcare, Shenzhen, China) for 1–2.5 mm thickness chest CT scans. The procedure was as follows: the patient lay flat on the scanning table, his or her hands were raised to the head to avoid scan occlusion, the breath was held according to the instrument prompts, the range was from above the lung apex to below the level of the diaphragm, and the acquisition parameters included voltage (120 KV), current (160 mA), scan layer thickness (1.0-2.5 mm), matrix (512×512), spacing (0.8–1.5), frame rotation speed (0.5 s/r), window width 1,500 HU, window position −600 HU.
Histopathological assessment
Two experienced lung cancer pathologists performed a comprehensive histological assessment of all surgically resected lung specimens according to the 2011 version of the International Association for the Study of Lung Cancer (IASLC), the American Thoracic Society (ATS), and the European Respiratory Society (ERS) classification criteria (1). All patients included in the study were divided into two groups: the LPA group (LPA) and the non-LPA group (APA, PPA, MPA, and SPA). These two groups were used as a clinical endpoint for the classification problem.
Segmentation and radiomics feature extraction
All case scan CT images recorded for this study were loaded into The Darwin research platform (https://arxiv.org/abs/2009.00908) and performed image preprocessing (standardized image resampling). The gross tumor volume (GTV) was defined as the tumor that was identified within the visible tumor border. The peritumoral volume (PTV) was defined as PTV0~+3 (3 mm external to the tumor border). We used a compiled artificial intelligence (AI) algorithm which based on Fast Region-based Convolutional Network method (Faster R-CNN; https://arxiv.org/abs/1506.01497) to help two radiologists who skilled in image processing software delineate the target nodules semi-automatically. The radiologists were blinded to all clinical information. Next, they adjusted the regions of interest (ROIs) of the target tumor and avoided blood vessels, bronchi, and adjacent structures along the nodal border that were recognisable to the naked eye. Finally, a senior physician reviewed the adjustments. Meanwhile, they also evaluated the characteristics of nodules, like pure, part-solid or solid. Some previous findings showed that a resection margin >15 mm did not decrease the risk of recurrence or a resection margin ≥20 mm was the safe margin (20,21). Liu et al. found that a 6 mm area surrounding the tumor could provide biological information related to the heterogeneity of lung adenocarcinoma (22). Based on the above research, we used an extension algorithm with 3 and 5 mm spacing to extend the segmented tumor outer contour outward to 20 mm, respectively (3, 6, 9, 12, 15, 18 mm and 5, 10, 15, 20 mm), while manually calibrating the tumor margin. The peritumoral region included pulmonary vessels and bronchi. Figure 1 shows the flow of our study.
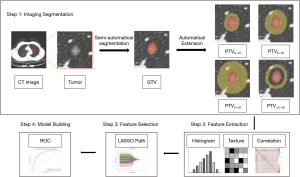
This study extracted first-order features, shape features, and texture features, which were image histology features, by using the above software and processes the CT images using filters: wavelet transform and Laplace operator to obtain the transformed features. Definitions and formulas of these radiomic features are available at https://pyradiomics.readthedocs.io/en/latest/features.html.
Feature selection and radiomics model construction
The cases in this study were allocated as training or test cohorts in a ratio of 7:3. The feature selection and model construction for each target region were handled consistently. First, the extracted radiomics features were normalised using a standardisation method to eliminate the magnitudes. The Pearson Correlation Coefficient (PCC) method (excluding features with absolute correlation coefficient ≥0.9) and the F-Value method were chosen to reduce the dimensionality. The 10-fold cross-validation method prevented overfitting and improved the model’s generalization ability. The redundant radiomics features were removed using the Least Absolute Shrinkage and Selection Operator (LASSO) method. The radiomics score (rad-score) was calculated based on candidate features. Next, this study used the rad-scores to establish the logistic regression (LR) independent prediction model respectively. Finally, optimal radiomics features selected from intratumoral and peritumoral features to construct GTV + PTV model. The best features of GTV + PTV model were combined with the clinical features to build clinical-intratumoral-peritumoral conjoint model (Clinical + GTV + PTV model).
Construction of the clinical model and nomogram
This study used univariate analysis and multivariate LR analysis to screen the independent clinical predictors of LPA tumor and constructed the clinical model. The nomogram was constructed based on independent clinical predictors and the rad-score of the best radiomics model.
Statistical analysis
Clinical data were analysed statistically using SPSS software (version 27.0 IBM SPSS Statistics, Armonk, NY, USA). The methods were as follows: the Student t-test (normally distributed values) and Mann-Whitney U test (non-normally distributed values) assessed the continuous measurement data. The Chi-squared test or Fisher exact test was used for comparison between categorical variables.
