Complement and coagulation cascades are associated with prognosis and the immune microenvironment of lower-grade glioma
Highlight box
Key findings
• This study is the first to elucidate the role of complement and coagulation cascades (CCC) genes in the prognosis, immune microenvironment, and chemotherapy efficacy of patients with lower-grade glioma (LGG).
What is known and what is new?
• Known: abnormal coagulation is a common feature of glioma.
• New: CCC are associated with the prognosis and immune infiltration of LGG. In this study, we report for the first time the association between TFPI and C2 and the prognosis of LGG.
What is the implication, and what should change now?
• Implication: the CCC gene-based risk signature could be used to predict the prognosis of LGG patients. Individualized treatment for LGG patients can be developed based on this signature.
Introduction
The 2021 5th edition of the World Health Organization (WHO) Classification of Tumors of the Central Nervous System reflects the discovery of genetic alterations underlying many brain tumors (1,2). Glioma is the most common brain tumor (3). With the publication of the 2021 5th edition of the WHO Classification of Tumors of the Central Nervous System, gliomas are reorganized into adult-type diffuse gliomas, pediatric-type diffuse low-grade and high-grade gliomas, circumscribed astrocytic gliomas, and ependymal tumors (4).
Low-grade glioma has long been referred to as grades I and II gliomas, whereas the term high-grade glioma encompassed grades III and IV tumors. The neuropathological dividing line between grades II and III gliomas is blurred, whereas there is a clear distinction between grades III and IV gliomas (5). For example, isocitrate dehydrogenase (IDH) mutations are characteristic of the vast majority of grades II and III gliomas but are significantly less common in grade IV glioma (6,7). As a result, researchers have progressively used lower-grade glioma (LGG) to refer to grades II and III gliomas (8,9). LGG has a high risk of malignantly transformation into glioblastoma (GBM) and early identification of transformation into GBM remains challenging (10). Several studies explored the relationship between magnetic resonance imaging and the malignant progression of LGG and found that changes in relative cerebral blood volume measured at longitudinal perfusion-weighted magnetic resonance imaging can predict the malignant transformation of LGG (11,12). Complete surgical resection is the currently main treatment for LGG, when feasible (9). Nevertheless, the characteristics of invasive growth and involving eloquent regions of LGG make it difficult to be complete surgical resected in some LGG patients (13,14). Due to the heterogeneity of LGG and the presence of the brain-blood barrier, treatments such as chemotherapy and immunotherapy are not satisfactory (15,16). Therefore, it is of great clinical importance to find new biomarkers and develop new therapeutic strategies for treating LGG (17,18).
There is reported substantial crosstalk between the complement system and coagulation cascades (19). The complement and coagulation cascades (CCC) could favor tumor development and progression by promoting the suppression of tumor immunity (20). Abnormal coagulation is a common feature of glioma (21). Cancer-associated thrombosis is significantly related to morbidity and mortality in patients with glioma (22). Activated complement signaling participates in various interactions between glioma cells and the tumor microenvironment (TME), promoting glioma growth (23). However, the role of CCC genes in LGG remains not systematically elucidated. Accordingly, we comprehensively analyzed the role of CCC genes in LGG based on RNA-sequencing (RNA-seq) and clinical data extracted from The Cancer Genome Atlas (TCGA) and Chinese Glioma Genome Atlas (CGGA) (24). The current study investigated the relationship between CCC genes and the prognosis, immune microenvironment, and chemotherapy efficacy of patients with LGG. We present this article in accordance with the TRIPOD reporting checklist (available at https://tcr.amegroups.com/article/view/10.21037/tcr-23-906/rc).
Methods
Data extraction
The study was conducted in accordance with the Declaration of Helsinki (as revised in 2013). Figure 1 shows the workflow chart for this study. A total of 769 patients with LGG were included in this study, 498 in TCGA, and 271 in CGGA. Among them, patients in TCGA were divided into the training set, and patients in CGGA were divided into the validation set. RNA-seq and clinical data of patients were collected from TCGA and CGGA. The clinical characteristics of patients in the study are summarized in Table 1, and there were no significant differences in the clinical characteristics except IDH mutation between the training and validation sets.
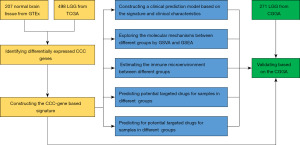
Table 1
Clinical characteristics | Classification | TCGA (n=498) | CGGA (n=271) | P value |
---|---|---|---|---|
Age, years | >40 | 255 | 130 | 0.287 |
≤40 | 243 | 140 | ||
NA | 0 | 1 | ||
Gender | Male | 277 | 151 | 0.979 |
Female | 221 | 120 | ||
Histologic grade | WHO II | 240 | 130 | 0.956 |
WHO III | 258 | 141 | ||
IDH mutation | Mutant | 399 | 176 | 0.008 |
Wildtype | 90 | 65 | ||
NA | 9 | 30 | ||
1p/19q Codel* | Codel | 161 | 81 | 0.795 |
Non-codel | 328 | 158 | ||
NA | 9 | 32 |
*, 1p/19q co-deletion status. TCGA, The Cancer Genome Atlas; CGGA, the Chinese Glioma Genome Atlas; P value, significant difference between the TCGA dataset and the CGGA dataset; WHO, World Health Organization; IDH, isocitrate dehydrogenase; NA, not available.
Identification of differentially expressed CCC genes
The CCC genes were obtained from the Molecular Signatures Database (25). RNA-seq data of 207 normal brain tissues from Genotype-Tissue Expression Project (GTEx) were collected from TCGA TARGET GTEx dataset in University of California Santa Cruz Xena database (26). Principal component analysis (PCA) was used to see if the discrimination between TCGA and GTEx was significant. R package “limma” was used to identify differentially expressed genes between TCGA and GTEx, and genes with logFC (Log2 foldchange) >1 and q<0.05 were considered as differentially expressed genes (27).
Construction of the risk signature and clinical prediction model
Univariate Cox regression, least absolute shrinkage and selection operator (LASSO) analysis (28), co-linearity analysis, and multivariate Cox regression were used to construct the risk signatures based on the identified differentially expressed CCC genes. The hazard ratio (HR) was used to assess the impact of risk factors on the prognosis of patients with LGG. The risk scores were calculated using the following algorithm: Risk Score = coefficient of Gene1 × expGene1 + coefficient of Gene2 × expGene2 + … + coefficient of Genen × expGenen; in which the coefficient was the regression coefficient of genes obtained using multivariate Cox regression analysis, and ‘exp’ was the expression of corresponding genes. To explore the relationship between the risk score and clinical characteristics of patients with LGG, a clinical prediction model was constructed based on the risk score and clinical characteristics. R package “regplot” (https://cran.r-project.org/web/packages/regplot/) were used to build and visualize the nomogram. The model’s predictive value was assessed by calibration curves, receiver operating characteristic (ROC) curves and the area under the curve (AUC).
Exploration of the molecular mechanism
The logFC of each gene between the high- and low-risk groups was calculated by R package “limma”. Gene set enrichment analysis (GSEA) and gene set variation analysis (GSVA) were used for enrichment analysis on the Kyoto Encyclopedia of Genes and Genomes (KEGG) pathways and Gene Ontology (GO) terms, the latter including biological process (BP), molecular function (MF), and cell component (CC) (29-32).
