Identification of C4BPA as biomarker associated with immune infiltration and prognosis in breast cancer
Highlight box
Key findings
• C4BPA expression is significantly decreased in breast cancer (BC) tissues compared to normal breast tissues (P<0.05).
• C4BPA has a notable prognostic and diagnostic capacity for BC.
• Low C4BPA expression is associated with poor progression-free interval and overall survival, particularly in patients with Luminal A subtype.
• C4BPA expression correlates with the functionality of immune infiltrating cells, indicating its potential role in the tumor microenvironment.
What is known and what is new?
• Previous research has highlighted C4BPA as a potential biomarker for certain cancers, and this study further establishes its significance in BC prognosis and diagnostics.
What is the implication, and what should change now?
• These findings emphasize the potential of C4BPA as a diagnostic biomarker for BC, with implications for therapeutic strategies and prognostic assessments in clinical settings.
Introduction
Breast cancer (BC) is one of the most common malignancies in women. Globally, approximately 1.3 million people are diagnosed with BC (1,2), and 600,000 die of BC every year (3). At present, the incidence and mortality rates of BC in China have gradually increased, suggesting that the disease burden of BC in Chinese women is becoming increasingly severe (4). Despite recent advances in diagnosis and therapy, patients with recurrent and metastatic BC have poor survival (5). Thus, it is of urgent importance to discover sensitive biomarkers and innovative therapeutics to improve BC’s clinical diagnosis and treatment.
Numerous molecular indicators, including DNA mutations, abnormal messenger ribonucleic acid (mRNA) expression, and microRNAs, have been associated with the occurrence and progression of BC. C4BPA, also known as Complement Component 4 Binding Protein Alpha, Proline-Rich Protein, is a novel biomarker for multiple types of cancers (6). For example, C4BP has been linked to aggressive tumor characteristics and a poor prognosis in individuals with non-small cell lung cancer stage IIb and IIIa (7). Furthermore, the present study endeavors to elucidate the potential of C4BPA as an autophagy-immune-derived biomarker for prognostication and therapeutic responsiveness among patients diagnosed with lung adenocarcinoma (8). Additionally, C4BPA has been identified as a promising serum biomarker for the early detection of pancreatic ductal adenocarcinoma (PDAC) and the prediction of lymph node metastasis in PDAC cases (9,10). Reports have indicated that C4BPA harbors considerable biomarker potential in the context of patient outcomes in myeloma and suggests its prospective utility as a molecular signature in hepatocellular carcinoma (11,12). Besides, fully-silylated C4BPA, A2160, is a potentially novel serum biomarker for ovarian cancer (13). Furthermore, C4BPA has been identified in tissue and blood samples taken from BC patients (14). Yet, the precise functions of C4BPA in BC are still unknown.
In this research endeavor, we explored the correlation between C4BPA and BC as well as the prognostic value of C4BPA in BC from The Cancer Genome Atlas (TCGA) database. Furthermore, we performed gene set enrichment analysis (GSEA) function and pathway analysis to learn more about the molecular mechanism of C4BPA in BC pathogenesis. Additionally, the putative mechanism by which C4BPA modifies the occurrence and progression of BC was also thoroughly studied and addressed by investigating the connection between immune infiltration and C4BPA expression. Finally, we looked into the relationship between the clinical pathological characteristics of BC and the degree of C4BPA expression. We present this article in accordance with the TRIPOD reporting checklist (available at https://tcr.amegroups.com/article/view/10.21037/tcr-23-1215/rc).
Methods
RNA-sequencing (RNA-seq) data and bioinformatics analysis
TCGA (https://cancergenome.nih.gov/), a free public platform, is a source of abundant cancer-related dataset. The TCGA database was used to download the gene expression data (HTseq-Counts) and associated clinical data for BC for this analysis; the criteria for exclusion were normal BC samples and overall survival (OS) times of <1 month. The level 3 HTSeq-Counts data of 1,065 BC patients, the clinic data, and the RNA-seq gene expression were retained and subsequently investigated. Differential expression analysis and pan-cancer analysis data containing RNA-seq data in transcripts per million (TPM) format in TCGA and GTEx (https://www.gtexportal.org/) were uniformly processed by the Toil process downloaded from University of California Santa Cruz (UCSC) XENA (https://xenabrowser.net/datapages/) (15). The study was conducted in accordance with the Declaration of Helsinki (as revised in 2013).
RNA-seq data preprocessing and differentially expressed genes (DEGs) analysis
The gene expression levels of C4BPA in TCGA breast invasive carcinoma (BRCA/BIC) were divided into high expression and low expression groups based on the median value. Then, the unpaired Wilcoxon rank-sum test within the DESeq2 (3.8) software and R were used to compare expression profiles (HTSeq-Counts) between high and low C4BPA expression groups in order to evaluate DEGs and assess the prognostic value of various C4BPA expression levels (16). As threshold values for the DEGs, |log2-Fold Change (FC)| >1.5 and adjusted P value <0.05 were taken into consideration.
Kyoto Encyclopedia of Genes and Genomes (KEGG) and Gene Ontology (GO) enrichment analyses of DEGs
R package cluster Profiler was used to conduct pathway enrichment analysis of DEGs of BC significantly associated with C4BPA alterations (17). The GO terms for molecular functions (MFs), biological processes (BPs), and cellular components (CCs), as well as KEGG pathways, were enriched. Significant statistical differences were defined as P<0.01, the count ≥3, and the enrichment factor >1.5.
GSEA
The fundamental mechanisms of C4BPA in BC were also investigated by GSEA (18) [PMID: 16199517], performed by R package cluster Profiler (17) [PMID: 22455463]. This is a computational method for determining if a priori-defined list of genes displays statistically meaningful concordant variations between groups with high and low C4BPA expression. This study employed a permutation test with 1000 repetitions to identify the significantly altered pathways. The level of C4BPA expression was used as a phenotypic label. The analysis included gene sets from molecular signatures database (MSigDB) pathways. The pathways that were greatly enhanced for each phenotype were categorized using the adjusted P value (<0.1), false discovery rate (FDR) q-value (<0.25) and normalized enrichment score (NES; |NES| >1).