The radiomics data analysis was based on R software (version: 3.6.3; http://www.r-project.org). Area under the receiver operating characteristic (ROC) curve (AUC), accuracy (ACC), sensitivity (SEN), and specificity (SPE) were calculated by plotting the ROC curves. Significant differences between the AUCs were estimated using the Delong test, which could determine the statistical significance between the models. P<0.05 was considered statistically significant. Data in the parentheses referred to the 95% confidence interval of AUC.
Results
Characteristics of the study cohorts
A total of 937 lung adenocarcinomas were included in this study, with 603 (64.4%) LPAs and 334 (35.6%) non-LPAs (259 APAs, 51 PPAs, 1 MPA, and 23 SPAs). Significant differences were found in patient age, smoking history, CEA, lesion location, length, width and clinic behavior (P<0.05). Table 1 shows the details. After multivariate analysis, we found patient age, smoking history, CEA, lesion location (right or left upper lobe, right middle lobe, right lower lobe), length, width and clinic behavior (pure, solid) as the independent predictors of differentiating the LPA or Non-LPA lung cancer (P<0.05), and the feature importance could be found in Figure 2A.
Table 1
Characteristics | Lepidic (n=603) | Non-lepidic (n=334) | P values |
---|---|---|---|
Age (years), median [range] | 61 [18–83] | 63 [28–83] | <0.01 |
Sex, n [%] | 0.337 | ||
Female | 424 [70] | 224 [67] | |
Male | 179 [30] | 110 [33] | |
BMI (kg/m2), median [range] | 22.7 [14.9–35.3] | 22.9 [15.2–38.1] | 0.194 |
Length (mm), median [range] | 11 [3–30] | 15 [5–30] | <0.01 |
Width (mm), median [range] | 9 [3–30] | 12 [3–26] | <0.01 |
Smoking, n [%] | 0.006 | ||
Yes | 72 [12] | 62 [19] | |
No | 531 [88] | 272 [81] | |
Drinking, n [%] | 0.036 | ||
Yes | 71 [12] | 56 [17] | |
No | 532 [88] | 278 [83] | |
Hypertension, n [%] | 0.002 | ||
Yes | 184 [31] | 135 [40] | |
No | 419 [69] | 199 [60] | |
Diabetes, n [%] | 0.637 | ||
Yes | 53 [9] | 33 [10] | |
No | 550 [91] | 301 [90] | |
Clinic behavior, n [%] | <0.01 | ||
Pure | 294 [49] | 14 [4] | |
Opacity | 278 [46] | 197 [59] | |
Solid | 31 [5] | 123 [37] | |
Lesion location, n [%] | <0.01 | ||
Right upper lobe | 265 [44] | 115 [34] | |
Right middle lobe | 34 [6] | 32 [10] | |
Right lower lobe | 91 [15] | 66 [20] | |
Left upper lobe | 161 [27] | 60 [18] | |
Left lower lobe | 52 [9] | 61 [18] | |
CEA, n [%] | <0.01 | ||
Abnormal | 36 [6.0] | 41 [12.3] | |
Normal | 567 [94.0] | 293 [87.7] | |
CA125, n [%] | >0.99 | ||
Abnormal | 9 [1.5] | 5 [1.5] | |
Normal | 594 [98.5] | 329 [98.5] | |
CA50, n [%] | 0.109 | ||
Abnormal | 3 [0.5] | 6 [1.8] | |
Normal | 600 [99.5] | 328 [98.2] | |
CA242, n [%] | >0.99 | ||
Abnormal | 4 [0.7] | 2 [0.6] | |
Normal | 599 [99.3] | 332 [99.4] | |
NSE, n [%] | 0.754 | ||
Abnormal | 2 [0.3] | 0 [0.0] | |
Normal | 601 [99.7] | 334 [100.0] | |
Surgical decision, n [%] | <0.01 | ||
Wedge resection | 267 [44] | 78 [23] | |
Segmentectomy | 190 [32] | 61 [18] | |
Lobectomy | 146 [24] | 195 [58] |
Statistical difference between each set is resolved using Mann-Whitney U and Chi-squared tests for continuous and categorical variables, respectively. P values is derived from the univariable association analyses between dataset LPA group and dataset non-LPA group. Pure: pure ground-glass nodule; Opacity: mixed ground-glass nodule; Solid: solid nodule. BMI, body mass index; CEA, carcinoembryonic antigen; CA, cancer antigen; NSE, neuron-specific enolase; LPA, lepidic-predominant adenocarcinoma; non-LPA, acinar-predominant adenocarcinoma, papillary-predominant adenocarcinoma, micropapillary-predominant adenocarcinoma and solid-predominant adenocarcinoma.