Estimation of the immune microenvironment and prediction of the immunotherapy sensitivity
The Estimation of Stromal and Immune cells in Malignant Tumor tissues using Expression (ESTIMATE) data analysis was conducted to calculate the tumor purity of each sample (33). CIBERSORT was conducted to estimate the infiltration of immunocytes of each sample (34). The tumor immune dysfunction and exclusion (TIDE) framework was used to estimate the efficacy of immune checkpoint (ICP) therapy of each patient by characterizing dysfunctional T cells and infiltrating cytotoxic T lymphocyte levels (35). To further determine the integrated relationship between risk score and ICP therapy’s efficacy, we plotted Kaplan-Meier (KM) curves for patients in different risk groups and different ICP expressions. RNA-seq data and corresponding clinical data of two immunotherapy cohorts, including GSE135222 (36) and GSE78220 (37), were collected to evaluate the predictive abilities of the efficacy of the immunotherapy. GSE135222 is an anti-programmed cell death 1 (PD-1)/programmed death-ligand 1 (PD-L1) cohort of patients with lung cancer. GSE78220 is a melanoma cohort where patients were treated with anti-PD-1 checkpoint inhibition therapy. Based on the response to ICP therapy, patients were categorized into two groups, stable disease or progressive disease (SD/PD) and complete response or partial response (CR/PR).
Prediction of chemotherapy drug efficacy
The sensitivity of each sample is predicted by R package ‘oncoPredict’ based on drug-sensitive data and expression data (38). The drug-sensitive data and expression data were obtained from the Genomics of Drug Sensitivity in Cancer database (the ‘GDSC2’ dataset, https://www.cancerrxgene.org/), the Cancer Therapeutics Response Portal (the ‘CTRP v2’ dataset, https://portals.broadinstitute.org/ctrp), and the Cancer Cell Line Encyclopedia (39-41). Sensitivity to drugs was assessed as sensitivity value, and lower sensitivity value suggested more sensitive to potential drugs. SwissTargetPrediction (42), SuperPred (43), and similarity ensemble approach (SEA) (44) are three dependable tools to predict the targets of small molecular drugs. After screening out the potential drugs, the possible interactions between CCC genes and the potential drugs were estimated utilizing these tools. The three-dimensional (3D) structures of the potential drugs were obtained from PubChem (45).
Cell culture
Given that there were no widely used LGG cell lines, and GBM and LGG belonged to gliomas, we used two GBM cells to perform the drug sensitivity experiments, which is an acceptable practice as another published study has used GBM cell lines in LGG-related study (46). The glioma cell lines including U251 and U87 purchased from the Cell Library of the Chinese Academy of Sciences (Shanghai, China) were cultured in complete medium [Dulbecco’s modified Eagle medium (DMEM; Servicebio, Wuhan, China) with 10% fetal bovine serum (FBS, Gibco, California, USA) and 10 µL/mL penicillin-streptomycin (Biosharp, Beijing, China)] at a humidified chamber at 37 ℃ with 5% CO2. For the drug sensitivity experiments, glioma cells were cultured in normal complete medium and complete medium containing 20 µM MG-132 (MedChemExpress, New Jersey, USA), respectively, to explore the impact of MG-132 on the malignant phenotype of glioma cells.
Cell Counting Kit-8 (CCK8) proliferation assay
CCK8 assay was conducted to evaluate cell proliferation ability according to the manufacturer’s protocol (Vazyme Biotech, Nanjing, China). Firstly, U251 and U87 cells were seeded in 96-well plates with three repetitions at a density of 5,000 cells/100 µL/well. Then, 10 µL of CCK8 solution was added to each well of the plates and incubated for 2 h at 37 ℃ at 0 and 24 h. Finally, the optical density (OD) value was measured at 450 nM by using a BioTek Synergy HT Microplate Reader (California, USA). Since the OD value was linearly related to the number of cells, we used the OD value to estimate the effect of MG-132 on the proliferation ability of glioma cells.
Colony formation assay
For colony formation assay, U251 and U87 cells were seeded in 6-well plates with three repetitions at a density of 500 cells/1 mL/well. After 14-day incubation, when the colonies could be visible to the naked eye, these plates were washed with phosphate-buffered saline (PBS, Servicebio) twice, fixed by 4% paraformaldehyde (Servicebio) for 30 min and stained with 0.1% crystal violet solution (Servicebio) for 15 min. Then the colony assay was photographed for further analysis.
Wound healing assay
Wound healing assay was performed to evaluate cell migration ability. U251 and U87 cells were seeded in 6-well plates with three repetitions at a density of 500,000 cells/1 mL/well. After cultured for 24 h, a 10-µL disposable pipette tip was run over the surface of the cells to cause a wound. The cells were washed three times with PBS and cultured with 3% FBS (low concentration) medium to reduce the interference of cell proliferation with results. The extent of wound healing was measured at 0 and 24 h, respectively using a microscope at 4× magnification. The area of the wound was measured using ImageJ (National Institutes of Health, Maryland, USA) (47). The wound healing percentage was calculated using the following formula: wound healing percentage = (Area0h − Area24h)/Area0h.
Statistical analysis
Statistical analyses and data visualizations were performed using R version 4.1.3. Spearman correlation analysis was used to evaluate the correlation between data. The Student’s t-test was used to compare the differences of continuous variables between the two normally distributed datasets. The Wilcoxon rank-sum test was used to compare the differences of continuous variables among non-normally distributed datasets. The Chi-square test was used to compare the differences between the two groups of discrete random variables. Kaplan-Meier analysis was used to compare the differences in survival between the two groups. Two-sided P≤0.05 was considered statistically significant and was stratified to P<0.01, P<0.001, and P<0.0001.
Results
Identification of differentially expressed CCC genes
PCA showed a clear separative trend between LGG and normal brain tissue from the “TCGA TARGET GTEx” dataset (PC1 + PC2 =76.3%>75%) (Figure 2A). A total of 5,628 differentially expressed genes were identified between LGG and normal brain tissue (Figure 2B). Among them, 20 CCC genes were highly expressed in LGG (Figure 2C). According to the results of univariate Cox regression, 12 CCC genes, including F2R, F5 (HR <1), CFI, SERPINE1, SERPINA1, PLAU, TFPI, C1QC, C1R, C2, C3AR1, C5AR1, were associated with the prognosis of patients with LGG in both TCGA (Figure 2D) and CGGA (Figure 2E).
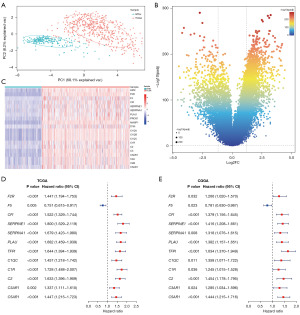
Construction of a six-CCC-gene signature related to the prognosis of patients with LGG
Six CCC genes were selected to construct the risk signature using LASSO and co-linearity analysis (Figure 3A,3B). Patients in the high-risk group had shorter overall survival (P<0.0001), faster arrival at censored, and worse prognosis in TCGA (Figure 3C,3D). These six CCC genes, including F2R, SERPINA1, TFPI, C1QC, C2, and C3AR1, were highly expressed in the high-risk group in TCGA (Figure 3E). The AUC of the risk signature in predicting one-, three-, and five-year survival of patients with LGG was 0.774, 0.695, and 0.628 in TCGA (Figure 3F). Similar to the TCGA dataset, patients in the high-risk group in CGGA had a shorter overall survival time (P<0.0001), high expression of six CCC genes in the signature, and censored status was enriched in the high-risk group (Figure 3G-3I). The AUC of the risk signature in predicting one-, three-, and five-year survival of patients with LGG was respectively 0.571, 0.607, and 0.647 (Figure 3J).
