Developing and validating the model of tumor-infiltrating immune cell to predict survival in patients receiving radiation therapy for head and neck squamous cell carcinoma
Highlight box
Key findings
• We found that the infiltration of immune cells in the tumor microenvironment (TME) can predict clinical response to radiotherapy (RT) using a novel prognostic model.
What is known and what is new?
• The TME is a key determinant of the response of head and neck cancer to RT and chemotherapy treatments. Insights into the immune landscape of tumors can allow tailoring of therapies to personalize cancer treatment and enhance therapeutic outcomes.
• We developed a prognostic model based on infiltrating immune cells in the TME to predict survival in patients who received RT for head and neck squamous cell carcinoma.
What is the implication, and what should change now?
• Tumor-infiltrating immune cell signature can predict survival outcomes after RT for head and neck cancer. Further prospective investigations should incorporate immune cell characteristics, human papillomavirus status, and tumor mutational burden.
Introduction
Head and neck squamous cell carcinoma (HNSCC) is the sixth most common cancer in the world and can develop in the oral cavity, oropharynx, hypopharynx, larynx, and nasopharynx. Exposure to tobacco, alcohol, and high-risk oncogenic human papillomavirus (HPV) increase the risk of HNSCC. Worldwide, over 500,000 new cases of HNSCC are reported annually (1). Generally, the treatment strategy for HNSCC is multimodal, consisting of surgery, radiotherapy (RT), and chemotherapy. For locally advanced disease, the use of RT as an adjunct to surgery or in conjunction with chemotherapy enhances local control rates. Despite advances in diagnostic and therapeutic approaches, the 5-year overall survival (OS) rate of HNSCC patient only ranges between 40% and 50% (2). Prognosis is generally more favorable for HPV associated oropharyngeal carcinoma, but overall, nearly 50% of patients with locally advanced HNSCC will recur (3). HNSCC is classified based on TNM staging, which includes tumor invasion parameters (T), lymph node involvement (N), and metastases (M). However, this staging system does not account for individual differences in tumor biology and immune features (4). Therefore, this staging has limited prognostic accuracy and does not afford the ability to tailor treatment or surveillance protocols to unique characteristics of the tumor.
HNSCC is characterized by complex relationships between epithelial cells, blood vessels, lymphatic vessels, cytokines, chemokines, and infiltrating immune cells, which collectively form the tumor microenvironment (TME) (5,6). Different types of immune cells participate in tumorigenesis, progression, and recurrence. In general, the TME of most solid tumors is highly immunosuppressive (7,8). Tumor-infiltrating immune cells (TIICs) have been purported to influence the prognosis in HNSCC patients, but data are still accruing. For patients with pancreatic ductal adenocarcinoma (PDAC), a prognostic model based on the TIICs signature could improve the prediction of survival and chemotherapy benefits (9). However, this model only focuses on patients' response to chemotherapy, for patients with head and neck cancer, RT is more important. In recent years, immune checkpoint inhibitors (ICIs) have shown great efficacy and safety in HNSCC, further highlighting the impact of the tumor immune microenvironment (TIME) on clinical outcomes of patients (10-12). These observations suggest a need for tumor-specific prognostic models that leverage data on immune invasion of the TME. In this study, we developed a model whereby data on TIICS in the TME could be used predict survival outcomes in patients undergoing RT for HNSCC.
We analyzed RNA-seq and microarray data from The Cancer Genome Atlas (TCGA) and Gene Expression Omnibus (GEO) databases to build a scoring system, using single-sample gene set enrichment analysis (ssGSEA) to quantify TIICs and improve the accuracy of predicting prognosis for patients with HNSCC. In our study, we collected 397 patients with HNSCC with detailed clinical information from TCGA and GEO (GSE39366) public databases. We used bioinformatics analysis to identify differences in composition of TIICs. In addition, we identified differences in the clinical characteristics, biological function, and tumor mutation burden (TMB) between the tumor-infiltrating cells score (TICS) subgroups. Furthermore, GEO database cohorts were used to validate the results. We present this article in accordance with the TRIPOD reporting checklist (available at https://tcr.amegroups.com/article/view/10.21037/tcr-23-2345/rc).
Methods
Data acquisition and preprocessing
The RNA-seq data with corresponding somatic mutation data of HNSCC patients (N=515) were extracted from the cBio Cancer Genomics Portal (http://cbioportal.org) (an open-access resource for interactive exploration of TCGA database) as the training cohort19. Meanwhile, all patients’ clinical characteristics and follow-up data was obtained, including age, gender, TNM stage, HPV subtype, survival time (months), survival status and treatment. The transcriptome profiles and clinical data of a HNSCC cohort (GSE39366 from GPL9053 platform) (N=138) was downloaded from the Gene Expression Omnibus (GEO) available database (https://www.ncbi.nlm.nih.gov/gds/) for validation. The RNA expression data of original read counts were employing to facilitate the evaluation of immune cell infiltration. The matrix data of gene expression amounts were normalized by the “DESeq2” package of R software (13). These data were obtained from the online public sources, so all informed consents were available. All data included in the analysis were deidentified, and therefore the study was exempt from institutional review board review. The study was conducted in accordance with the Declaration of Helsinki (as revised in 2013).
After merging the transcriptome data and the complete clinical information of patients treated with RT, 293 HNSCC samples from TCGA database were obtained as the training set and 104 HNSCC patients from GSE39366 were taken as validation. Table 1 summarizes the characteristics of all patients in the training and validation cohort.
Table 1
Characteristic | TCGA cohort (N=293) (%) | GEO cohort (N=104) (%) |
---|---|---|
Gender | ||
Male | 230 (78.5) | 75 (72.1) |
Female | 63 (21.5) | 29 (27.9) |
Age (years) | ||
≤60 | 163 (55.6) | 67 (64.4) |
>60 | 130 (44.4) | 37 (35.6) |
T stage | ||
T1–2 | 79 (27.0) | 24 (23.1) |
T3–4 | 167 (57.0) | 62 (59.6) |
Unknown | 47 (16.0) | 18 (17.3) |
N stage | ||
N0 | 73 (24.9) | 25 (24.0) |
N1–3 | 163 (55.6) | 61 (58.7) |
Unknown | 57 (19.5) | 18 (17.3) |
HPV | ||
Positive | 50 (17.1) | 13 (12.5) |
Negative | 228 (77.8) | 62 (59.6) |
Unknown | 15 (5.1) | 29 (27.9) |
HNSCC, head and neck squamous cell carcinoma; TCGA, The Cancer Genome Atlas; GEO, Gene Expression Omnibus; HPV, human papillomavirus.