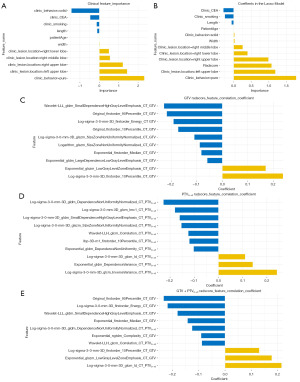
Feature selection and model situation
Each target region was extracted with 1,781 radiomics features, which were collected and summarised as follows: 342 first order feature, 456 GLCM (gray level co-occurrence matrix feature), 266 GLDM (gray level dependence matrices feature), 304 GLRLM (gray level run length matrix feature), 304 GLSZM (gray level size zone matrix feature), 95 NGTDM (neighboring gray tone difference matrix feature), and 14 shape based. We used the above method to select and retain the top 10 features with greater weight in the target region to calculate rad-scores for the GTV, PTV, and GTV + PTV models. We converted 12 best radiomics features to rad-score which combined with the clinical features to construct the Clinical + GTV + PTV0~+3 model. The models’ coefficients could be found in Figure 2B-2E.
Model effectiveness
This study used the above independent clinical predictors to establish the clinical model (Figure 3A). The corresponding AUC values, ACC, SEN, and SPE of each model are shown in Table 2. The results showed that the highest AUC value among the 19 independent models was for the PTV0~+3 radiomics model with an AUC value of 0.849 (95% CI: 0.818, 0.881) for the training set and 0.854 (95% CI: 0.808, 0.9) for the testing set. The GTV model AUC value was similar to the PTV0~+3 peritumoral model. The AUC value of the clinical model was relatively low. The features of GTV + PTV model were selected from features extracted within the intratumoral region and the peritumoral 0–3 mm region, with an AUC value of 0.862 (95% CI: 0.832, 0.892) for the training set and 0.856 (95% CI: 0.809, 0.903) for the testing set. We constructed the Clinical + GTV + PTV0~+3 model with an AUC value of 0.8804 (95% CI: 0.853, 0.908) in the training set and 0.8919 (95% CI: 0.855,0.929) in the testing set. The ROC curves for the above models are shown in Figure 3B-3F.
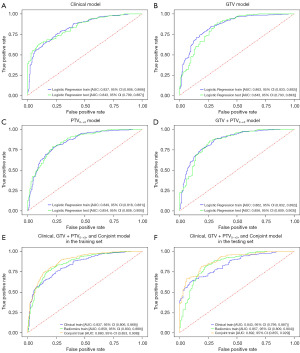
Table 2
Model name | Training set | Testing set | |||||||
---|---|---|---|---|---|---|---|---|---|
AUC | SEN | SPE | ACC | AUC | SEN | SPE | ACC | ||
Clinical | 0.8374 | 0.7536 | 0.7511 | 0.7527 | 0.8432 | 0.7569 | 0.703 | 0.7376 | |
GTV | 0.8628 | 0.846 | 0.7253 | 0.8031 | 0.8427 | 0.8232 | 0.703 | 0.7801 | |
PTV0~+3 | 0.8492 | 0.8492 | 0.782 | 0.7725 | 0.8541 | 0.7901 | 0.7822 | 0.7872 | |
PTV0~+5 | 0.8428 | 0.8318 | 0.7082 | 0.7878 | 0.8498 | 0.7956 | 0.7327 | 0.773 | |
PTV0~+6 | 0.836 | 0.8081 | 0.7253 | 0.7787 | 0.829 | 0.8011 | 0.7129 | 0.7695 | |
PTV0~+9 | 0.8245 | 0.8389 | 0.6738 | 0.7802 | 0.8083 | 0.8177 | 0.7228 | 0.7837 | |
PTV0~+10 | 0.8239 | 0.7488 | 0.7597 | 0.7527 | 0.7908 | 0.7293 | 0.7327 | 0.7305 | |
PTV0~+12 | 0.8104 | 0.7180 | 0.7768 | 0.7389 | 0.7913 | 0.6575 | 0.7525 | 0.6915 | |