The correlation between C4BPA gene expression and immune infiltration
The single-sample GSEA (ssGSEA) method (an extension of GSEA) was used to analyze the immune infiltration of BC using the gene set variation analysis (GSVA) package in R (3.6.3) for 24 different types of immune cells in tumor samples. Genes predicting the number of 24 tumor-infiltrating immune cells in different tissue samples are part of the immune cell signature [PMID: 24138885] (19). The Wilcoxon rank sum test was used to compare the immune cell infiltration in the high-expression and low-expression groups of C4BPA. And the relationship between C4BPA and these immune cells was investigated by Spearman’s correlation method.
Protein-protein interaction (PPI) network construction and molecular analysis
The creation of PPI networks was accomplished through the successive utilization of a sophisticated Search Tool for the Retrieval of Interacting Genes (20). Cytoscape software (version 3.8.0) was used in this work to view and analyze the data from the STRING database (https://cn.string-db.org/). The DEGs with a confidence score >0.4 were marked as significant.
Prognostic model generation and prediction
The ideal model was selected by utilizing a multivariate Cox regression analysis and the Akaike information criterion (AIC) approach. Subsequently, a nomogram was created with the rms package to assess the prognosis of BC patients, followed by a calibration plot to evaluate its predictive accuracy. The risk score for each patient was determined by summing the scores of individual parameters, which were obtained by multiplying the parameter value by its coefficient. Next, the TCGA BC cohort was segregated into two distinct groups, namely high-risk and low-risk, based on the median risk score value. The disparity in the OS between these groups was determined using the Kaplan-Meier method and analyzed via a two-sided log-rank test utilizing the survminer package. The predictive performance of the prognostic model was assessed by constructing a receiver operating characteristic (ROC) curve. Additionally, prognostic information comes from a Cell paper (21). This study set the statistical significance level for all two-tailed tests at 0.05.
Statistical analysis
R was used to conduct all statistical analyses (v.3.6.2). Wilcoxon rank-sum tests were utilized to compare the expression of C4BPA between the BC and normal groups, while Wilcoxon signed-rank tests were used to compare expression in adjacent groups. Clinical pathologic features and C4BPA were analyzed using the Wilcoxon signed-rank test and logistic regression. The Cox regression and the Kaplan-Meier method were used to evaluate clinicopathologic characteristics associated with OS in TCGA patients. Furthermore, multivariate Cox analysis was utilized to compare the influence of C4BPA expression on survival and other clinical features [including T stage, N stage, M stage, pathologic stage, progesterone receptor (PR) status, and estrogen receptor (ER) status]. The cut-off value for C4BPA expression was determined by its median value, and a P value of less than 0.05 was considered meaningful.
Results
C4BPA gene expression levels in pan-cancer and BC and identification of DEGs
According to the screening criteria, 1,065 RNA-seq data with clinical information were available, including 110 cases of BC with paired normal samples. To investigate differences in C4BPA expression between various cancer types and normal tissues, we analyzed the TCGA and GTEx databases. Our findings revealed that C4BPA expression was significantly lower in multiple malignancies compared to normal tissues (P<0.05, Figure 1A). Next, our team conducted a Wilcoxon rank-sum test to assess the influence of C4BPA expression in patients with BC in the TCGA and GTEx/TCGA. C4BPA expression levels were found to be substantially lower in 1,065 tumor tissues than in 111 normal tissues (P<0.001; Figure 1B), and C4BPA expression levels in 110 tumor tissues were much lower than that in 110 matched normal breast tissues in the TCGA cohort (P<0.001; Figure 1C). Moreover, the GTEx/TCGA cohort findings were aligned with those from the TCGA cohort, showing that C4BPA expression levels in tumor tissues were lower than that in normal tissue (P<0.001; Figure 1D). Additionally, we employed the ROC curve to assess the ability of C4BPA expression level to discriminate BC tumors from non-tumor tissues. The analysis revealed that C4BPA exhibited a remarkable area under the curve (AUC) value of 0.870, indicating its potential as a reliable biomarker for distinguishing BC tissues from non-tumor tissues (Figure 1E).
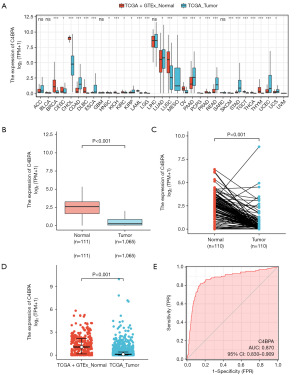
In addition, by dividing the expression levels of C4BPA in BRCA from TCGA into high-expression and low-expression groups based on the median value, and conducting differential gene expression analysis with the criteria of logFC >1.5 and adj.P <0.05, we identified a total of 335 DEGs, of which 235 were upregulated and 100 were downregulated (Figure S1A). Furthermore, in TCGA BRCA, when C4BPA was divided into high expression and low expression groups, the co-expression differential gene pattern is shown in Figure S1B: we selected the top 10 differential genes with the lowest and highest P values.
Gene function annotation and analysis of DEGs
We carried out an extensive gene function annotation using KEGG and GO with the aim of getting comprehensive information on gene function. Our analysis revealed significant findings, with 57 GO terms associated with BPs, three GO terms associated with CCs, and three GO terms associated with MFs (Figure 2A). The top three GO terms of each part are presented in Table S1. The BP findings showed that DEGs were primarily enriched in signal release, positive regulation of cell secretion, and positive regulation of secretion; the CC results revealed that DEGs were primarily enriched in multivesicular body, cornified envelope, and lamellar body; and the MF results indicated that DEGs were predominantly enriched in receptor-ligand activity, syntaxin-1 binding, and hormone activity. KEGG signaling pathway analysis identified the top five pathways, which included drug metabolism-cytochrome P450, metabolism of xenobiotics by cytochrome P450, chemical carcinogenesis, retinol metabolism, and complement and coagulation cascades (Figure 2B; Table S2).