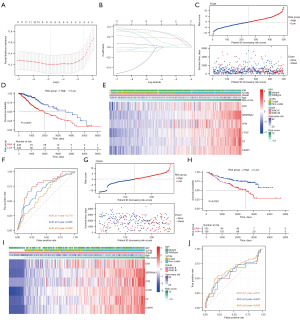
Construction of a clinical prediction model based on the risk signature and clinical characteristics
When it comes to several clinical biomarkers of glioma, the risk score was significantly elevated in patients WHO III (P<0.001), IDH wildtype (P<0.001), and 1p/19q non-codeleted (P<0.001) in TCGA (Figure 4A) and aged >40 years (P<0.01), and 1p/19q non-codeleted (P<0.001) in CGGA (Figure 4B). Based on these clinical characteristics and the risk score, a clinical prediction model was constructed with high accuracy in predicting patients’ three-year and five-year survival in TCGA (Figure 4C,4D). The AUC of the clinical prediction model in predicting one-, three-, and five-year survival of patients with LGG was 0.890, 0.892, and 0.823 in TCGA (Figure 4E). In CGGA, the clinical prediction model had high accuracy in predicting patients’ three-year and five-year survival as well (Figure 4F,4G), and the AUC of the clinical prediction model in predicting one-, three-, and five-year survival of patients with LGG was 0.831, 0.849, and 0.813 (Figure 4H).
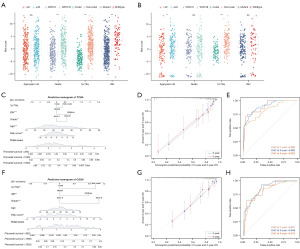
Exploration of molecular mechanisms underlying the risk signature
GSVA results showed the top 15 enriched KEGG pathways in high-risk and low-risk groups in TCGA (Figure 5A). The CCC pathway was activated in the high-risk group (Figure 5B). GSEA results indicated that the JAK-STAT signaling pathway, neutrophil extracellular trap formation, NOD-like receptor signaling pathway, Notch signaling pathway, PI3K-Akt signaling pathway, platelet activation, and Rap1 signaling pathway were enriched in the high-risk group in TCGA (Figure 5B). The top 15 enriched KEGG pathways in high-risk and low-risk groups in CGGA were similar to those in TCGA, with a high degree of overlap (Figure 5C). The CCC pathway, JAK-STAT signaling pathway, neutrophil extracellular trap formation, NOD-like receptor signaling pathway, Notch signaling pathway, PI3K-Akt signaling pathway, platelet activation, and Rap1 signaling pathway were enriched in the high-risk group in CGGA, as well (Figure 5D). By means of GSVA, the enriched GO terms in the high-risk group in TCGA were displayed, including upregulated and down-regulated (Figure 5E). GSEA results on GO terms showed that blood coagulation, complement activation, classical pathway, negative regulation of humoral immune response, negative regulation of natural killer (NK) cell-mediated immunity, positive regulation of macrophage activation, receptor signaling pathway via JAK-STAT, regulation of inflammatory response were enriched in the high-risk group in TCGA (Figure 5F). In CGGA, the results of GSVA and GSEA showed good agreement with those in TCGA (Figure 5G,5H).
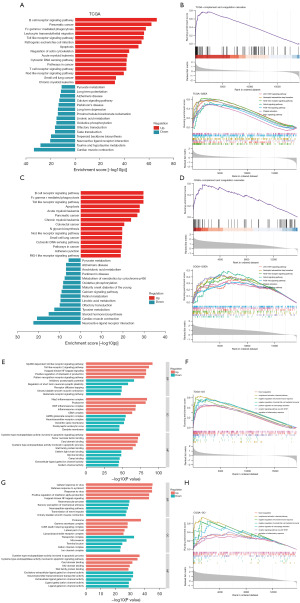
Different immune landscape in different risk groups
ESTIMATE results demonstrated a strong correlation between risk score and stromal score (R=0.77), immune score (R=0.86), ESTIMATE score (R=0.85), and tumor purity (R=−0.83) (Figure 6A). The CIBERSORT results in TCGA showed that macrophage M2 (R=0.31), monocyte (R=0.18), T cell CD4 memory activated (R=0.12), and macrophage M1 (R=0.11) were positively correlated with the risk score, while B cell plasma (R=−0.35), B cell naïve (R=−0.33), T cell follicular helper (R=−0.29), NK cell activated (R=−0.21), Mast cell activated (R=−0.16), Tregs (R=−0.14), T cell CD4 naïve (R=−0.14), and macrophage M0 (R=−0.09) were negatively correlated with risk score (Table 2, Figure 6B). In TCGA, macrophage M2 (P<0.001), monocyte (P<0.001), and myeloid dendritic cell activated (P<0.05) were highly infiltrated in the high-risk group, and B cell naïve (P<0.001), B cell plasma (P<0.001), Mast cell activated (P<0.001), neutrophil (P<0.05), NK cell activated (P<0.001), T cell CD4 naïve (P<0.01), T cell follicular helper (P<0.001), and Tregs (P<0.01) were highly infiltrated in the low-risk group (Figure 6C). Consistent with results on tests with TCGA datasets, there was a strong correlation among the risk score and stromal score (R=0.46), immune score (R=0.50), ESTIMATE score (R=0.51), and tumor purity (R=−0.47) in CGGA (Figure 6D). After comparing the CIBERSORT results in TCGA, we found that macrophage M2 (R=0.25, P<0.001) and monocyte (R=0.41, P<0.001) were positively correlated with the risk score and highly infiltrated in the high-risk group in CGGA (Table 2, Figure 6E,6F). Meanwhile, B cell plasma (R=−0.50, P<0.001), T cell follicular helper (R=−0.41, P<0.001), B cell naïve (R=−0.25, P<0.001), and NK cell activated (R=−0.15, P<0.01) were negatively correlated with the risk score and highly infiltrated in the low-risk group in CGGA.
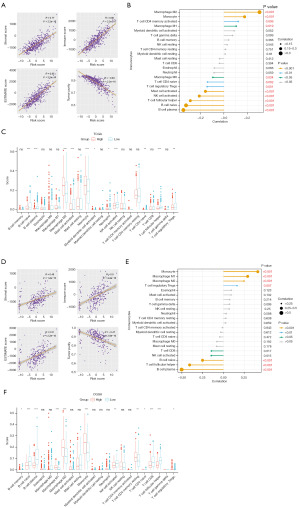
Table 2
Cell type | TCGA | CGGA | |||
---|---|---|---|---|---|
Correlation | P value | Correlation | P value | ||
B cell memory | 0.0405648 | 0.366346 | 0.0757 | 0.21416 | |
B cell naive | −0.325094 | 1.01E−13 | −0.25242 | 2.62E−05 | |
B cell plasma | −0.347916 | 1.29E−15 | −0.5042 | 7.01E−19 | |
Eosinophil | −0.082572 | 0.065594 | 0.093876 | 0.123157 | |
Macrophage M0 | −0.094908 | 0.034223 | −0.07951 | 0.191926 | |
Macrophage M1 | 0.1050114 | 0.019077 | 0.292333 | 9.70E−07 | |
Macrophage M2 | 0.3050242 | 3.49E−12 | 0.24784 | 3.70E−05 | |
Mast cell activated | −0.158601 | 0.000381 | 0.091634 | 0.132404 | |
Mast cell resting | −0.036861 | 0.411755 | −0.08207 | 0.177937 | |
Monocyte | 0.1769645 | 7.17E−05 | 0.414568 | 1.11E−12 | |
Myeloid dendritic cell activated | 0.0871031 | 0.052065 | 0.026895 | 0.659373 | |
Myeloid dendritic cell resting | 0.0108241 | 0.809594 | −0.03094 | 0.612098 | |
Neutrophil | −0.084768 | 0.058713 | 0.033011 | 0.588461 | |
NK cell activated | −0.211297 | 1.96E−06 | −0.14694 | 0.015485 | |
NK cell resting | 0.0272013 | 0.544774 | 0.070102 | 0.250101 | |
T cell CD4 memory activated | 0.1221341 | 0.006354 | −0.01208 | 0.843078 | |
T cell CD4 memory resting | 0.014237 | 0.751297 | 0.028692 | 0.638186 | |
T cell CD4 naive | −0.141191 | 0.001585 | −0.0439 | 0.471666 | |
T cell CD8 | −0.038265 | 0.394173 | −0.14532 | 0.016669 | |
T cell follicular helper | −0.288333 | 5.45E−11 | −0.4082 | 2.64E−12 | |
T cell gamma delta | 0.0739388 | 0.099328 | 0.073939 | 0.099328 | |
T cell regulatory Tregs | −0.144271 | 0.001245 | 0.163766 | 0.006898 |
TCGA, The Cancer Genome Atlas; CGGA, the Chinese Glioma Genome Atlas; NK cell, natural killer cell.