PTV0~+15 | 0.7883 | 0.7512 | 0.7093 | 0.7344 | 0.7601 | 0.6961 | 0.7426 | 0.7128 | |
PTV0~+18 | 0.7771 | 0.7749 | 0.6567 | 0.7328 | 0.7445 | 0.7182 | 0.6832 | 0.7057 | |
PTV0~+20 | 0.7063 | 0.628 | 0.7124 | 0.658 | 0.6367 | 0.5249 | 0.6535 | 0.5709 | |
PTV+3~+6 | 0.819 | 0.819 | 0.7701 | 0.721 | 0.7911 | 0.7569 | 0.7228 | 0.7447 | |
PTV+6~+9 | 0.8070 | 0.7512 | 0.7253 | 0.7420 | 0.7804 | 0.7072 | 0.7129 | 0.7092 | |
PTV+9~+12 | 0.7825 | 0.7678 | 0.6953 | 0.7420 | 0.7181 | 0.6906 | 0.6436 | 0.6738 | |
PTV+12~+15 | 0.7317 | 0.6872 | 0.6824 | 0.6855 | 0.6780 | 0.6133 | 0.6436 | 0.6241 | |
PTV+15~+18 | 0.6887 | 0.7559 | 0.5579 | 0.6855 | 0.6036 | 0.6851 | 0.4752 | 0.6099 | |
PTV+5~+10 | 0.8015 | 0.7962 | 0.6695 | 0.7511 | 0.7997 | 0.7459 | 0.6535 | 0.7128 | |
PTV+10~+15 | 0.7121 | 0.7085 | 0.6052 | 0.6718 | 0.6709 | 0.6243 | 0.6139 | 0.6206 | |
PTV+15~+20 | 0.7369 | 0.6682 | 0.6910 | 0.6763 | 0.6311 | 0.5470 | 0.6238 | 0.5745 | |
GTV + PTV0~+3 | 0.8623 | 0.8768 | 0.6953 | 0.8122 | 0.8558 | 0.8564 | 0.7426 | 0.8156 | |
Clinical + GTV + PTV0~+3 | 0.8804 | 0.8081 | 0.7983 | 0.8046 | 0.8919 | 0.8232 | 0.7822 | 0.8085 |
AUC, area under the receiver operating characteristic curve; SEN, sensitivity; SPE, specificity; ACC, accuracy; GTV, gross tumor volume; PTV, peritumoral volume; PTV0~+3, the volume of the region 3 mm external to the visible tumor border.
In Figure 4, the image showed the trend of model predictive efficacy with peritumoral distance. The longer the peritumoral distance, the worse the prediction efficiency. The training set AUC values for each independent model were >0.80 in the 0–9 mm range outside the tumor. According to Delong’s test (Table 3), there was no significant difference in the ROC curve area between the GTV, PTV0~+3 and PTV0~+5 models (P>0.05). The PTV0~+5 model was significantly different from the PTV0~+6 model. The Clinical + GTV + PTV0~+3 model was statistically significantly different from the GTV + PTV0~+3 model, Z =2.6685, P=0.0076.
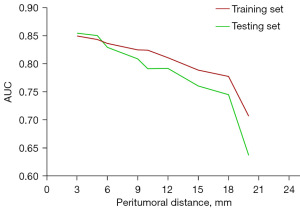
Table 3
Comparison | Z values | P values |
---|---|---|
GTV vs. PTV0~+3 | 0.9245 | 0.3552 |
GTV vs. PTV0~+5 | 0.4479 | 0.6542 |
GTV vs. PTV0~+6 | 0.5725 | 0.567 |
GTV vs. PTV0~+9 | 2.4117 | 0.0159 |
GTV vs. GTV+PTV 0~+3 | 1.6192 | 0.1054 |
GTV vs. Clinical + GTV+ PTV0~+3 | 3.2775 | 0.001 |
PTV0~+3vs. GTV+PTV 0~+3 | 0.2066 | 0.8363 |
PTV0~+3vs. Clinical + GTV + PTV0~+3 | 2.6368 | 0.0084 |
GTV + PTV0~+3vs. Clinical + GTV + PTV0~+3 | 2.6685 | 0.0076 |
PTV0~+3vs. PTV0~+5 | 0.4224 | 0.6728 |
PTV0~+3vs. PTV0~+6 | 1.8903 | 0.0587 |
PTV0~+5vs. PTV0~+6 | 2.37 | 0.0178 |
PTV0~+3vs. PTV+3~+6 | 3.3888 | 0.0007 |
PTV0~+3vs. PTV0~+9 | 3.1913 | 0.0014 |
GTV, gross tumor volume; PTV, peritumoral volume; PTV0~+3, the volume of the region 3 mm external to the visible tumor border.