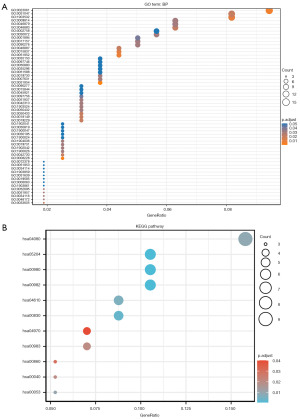
To further study the biological functions of C4BPA, we conducted GSEA and discovered that low expression of C4BPA was notably linked with reactome acetylcholine neurotransmitter release cycle, BC, BC relapse signaling pathways (Figure 3A-3D; Table S3).
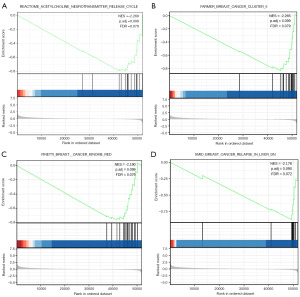
Correlation analysis of C4BPA gene and immune cell infiltration
To explore the relationship between C4BPA expression in BC and the abundance of immune infiltrates, we extracted 24 marker genes of immune cells from literature in Immunity [PMID: 24138885] (19). The infiltration of these 24 immune cells in BC was analyzed by ssGSEA method, and the difference in the infiltration level of these 24 immune cells between the high and low C4BPA expression groups was analyzed by Wilcoxon rank sum test. In addition, we utilized the Wilcoxon rank-sum test to conduct an in-depth examination of the relationship between the expression of C4BPA and immune infiltration. Our findings indicate a significant association between the C4BPA signature and immune infiltration in cases of BC. Notably, C4BPA demonstrated a positive correlation with various immune cell types, notably B cells (P<0.001), neutrophils (P<0.001), plasmacytoid dendritic cells (pDCs) (P<0.001), CD8 T cells (P<0.001), and T cells (P<0.001) (Figures 4,5A-5E). Intriguingly, an unexpected inverse relationship was observed between C4BPA expression and Th2 cells (P<0.001) (Figure 5F). Moreover, we employed the Spearman correlation method to further analyze the association between C4BPA expression and immune infiltration. Our results revealed that immune cell infiltration in BC was connected to the C4BPA signature. C4BPA was positively linked with immune cell types, the top five immune cell type were B cells (R=0.280, P<0.001), neutrophils (R=0.236, P<0.001), pDCs (R=0.236, P<0.001), CD8 T cells (R=0.231, P<0.001) and T cells (R=0.223, P<0.001) (Figure 6A-6E). Unexpectedly, our results also showed an inverse correlation between C4BPA expression and Th2 cells (R=−0.183, P<0.001) (Figure 6F).
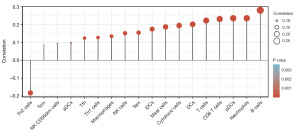
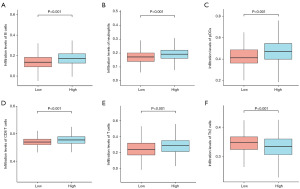
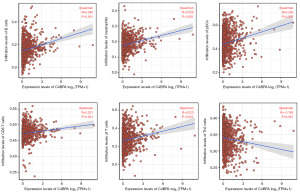
PPI network analysis
The PPI network was established using PPI pairs derived from the STRING database. Utilizing a minimum required interaction score of >0.4, we identified 208 edges and 164 nodes within the PPI network (Figure 7A). In addition, based on the STRING library, we obtained molecules directly associated with C4BPA (Figure 7B): CD40, NFKBIA, RELA, NFKB1, C4BPB, and CFI.
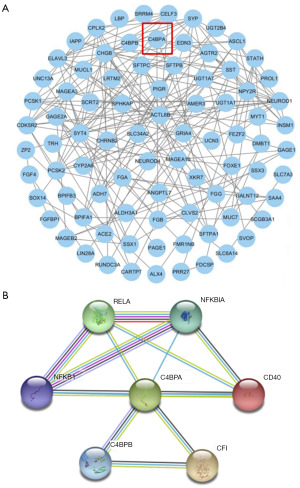
Assessment of the prognostic value of C4BPA gene
The Kaplan-Meier analysis demonstrated that patients with low C4BPA expression had a significantly shorter progression-free interval (PFI) [hazard ratio (HR) =0.61; 95% confidence interval (CI): 0.43–0.85; P=0.003] and OS (HR =0.61; 95% CI: 0.44–0.85; P=0.004) compared to those with high C4BPA expression (Figure 8A,8B). The Cox univariate and multivariate analyses of PFI in BC patients are presented in Table 1. In the univariate analysis, several variables including T stage, N stage, M stage, pathologic stage, PR status, ER status, and C4BPA expression were found to have a significant association with PFI, with P values <0.01. Multivariate analysis revealed that M stage (P<0.001), pathologic stage (P=0.012), and C4BPA expression (P=0.003) were autonomous prognostic factors for PFI in BC patients.
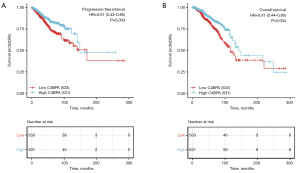
Table 1
Characteristics | Total, N | Univariate analysis | Multivariate analysis | |||
---|---|---|---|---|---|---|
HR (95% CI) | P value | HR (95% CI) | P value | |||
T stage (T1&T2 vs. T3&T4) | 1,061 | 0.472 (0.327–0.682) | <0.001 | 0.955 (0.554–1.644) | 0.867 | |
N stage (N1&N2&N3 vs. N0) | 1,045 | 2.250 (1.559–3.245) | <0.001 | 1.273 (0.786–2.062) | 0.326 | |
M stage (M1 vs. M0) | 909 | 8.288 (4.812–14.275) | <0.001 | 3.947 (1.969–7.913) | <0.001 | |
Pathologic stage (stage III&stage IV vs. stage I&stage II) | 1,041 | 2.962 (2.102–4.173) | <0.001 | 2.121 (1.176–3.826) | 0.012 | |
PR status (positive vs. negative) | 1,011 | 0.567 (0.405–0.795) | <0.001 | 0.593 (0.342–1.026) | 0.062 | |
ER status (positive vs. negative) | 1,014 | 0.599 (0.419–0.857) | 0.005 | 0.656 (0.369–1.168) | 0.152 | |
HER2 status (positive vs. negative) | 705 | 1.221 (0.707–2.109) | 0.475 | – | – | |
Race (White vs. Asian&Black or African American) | 975 | 0.848 (0.572–1.256) | 0.410 | – | – | |
Histological type (infiltrating ductal carcinoma vs. infiltrating lobular carcinoma) | 959 | 1.236 (0.772–1.979) | 0.379 | – | – | |
Anatomic neoplasm subdivisions (right vs. left) | 1,064 | 0.850 (0.610–1.184) | 0.337 | – | – | |
TP53 status (Mut vs. WT) | 955 | 1.258 (0.879–1.802) | 0.210 | – | – | |
PIK3CA status (Mut vs. WT) | 955 | 0.838 (0.565–1.245) | 0.382 | – | – | |
C4BPA (high vs. low) | 1,064 | 0.607 (0.434–0.848) | 0.003 | 0.562 (0.382–0.827) | 0.003 |
PFI, progression-free interval; HR, hazard ratio; CI, confidence interval; PR, progesterone receptor; ER, estrogen receptor; HER2, human epidermal growth factor receptor 2; Mut, mutation; WT, wild type.