Association between risk score and the efficacy of immunotherapy
To explore the association between risk score and the efficacy of immunotherapy, we evaluated the differences in expression of ICPs in high- and low-risk groups and found several ICPs were highly expressed in the high-risk group in TCGA (Figure 7A). There was a positive correlation between the TIDE score and risk score in TCGA (Figure 7B). The prognosis of patients in different stratification based on risk score and TIDE score was significantly different (P<0.0001), suggesting that patients in the high-risk group may have greater resistance to immunotherapy (Figure 7C). Consistent with results on tests with TCGA datasets, these ICPs were highly expressed in the high-risk group in CGGA (Figure 7D). The TIDE score was positively correlated with the risk score (Figure 7E), and the higher the TIDE score and risk score, the worse the patient’s prognosis (Figure 7F). Moreover, we also explored the association between the risk score, ICPs (including CD28, CTLA4, PDCD1, RASA2) expression, and the patient’s prognosis in both TCGA and CGGA (Figure 7G,7H). The results indicated that patients with lower expression of ICPs and lower risk scores had a better prognosis (P<0.0001). Then we validated our findings in two public immunotherapy cohorts, GSE135222 and GSE78220. All patients were assigned a corresponding risk score based on the risk signature and classified as high- or low-risk patients. According to our findings, lung cancer patients with higher risk scores had worse prognoses (P=0.03) and less favorable responses to immunotherapy (P<0.0001) (Figure 7I). In melanoma patients, we found a similar trend, although not statistically significant (P=0.092) (Figure 7J). These results suggest that this risk signature is an effective predictor of patient response to immunotherapy, with high-risk patients responding more poorly to immunotherapy and low-risk patients benefiting more from immunotherapy.
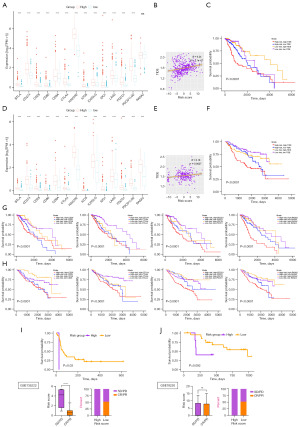
Prediction of potential drugs for high-risk patients
The flow chart for screening potential drugs is shown in Figure 8A. Eight overlapped potential drugs were identified using oncoPredict, including KU-55933, PLX-4720, AZD8055, AZD6482, NVP-ADW742, BMS-536924, BMS-345541, MG-132 (Figure 8B,8C). The correlation between risk scores and predicted sensitivities based on different datasets was not entirely consistent. Through the strategy of screening potential drugs, MG-132, BMS-536924, PLX-4720, and AZD6482 were identified as potential sensitive drugs for high-risk patients because the sensitivity values of these drugs were negatively correlated with the risk score and lower in the high-risk group, suggesting being more sensitive (Figure 8C-8E). Among them, MG-132 was particularly recommended for high-risk patients because of the lowest sensitivity values. Then we obtained the 3D structure of these sensitive drugs (Figure 8F). To explore the relationship between the drugs and CCC, we predicted possible interactive targets between these four drugs and the CCC genes (Figure 8G). The results showed that MG-132 had the most possible targets for CCC genes, indicating MG-132 might be able to exert anti-LGG activity by interacting with CCC.
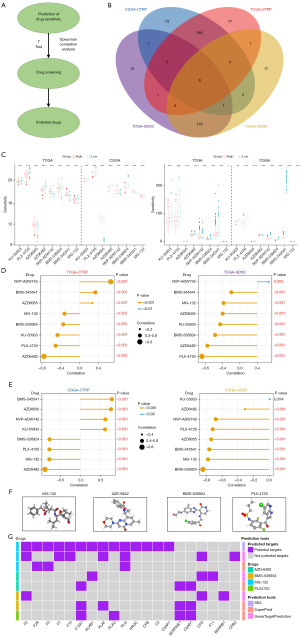
We performed in vitro experiments to explore the anti-glioma effect of the MG-132. The CCK8 assay and colony formation assay demonstrated that MG-132 could significantly suppress the proliferation of glioma cells (Figure 9A,9B). The results of the wound healing assay indicated that MG-132 could inhibit the migration of U251 (P<0.001) and U87 cells (P<0.0001) (Figure 9C). In summary, our findings reflected the accuracy of our drug prediction and suggested that MG-132 was a powerful anti-glioma drug.
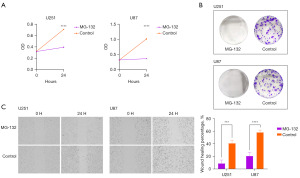
Discussion
Hypercoagulability and thrombotic complications are common in patients with glioma (48,49). Several previous studies have reported that activation of CCC may be associated with the development of glioma (50-52). However, the specific mechanism of how the CCC is involved in glioma development and the mechanism of coagulation in gliomas has not been enclosed yet. To the best of our knowledge, this study is the first to elucidate the role of CCC genes in the prognosis, immune microenvironment, and chemotherapy efficacy of patients with LGG. In the current study, we found that 20 CCC genes were highly expressed in LGG, of which 12 CCC genes were related to worse prognosis in patients with LGG. Then we constructed a risk signature based on six of those CCC genes and divided the patients into high- and low-risk groups. GSEA results demonstrated that CCC was activated in the high-risk group, suggesting that CCC might influence the prognosis of patients with LGG in some ways. Subsequently, through a comprehensive bioinformatics analysis, we found that CCC may affect the prognosis of LGG patients by influencing the immune microenvironment and treatment resistance of LGG.
A complete surgical resection is the optimal treatment of LGG, when safe (8). Gross total resection (GTR) had superior efficacy on survival, functional outcome, tumor progression, seizure control, malignant transformation, morbidity, and mortality in LGG patients (53). Compared with LGG patients who had a subtotal or partial resection, a higher proportion of LGG patients had a better prognosis following GTR (54). Although GTR, when feasible, is the most important treatment for LGG, the invasive growth and involving functional brain regions of LGG make complete surgical resection difficult. For LGG patients following incomplete resection, they could benefit from radiotherapy (55,56). When the effects of surgery and radiotherapy remain unsatisfactory, chemotherapies can be used as an adjunctive treatment (57). Moreover, targeted therapies might be effective in specific LGG patients. BRAF and MEK inhibitors are recommended for pilocytic astrocytomas, pleomorphic xanthoastrocytomas, and gangliogliomas when BRAF altered (58,59), and mTOR inhibitor everolimus is recommended for subependymal giant cells astrocytomas (60).