Development of nomogram
The Clinical + GTV + PTV0~+3 model was selected as the best radiomics model to construct the nomogram. The nomogram was created incorporating the significant clinical predictors and rad-scores. It was shown in Figure 5.
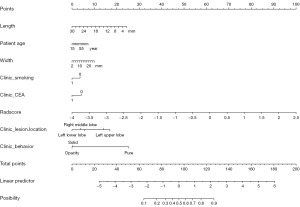
Discussion
High throughput imaging data radiomics analysis energizes the field of lung nodule staging studies (23). In our work, we constructed a Clinical + GTV + PTV0~+3 model (the PTV0~+3 model had the best AUC in peritumoral models) and found that sufficient information was concentrated in the migration zone between normal lung tissue and tumor lesions in the 0–6 mm area surrounding the tumor. The outcome revealed that intratumoral and peritumoral features could improve the prediction of early-stage lung adenocarcinoma subtypes. It provided one more reliable basis for clinical procedures and lesion margin selection.
Radiomics could quantify subtle differences in lung nodules’ internal structure and visualize the lesions’ biological significance (24,25). The current relevant radiomics studies discussed cases with small data sets, which were easily interfered with by extraneous conditions such as images and feature study methods, and intrinsic factors such as the redundancy of radiomics features and the high correlation of some features. These reasons made the selection of radiomics features for model building very different among studies. The radiomics model built in this study contained mainly first-order statistics, a grey dependency matrix, and a grey co-occurrence matrix. In the GTV model, it could be found that the original image had more value, while the derived 3D image had more weight in the peritumoral model. The difference in the distribution of blood vessels and lymphatic vessels around the tumor may be the reason for this (14).
Chen et al. reported that the association between peritumoral radiomics features which extracted from the 0–6 mm region external to the tumor border and lymph node metastasis in clinical stage IA non-small cell lung cancer was a reasonable result (26). Similarly, in our study, the Delong test implied that factors for early lung adenocarcinoma progression might be concentrated in the peritumoral 0–6 mm range. This result might be associated with imaging signs such as indistinct tumor margins or small burr structures that were not accessible to the naked eye of radiologists. In the available experimental results, the diagnostic efficacy of the models decreased as the peritumoral expansion distance increases (Figure 4), which may be related to the size of some lesions and the presence of normal tissue within the expanded area diluting the proportion of peritumoral carcinogenic components. Astonishingly, most independent models still have good diagnostic efficacy (AUC >78%). Das et al. (27) used the multiple LR method to predict lymph node metastasis in 163 cases of cT1N0M0 lung adenocarcinoma by combining intratumoral and peritumoral expansion of 5 mm and lymph node radiomics features. However, the peritumoral 5 mm model only had 50% SPE. The AUCs of the intratumoral and peritumoral model testing sets were 0.76 and 0.72, respectively, which were low compared with the present study. Perrone et al. (28) developed and validated a model to extract quantitative radiomics features from CT images to predict the evolution of non-small cell lung cancer tumors. Nevertheless, Perrone et al. used the “virtual biopsy” concept to extract and analyse only part of the peritumoral area, which may miss more essential parameters. In this study, the complete tumor margin was included, allowing a more comprehensive analysis of the role of peritumoral environmental factors from a multidimensional perspective.
Currently, several studies revealed that the radiomics model of the +3 mm peritumoral area effectively predicted chemotherapy response or EGFR mutation in non-small-cell lung cancer (19,29,30). This study of differentiating LPA and Non-LPA lung cancer also obtained the same peritumoral area (the PTV0~+3 model had the best AUC in peritumoral models). Different from the above studies in which the efficacy of the peritumoral model was higher than that of the intratumoral model, the predictive performance of the GTV, PTV0~+3 and PTV0~+5 models was consistent in this study. It implied that the intratumoral and some peritumoral regions of invasive adenocarcinoma had similar developmental trends in early cancer evolution. However, the optimal peritumoral region can vary depending on the ACC of intratumoral segmentation, further evaluation should be performed.