Moreover, univariate Cox proportional hazards analyses showed that C4BPA expression (P=0.004), T stage (P=0.007), N stage (P<0.001), M stage (P<0.001) and pathologic stage (P<0.001) were independent prognostic factors for OS in BC patients. Multifactorial Cox regression further indicated that C4BPA (P=0.013), M stage (P=0.002), pathologic stage (P=0.041) and ER status (P=0.004) were independent prognostic factors in OS (P<0.05) (Table 2).
Table 2
Characteristics | Total, N | Univariate analysis | Multivariate analysis | |||
---|---|---|---|---|---|---|
HR (95% CI) | P value | HR (95% CI) | P value | |||
T stage (T1&T2 vs. T3&T4) | 1,061 | 0.598 (0.412–0.868) | 0.007 | 0.586 (0.305–1.127) | 0.109 | |
N stage (N1&N2&N3 vs. N0) | 1,045 | 2.145 (1.497–3.073) | <0.001 | 1.061 (0.576–1.957) | 0.849 | |
M stage (M1 vs. M0) | 909 | 4.327 (2.508–7.465) | <0.001 | 4.294 (1.718–10.733) | 0.002 | |
Pathologic stage (stage III&stage IV vs. stage I&stage II) | 1,041 | 2.519 (1.787–3.549) | <0.001 | 2.153 (1.034–4.483) | 0.041 | |
PR status (positive vs. negative) | 1,011 | 0.762 (0.541–1.074) | 0.120 | – | – | |
ER status (positive vs. negative) | 1,014 | 0.704 (0.487–1.017) | 0.062 | 0.471 (0.281–0.787) | 0.004 | |
HER2 status (positive vs. negative) | 705 | 1.611 (0.981–2.644) | 0.059 | 1.294 (0.752–2.226) | 0.353 | |
Race (White vs. Asian&Black or African American) | 975 | 0.880 (0.593–1.306) | 0.526 | – | – | |
Histological type (infiltrating ductal carcinoma vs. infiltrating lobular carcinoma) | 959 | 1.162 (0.738–1.830) | 0.516 | – | – | |
Anatomic neoplasm subdivisions (right vs. left) | 1,064 | 0.776 (0.559–1.077) | 0.130 | – | – | |
TP53 status (Mut vs. WT) | 955 | 1.218 (0.858–1.730) | 0.269 | – | – | |
PIK3CA status (Mut vs. WT) | 955 | 1.015 (0.696–1.479) | 0.938 | – | – | |
C4BPA (high vs. low) | 1064 | 0.614 (0.441–0.854) | 0.004 | 0.545 (0.337–0.882) | 0.013 |
OS, overall survival; HR, hazard ratio; CI, confidence interval; PR, progesterone receptor; ER, estrogen receptor; HER2, human epidermal growth factor receptor 2; Mut, mutation; WT, wild type.
In addition, the nomogram was constructed to evaluate the prognostic prediction model of C4BPA. The Concordance Index (C-index), which reflects the discrimination ability of the model, was used to assess the predictive accuracy of the nomograms. The C-indexes were 0.713 (0.684–0.741) and 0.712 (0.681–0.743) for PFI and OS, respectively. Both nomograms exhibited a high degree of concordance in the prediction value during validation (Figure 9A,9B). At the same time, we employed the calibration curve of the predictive model to validate the performance of Cox multi-factor model in predicting PFI and OS risks. The predictive model calibration curve exhibited the degree of concordance between the risk probabilities projected by the Cox multifactor model and the corresponding actual probabilities (Figure 10A,10B).
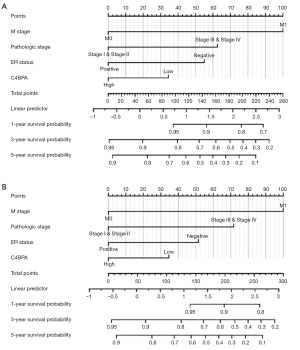
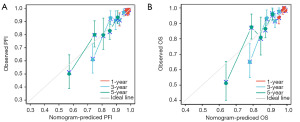
Correlation between C4BPA gene expression and clinicopathological characteristics in BC
We identified the potential clinical significance of C4BPA in BC. To achieve this goal, we separated patients into two groups (C4BPA low expression group and C4BPA high expression group) based on the relative expression levels of C4BPA in cancer/adjacent tissues, with the median value as the cut-off. The relationship between C4BPA expression and clinicopathological characteristics is analyzed and presented in Table 3. Low C4BPA expression was positively correlated with race (P<0.001), age (P<0.001), T stage (P=0.021), ER status (P<0.001), histological type (P<0.001), PR status (P=0.019) as well as PAM50 (P<0.001) (Figure S2A-S2G). However, the C4BPA was not significantly associated with the N stage, M stage, pathologic stage, PR status, human epidermal growth factor receptor 2 (HER2) status, anatomic neoplasm subdivisions, TP53 status, and PIK3CA status (all P>0.05).