Calculation of TICS and TMB
For each tumor sample, the abundance of each of 26 immune cell types was calculated using the ssGSEA algorithm in the “GSVA” package of R software (R version 4.2.1). Immune score, Estimation of STromal and Immune cells in MAlignant Tumor tissues using Expression data (ESTIMATE) score, and stromal score of HNSCC samples were calculated using the “estimate” package. The TMB was defined as the number of somatic mutations per megabase, including single nucleotide variants (SNVs) and small insertions and deletions (Indels). We analyzed the relationship between different TMB subgroups and OS.
Construction and evaluation of a TICS model
Prognostic-related TIICs were filtered through the univariate and multivariate Cox regression analysis. Then, a TICS model was constructed using immune cells that had prognostic significance for cancer survival (P<0.05). The cell types included mast cells, activated B cells, effector memory CD4 T cells, CD56bright natural killer (NK) cells and eosinophils. Their coefficient from multivariate Cox regression was incorporated using the following formula: . GSVA score reflects the prevalence of infiltrating immune cells associated with progression-free survival (PFS). According to the meta-analysis, n is the number of immune cells associated with recurrence-free survival (RFS). HRi is the hazard ratio (HR) of each PFS-related immune cell and SE(HRi) is the standard error of HRi. Based on the optimal cutoff value of the TICS truncated by the “survminer” package in the R software, patients with HNSCC were divided into high and low score groups, and Kaplan-Meier (KM) survival analysis was performed in the two TICS subgroups using the log-rank test, and time-dependent receiver operating characteristic (ROC) curves of the TICS model were drawn. Furthermore, the validation cohort downloaded from GEO was used to construct a prognostic TICS model using the same method.
Differential analysis
The differential analysis of DEGs was conducted according to Gene Ontology (GO) and Kyoto Encyclopedia of Genes and Genomes (KEGG) based on the “clusterProfiler” package in R (P<0.05 and |log2fold change (FC)| >1) (14). Subsequently, the P value was adjusted for multiple tests to control the false discovery rate (FDR) using the Benjamini-Hochberg (BH) procedure. A database of chemotherapy drugs was extracted from Genomics of Drug Sensitivity in Cancer (GDSC) (15) and a 50% maximal inhibitory concentration (IC50) was calculated using the pRRophetic R package (16). A total of 14 m6A regulatory factors were extracted from TGGA to analyze the difference of m6A between TICS subgroups (17).
Clinical and pathological characteristics of the TICS model
We collected data on clinical characteristics of the patients and HNSCC tumors, including gender, age, TNM staging, HPV status, along with the TICS, immune score, ESTIMATE score, stromal score, TMB, immune checkpoint related-genes, major histocompatibility complex (MHC) genes and chemokines genes. Data from samples were used to create a heat map. The prognostic evaluation of the clinical pathological characteristics was based on univariate and multivariate Cox regression. Then, a nomogram was constructed to clearly visualize the results of multivariate Cox regression and a calibration chart was also developed to verify the ability of TICS to predict 1-, 3-, and 5-year survival.
Statistical analysis
We analyzed all our data with R 4.2.1 (Auckland, New Zealand) including the Wilcoxon rank-sum test, analysis of variance (ANOVA), and BH. The P values for multiple comparisons were adjusted using BH. Using the “survminer” R package, the optimal cutoff values for TICS and TMB were determined. TICS and other continuous variables were correlated using Spearman’s correlation coefficient. The KM survival curve was used to distinguish the difference of the clinical outcome between the different TICS subgroups, and the significance of the difference was calculated by the log-rank test. Univariate and multivariate Cox regression was undertaken to identify the relevant independent prognostic factors. In additional, time-ROC was used to evaluate the prognostic diagnostic accuracy of TICS and other variables for the prognosis of HNCSS patients. Calibration curve was used to evaluate the predictive accuracy of nomogram model. All P values were reported 2-sided with a significance level of 0.05.
Results
Construction of the TICS prognostic model
The baseline data of TCGA and GEO cohort are shown in Table 1. Univariate and multivariate cox regression were conducted to analyze the prognostic value of 26 immune cells. Following screening of all 26 immune cell populations, we identified 5 significant prognostic immune cells based on their stable and pooled HR and coefficients (mast cells; activated B cells; effector memory CD4 T cells; CD56bright NK cells; eosinophils). The selected 5 TIICs all demonstrated a protective effect for progression free survival in patients with HNSCC (all HR <1, P<0.05) (Figure 1A).
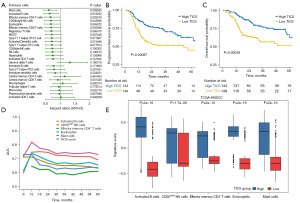
The TICS of each sample was counted using the GVSA formula outlined in the methods section. According to the optimal cutoff value, all patients in TCGA and GEO database were divided into two subgroups. The baseline characteristics between the two TICS subgroups in the training and validation cohorts are shown in Table 2. The results of survival curves suggested that patient in the high TICS subgroup had a significantly better PFS (log-rank P=0.00087, Figure 1B). Moreover, higher TICS predicted better OS (log-rank P=0.00038, Figure 1C). TICS had greater predictive value than any of the 5 separate immune cells according to the time-dependent ROC analysis in the training cohort (Figure 1D, all P<0.05). The high TICS subgroups expressed higher levels of each of the 5 significant prognostic immune cells, which reinforced the protective effect of these cell populations (Wilcoxon test, all P<0.001) (Figure 1E). The abundance of tumor infiltrating cell types was significantly higher in the high TICS group for all cell types with the exception of central memory CD4 T cells (Figure S1).