To date, the identification of lung cancer relies on the imaging pattern of lung nodules on imaging and follow-up strategies. An increase in the solid component of nodules on follow-up CT images was one of the bases on which imaging physicians judge their benign or malignant (31). However, the proportion of solid components did not precisely match the degree of invasiveness (32). In our study, approximately 20% of the 154 lung adenocarcinomas that appeared solid had a predominantly lepidic growth pattern on pathology. It suggested that solid nodules did not always imply a higher degree of malignancy; the finding coincides with the experiments of Hattori et al. and Deng et al. (33,34). The nomogram showed that the pure ground glass nodule characteristic, negative CEA results and non-smoking behavior made the lesion types more inclined to the LPA type. Seeking a basis for selecting a procedure solely from imaging follow-up might be overtreating. Wu et al.’s studies have shown that peritumoral radiomics features of CT images have increasing advantages in non-small cell lung cancer research (35). Our experiment used a combination of intratumoral, peritumoral and clinical labels to construct the predictive model of high diagnostic value (testing set AUC: 0.8919), which could assist in making more appropriate clinical decisions. Vaidya et al. (36) confirmed that combining the advanced image analysis via radiomics and the routine visual assessment of CT scans could help better differentiate adenocarcinoma invasiveness. This was similar to the method used in this study. However, this study found no significant difference in the probability of nodules appearing pure or opacity behavior in LPA group (pure 49%, opacity 46%). The AUC of clinical model was lower than the AUC of radiomics fusion model, so we paid more attention to the experiment of radiomics.
This study’s advantage was using software-compiled AI algorithms to assist and semi-automatically segment the target lesion ROI, reducing errors in lesion margin outlining (37) and using a radiomics approach to visualise the carcinogenic environmental factors inside and outside the tumor. Zhu et al. (38) included data from 1,018 patients diagnosed with ground glass nodule-like lung adenocarcinoma and extracted 1,223 radiomics features within the boundary and around the nodules on enhanced CT to conduct experiments. In this study, 1,781 initial radiomics features were extracted from each target region, which allowed for more excellent mining of potential information on the tumor CT images and increased the reliability of the findings. This study made additional efforts to subdivide the peritumoral regions to enhance the scientific and rigorous nature of the experiment.
There are several shortcomings in this study: (I) a retrospective study with a 5-year time window for collecting patient data, which cannot avoid some biases even when statistical methods were applied to adjust for it; (II) a single-centre study, which requires external validation by other institutions; (III) a grouping based on post-operative pathology reports, with a skewed sample distribution.
Conclusions
The ability of intratumoral and peritumoral radiomics based on preoperative CT images were of high value in predicting early-stage lung invasive adenocarcinoma pathological subtypes (LPA or non-LPA). The diagnostic efficacy of intratumoral-peritumoral radiomics combined clinical model was further improved, with particular reference significance for the preoperative staging and treatment decision.
Acknowledgments
Funding: This work was supported by
Footnote
Reporting Checklist: The authors have completed the TRIPOD reporting checklist. Available at https://tcr.amegroups.com/article/view/10.21037/tcr-23-1324/rc
Data Sharing Statement: Available at https://tcr.amegroups.com/article/view/10.21037/tcr-23-1324/dss
Peer Review File: Available at https://tcr.amegroups.com/article/view/10.21037/tcr-23-1324/prf
Conflicts of Interest: All authors have completed the ICMJE uniform disclosure form (available at https://tcr.amegroups.com/article/view/10.21037/tcr-23-1324/coif). The authors have no conflicts of interest to declare.
Ethical Statement: The authors are accountable for all aspects of the work in ensuring that questions related to the accuracy or integrity of any part of the work are appropriately investigated and resolved. The study was conducted in accordance with the Declaration of Helsinki (as revised in 2013). The study was approved by the Ethics Committee of the Shaoxing People’s Hospital, Shaoxing Hospital, Zhejiang University School of Medicine (No. 2021-K-Y-363-01) and the patient consent requirement was waived because of its retrospective nature.
Open Access Statement: This is an Open Access article distributed in accordance with the Creative Commons Attribution-NonCommercial-NoDerivs 4.0 International License (CC BY-NC-ND 4.0), which permits the non-commercial replication and distribution of the article with the strict proviso that no changes or edits are made and the original work is properly cited (including links to both the formal publication through the relevant DOI and the license). See: https://creativecommons.org/licenses/by-nc-nd/4.0/.