Table 3
Characteristics | Level | Low expression of C4BPA | High expression of C4BPA | P value |
---|---|---|---|---|
T stage, n (%) | T1 | 123 (23.1) | 152 (28.6) | 0.010 |
T2 | 328 (61.5) | 287 (53.9) | ||
T3 | 58 (10.9) | 79 (14.9) | ||
T4 | 22 (4.1) | 13 (2.4) | ||
N stage, n (%) | N0 | 247 (47.5) | 260 (49.4) | 0.626 |
N1 | 183 (35.2) | 166 (31.6) | ||
N2 | 56 (10.8) | 60 (11.4) | ||
N3 | 34 (6.5) | 40 (7.6) | ||
M stage, n (%) | M0 | 450 (97.4) | 439 (98.2) | 0.546 |
M1 | 12 (2.6) | 8 (1.8) | ||
Pathologic stage, n (%) | Stage I | 84 (16.2) | 96 (18.3) | 0.481 |
Stage II | 309 (59.8) | 297 (56.6) | ||
Stage III | 113 (21.9) | 125 (23.8) | ||
Stage IV | 11 (2.1) | 7 (1.3) | ||
PR status, n (%) | Negative | 155 (31.2) | 183 (35.5) | 0.162 |
Positive | 342 (68.8) | 332 (64.5) | ||
ER status, n (%) | Negative | 96 (19.2) | 141 (27.3) | 0.003 |
Positive | 403 (80.8) | 375 (72.7) | ||
HER2 status, n (%) | Negative | 269 (74.7) | 279 (80.9) | 0.061 |
Positive | 91 (25.3) | 66 (19.1) | ||
PAM50, n (%) | Basal | 78 (14.6) | 112 (21.1) | <0.001 |
Her2 | 44 (8.3) | 38 (7.1) | ||
LumA | 247 (46.3) | 304 (57.1) | ||
LumB | 161 (30.2) | 41 (7.7) | ||
Normal | 3 (0.6) | 37 (7.0) | ||
Histological type, n (%) | Infiltrating ductal carcinoma | 402 (85.4) | 355 (72.7) | <0.001 |
Infiltrating lobular carcinoma | 69 (14.6) | 133 (27.3) | ||
Race, n (%) | Asian | 39 (8.4) | 21 (4.1) | <0.001 |
Black or African American | 65 (13.9) | 114 (22.4) | ||
White | 363 (77.7) | 374 (73.5) | ||
Anatomic neoplasm subdivisions, n (%) | Left | 267 (50.1) | 286 (53.8) | 0.256 |
Right | 266 (49.9) | 246 (46.2) | ||
TP53 status, n (%) | Mut | 173 (35.5) | 162 (34.5) | 0.802 |
WT | 314 (64.5) | 307 (65.5) | ||
PIK3CA status, n (%) | Mut | 151 (31.0) | 163 (34.8) | 0.244 |
WT | 336 (69.0) | 306 (65.2) | ||
Age (years), mean (SD) | – | 59.61 (13.87) | 57.10 (12.36) | 0.002 |
PR, progesterone receptor; ER, estrogen receptor; HER2, human epidermal growth factor receptor 2; Mut, mutation; WT, wild type; SD, standard deviation.
The logistic regression method was also employed to demonstrate the association between the clinicopathological features of BC and the degree of C4BPA expression. C4BPA was found to be substantially connected with ER status (P=0.002) and histological type (P<0.001) (Table 4).
Table 4
Characteristics | N | Odds ratio (95% CI) | P value |
---|---|---|---|
T stage (T1&T2 vs. T3&T4) | 1,062 | 1.18 (0.85–1.64) | 0.318 |
N stage (N1&N2&N3 vs. N0) | 1,046 | 0.93 (0.73–1.18) | 0.532 |
M stage (M1 vs. M0) | 909 | 0.68 (0.27–1.67) | 0.409 |
Pathologic stage (stage III&stage IV vs. stage I&stage II) | 1,042 | 1.06 (0.80–1.41) | 0.664 |
PR status (positive vs. negative) | 1,012 | 0.82 (0.63–1.07) | 0.143 |
ER status (positive vs. negative) | 1,015 | 0.63 (0.47–0.85) | 0.002 |
HER2 status (positive vs. negative) | 705 | 0.70 (0.49–1.00) | 0.050 |
Histological type (infiltrating ductal carcinoma vs. infiltrating lobular carcinoma) | 959 | 2.18 (1.58–3.03) | <0.001 |
TP53 status (Mut vs. WT) | 956 | 0.96 (0.73–1.25) | 0.750 |
PIK3CA status (Mut vs. WT) | 956 | 1.19 (0.90–1.55) | 0.217 |
CI, confidence interval; PR, progesterone receptor; ER, estrogen receptor; HER2, human epidermal growth factor receptor 2; Mut, mutation; WT, wild type.
Prognostic analysis of C4BPA gene in various subgroups of BIC
To more accurately predict the prognostic factors for survival of C4BPA in BIC, we performed a prognostic analysis of various subgroups of BRCA in TCGA BRCA. Figure 11A depicts the remarkable prognostic value of C4BPA in predicting PFI in multiple subgroups of BRCA in TCGA BIC; C4BPA was found in ER-positive (HR =0.448; 95% CI: 0.288–0.697; P<0.001), PR-positive (HR =0.465; 95% CI: 0.287–0.753; P=0.002), HER2-negative (HR =0.471; 95% CI: 0.268–0.825; P=0.009) and Luminal A (HR =0.578; 95% CI: 0.351–0.955; P=0.032) subgroups of the PAM50. Similarly, the remarkable prognostic value of C4BPA for OS in various subgroups of BRCA in TCGA BIC is shown in Figure 11B; C4BPA was found in ER-positive (HR =0.449; 95% CI: 0.291–0.691; P<0.001), PR positive (HR =0.411; 95% CI: 0.259–0.653; P<0.001), and Luminal A (HR =0.587; 95% CI: 0.355–0.972; P=0.038) and Luminal B (HR =0.214; 95% CI: 0.051–0.899; P=0.035) subgroups of the PAM50.