The constructed risk signature consisted of six CCC genes, including F2R, SERPINA1, TFPI, C1QC, C2, and C3AR1. Univariate Cox analysis showed that all of the six genes were risk factors for poor prognosis in glioma patients, and highly expressed in the high-risk group. Of these six genes, F2R (61), SERPINA1 (62), C1QC (63), and C3AR1 (64) have been reported to be associated with worse prognosis or the development of glioma. In this study, we reported for the first time the association between TFPI and C2 and the prognosis of LGG. Several studies have shown that TFPI is associated with metastasis of lung cancer and progression of breast cancer (65-68). Meanwhile, C2 is reported to be a prognostic factor for hepatocellular carcinoma and colorectal cancer (69,70). We then constructed a clinical prediction model based on this risk signature and clinical characteristics. This model could accurately predict the prognosis of patients with LGG and be further applied to the clinical settings.
To discover the possible mechanisms by which CCC affects the prognosis of patients with LGG, GSVA and GSEA were performed based on KEGG pathways and GO terms. In addition to CCC, the platelet activation pathway was also activated in the high-risk group, indicating a synergistic relationship between CCC and platelet activation in LGG. By comparing the results of GSEA and GSVA, we found that the JAK-STAT signaling pathway, NOD-like receptor signaling pathway, and Notch signaling pathway were activated in the high-risk group. Complement activation could interact with inflammasomes, which are comprised of upstream sensor proteins of the NOD-like receptor family (71,72). JAK-STAT and Notch signaling pathways were demonstrated to play an important role in coagulation (73,74). Consistent with findings from previous studies, which have reported the simultaneous upregulation or activation of these pathways, our study suggested that there may be crosstalk between CCC and these pathways, and proteins in JAK-STAT, NOD-like receptor, and Notch signaling pathways might involve in the CCC progression (75,76).
The current study demonstrated that CCC might be associated with the progression of LGG. The complement system has been proven to play a crucial role in the progression of LGG (77). A study found higher levels of C1Q expression were correlated with unfavorable prognosis in LGG (63). Other studies suggested that C3 and C1RL can serve as diagnostic biomarkers and potential targets of therapy for LGG patients (78,79). On the other hand, TF is related to epithelial-mesenchymal transition (EMT) and has the potential to become an effective target against EMT and thrombotic events in LGG. PROS1, a vital blood coagulation gene, was considered to promote the glioma immunosuppressive microenvironment (80). In addition, another study showed PROS1 was enriched in complement activation in LGG, indicating the critical role of CCC in the progression of LGG (81).
The complex tumor microenvironment is closely related to the occurrence and development of glioma (82,83). According to our findings, macrophage M2 and monocyte were highly infiltrated in the high-risk group, while T cell follicular helper and NK cell were highly infiltrated in the low-risk group. These findings suggest that there may be crosstalk between the CCC and immune cells in LGG. Microglia and macrophage M2 play an important role in glioma maintenance and progression (84,85). Macrophage M2 could produce complement C5 to promote chemotherapy resistance of glioma (86). A study showed that T cell follicular helper could exert antitumor immune effects in a CD8-dependent manner (87). T cells also stimulate humoral responses and induce the production of tumor-specific antibodies to activate the complement system on tumor cells, indicating T cell follicular helper might be related to the activation of the complement system in a CD8-dependent manner in LGG (88). NK cell is a powerful antitumor immune cell that could effectively kill glioma cells upon activation, and several clinical studies have been conducted on NK cell immunotherapy for the treatment of glioma (89-91). Meanwhile, we found that the negative regulation of NK cell-mediated immunity pathway was suppressed in the high-risk group. In summary, CCC might be associated with the high infiltration of these immunosuppressive immunocytes and decreased infiltration of antitumor immunocytes may be the reason for the poor prognosis of patients in the high-risk group.
Immunotherapy is a promising treatment strategy for glioma (92,93). In the current study, we found that the expression of several ICPs was upregulated in the high-risk group. The results of TIDE demonstrated that immunotherapy resistance might be present in high-risk patients, providing novel insight into the clinical choice of applying immunotherapy to patients with LGG. Moreover, stratification based on the expression of ICPs and risk score could accurately distinguish patients with different prognoses. Patients with high expression of ICPs and high risk scores had the worst prognosis, while risk scores had a more significant impact on the prognosis of patients with LGG compared to the expression of ICPs. Immune resistance is an important reason why immunotherapy for glioma has not achieved widespread use, and the development of tools to predict immunological resistance in glioma patients is therefore of great clinical importance (94,95). We validated our results in the GSE135222 and GSE78220 cohorts. Compared to low-risk patients, high-risk patients in two cohorts had shorter OS, poorer prognosis, and a higher proportion of responses to immunotherapy manifesting as SD/PD. These findings indicated that the CCC-based risk signature is able to accurately predict the response of patients to immunotherapy, providing a tool to predict the response to immunotherapy in patients with LGG.
Chemotherapy is one of the most important treatments for glioma (96-99). However, chemotherapy drugs for patients with LGG are still scarce, so the discovery of novel chemotherapeutic agents and strategies for patients with LGG is of great clinical importance (100-102). The current study identified MG-132, BMS-536924, PLX-4720, and AZD6482 as potential sensitive drugs for high-risk patients with LGG. MG-132 has been shown to promote glioma cell apoptosis and thus exert anti-glioma activity (103,104). A study reports that BMS-536924 could inhibit glioma growth in vitro and in vivo (105). Several studies show that PLX4720 offers significant advantages in treating BRAF V600E gliomas (106,107). AZD6482 is reported to exert antiproliferative activity and induce apoptosis in human GBM cells (108). We predicted the possible interactive targets between these four drugs and the CCC gene, and all of these sensitive drugs had possible targets interacting with CCC genes. Among them, MG-132 had the most possible targets, including F2R, C2, and C3AR1, which belonged to the six CCC genes that constructed the risk signature. What’s more, MG-132 was particularly recommended for high-risk patients because of its highest sensitivity. Thus, we performed in vitro experiments to validate the anti-glioma effect of MG-132, and the results demonstrated the strong anti-glioma activity of MG-132. Corresponding clinical trials based on our findings could be conducted to enrich the chemotherapy strategy for LGG.
The current study still had some limitations. The validation of this study was based on the CGGA dataset and showed strong predictive ability. However, other essential genes with prognostic values were not considered in this study. Furthermore, given that the prognostic signature was constructed and validated by leveraging data from public databases, evidence from biological experiments is required to further validate the statistical evidence we have provided.
Conclusions
In summary, through integrated bioinformatics analysis, this study investigated the relationship between CCC genes and the prognosis, immune microenvironment, and chemotherapy efficacy of patients with LGG. Our findings demonstrated that CCC genes are associated with the prognosis and immune infiltration of LGG and provide possible immunotherapeutic and novel chemotherapeutic strategies for patients with LGG.
Acknowledgments
Funding: None.
Footnote
Reporting Checklist: The authors have completed the TRIPOD reporting checklist. Available at https://tcr.amegroups.com/article/view/10.21037/tcr-23-906/rc
Peer Review File: Available at https://tcr.amegroups.com/article/view/10.21037/tcr-23-906/prf
Conflicts of Interest: All authors have completed the ICMJE uniform disclosure form (available at https://tcr.amegroups.com/article/view/10.21037/tcr-23-906/coif). The authors have no conflicts of interest to declare.