References
- Nicholson AG, Tsao MS, Beasley MB, et al. The 2021 WHO Classification of Lung Tumors: Impact of Advances Since 2015. J Thorac Oncol 2022;17:362-87. [Crossref] [PubMed]
- Song W, Hou Y, Zhang J, et al. Comparison of outcomes following lobectomy, segmentectomy, and wedge resection based on pathological subtyping in patients with pN0 invasive lung adenocarcinoma ≤1 cm. Cancer Med 2022;11:4784-95. [Crossref] [PubMed]
- Altorki N, Wang X, Kozono D, et al. Lobar or Sublobar Resection for Peripheral Stage IA Non-Small-Cell Lung Cancer. N Engl J Med 2023;388:489-98. [Crossref] [PubMed]
- Suzuki K, Watanabe SI, Wakabayashi M, et al. A single-arm study of sublobar resection for ground-glass opacity dominant peripheral lung cancer. J Thorac Cardiovasc Surg 2022;163:289-301.e2. [Crossref] [PubMed]
- Tsai PC, Liu C, Yeh YC, et al. Prognostic histologic subtyping of dominant tumor in resected synchronous multiple adenocarcinomas of lung. Sci Rep 2021;11:9539. [Crossref] [PubMed]
- Ito H, Date H, Shintani Y, et al. The prognostic impact of lung adenocarcinoma predominance classification relating to pathological factors in lobectomy, the Japanese Joint Committee of Lung Cancer Registry Database in 2010. BMC Cancer 2022;22:875. [Crossref] [PubMed]
- Madani MH, Riess JW, Brown LM, et al. Imaging of lung cancer. Curr Probl Cancer 2023;47:100966. [Crossref] [PubMed]
- Li Z, Wu W, Pan X, et al. Serum tumor markers level and their predictive values for solid and micropapillary components in lung adenocarcinoma. Cancer Med 2022;11:2855-64. [Crossref] [PubMed]
- He JH, Ruan JX, Lei Y, et al. Supplementary benefits of CT-guided transthoracic lung aspiration biopsy for core needle biopsy. Front Microbiol 2022;13:1005241. [Crossref] [PubMed]
- Kao TN, Hsieh MS, Chen LW, et al. CT-Based Radiomic Analysis for Preoperative Prediction of Tumor Invasiveness in Lung Adenocarcinoma Presenting as Pure Ground-Glass Nodule. Cancers (Basel) 2022;14:5888. [Crossref] [PubMed]
- Perez-Johnston R, Araujo-Filho JA, Connolly JG, et al. CT-based Radiogenomic Analysis of Clinical Stage I Lung Adenocarcinoma with Histopathologic Features and Oncologic Outcomes. Radiology 2022;303:664-72. [Crossref] [PubMed]
- Zhu M, Yang Z, Zhao W, et al. Predicting Ki-67 labeling index level in early-stage lung adenocarcinomas manifesting as ground-glass opacity nodules using intra-nodular and peri-nodular radiomic features. Cancer Med 2022;11:3982-92. [Crossref] [PubMed]
- Meng Y, Yang Y, Hu M, et al. Artificial intelligence-based radiomics in bone tumors: Technical advances and clinical application. Semin Cancer Biol 2023;95:75-87. [Crossref] [PubMed]
- Yang Y, Yang X, Wang Y, et al. Combined Consideration of Tumor-Associated Immune Cell Density and Immune Checkpoint Expression in the Peritumoral Microenvironment for Prognostic Stratification of Non-Small-Cell Lung Cancer Patients. Front Immunol 2022;13:811007. [Crossref] [PubMed]
- Alvarez MR, Zhou Q, Tena J, et al. Glycomic, Glycoproteomic, and Proteomic Profiling of Philippine Lung Cancer and Peritumoral Tissues: Case Series Study of Patients Stages I-III. Cancers (Basel) 2023;15:1559. [Crossref] [PubMed]
- Noritake O, Aokage K, Suzuki A, et al. Prognostic impact of the number of peri-tumoral alveolar macrophages in patients with stage I lung adenocarcinoma. J Cancer Res Clin Oncol 2022;148:3437-47. [Crossref] [PubMed]
- Zhang X, Zhang G, Qiu X, et al. Radiomics under 2D regions, 3D regions, and peritumoral regions reveal tumor heterogeneity in non-small cell lung cancer: a multicenter study. Radiol Med 2023;128:1079-92. [Crossref] [PubMed]
- Chen Q, Shao J, Xue T, et al. Intratumoral and peritumoral radiomics nomograms for the preoperative prediction of lymphovascular invasion and overall survival in non-small cell lung cancer. Eur Radiol 2023;33:947-58. [Crossref] [PubMed]
- Yamazaki M, Yagi T, Tominaga M, et al. Role of intratumoral and peritumoral CT radiomics for the prediction of EGFR gene mutation in primary lung cancer. Br J Radiol 2022;95:20220374. [Crossref] [PubMed]