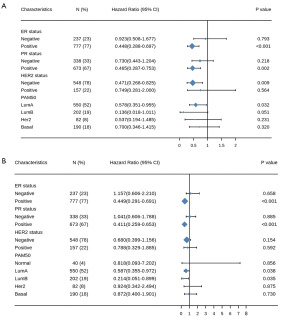
To further evaluate the prognostic significance of C4BPA for PFI and OS in each subgroup of BIC, we utilized the survminer software to construct Kaplan-Meier plots. The analysis revealed that BC patients with low expression of C4BPA had a shorter PFI in the ER-positive (HR =0.45; 95% CI: 0.29–0.70; P<0.001, Figure 12A), PR positive (HR =0.46; 95% CI: 0.29–0.75; P=0.002, Figure 12B), HER2-negative (HR =0.47; 95% CI: 0.27–0.83; P=0.009, Figure 12C), and Luminal A in PAM50 (HR =0.58; 95% CI: 0.35–0.95; P=0.032, Figure 12D) compared to the ones with high C4BPA expression. In addition, they had a worse OS in ER-positive (HR =0.45; 95% CI: 0.29–0.69; P<0.001, Figure 13A), PR positive (HR =0.61; 95% CI: 0.44–0.86; P=0.005, Figure 13B), Luminal A of PAM50 (HR =0.59; 95% CI: 0.36–0.97; P=0.038, Figure 13C), Luminal B of PAM50 (HR =0.21; 95% CI: 0.05–0.90; P=0.035, Figure 13D).
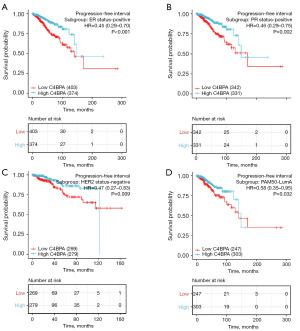
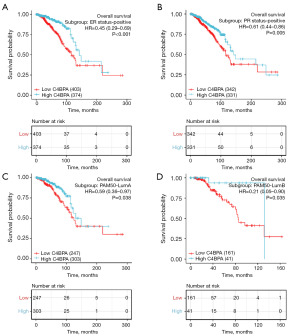
Discussion
C4BPA is thought to be a novel cancer biomarker. Its function has already been examined in pancreatic cancer, lung cancer, ovarian cancer, and so on. Yet, it is still unclear whether C4BPA could be used for effective early diagnosis and predicting prognosis in cancer patients. Herein, we explored the expression and prognostic significance of C4BPA in BC.
In this study, 335 DEGs (235 up-regulated and 100 down-regulated) were screened using the TCGA database and subjected to a co-differential gene analysis. The functional annotation and enrichment analyses of these DEGs revealed that most DEGs mainly affected the positive regulation of signal release and cellular secretion in the BPs and were mainly located in the intracellular part of the CCs. In addition, most DEGs primarily affected receptor ligand activity, syntaxin-1 binding activity, and hormone activity in the MFs. KEGG enrichment analysis revealed that a significant proportion of the DEGs were enriched in several pathways including drug metabolism-cytochrome P450, chemotaxis, retinol metabolism, and complement and coagulation cascades. Cytochrome P450 genes can induce various solid tumors, such as colon cancer, by activating PI3K/Akt (22-24). Recent studies have identified a possible relationship between polymorphic variants of certain carcinogen-metabolizing enzyme genes and tumor susceptibility (25). Previous studies have also shown that polymorphisms in cytochrome P450 may increase the risk of BC (26,27).
We performed a GSEA enrichment analysis on the DEGs identified in the C4BPA high/low expression group to learn more about the function of the C4BPA protein in BC. We also discovered that there was a notable correlation between low expression of C4BPA with the acetylcholine neurotransmitter release cycle, as well as BC and BC metastasis signaling pathways. These results suggest that C4BPA may represent a promising candidate for prognostic stratification and therapeutic intervention in BC.
The primary goal of this research was to determine how C4BPA expression and various immune infiltration levels in BC relate to one another. According to our findings, C4BPA was negatively connected with Th2 cells and favorably correlated with immune cell types like B cells, neutrophils, pDCs, CD8 T cells, and T cells. These correlations suggest that C4BPA may facilitate the function of B cells, neutrophils, pDCs, CD8 T cells, and T cells, thereby impeding the function of Th2 cells and exerting its inhibitory effect on BC. CD8 T cells are primarily cytotoxic T cells that can directly eliminate tumor cells and inhibit tumor growth through cell lysis and apoptosis, while Th2 cells play a dominant role in humoral immunity and promote tumor growth. In addition, research has shown that various malignant tumors, such as non-small cell lung cancer (28), BC (29), cervical cancer (30), bladder cancer (31), and lymphoma (32) have been found to be closely related to the drift from Th1 to Th2 cells; this drift becomes more pronounced as the malignancy of the tumor increases. Thus, low expression of C4BPA may promote the development of BC by inhibiting the function of B cells, neutrophils, pDCs, CD8 T cells, T cells, thereby promoting the function of Th2 cells.
In this study, we analyzed the interrelationships of C4BPA with other proteins by constructing a PPI network. We found a strong association between C4BPA and CD40, while C4BPA was part of the complement regulator C4BP. In particular, three NF-kB pathway members (RElA, NF-Kb1, and NF-KBIA) were estimated to be represented close to C4BPA at inter-action nodes. In addition, previous research using immunoprecipitation (IP) assays confirmed the connection between C4BPA and members of the NF-kB family, highlighting the critical role of C4BPA in the regulation of NF-kB-dependent apoptosis (33).