Table 2
Characteristic | TCGA cohort | GEO cohort | |||||
---|---|---|---|---|---|---|---|
High score (N=144) (%) | Low score (N=149) (%) | P value | High score (N=53) (%) | Low score (N=51) (%) | P value | ||
Gender | 0.577 | 0.923 | |||||
Male | 115 (79.9) | 115 (77.2) | 38 (71.7) | 37 (72.5) | |||
Female | 29 (20.1) | 34 (22.8) | 15 (28.3) | 14 (27.5) | |||
Age (years) | 0.465 | 0.953 | |||||
≤60 | 77 (53.5) | 86 (57.7) | 34 (64.2) | 33 (64.7) | |||
>60 | 67 (46.5) | 63 (42.3) | 19 (35.8) | 18 (35.3) | |||
T stage | 0.033 | 0.350 | |||||
T1–2 | 46 (31.9) | 33 (22.1) | 14 (26.4) | 10 (19.6) | |||
T3–4 | 71 (49.3) | 96 (64.4) | 28 (52.8) | 34 (66.7) | |||
Unknown | 27 (18.8) | 20 (13.4) | 11 (20.8) | 7 (13.7) | |||
N stage | 0.161 | 0.200 | |||||
N0 | 30 (20.8) | 43 (28.9) | 9 (17.0) | 16 (31.4) | |||
N1–3 | 81 (56.3) | 82 (55.0) | 33 (62.3) | 28 (54.9) | |||
Unknown | 33 (22.9) | 24 (16.1) | 11 (20.8) | 7 (13.7) | |||
HPV | 0.011 | 0.101 | |||||
Positive | 34 (23.6) | 16 (10.7) | 10 (18.9) | 3 (5.9) | |||
Negative | 102 (70.8) | 126 (84.6) | 31 (58.5) | 31 (60.8) | |||
Unknown | 8 (5.6) | 7 (4.7) | 12 (22.6) | 17 (33.3) |
TCGA, The Cancer Genome Atlas; GEO, Gene Expression Omnibus; TICS, tumor-infiltrating cell score; HPV, human papillomavirus.
Validation of the TICS prognostic model
An independent cohort with 104 HNSCC patients from the GEO dataset GSE39366 was used to externally validate the model accuracy. Table 2 shows the clinical data between the high TICS group (n=53) and the low TICS group (n=51) in the training sets. Similarly, in the GEO validation set, the KM curve indicated that the RFS of patients in the high TICS group were better than those in the low TICS group (log-rank P=0.025) (Figure 2A). The expression levels of the 5 prognostic-related cells were significantly higher in the high TICS group (Wilcoxon test, all P<0.001) (Figure 2B) and the abundance of tumor-infiltrating cells was significantly higher (Figure S2).
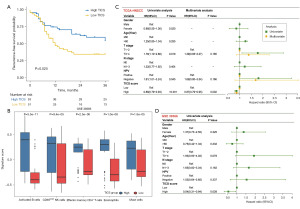
Prognostic analysis of TICS subgroups
Subsequently, to examine independent predictors of prognosis in both training and validation cohorts, univariate and multivariate Cox regression analyses were conducted on TICS and multiple clinical features (gender, age, T stage, N stage, HPV status). Figure 2C shows that high T stage (HR =1.78, P=0.019) and HPV negativity [HR =1.81, 95% confidence interval (CI): 1.01–3.24, P=0.045] were significant risk factors in univariate analyses, whereas TICS (HR =0.86, 95% CI: 0.79–0.93, P<0.001) was the only protective factor in the training set. Next, the multivariate Cox analyses revealed that high TICS (HR =0.87, 95% CI: 0.79–0.95, P=0.002) was still the significant protective factor. In the GEO validation cohorts, only TICS was an independent prognostic factor for RFS (HR =0.54, 95% CI: 0.31–0.94, P=0.028) (Figure 2D).
Given the significant differences in TIME of HPV positive and negative HNSCC and the remarkable prognostic impact of the HPV status, we divided all samples into subgroups to equalize the discrepancy of HPV status. We first compared the clinical outcome of the HPV subgroups. As expected, HPV-positive HNSCC patients showed better OS than HPV-negative patients (log-rank P=0.0015) (Figure 3A). To further verify the accuracy of the model, we evaluated the synergistic effect of HPV status and TICS in prognostic stratification of HNSCC. Stratified survival analysis revealed that HPV-negative patients who had low TICS and the lowest PFS among the four subgroups (log-rank P=0.0045) (Figure 3A). Furthermore, multiple comparison revealed that in the HPV-negative subgroup, TICS score could still predict progression free-survival (high TICS/HPV- vs. low TICS/HPV-, log-rank test, P=0.031) (Figure 3A). Thus, a nomogram was established based on TICS and HPV to predict the PFS (Figure 3B). The prediction capacity of TICS was performed using a calibration diagram for 1-, 3-, and 5-year survival predictions (Figure 3C-3E). In the calibration plot, a solid blue line represented the predicted survival, and a diagonal dotted line represented the actual survival. Prediction capacity enhances as the blue solid line approaches the diagonal dotted line.
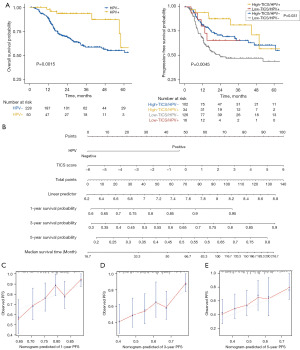
Biofunctional analysis of TICS subgroups
To clarify the potential biological characteristics of distinct immunophenotypes, we performed DEG analysis to determine the transcriptome variations between TICS subgroups by “DESeq2” package of R software. A total of 1,686 DEGs with log2FC values >1 and FDR-adjusted P values <0.05 were identified, with 1,475 up-regulated genes and 211 down-regulated genes in the high TICS group (Figure S3). To explore the molecular mechanisms of DEGs, GO analysis showed that the 1,475 up-regulated genes were mainly enriched in the biological processes of immune-related pathways such as immune cell activation, adhesion, and proliferation (Figure 4A), whereas the 211 down-regulated genes were mostly enriched in receptor ligand activity and signaling receptor activator activity (Figure 4B). GSEA analysis revealed that “positive T cell selection”, “immune receptor activity”, “chemokine binding”, and “T cell receptor complex” pathways were most frequently enriched in the high TICS group (Figure 4). The functional differences between the TICS subgroups were explored, which may be potential targets to prolong the survival of patients.
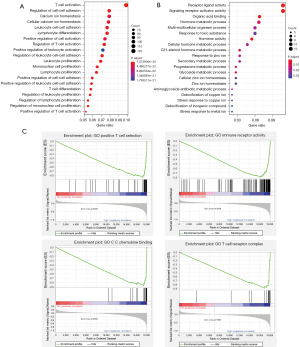
Given the high number of immune-related pathways that were enriched, we investigated whether immune status and clinical features differed between patients in the TICS subgroups. The immune checkpoints, chemokines, and MHC gene sets were collected and analyzed in the training cohort. Most immune checkpoint, chemokines, and MHC genes were more highly expressed in the high TICS group than in the low TICS group (Figure 5A, all P<0.001).