- Akinci D'Antonoli T, Farchione A, Lenkowicz J, et al. CT Radiomics Signature of Tumor and Peritumoral Lung Parenchyma to Predict Nonsmall Cell Lung Cancer Postsurgical Recurrence Risk. Acad Radiol 2020;27:497-507. [Crossref] [PubMed]
- Vaidya P, Bera K, Gupta A, et al. CT derived radiomic score for predicting the added benefit of adjuvant chemotherapy following surgery in stage I, II resectable non-small cell lung cancer: a retrospective multicohort study for outcome prediction. Lancet Digit Health 2020;2:e116-28. [Crossref]
- Liu K, Li K, Wu T, et al. Improving the accuracy of prognosis for clinical stage I solid lung adenocarcinoma by radiomics models covering tumor per se and peritumoral changes on CT. Eur Radiol 2022;32:1065-77. [Crossref] [PubMed]
- Chetan MR, Gleeson FV. Radiomics in predicting treatment response in non-small-cell lung cancer: current status, challenges and future perspectives. Eur Radiol 2021;31:1049-58. [Crossref] [PubMed]
- Holzinger A, Haibe-Kains B, Jurisica I. Why imaging data alone is not enough: AI-based integration of imaging, omics, and clinical data. Eur J Nucl Med Mol Imaging 2019;46:2722-30. [Crossref] [PubMed]
- Chen K, Bai J, Reuben A, et al. Multiomics Analysis Reveals Distinct Immunogenomic Features of Lung Cancer with Ground-Glass Opacity. Am J Respir Crit Care Med 2021;204:1180-92. [Crossref] [PubMed]
- Chen QL, Li MM, Xue T, et al. Radiomics nomogram integrating intratumoural and peritumoural features to predict lymph node metastasis and prognosis in clinical stage IA non-small cell lung cancer: a two-centre study. Clin Radiol 2023;78:e359-67. [Crossref] [PubMed]
- Das SK, Fang KW, Xu L, et al. Integrative nomogram of intratumoral, peritumoral, and lymph node radiomic features for prediction of lymph node metastasis in cT1N0M0 lung adenocarcinomas. Sci Rep 2021;11:10829. [Crossref] [PubMed]
- Perrone M, Raimondi E, Costa M, et al. Inflammatory Microenvironment in Early Non-Small Cell Lung Cancer: Exploring the Predictive Value of Radiomics. Cancers (Basel) 2022;14:3335. [Crossref] [PubMed]
- Chang R, Qi S, Zuo Y, et al. Predicting chemotherapy response in non-small-cell lung cancer via computed tomography radiomic features: Peritumoral, intratumoral, or combined? Front Oncol 2022;12:915835. [Crossref] [PubMed]
- Kawazoe Y, Shiinoki T, Fujimoto K, et al. Investigation of the combination of intratumoral and peritumoral radiomic signatures for predicting epidermal growth factor receptor mutation in lung adenocarcinoma. J Appl Clin Med Phys 2023;24:e13980. [Crossref] [PubMed]
- Mazzone PJ, Lam L. Evaluating the Patient With a Pulmonary Nodule: A Review. JAMA 2022;327:264-73. [Crossref] [PubMed]
- Bertolaccini L, Spaggiari L. The importance of being solid for a ground glass opacity of the lung. Ann Transl Med 2021;9:1043. [Crossref] [PubMed]
- Hattori A, Matsunaga T, Fukui M, et al. Prognostic influence of a ground-glass opacity component in hypermetabolic lung adenocarcinoma. Eur J Cardiothorac Surg 2022;61:249-56. [Crossref] [PubMed]
- Deng J, Zhao M, Wang T, et al. A modified T categorization for part-solid lesions in Chinese patients with clinical stage I Non-small cell lung cancer. Lung Cancer 2020;145:33-9. [Crossref] [PubMed]
- Wu L, Lou X, Kong N, et al. Can quantitative peritumoral CT radiomics features predict the prognosis of patients with non-small cell lung cancer? A systematic review. Eur Radiol 2023;33:2105-17. [Crossref] [PubMed]
- Vaidya P, Bera K, Linden PA, et al. Combined Radiomic and Visual Assessment for Improved Detection of Lung Adenocarcinoma Invasiveness on Computed Tomography Scans: A Multi-Institutional Study. Front Oncol 2022;12:902056. [Crossref] [PubMed]
- Cho HH, Lee G, Lee HY, et al. Marginal radiomics features as imaging biomarkers for pathological invasion in lung adenocarcinoma. Eur Radiol 2020;30:2984-94. [Crossref] [PubMed]
- Zhu M, Yang Z, Wang M, et al. A computerized tomography-based radiomic model for assessing the invasiveness of lung adenocarcinoma manifesting as ground-glass opacity nodules. Respir Res 2022;23:96. [Crossref] [PubMed]