In this work, we also explored the relationship between clinicopathological characteristics, survival of BIC, and C4BPA expression in BIC. Firstly, we used bioinformatics methods, statistical methods, and relevant databases to evaluate C4BPA expression levels in BIC tissues and normal tissues. Our analysis revealed that C4BPA expression was substantially lower in BIC tissues compared to normal tissues. We also compared the expression levels of C4BPA in various human cancers and observed a downregulation of its expression in most cancers than in normal tissues, including BIC, which is consistent with the previous finding that C4BPA was of low expression in BC. Then, we used ROC curves to verify that C4BPA could be an ideal biomarker for differentiating BC from non-tumorous tissues. Next, by analyzing the correlation between C4BPA expression and the clinicopathological characteristics of BIC, we found that C4BPA expression was associated with T stage, ER status, PAM50, histological type, age, and race. The size and histological type of the malignant tumor are indicators of prognosis, which can be used to determine the recurrence and metastasis of the patient on a macroscopic level. Therefore, we further analyzed the relationship between the expression of C4BPA and PFI and OS by Kaplan-Meier survival curves. The survival curve demonstrated that low expression of C4BPA was associated with a shorter PFI and OS and was more likely to lead to tumor recurrence or distant metastases and a worse prognosis. Therefore, we set PFI and OS as our study endpoints. The Cox univariate results proved that the length of PFI was connected with the expression of C4BPA, T-stage, N-stage, M-stage, pathological stage, PR status, and ER status; the length of OS was associated with the expression of C4BPA, T-stage, N-stage, M-stage, and pathological stage.
Furthermore, the Cox multifactorial results demonstrated that the expression of C4BPA, M-stage, and pathological stage were associated with PFI, while the expression of C4BPA, M-stage, pathological stage, and ER status were associated with OS. Therefore, this suggests that C4BPA could be a unique prognostic factor influencing the long-term survival of patients with BIC. T-stage, N-stage, M-stage, TNM-stage, and ER status are recognized as prognostic factors. The correlation between the differential expression of C4BPA and PFI and OS suggested that C4BPA might be a new target for improving BIC prognosis. In addition, we evaluated the prognostic prediction model of C4BPA by constructing a nomogram that transformed the complex Cox multi-factor regression model into a visual graph. It made the results of the predicted model concrete and facilitated the assessment of patients. Thus, the nomogram improved the prognostic model for BC in general. At the same time, we used calibration curves to validate the PFI and OS risk prediction efficacy of the Cox multi-factor model and obtained a highly accurate prognostic model for BC.
BC is a heterogeneous tumor, and different subtypes of BC may have different gene expressions and biological behavior (34). In this study, the prognosis of BRCA subgroups in TCGA BIC was analyzed. In HER2-negative, Luminal A BC patients with low C4BPA expression had a shorter PFI compared to those with high C4BPA expression. In addition, in Luminal A and Luminal B BC patients with low C4BPA expression had worse OS compared to those with high C4BPA expression. Therefore, we can conclude that Luminal A BC patients with low C4BPA expression may have a worse prognosis compared to other BC subtypes.
This study has a few limitations. Experimental validation was not included in this study; we plan to further examine the expression of C4BPA in BC by quantitative polymerase chain reaction (qPCR) and immunohistochemistry and investigate the biological function mechanism of C4BPA in BC by employing cellular and animal experiments.
Conclusions
In conclusion, this study concluded that the expression of C4BPA was lower in BC tissues compared to normal breast tissues, which suggested that C4BPA has potential as a reliable biomarker for differentiating BC from non-tumor tissue. In addition, low expression of C4BPA may be linked to poor prognosis in BC, and it may be a promising prognostic biomarker in BC. Also, Luminal A BC patients with low C4BPA expression may have worse PFI and OS compared to patients with other BC subtypes.
Acknowledgments
Funding: This work was supported by
Footnote
Reporting Checklist: The authors have completed the TRIPOD reporting checklist. Available at https://tcr.amegroups.com/article/view/10.21037/tcr-23-1215/rc
Peer Review File: Available at https://tcr.amegroups.com/article/view/10.21037/tcr-23-1215/prf
Conflicts of Interest: All authors have completed the ICMJE uniform disclosure form (available at https://tcr.amegroups.com/article/view/10.21037/tcr-23-1215/coif). The authors have no conflicts of interest to declare.
Ethical Statement: The authors are accountable for all aspects of the work in ensuring that questions related to the accuracy or integrity of any part of the work are appropriately investigated and resolved. The study was conducted in accordance with the Declaration of Helsinki (as revised in 2013).
Open Access Statement: This is an Open Access article distributed in accordance with the Creative Commons Attribution-NonCommercial-NoDerivs 4.0 International License (CC BY-NC-ND 4.0), which permits the non-commercial replication and distribution of the article with the strict proviso that no changes or edits are made and the original work is properly cited (including links to both the formal publication through the relevant DOI and the license). See: https://creativecommons.org/licenses/by-nc-nd/4.0/.
References
- Harbeck N, Penault-Llorca F, Cortes J, et al. Breast cancer. Nat Rev Dis Primers 2019;5:66. [Crossref] [PubMed]
- Wang L, Li HP, Liu AN, et al. A Scoring System to Predict Arm Lymphedema Risk for Individual Chinese Breast Cancer Patients. Breast Care (Basel) 2016;11:52-6. [Crossref] [PubMed]
- Parsons J, Francavilla C. 'Omics Approaches to Explore the Breast Cancer Landscape. Front Cell Dev Biol 2020;7:395. [Crossref] [PubMed]
- Shi J, Liang D, Li D, et al. Epidemiological status of global female breast cancer. China Cancer 2017;26:683-90.