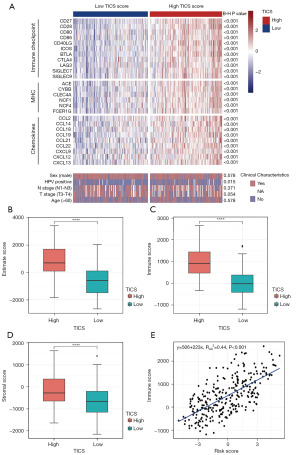
Immune status which included the immune score, stromal score, and estimated score, was assessed using ESTIMATE algorithms. Then the significance of each sample between the two groups of TICS was further compared using Wilcoxon test. As shown in the boxplots, there were statistically significant differences in immune scores, stromal scores, and estimated scores among 293 patients with HNSCC, and all of them were highly expressed in the high TICS group (Figure 5B-5D). Based on the scatterplot of correlation coefficients, the TICS score and immune score showed a significant, strong positive correlation (R2=0.44, P<0.001, Figure 5E).
The expression levels of m6A regulators were compared between the two TICS groups. A total of 14 m6A regulators were matched, and the expression levels of FMR1, METTL14, RBM15 and RBM15B were significantly higher in high TICS group: however, the remaining 8 m6A regulators displayed no significant differences between the two TICS groups (Figure 6A-6D).
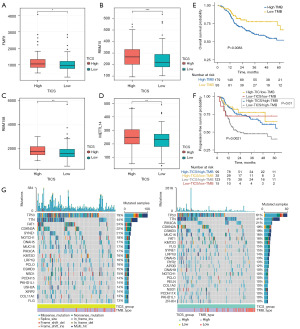
Somatic genome characteristics of TICS subgroups
We next explored the relationship between TMB, TICS and post-radiation HNSCC survival outcomes. The KM survival curve showed that the survival outcomes of HNSCC patients with low TMB were significantly higher than patients with high TMB (log-rank P=0.0083, Figure 6E). Next, we analyzed patients in four groups, based on high/low TMB and high/low TICS. The stratified analysis showed that patients with high TICS score and low TMB had the best PFS. As demonstrated in Figure 6F, patients with low TICS and high TMB had the worst PFS (log-rank P=0.0021). In addition, multiple comparison showed that in the high TMB subgroup, TICS still showed significant prognostic value (high TICS/high TMB vs. low TICS/high TMB, log-rank test, P=0.01, adjust by BH method). Moreover, we assessed the distribution of somatic variants in HNSCC between the low and high TICS subgroups to elucidate the genetic imprints (Figure 6G). In both the high and low TICS subgroup, TP53 and TTN were the most frequently mutated genes, consistent with the role of these mutations in cell cycle dysregulation, cancer progression, tumor angiogenesis, and metastasis.
Sensitivity of chemotherapy drugs
Given the significance of chemotherapy in the treatment of HNSCC, we quantified the responses to 123 chemotherapeutic drugs among HNSCC patients with different TICS using GDSC database. The IC50 values for 12 commonly used chemotherapy drugs were compared between two TICS subgroups, with a lower IC50 value indicating better sensitivity to the drug in this group. We found significantly lower IC50 levels for bortezomib, crizotinib, and phenformin in the high TICS subgroup (Figure 7A-7C). Conversely, significantly lower IC50 levels for bleomycin, cetuximab, cisplatin, cytarabine, docetaxel, doxorubicin, epothilone B, gemcitabine, and tipifarnib were found in the low TICS subgroup (Figure 7D-7L), which indicated that HNSCC patients with low TICS were more susceptible to these drugs. Thus, chemotherapy regimen such as GP (gemcitabine plus platinum) and TP (docetaxel plus platinum), or anti-EGFR targeted therapy (cetuximab) may better improve the survival of low TICS patients.
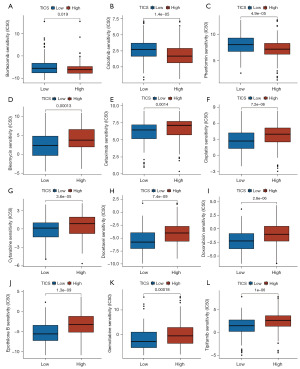
Discussion
Individualized surveillance plans and clinical decisions require highly accurate prognostic assessments of HNSCC. We aimed to develop specific immune cell models to identify patients who might benefit from RT according to the biological characteristics of head and neck tumors. We identified 5 immune cells significantly associated with prognosis of HNSCC: mast cells, activated B cells, effector memory CD4 T cells, CD56bright NK cells, and eosinophils.
Tumors have distinct immune microenvironments, which can influence prognosis. By analyzing the expression data of the reference queue, Cell-type Identification by Estimating Relative Subsets of RNA Transcripts (CIBERSORT) determines the relative expression of immune cells. As a result of the heterogeneity of the tumors, the reference cohort was not completely consistent with the actual patient situation, but the ssGSEA method provided a stable production route without such shortcomings. A unique advantage of TICS is that it integrates OS and immune microenvironment characteristics; TICS rectified the prognostic coefficient of OS for the equivalent expression of TIICs.
The TME and peripheral blood of patients with advanced HNSCC have been found to be populated with dysfunctional immune cells (18,19). Due to the immunosuppressive effects of the TME (20,21), these changes enable tumors to escape immune recognition. Human leukocyte antigen (HLA) class 1 expression is frequently decreased or altered in tumor cells (22) and the antigen processing machinery is often deficient in tumor cells (23,24).