Ethical Statement: The authors are accountable for all aspects of the work in ensuring that questions related to the accuracy or integrity of any part of the work are appropriately investigated and resolved. The study was conducted in accordance with the Declaration of Helsinki (as revised in 2013).
Open Access Statement: This is an Open Access article distributed in accordance with the Creative Commons Attribution-NonCommercial-NoDerivs 4.0 International License (CC BY-NC-ND 4.0), which permits the non-commercial replication and distribution of the article with the strict proviso that no changes or edits are made and the original work is properly cited (including links to both the formal publication through the relevant DOI and the license). See: https://creativecommons.org/licenses/by-nc-nd/4.0/.
References
- Bale TA, Rosenblum MK. The 2021 WHO Classification of Tumors of the Central Nervous System: An update on pediatric low-grade gliomas and glioneuronal tumors. Brain Pathol 2022;32:e13060. [Crossref] [PubMed]
- Louis DN, Perry A, Wesseling P, et al. The 2021 WHO Classification of Tumors of the Central Nervous System: a summary. Neuro Oncol 2021;23:1231-51. [Crossref] [PubMed]
- Lapointe S, Perry A, Butowski NA. Primary brain tumours in adults. Lancet 2018;392:432-46. [Crossref] [PubMed]
- Horbinski C, Berger T, Packer RJ, et al. Clinical implications of the 2021 edition of the WHO classification of central nervous system tumours. Nat Rev Neurol 2022;18:515-29. [Crossref] [PubMed]
- van den Bent MJ. Interobserver variation of the histopathological diagnosis in clinical trials on glioma: a clinician's perspective. Acta Neuropathol 2010;120:297-304. [Crossref] [PubMed]
- Olar A, Wani KM, Alfaro-Munoz KD, et al. IDH mutation status and role of WHO grade and mitotic index in overall survival in grade II-III diffuse gliomas. Acta Neuropathol 2015;129:585-96. [Crossref] [PubMed]
- Reuss DE, Mamatjan Y, Schrimpf D, et al. IDH mutant diffuse and anaplastic astrocytomas have similar age at presentation and little difference in survival: a grading problem for WHO. Acta Neuropathol 2015;129:867-73. [Crossref] [PubMed]
- Youssef G, Miller JJ. Lower Grade Gliomas. Curr Neurol Neurosci Rep 2020;20:21. [Crossref] [PubMed]
- Schiff D, Van den Bent M, Vogelbaum MA, et al. Recent developments and future directions in adult lower-grade gliomas: Society for Neuro-Oncology (SNO) and European Association of Neuro-Oncology (EANO) consensus. Neuro Oncol 2019;21:837-53. [Crossref] [PubMed]
- Duffau H. Paradoxes of evidence-based medicine in lower-grade glioma: To treat the tumor or the patient? Neurology 2018;91:657-62. [Crossref] [PubMed]
- Caseiras GB, Chheang S, Babb J, et al. Relative cerebral blood volume measurements of low-grade gliomas predict patient outcome in a multi-institution setting. Eur J Radiol 2010;73:215-20. [Crossref] [PubMed]
- Danchaivijitr N, Waldman AD, Tozer DJ, et al. Low-grade gliomas: do changes in rCBV measurements at longitudinal perfusion-weighted MR imaging predict malignant transformation? Radiology 2008;247:170-8. [Crossref] [PubMed]
- Coburger J, Onken J, Rueckriegel S, et al. Eloquent Lower Grade Gliomas, a Highly Vulnerable Cohort: Assessment of Patients' Functional Outcome After Surgery Based on the LoG-Glio Registry. Front Oncol 2022;12:845992. [Crossref] [PubMed]
- Diaz S, Reyns N, Özduman K, et al. Microsurgical resection of gliomas of the cingulate gyrus: a systematic review and meta-analysis. Neurosurg Rev 2023;46:217. [Crossref] [PubMed]
- Sampson JH, Gunn MD, Fecci PE, et al. Brain immunology and immunotherapy in brain tumours. Nat Rev Cancer 2020;20:12-25. [Crossref] [PubMed]
- Ou A, Yung WKA, Majd N. Molecular Mechanisms of Treatment Resistance in Glioblastoma. Int J Mol Sci 2020;22:351. [Crossref] [PubMed]
- Dono A, Ballester LY, Primdahl D, et al. IDH-Mutant Low-grade Glioma: Advances in Molecular Diagnosis, Management, and Future Directions. Curr Oncol Rep 2021;23:20. [Crossref] [PubMed]
- Krauze AV, Camphausen K. Molecular Biology in Treatment Decision Processes-Neuro-Oncology Edition. Int J Mol Sci 2021;22:13278. [Crossref] [PubMed]
- Conway EM. Reincarnation of ancient links between coagulation and complement. J Thromb Haemost 2015;13:S121-32. [Crossref] [PubMed]
- Guglietta S, Rescigno M. Hypercoagulation and complement: Connected players in tumor development and metastases. Semin Immunol 2016;28:578-86. [Crossref] [PubMed]
- Cho A, McKelvey KJ, Lee A, et al. The intertwined fates of inflammation and coagulation in glioma. Mamm Genome 2018;29:806-16. [Crossref] [PubMed]
- Morgan ER, Mason WP, Maurice C. A critical balance: managing coagulation in patients with glioma. Expert Rev Neurother 2016;16:803-14. [Crossref] [PubMed]
- Zhu H, Yu X, Zhang S, et al. Targeting the Complement Pathway in Malignant Glioma Microenvironments. Front Cell Dev Biol 2021;9:657472. [Crossref] [PubMed]
- Zhao Z, Zhang KN, Wang Q, et al. Chinese Glioma Genome Atlas (CGGA): A Comprehensive Resource with Functional Genomic Data from Chinese Glioma Patients. Genomics Proteomics Bioinformatics 2021;19:1-12. [Crossref] [PubMed]
- Liberzon A, Birger C, Thorvaldsdóttir H, et al. The Molecular Signatures Database (MSigDB) hallmark gene set collection. Cell Syst 2015;1:417-25. [Crossref] [PubMed]
- The Genotype-Tissue Expression (GTEx) project. Nat Genet 2013;45:580-5. [Crossref] [PubMed]
- Ritchie ME, Phipson B, Wu D, et al. limma powers differential expression analyses for RNA-sequencing and microarray studies. Nucleic Acids Res 2015;43:e47. [Crossref] [PubMed]
- Tibshirani R. The lasso method for variable selection in the Cox model. Stat Med 1997;16:385-95. [Crossref] [PubMed]
- Subramanian A, Tamayo P, Mootha VK, et al. Gene set enrichment analysis: a knowledge-based approach for interpreting genome-wide expression profiles. Proc Natl Acad Sci U S A 2005;102:15545-50. [Crossref] [PubMed]
- Hänzelmann S, Castelo R, Guinney J. GSVA: gene set variation analysis for microarray and RNA-seq data. BMC Bioinformatics 2013;14:7. [Crossref] [PubMed]
- Kanehisa M, Furumichi M, Sato Y, et al. KEGG: integrating viruses and cellular organisms. Nucleic Acids Res 2021;49:D545-51. [Crossref] [PubMed]
- The Gene Ontology Resource. 20 years and still GOing strong. Nucleic Acids Res 2019;47:D330-8. [Crossref] [PubMed]