- Wang CCN, Li CY, Cai JH, et al. Identification of Prognostic Candidate Genes in Breast Cancer by Integrated Bioinformatic Analysis. J Clin Med 2019;8:1160. [Crossref] [PubMed]
- Sogawa K, Takano S, Iida F, et al. Identification of a novel serum biomarker for pancreatic cancer, C4b-binding protein α-chain (C4BPA) by quantitative proteomic analysis using tandem mass tags. Br J Cancer 2016;115:949-56. [Crossref] [PubMed]
- Liu YS, Luo XY, Li QR, et al. Shotgun and targeted proteomics reveal that pre-surgery serum levels of LRG1, SAA, and C4BP may refine prognosis of resected squamous cell lung cancer. J Mol Cell Biol 2012;4:344-7. [Crossref] [PubMed]
- Li Q, Xie D, Yao L, et al. Combining autophagy and immune characterizations to predict prognosis and therapeutic response in lung adenocarcinoma. Front Immunol 2022;13:944378. [Crossref] [PubMed]
- Sogawa K, Yamanaka S, Takano S, et al. Fucosylated C4b-binding protein α-chain, a novel serum biomarker that predicts lymph node metastasis in pancreatic ductal adenocarcinoma. Oncol Lett 2021;21:127. [Crossref] [PubMed]
- Sasaki K, Takano S, Tomizawa S, et al. C4b-binding protein α-chain enhances antitumor immunity by facilitating the accumulation of tumor-infiltrating lymphocytes in the tumor microenvironment in pancreatic cancer. J Exp Clin Cancer Res 2021;40:212. [Crossref] [PubMed]
- Ferreira BV, Carneiro EA, Pestana C, et al. Patient-Derived Extracellular Vesicles Proteins as New Biomarkers in Multiple Myeloma - A Real-World Study. Front Oncol 2022;12:860849. [Crossref] [PubMed]
- Dong W, Xia Z, Chai Z, et al. Proteomic analysis of small extracellular vesicles from the plasma of patients with hepatocellular carcinoma. World J Surg Oncol 2022;20:387. [Crossref] [PubMed]
- Mikami M, Tanabe K, Matsuo K, et al. Fully-sialylated alpha-chain of complement 4-binding protein: Diagnostic utility for ovarian clear cell carcinoma. Gynecol Oncol 2015;139:520-8. [Crossref] [PubMed]
- Suman S, Basak T, Gupta P, et al. Quantitative proteomics revealed novel proteins associated with molecular subtypes of breast cancer. J Proteomics 2016;148:183-93. [Crossref] [PubMed]
- Vivian J, Rao AA, Nothaft FA, et al. Toil enables reproducible, open source, big biomedical data analyses. Nat Biotechnol 2017;35:314-6. [Crossref] [PubMed]
- Love MI, Huber W, Anders S. Moderated estimation of fold change and dispersion for RNA-seq data with DESeq2. Genome Biol 2014;15:550. [Crossref] [PubMed]
- Yu G, Wang LG, Han Y, et al. clusterProfiler: an R package for comparing biological themes among gene clusters. OMICS 2012;16:284-7. [Crossref] [PubMed]
- Subramanian A, Tamayo P, Mootha VK, et al. Gene set enrichment analysis: a knowledge-based approach for interpreting genome-wide expression profiles. Proc Natl Acad Sci U S A 2005;102:15545-50. [Crossref] [PubMed]
- Bindea G, Mlecnik B, Tosolini M, et al. Spatiotemporal dynamics of intratumoral immune cells reveal the immune landscape in human cancer. Immunity 2013;39:782-95. [Crossref] [PubMed]
- Szklarczyk D, Gable AL, Lyon D, et al. STRING v11: protein-protein association networks with increased coverage, supporting functional discovery in genome-wide experimental datasets. Nucleic Acids Res 2019;47:D607-13. [Crossref] [PubMed]
- Liu J, Lichtenberg T, Hoadley KA, et al. An Integrated TCGA Pan-Cancer Clinical Data Resource to Drive High-Quality Survival Outcome Analytics. Cell 2018;173:400-416.e11. [Crossref] [PubMed]
- Scott KL, Chin L. Signaling from the Golgi: mechanisms and models for Golgi phosphoprotein 3-mediated oncogenesis. Clin Cancer Res 2010;16:2229-34. [Crossref] [PubMed]
- Farber-Katz SE, Dippold HC, Buschman MD, et al. DNA damage triggers Golgi dispersal via DNA-PK and GOLPH3. Cell 2014;156:413-27. [Crossref] [PubMed]
- Scott KL, Kabbarah O, Liang MC, et al. GOLPH3 modulates mTOR signalling and rapamycin sensitivity in cancer. Nature 2009;459:1085-90. [Crossref] [PubMed]
- Gao Y, Jiang D. Cytochrome P450 and tumor. China Oncology 2009;19:60-4.
- Zhang Y, Wise JP, Holford TR, et al. Serum polychlorinated biphenyls, cytochrome P-450 1A1 polymorphisms, and risk of breast cancer in Connecticut women. Am J Epidemiol 2004;160:1177-83. [Crossref] [PubMed]
- Mills PK, Yang R. Regression analysis of pesticide use and breast cancer incidence in California Latinas. J Environ Health 2006;68:15-22; quiz 43-4. [PubMed]
- Li J, Wang Z, Mao K, et al. Clinical significance of serum T helper 1/T helper 2 cytokine shift in patients with non-small cell lung cancer. Oncol Lett 2014;8:1682-6. [Crossref] [PubMed]
- Yu J, Du W, Yan F, et al. Myeloid-derived suppressor cells suppress antitumor immune responses through IDO expression and correlate with lymph node metastasis in patients with breast cancer. J Immunol 2013;190:3783-97. [Crossref] [PubMed]
- Li Z, Chuai J, Lin N, et al. Shift of Th1/Th2 Type Cytokine in Patients with Cervical Cell Carcinoma. Progress in Modern Biomedicine 2014;14:1357-60.
- Zheng K, Huang F, Lu L, et al. Antitumor effect of agonistic CD40 antibody via the regulation of Th1/Th2 balance in the tumor microenvironment. Chin J Clin Oncol 2018;45:163-6.
- Tosolini M, Kirilovsky A, Mlecnik B, et al. Clinical impact of different classes of infiltrating T cytotoxic and helper cells (Th1, th2, treg, th17) in patients with colorectal cancer. Cancer Res 2011;71:1263-71. [Crossref] [PubMed]
- Olcina MM, Kim RK, Balanis NG, et al. Intracellular C4BPA Levels Regulate NF-κB-Dependent Apoptosis. iScience 2020;23:101594. [Crossref] [PubMed]
- Cejalvo JM, Martínez de Dueñas E, Galván P, et al. Intrinsic Subtypes and Gene Expression Profiles in Primary and Metastatic Breast Cancer. Cancer Res 2017;77:2213-21. [Crossref] [PubMed]