In previous studies, we showed that oropharyngeal squamous cell carcinoma (OPSCC), which arises from squamous epithelium related to tonsillar lymphoid tissue, has more infiltrating immune cells (CD4, CD8, and Foxp3) than other subsites. Furthermore, CD8+ T cell abundance was correlated with enhanced prognosis in OPSCC. Increased tumor-infiltrating lymphocyte (TIL) density was associated with enhanced disease-free survival (DFS) and OS in patients with resectable laryngeal cancer (25,26). Balermpas et al. reported on a cohort of 161 patients with HNSCC treated with surgery and chemoradiation and identified high CD8 expression as an independent prognostic factor for OS (27). A glycoprotein heterodimer with alpha and beta chains covalently linked by a disulfide bond, CD8 serves as a co-receptor for T-cells. In the context of tumor targeting, CD8+ TILs play a crucial role in cell-mediated immunity. Using CD8 in conjunction with the T-cell receptor, TILs can kill tumor cells by binding to the MHC I molecule (28-30). Recently, preclinical studies on the combination of radiation and ICIs have highlighted the influence of host CD8+ cytotoxic TILs on radiation response (31-34). RT responses were dramatic when TILs were stimulated, including long-term immunity, whereas anti-CD8 antibody abolished this therapeutic response, which provided solid evidence on their major role in radiation response (34-36). HNSCC promotes Fas/FasL-mediated and Bax/Bcl-XL-mediated apoptosis of circulating TILs. In addition, apoptosis-sensitive TIL subsets, such as CD8(+) CCR7(+) T cells, can predict recurrence in HNSCC patients (37-39).
In our study, mast cells, activated B cells, effector memory CD4 T cells, CD56bright NK cells, and eosinophils, were all positively related to TICS. It is noteworthy that CD8+ T cells are not incorporated in TICS. Although infiltrating cytotoxic T cells are connected to the efficacy of immunotherapy, the fate is largely determined by TME. The immunosuppressive TME of HNSCC may deplete the infiltrating CD8+ T cells.
TIL B cells in HNSCC can present tumor antigen to CD4+ T cells, contributing to antitumor immunity. This is consistent with our prognosis model. The activated B cells were expressed at a high level in the high TICS subgroup with a favorable prognosis. This finding indicates that the B cells in the TME may be activated and act with immune regulatory molecules to fight against tumor cells, potentially combining with T cell-mediated immunotherapy in the future. Since differences between HPV positivity and HPV negativity have been reported in HNSCC patients in previous studies (1,3), these differences should be taken into account in subsequent B cell-focused immunotherapy.
After adjusting for tumor type and stage, mast cell density was correlated with prognosis. Mast cells play a significant role in the TME by influencing proliferation, angiogenesis, invasiveness, and metastasis. Despite being underrecognized, mast cells are very promising cancer immunotherapy targets. The relationship of mast cells to prognosis identified in this study has never been reported in HNSCC before. Mast cells are thought to be related to chemotherapy resistance of breast cancer based on bioinformatic analysis (40). In gastric cancer (41,42), esophageal squamous cell carcinoma (43), ovarian cancer (44), and diffuse large B-cell lymphoma (45), mast cells have been identified as favorable prognostic factors, whereas in breast cancer (46), pulmonary adenocarcinoma (47), and melanoma (48), mast cells have been identified as negative prognostic factors. Mast cells accumulate in cancer due to various growth factors and chemokines, including stem cell factor (SCF), vascular endothelial growth factor (VEGF), CCL2, IL-8, complement, and PGE2 (49,50). Mast cells can be either tumorigenic or antineoplastic due to their various properties and immunomodulatory effects on activation or degranulation. Therefore, the effect of mast cells on HNSCC depends on which receptors of immune cells recruited in the TME are activated.
NK cells are key to anti-tumor immunity. NK cells have the potential to fight a wide range of heterogeneous tumor types (51). However, TME can lead to dysfunction of NK cells, and the degree of damage is associated with the prognosis of many tumors. Previous studies have found that the TME is helpful to tumor cell metabolism, but harmful to lymphocyte metabolism, and NK cells require substantial biological energy to achieve an effective anti-tumor response (52,53). Metabolic flexibility is crucial to the anti-tumor activity of NK cells in the TME. Through IL-21 signaling of STAT3 (53), Warburg-like NK cells are amplified, making them better adapted to TME, thereby exerting powerful anti-tumor effects. The NK cells identified and verified in this study show improved prognosis in HNSCC. Thus, immunotherapy to target NK cells based on metabolic flexibility provide more opportunities for future treatment of HNSCC.
Physiologically, eosinophils make up 1–3% of the total leukocyte population. The chemokines CCL17 and CCL22 exert immunomodulatory effects on eosinophils by recruiting Th2 cells and Treg cells. Eosinophils have been reported to exert cytotoxic effects on breast cancer in vitro (54), whereas eosinophil count in vivo is inversely correlated with recurrence risk in breast cancer patients (55). Eosinophils have obvious anti-tumor effects in melanoma (56), colorectal cancer (57), non-small cell lung cancer (NSCLC) (58), and oral squamous cell carcinoma (OSCC) (59). Conversely, eosinophils can promote tumorigenesis in cervical cancer (60). This suggests that diverse subsets of eosinophils may play dissimilar roles in different tumor histotypes. Our study shows that eosinophils have significant prognostic value in HNSCC, which is consistent with previous studies (55-59). Based on the above findings, the identification of more immune regulatory factors for eosinophils may provide novel targets for the immunotherapy in HNSCC, and eosinophils count may be used as a biomarker for cancer treatment to measure treatment response.
Checkpoints in the immune system protect against autoimmune reactions and maintain the immune system’s self-tolerance. Immune checkpoint molecules function as regulators to keep the immune system in check. Immune checkpoint molecules have been the subject of extensive research, including programmed cell death receptor-1 (PD-1), programmed cell death receptor ligand-1 (PD-L1), programmed cell death receptor ligand-2 (PD-L2), lymphocyte activation-gene-3 (LAG-3), cytotoxic T lymphocyte antigen-4 (CTLA-4), B and T lymphocyte attenuator (BTLA). These regulators play important roles in the anti-tumor immune response by mediating a fine balance between host immune competency and immunosuppression (61). Our study found that LAG-3, CTLA-4, and BTLA were up-regulated in the high TICS group. The finding that the high TICS group was associated with better prognosis is consistent with the immune regulatory factors in the HNSCC TME that upregulate immune signal transduction, immune cells attack cancer cells, and cytokine secretion.
In order to devise strategies for the manipulation of cells as future treatment strategies for HNSCC, it is essential to understand how immune cells are stimulated. However, a better understanding of how immune cells contribute to HNSCC pathogenesis is also needed.
The immune cell composition and abundance between HPV-negative and HPV-positive HNSCC tumors environment are notably different. What’s more, HPV-positive HNSCC has been reported to be more radiosensitive in-vivo compared to HPV-negative disease. Specifically, HPV-positive tumors generally have a greater abundance of immune infiltration and inflammatory cytokines, which may contribute to the better tumor clearance after irradiation and typically indicate a better survival. Thus, we divided patients into four subgroups based on the status of HPV infection and TLCS. Stratified survival analysis revealed that TICS model could still distinguish the prognosis of HNSCC within different HPV status, especially in the HPV-negative subgroup.