- Yoshihara K, Shahmoradgoli M, Martínez E, et al. Inferring tumour purity and stromal and immune cell admixture from expression data. Nat Commun 2013;4:2612. [Crossref] [PubMed]
- Newman AM, Liu CL, Green MR, et al. Robust enumeration of cell subsets from tissue expression profiles. Nat Methods 2015;12:453-7. [Crossref] [PubMed]
- Jiang P, Gu S, Pan D, et al. Signatures of T cell dysfunction and exclusion predict cancer immunotherapy response. Nat Med 2018;24:1550-8. [Crossref] [PubMed]
- Jung H, Kim HS, Kim JY, et al. DNA methylation loss promotes immune evasion of tumours with high mutation and copy number load. Nat Commun 2019;10:4278. [Crossref] [PubMed]
- Hugo W, Zaretsky JM, Sun L, et al. Genomic and Transcriptomic Features of Response to Anti-PD-1 Therapy in Metastatic Melanoma. Cell 2016;165:35-44. [Crossref] [PubMed]
- Maeser D, Gruener RF, Huang RS. oncoPredict: an R package for predicting in vivo or cancer patient drug response and biomarkers from cell line screening data. Brief Bioinform 2021;22:bbab260. [Crossref] [PubMed]
- Yang W, Soares J, Greninger P, et al. Genomics of Drug Sensitivity in Cancer (GDSC): a resource for therapeutic biomarker discovery in cancer cells. Nucleic Acids Res 2013;41:D955-61. [Crossref] [PubMed]
- Rees MG, Seashore-Ludlow B, Cheah JH, et al. Correlating chemical sensitivity and basal gene expression reveals mechanism of action. Nat Chem Biol 2016;12:109-16. [Crossref] [PubMed]
- Barretina J, Caponigro G, Stransky N, et al. The Cancer Cell Line Encyclopedia enables predictive modelling of anticancer drug sensitivity. Nature 2012;483:603-7. [Crossref] [PubMed]
- Gfeller D, Michielin O, Zoete V. Shaping the interaction landscape of bioactive molecules. Bioinformatics 2013;29:3073-9. [Crossref] [PubMed]
- Nickel J, Gohlke BO, Erehman J, et al. SuperPred: update on drug classification and target prediction. Nucleic Acids Res 2014;42:W26-31. [Crossref] [PubMed]
- Keiser MJ, Roth BL, Armbruster BN, et al. Relating protein pharmacology by ligand chemistry. Nat Biotechnol 2007;25:197-206. [Crossref] [PubMed]
- Kim S, Chen J, Cheng T, et al. PubChem in 2021: new data content and improved web interfaces. Nucleic Acids Res 2021;49:D1388-95. [Crossref] [PubMed]
- Xu S, Tang L, Liu Z, et al. Hypoxia-Related lncRNA Correlates With Prognosis and Immune Microenvironment in Lower-Grade Glioma. Front Immunol 2021;12:731048. [Crossref] [PubMed]
- Schneider CA, Rasband WS, Eliceiri KW. NIH Image to ImageJ: 25 years of image analysis. Nat Methods 2012;9:671-5. [Crossref] [PubMed]
- Navone SE, Guarnaccia L, Locatelli M, et al. Significance and Prognostic Value of The Coagulation Profile in Patients with Glioblastoma: Implications for Personalized Therapy. World Neurosurg 2019;121:e621-9. [Crossref] [PubMed]
- Czap AL, Becker A, Wen PY. Thrombotic Complications in Gliomas. Semin Thromb Hemost 2019;45:326-33. [Crossref] [PubMed]
- Zhou X, Li W, Yang J, et al. Tertiary lymphoid structure stratifies glioma into three distinct tumor subtypes. Aging (Albany NY) 2021;13:26063-94. [Crossref] [PubMed]
- Shi T, Chen J, Li J, et al. Identification of key gene modules and pathways of human glioma through coexpression network. J Cell Physiol 2019;234:1862-70. [Crossref] [PubMed]
- Zhou J, Guo Y, Fu J, et al. Construction and Validation of a Glioma Prognostic Model Based on Immune Microenvironment. Neuroimmunomodulation 2022;29:402-13. [Crossref] [PubMed]
- Tang S, Liao J, Long Y. Comparative assessment of the efficacy of gross total versus subtotal total resection in patients with glioma: A meta-analysis. Int J Surg 2019;63:90-7. [Crossref] [PubMed]
- Elsheikh M, Bridgman E, Lavrador JP, et al. Association of extent of resection and functional outcomes in diffuse low-grade glioma: systematic review & meta-analysis. J Neurooncol 2022;160:717-24. [Crossref] [PubMed]
- Buckner JC, Shaw EG, Pugh SL, et al. Radiation plus Procarbazine, CCNU, and Vincristine in Low-Grade Glioma. N Engl J Med 2016;374:1344-55. [Crossref] [PubMed]
- Shih HA, Sherman JC, Nachtigall LB, et al. Proton therapy for low-grade gliomas: Results from a prospective trial. Cancer 2015;121:1712-9. [Crossref] [PubMed]
- Johnson MO, Kirkpatrick JP, Patel MP, et al. The role of chemotherapy in the treatment of central neurocytoma. CNS Oncol 2019;8:CNS41. [Crossref] [PubMed]
- Berzero G, Bellu L, Baldini C, et al. Sustained Tumor Control With MAPK Inhibition in BRAF V600-Mutant Adult Glial and Glioneuronal Tumors. Neurology 2021;97:e673-83. [Crossref] [PubMed]
- Kaley T, Touat M, Subbiah V, et al. BRAF Inhibition in BRAF(V600)-Mutant Gliomas: Results From the VE-BASKET Study. J Clin Oncol 2018;36:3477-84. [Crossref] [PubMed]
- Ebrahimi-Fakhari D, Franz DN. Pharmacological treatment strategies for subependymal giant cell astrocytoma (SEGA). Expert Opin Pharmacother 2020;21:1329-36. [Crossref] [PubMed]
- Gao G, Yang M, Wang F, et al. Coagulation factor 2 thrombin receptor promotes malignancy in glioma under SOX2 regulation. Aging (Albany NY) 2020;12:7189. [Crossref] [PubMed]
- Liu W, Du M, Wan H, et al. Serpin family A member 1 is an oncogene in glioma and its translation is enhanced by NAD(P)H quinone dehydrogenase 1 through RNA-binding activity. Open Med (Wars) 2022;17:1645-54. [Crossref] [PubMed]
- Mangogna A, Belmonte B, Agostinis C, et al. Prognostic Implications of the Complement Protein C1q in Gliomas. Front Immunol 2019;10:2366. [Crossref] [PubMed]
- Liu Y, Zhao Y, Fang J, et al. Bioinformatics analysis of microenvironment-related genes associated with radioresistance in glioblastoma. Transl Cancer Res 2020;9:7495-504. [Crossref] [PubMed]
- Fei X, Wang H, Yuan W, et al. Tissue Factor Pathway Inhibitor-1 Is a Valuable Marker for the Prediction of Deep Venous Thrombosis and Tumor Metastasis in Patients with Lung Cancer. Biomed Res Int 2017;2017:8983763. [Crossref] [PubMed]
- Xing M, Yang Y, Huang J, et al. TFPI inhibits breast cancer progression by suppressing ERK/p38 MAPK signaling pathway. Genes Genomics 2022;44:801-12. [Crossref] [PubMed]
- Stavik B, Skretting G, Aasheim HC, et al. Downregulation of TFPI in breast cancer cells induces tyrosine phosphorylation signaling and increases metastatic growth by stimulating cell motility. BMC Cancer 2011;11:357. [Crossref] [PubMed]