In this study, the clinicopathological features, immune characteristics, and immune pathways were also compared among TICS subgroups. Several differences were identified in immune signatures and immune pathways. Therefore, TICS allows for immunobiological classification of HNSCC patients. Furthermore, TMB has been shown to be a promising biomarker for predicting cancer prognosis and immune response (62). We found that high TMB was associated with genes (TTN and TP53), which are mainly involved in cancer progression, tumor angiogenesis, and metastasis (63-65). Patients with low TICS and high TMB had the worst prognosis, attributed to weaker immune profile and greater dysregulation. TICS score predicted prognosis in the high TMB group. This suggests that messenger RNA (mRNA)-based immune cell signals may reflect somatic mutation of tumor DNA.
We further explored differences in response to chemotherapy drugs between TICS subgroups. According to our data, patients with HNSCC in the high TICS group were more sensitive to bortezomib, crizotinib, and phenformin. The patients in the low TICS group were more sensitive to bleomycin, cetuximab, cisplatin, cytarabine, docetaxel, doxorubicin, epothilone B, gemcitabine, and tipifarnib. Our research found that TICS identified potential biomarkers and therapeutic targets. Chemotherapy can decompose cancer cells, increasing the level of antigens available to antigen presenting cells (66). Ultimately, it suggested that immune cell characteristics are useful in predicting chemotherapeutic response.
Although the results of our study can improve the prediction of prognosis for HNSCC receiving RT, it had some limitations. Firstly, we observed only 26 types of immune cells; other types of immune cells in TME should be included as well, which may also be related to the prognosis of HNSCC. Secondly, we need to further explore the specific mechanisms that may be involved between immune cells and the prognosis of HNSCC and verify them through in vivo and in vitro experiments. While biobank data is a useful repository of relevant patient samples, it is important to validate the findings in this study in prospective studies of HNSCC patients, to translate the findings to the clinical treatment pathway.
Conclusions
The TICS scoring model based on the abundance of immune infiltration and prognosis can assist with individualized counseling and treatment planning for patients with HNSCC. The presence of a large proportion of infiltrating immune cells in TME is associated with better PFS in patients receiving RT.
Acknowledgments
The authors appreciate the great support from Dr. Yuvnik Trada (University of Sydney, Australia) in improving the quality of this paper.
Funding: This work was supported by
Footnote
Reporting Checklist: The authors have completed the TRIPOD reporting checklist. Available at https://tcr.amegroups.com/article/view/10.21037/tcr-23-2345/rc
Peer Review File: Available at https://tcr.amegroups.com/article/view/10.21037/tcr-23-2345/prf
Conflicts of Interest: All authors have completed the ICMJE uniform disclosure form (available at https://tcr.amegroups.com/article/view/10.21037/tcr-23-2345/coif). The authors have no conflicts of interest to declare.
Ethical Statement: The authors are accountable for all aspects of the work in ensuring that questions related to the accuracy or integrity of any part of the work are appropriately investigated and resolved. The study was conducted in accordance with the Declaration of Helsinki (as revised in 2013). This research used publicly available and de-identified data for analysis and thus ethical approval was not required.
Open Access Statement: This is an Open Access article distributed in accordance with the Creative Commons Attribution-NonCommercial-NoDerivs 4.0 International License (CC BY-NC-ND 4.0), which permits the non-commercial replication and distribution of the article with the strict proviso that no changes or edits are made and the original work is properly cited (including links to both the formal publication through the relevant DOI and the license). See: https://creativecommons.org/licenses/by-nc-nd/4.0/.
References
- Torre LA, Bray F, Siegel RL, et al. Global cancer statistics, 2012. CA Cancer J Clin 2015;65:87-108. [Crossref] [PubMed]
- Bai G, Yue S, You Y, et al. An integrated bioinformatics analysis of the S100 in head and neck squamous cell carcinoma. Transl Cancer Res 2023;12:717-31. [Crossref] [PubMed]
- Rettig EM, D'Souza G. Epidemiology of head and neck cancer. Surg Oncol Clin N Am 2015;24:379-96. [Crossref] [PubMed]
- Vahabi M, Blandino G, Di Agostino S. MicroRNAs in head and neck squamous cell carcinoma: a possible challenge as biomarkers, determinants for the choice of therapy and targets for personalized molecular therapies. Transl Cancer Res 2021;10:3090-110. [Crossref] [PubMed]
- Sharma P, Hu-Lieskovan S, Wargo JA, et al. Primary, Adaptive, and Acquired Resistance to Cancer Immunotherapy. Cell 2017;168:707-23. [Crossref] [PubMed]
- Chen YP, Wang YQ, Lv JW, et al. Identification and validation of novel microenvironment-based immune molecular subgroups of head and neck squamous cell carcinoma: implications for immunotherapy. Ann Oncol 2019;30:68-75. [Crossref] [PubMed]
- Mandal R, Şenbabaoğlu Y, Desrichard A, et al. The head and neck cancer immune landscape and its immunotherapeutic implications. JCI Insight 2016;1:e89829. [Crossref] [PubMed]
- Whiteside TL. Immunobiology of head and neck cancer. Cancer Metastasis Rev 2005;24:95-105. [Crossref] [PubMed]
- Gao Y, Chen S, Vafaei S, et al. Tumor-Infiltrating Immune Cell Signature Predicts the Prognosis and Chemosensitivity of Patients With Pancreatic Ductal Adenocarcinoma. Front Oncol 2020;10:557638. [Crossref] [PubMed]
- Hayman TJ, Bhatia AK, Jethwa KR, et al. Combinations of immunotherapy and radiation therapy in head and neck squamous cell carcinoma: a narrative review. Transl Cancer Res 2021;10:2571-85. [Crossref] [PubMed]
- Sharon S, Bell RB. Immunotherapy in head and neck squamous cell carcinoma: a narrative review. Front Oral Maxillofac Med 2022;4:28. [Crossref] [PubMed]
- Chen L, Mo DC, Hu M, et al. Combination therapy with immune checkpoint inhibitors in recurrent or metastatic squamous cell carcinoma of the head and neck: A meta-analysis. Int Immunopharmacol 2023;119:110270. [Crossref] [PubMed]
- Love MI, Huber W, Anders S. Moderated estimation of fold change and dispersion for RNA-seq data with DESeq2. Genome Biol 2014;15:550. [Crossref] [PubMed]
- Yu G, Wang LG, Han Y, et al. clusterProfiler: an R package for comparing biological themes among gene clusters. OMICS 2012;16:284-7. [Crossref] [PubMed]
- Yang W, Soares J, Greninger P, et al. Genomics of Drug Sensitivity in Cancer (GDSC): a resource for therapeutic biomarker discovery in cancer cells. Nucleic Acids Res 2013;41:D955-61. [Crossref] [PubMed]
- Geeleher P, Cox N, Huang RS. pRRophetic: an R package for prediction of clinical chemotherapeutic response from tumor gene expression levels. PLoS One 2014;9:e107468. [Crossref] [PubMed]
- Wang S, Sun C, Li J, et al. Roles of RNA methylation by means of N(6)-methyladenosine (m(6)A) in human cancers. Cancer Lett 2017;408:112-20. [Crossref] [PubMed]
- Czystowska M, Gooding W, Szczepanski MJ, et al. The immune signature of CD8(+)CCR7(+) T cells in the peripheral circulation associates with disease recurrence in patients with HNSCC. Clin Cancer Res 2013;19:889-99. [Crossref] [PubMed]
- Norouzian M, Mehdipour F, Ashraf MJ, et al. Regulatory and effector T cell subsets in tumor-draining lymph nodes of patients with squamous cell carcinoma of head and neck. BMC Immunol 2022;23:56. [Crossref] [PubMed]
- Ferris RL. Immunology and Immunotherapy of Head and Neck Cancer. J Clin Oncol 2015;33:3293-304. [Crossref] [PubMed]
- Davis RJ, Van Waes C, Allen CT. Overcoming barriers to effective immunotherapy: MDSCs, TAMs, and Tregs as mediators of the immunosuppressive microenvironment in head and neck cancer. Oral Oncol 2016;58:59-70. [Crossref] [PubMed]
- Robbins Y, Friedman J, Redman J, et al. Tumor cell HLA class I expression and pathologic response following neoadjuvant immunotherapy for newly diagnosed head and neck cancer. Oral Oncol 2023;138:106309. [Crossref] [PubMed]
- Dhatchinamoorthy K, Colbert JD, Rock KL. Cancer Immune Evasion Through Loss of MHC Class I Antigen Presentation. Front Immunol 2021;12:636568. [Crossref] [PubMed]
- Concha-Benavente F, Srivastava R, Ferrone S, et al. Immunological and clinical significance of HLA class I antigen processing machinery component defects in malignant cells. Oral Oncol 2016;58:52-8. [Crossref] [PubMed]
- Gkegka AG, Koukourakis MI, Katotomichelakis M, et al. Cancer Microenvironment Defines Tumor-Infiltrating Lymphocyte Density and Tertiary Lymphoid Structure Formation in Laryngeal Cancer. Head Neck Pathol 2023;17:422-32. [Crossref] [PubMed]
- Vassilakopoulou M, Avgeris M, Velcheti V, et al. Evaluation of PD-L1 Expression and Associated Tumor-Infiltrating Lymphocytes in Laryngeal Squamous Cell Carcinoma. Clin Cancer Res 2016;22:704-13. [Crossref] [PubMed]
- Balermpas P, Rödel F, Rödel C, et al. CD8+ tumour-infiltrating lymphocytes in relation to HPV status and clinical outcome in patients with head and neck cancer after postoperative chemoradiotherapy: A multicentre study of the German cancer consortium radiation oncology group (DKTK-ROG). Int J Cancer 2016;138:171-81. [Crossref] [PubMed]
- Farhood B, Najafi M, Mortezaee K. CD8(+) cytotoxic T lymphocytes in cancer immunotherapy: A review. J Cell Physiol 2019;234:8509-21. [Crossref] [PubMed]
- Wu D, Chen Y. Lipids for CD8(+) TILs: Beneficial or harmful? Front Immunol 2022;13:1020422. [Crossref] [PubMed]
- Philip M, Schietinger A. CD8(+) T cell differentiation and dysfunction in cancer. Nat Rev Immunol 2022;22:209-23. [Crossref] [PubMed]
- Herrera FG, Ronet C, Ochoa de Olza M, et al. Low-Dose Radiotherapy Reverses Tumor Immune Desertification and Resistance to Immunotherapy. Cancer Discov 2022;12:108-33. [Crossref] [PubMed]
- Deng L, Liang H, Burnette B, et al. Irradiation and anti-PD-L1 treatment synergistically promote antitumor immunity in mice. J Clin Invest 2014;124:687-95. [Crossref] [PubMed]
- Du SS, Chen GW, Yang P, et al. Radiation Therapy Promotes Hepatocellular Carcinoma Immune Cloaking via PD-L1 Upregulation Induced by cGAS-STING Activation. Int J Radiat Oncol Biol Phys 2022;112:1243-55. [Crossref] [PubMed]
- Vanpouille-Box C, Alard A, Aryankalayil MJ, et al. DNA exonuclease Trex1 regulates radiotherapy-induced tumour immunogenicity. Nat Commun 2017;8:15618. [Crossref] [PubMed]
- Sheng H, Huang Y, Xiao Y, et al. ATR inhibitor AZD6738 enhances the antitumor activity of radiotherapy and immune checkpoint inhibitors by potentiating the tumor immune microenvironment in hepatocellular carcinoma. J Immunother Cancer 2020;8:e000340. [Crossref] [PubMed]
- Sun R, Limkin EJ, Vakalopoulou M, et al. A radiomics approach to assess tumour-infiltrating CD8 cells and response to anti-PD-1 or anti-PD-L1 immunotherapy: an imaging biomarker, retrospective multicohort study. Lancet Oncol 2018;19:1180-91. [Crossref] [PubMed]
- Ruffin AT, Cillo AR, Tabib T, et al. B cell signatures and tertiary lymphoid structures contribute to outcome in head and neck squamous cell carcinoma. Nat Commun 2021;12:3349. [Crossref] [PubMed]
- Lei Y, Xie Y, Tan YS, et al. Telltale tumor infiltrating lymphocytes (TIL) in oral, head & neck cancer. Oral Oncol 2016;61:159-65. [Crossref] [PubMed]
- Borsetto D, Tomasoni M, Payne K, et al. Prognostic Significance of CD4+ and CD8+ Tumor-Infiltrating Lymphocytes in Head and Neck Squamous Cell Carcinoma: A Meta-Analysis. Cancers (Basel) 2021;13:781. [Crossref] [PubMed]
- Reddy SM, Reuben A, Barua S, et al. Poor Response to Neoadjuvant Chemotherapy Correlates with Mast Cell Infiltration in Inflammatory Breast Cancer. Cancer Immunol Res 2019;7:1025-35. [Crossref] [PubMed]
- Sammarco G, Varricchi G, Ferraro V, et al. Mast Cells, Angiogenesis and Lymphangiogenesis in Human Gastric Cancer. Int J Mol Sci 2019;20:2106. [Crossref] [PubMed]
- Eissmann MF, Dijkstra C, Jarnicki A, et al. IL-33-mediated mast cell activation promotes gastric cancer through macrophage mobilization. Nat Commun 2019;10:2735. [Crossref] [PubMed]
- Liu J, Chen H, Qiao G, et al. PLEK2 and IFI6, representing mesenchymal and immune-suppressive microenvironment, predicts resistance to neoadjuvant immunotherapy in esophageal squamous cell carcinoma. Cancer Immunol Immunother 2023;72:881-93. [Crossref] [PubMed]
- Devlin MJ, Miller R, Laforets F, et al. The Tumor Microenvironment of Clear-Cell Ovarian Cancer. Cancer Immunol Res 2022;10:1326-39. [Crossref] [PubMed]
- Solimando AG, Annese T, Tamma R, et al. New Insights into Diffuse Large B-Cell Lymphoma Pathobiology. Cancers (Basel) 2020;12:1869. [Crossref] [PubMed]
- Ribatti D, Annese T, Tamma R. Controversial role of mast cells in breast cancer tumor progression and angiogenesis. Clin Breast Cancer 2021;21:486-91. [Crossref] [PubMed]
- Ullah E, Nagi AH, Lail RA. Angiogenesis and mast cell density in invasive pulmonary adenocarcinoma. J Cancer Res Ther 2012;8:537-41. [Crossref] [PubMed]
- Öhrvik H, Grujic M, Waern I, et al. Mast cells promote melanoma colonization of lungs. Oncotarget 2016;7:68990-9001. [Crossref] [PubMed]
- Oldford SA, Marshall JS. Mast cells as targets for immunotherapy of solid tumors. Mol Immunol 2015;63:113-24. [Crossref] [PubMed]
- Aponte-López A, Fuentes-Pananá EM, Cortes-Muñoz D, et al. Mast Cell, the Neglected Member of the Tumor Microenvironment: Role in Breast Cancer. J Immunol Res 2018;2018:2584243. [Crossref] [PubMed]
- Cerwenka A, Lanier LL. Natural killers join the fight against cancer. Science 2018;359:1460-1. [Crossref] [PubMed]
- Assmann N, O'Brien KL, Donnelly RP, et al. Srebp-controlled glucose metabolism is essential for NK cell functional responses. Nat Immunol 2017;18:1197-206. [Crossref] [PubMed]
- Wang X, Lee DA, Wang Y, et al. Membrane-bound interleukin-21 and CD137 ligand induce functional human natural killer cells from peripheral blood mononuclear cells through STAT-3 activation. Clin Exp Immunol 2013;172:104-12. [Crossref] [PubMed]
- Cederberg RA, Franks SE, Wadsworth BJ, et al. Eosinophils Decrease Pulmonary Metastatic Mammary Tumor Growth. Front Oncol 2022;12:841921. [Crossref] [PubMed]
- Poncin A, Onesti CE, Josse C, et al. Immunity and Breast Cancer: Focus on Eosinophils. Biomedicines 2021;9:1087. [Crossref] [PubMed]
- Moreira A, Leisgang W, Schuler G, et al. Eosinophilic count as a biomarker for prognosis of melanoma patients and its importance in the response to immunotherapy. Immunotherapy 2017;9:115-21. [Crossref] [PubMed]
- Reichman H, Itan M, Rozenberg P, et al. Activated Eosinophils Exert Antitumorigenic Activities in Colorectal Cancer. Cancer Immunol Res 2019;7:388-400. [Crossref] [PubMed]
- Tanizaki J, Haratani K, Hayashi H, et al. Peripheral Blood Biomarkers Associated with Clinical Outcome in Non-Small Cell Lung Cancer Patients Treated with Nivolumab. J Thorac Oncol 2018;13:97-105. [Crossref] [PubMed]
- Mascitti M, Togni L, Rubini C, et al. Tumour-associated tissue eosinophilia (TATE) in oral squamous cell carcinoma: a comprehensive review. Histol Histopathol 2021;36:113-22. [PubMed]
- Xie F, Liu LB, Shang WQ, et al. The infiltration and functional regulation of eosinophils induced by TSLP promote the proliferation of cervical cancer cell. Cancer Lett 2015;364:106-17. [Crossref] [PubMed]
- McBride MA, Patil TK, Bohannon JK, et al. Immune Checkpoints: Novel Therapeutic Targets to Attenuate Sepsis-Induced Immunosuppression. Front Immunol 2021;11:624272. [Crossref] [PubMed]
- Samstein RM, Lee CH, Shoushtari AN, et al. Tumor mutational load predicts survival after immunotherapy across multiple cancer types. Nat Genet 2019;51:202-6. [Crossref] [PubMed]
- Wilkie MD, Lau AS, Vlatkovic N, et al. Metabolic signature of squamous cell carcinoma of the head and neck: Consequences of TP53 mutation and therapeutic perspectives. Oral Oncol 2018;83:1-10. [Crossref] [PubMed]
- Jiang AM, Ren MD, Liu N, et al. Tumor Mutation Burden, Immune Cell Infiltration, and Construction of Immune-Related Genes Prognostic Model in Head and Neck Cancer. Int J Med Sci 2021;18:226-38. [Crossref] [PubMed]
- Deneka AY, Baca Y, Serebriiskii IG, et al. Association of TP53 and CDKN2A Mutation Profile with Tumor Mutation Burden in Head and Neck Cancer. Clin Cancer Res 2022;28:1925-37. [Crossref] [PubMed]
- Szlasa W, Janicka N, Sauer N, et al. Chemotherapy and Physical Therapeutics Modulate Antigens on Cancer Cells. Front Immunol 2022;13:889950. [Crossref] [PubMed]