- Tinholt M, Vollan HK, Sahlberg KK, et al. Tumor expression, plasma levels and genetic polymorphisms of the coagulation inhibitor TFPI are associated with clinicopathological parameters and survival in breast cancer, in contrast to the coagulation initiator TF. Breast Cancer Res 2015;17:44. [Crossref] [PubMed]
- Zheng H, Liu H, Li H, et al. Characterization of stem cell landscape and identification of stemness-relevant prognostic gene signature to aid immunotherapy in colorectal cancer. Stem Cell Res Ther 2022;13:244. [Crossref] [PubMed]
- Ning G, Huang YL, Zhen LM, et al. Prognostic Value of Complement Component 2 and Its Correlation with Immune Infiltrates in Hepatocellular Carcinoma. Biomed Res Int 2020;2020:3765937. [Crossref] [PubMed]
- Kolev M, Le Friec G, Kemper C. Complement--tapping into new sites and effector systems. Nat Rev Immunol 2014;14:811-20. [Crossref] [PubMed]
- Chanchal S, Mishra A, Singh MK, et al. Understanding Inflammatory Responses in the Manifestation of Prothrombotic Phenotypes. Front Cell Dev Biol 2020;8:73. [Crossref] [PubMed]
- Beckman JD, DaSilva A, Aronovich E, et al. JAK-STAT inhibition reduces endothelial prothrombotic activation and leukocyte-endothelial proadhesive interactions. J Thromb Haemost 2023;21:1366-80. [Crossref] [PubMed]
- Chaurasia SN, Ekhlak M, Kushwaha G, et al. Notch signaling functions in noncanonical juxtacrine manner in platelets to amplify thrombogenicity. Elife 2022;11:e79590. [Crossref] [PubMed]
- Winkler S, Hempel M, Brückner S, et al. Identification of Pathways in Liver Repair Potentially Targeted by Secretory Proteins from Human Mesenchymal Stem Cells. Int J Mol Sci 2016;17:1099. [Crossref] [PubMed]
- Mittal P, Romero R, Tarca AL, et al. Characterization of the myometrial transcriptome and biological pathways of spontaneous human labor at term. J Perinat Med 2010;38:617-43. [Crossref] [PubMed]
- Yarmoska SK, Alawieh AM, Tomlinson S, et al. Modulation of the Complement System by Neoplastic Disease of the Central Nervous System. Front Immunol 2021;12:689435. [Crossref] [PubMed]
- Wu S, Miao K, Wang L, et al. Bioinformatics analysis of C3 in brain low-grade gliomas as potential therapeutic target and promoting immune cell infiltration. Med Oncol 2022;39:27. [Crossref] [PubMed]
- Wang J, Tong L, Lin G, et al. Immunological and clinicopathological characteristics of C1RL in 2120 glioma patients. BMC Cancer 2020;20:931. [Crossref] [PubMed]
- Wang J, Wu N, Feng X, et al. PROS1 shapes the immune-suppressive tumor microenvironment and predicts poor prognosis in glioma. Front Immunol 2023;13:1052692. [Crossref] [PubMed]
- Zhou Y, Xiao D, Jiang X. LncRNA RP3-525N10.2-NFKB1-PROS1 triplet-mediated low PROS1 expression is an onco-immunological biomarker in low-grade gliomas: a pan-cancer analysis with experimental verification. J Transl Med 2022;20:335. [Crossref] [PubMed]
- Barthel L, Hadamitzky M, Dammann P, et al. Glioma: molecular signature and crossroads with tumor microenvironment. Cancer Metastasis Rev 2022;41:53-75. [Crossref] [PubMed]
- Ma Q, Long W, Xing C, et al. Cancer Stem Cells and Immunosuppressive Microenvironment in Glioma. Front Immunol 2018;9:2924. [Crossref] [PubMed]
- Hambardzumyan D, Gutmann DH, Kettenmann H. The role of microglia and macrophages in glioma maintenance and progression. Nat Neurosci 2016;19:20-7. [Crossref] [PubMed]
- Radin DP, Tsirka SE. Interactions between Tumor Cells, Neurons, and Microglia in the Glioma Microenvironment. Int J Mol Sci 2020;21:8476. [Crossref] [PubMed]
- Li Z, Meng X, Wu P, et al. Glioblastoma Cell-Derived lncRNA-Containing Exosomes Induce Microglia to Produce Complement C5, Promoting Chemotherapy Resistance. Cancer Immunol Res 2021;9:1383-99. [Crossref] [PubMed]
- Niogret J, Berger H, Rebe C, et al. Follicular helper-T cells restore CD8(+)-dependent antitumor immunity and anti-PD-L1/PD-1 efficacy. J Immunother Cancer 2021;9:e002157. [Crossref] [PubMed]
- Yan D, Li W, Liu Q, et al. Advances in Immune Microenvironment and Immunotherapy of Isocitrate Dehydrogenase Mutated Glioma. Front Immunol 2022;13:914618. [Crossref] [PubMed]
- Lim J, Park Y, Ahn JW, et al. Autologous adoptive immune-cell therapy elicited a durable response with enhanced immune reaction signatures in patients with recurrent glioblastoma: An open label, phase I/IIa trial. PLoS One 2021;16:e0247293. [Crossref] [PubMed]
- Jiang H, Yu K, Cui Y, et al. Combination of Immunotherapy and Radiotherapy for Recurrent Malignant Gliomas: Results From a Prospective Study. Front Immunol 2021;12:632547. [Crossref] [PubMed]
- Ishikawa E, Tsuboi K, Saijo K, et al. Autologous natural killer cell therapy for human recurrent malignant glioma. Anticancer Res 2004;24:1861-71. [PubMed]
- Xu S, Tang L, Li X, et al. Immunotherapy for glioma: Current management and future application. Cancer Lett 2020;476:1-12. [Crossref] [PubMed]
- Wang H, Xu T, Huang Q, et al. Immunotherapy for Malignant Glioma: Current Status and Future Directions. Trends Pharmacol Sci 2020;41:123-38. [Crossref] [PubMed]
- Yu MW, Quail DF. Immunotherapy for Glioblastoma: Current Progress and Challenges. Front Immunol 2021;12:676301. [Crossref] [PubMed]
- Jackson CM, Choi J, Lim M. Mechanisms of immunotherapy resistance: lessons from glioblastoma. Nat Immunol 2019;20:1100-9. [Crossref] [PubMed]
- Bi J, Chowdhry S, Wu S, et al. Altered cellular metabolism in gliomas - an emerging landscape of actionable co-dependency targets. Nat Rev Cancer 2020;20:57-70. [Crossref] [PubMed]
- Seliger C, Hau P. Drug Repurposing of Metabolic Agents in Malignant Glioma. Int J Mol Sci 2018;19:2768. [Crossref] [PubMed]
- Ghotme KA, Barreto GE, Echeverria V, et al. Gliomas: New Perspectives in Diagnosis, Treatment and Prognosis. Curr Top Med Chem 2017;17:1438-47. [Crossref] [PubMed]
- Wang H, Xu T, Jiang Y, et al. The challenges and the promise of molecular targeted therapy in malignant gliomas. Neoplasia 2015;17:239-55. [Crossref] [PubMed]
- Chua J, Nafziger E, Leung D. Evidence-Based Practice: Temozolomide Beyond Glioblastoma. Curr Oncol Rep 2019;21:30. [Crossref] [PubMed]
- van den Bent MJ. Chemotherapy for low-grade glioma: when, for whom, which regimen? Curr Opin Neurol 2015;28:633-938. [Crossref] [PubMed]
- Donovan LE, Lassman AB. Chemotherapy Treatment and Trials in Low-Grade Gliomas. Neurosurg Clin N Am 2019;30:103-9. [Crossref] [PubMed]
- Fan WH, Hou Y, Meng FK, et al. Proteasome inhibitor MG-132 induces C6 glioma cell apoptosis via oxidative stress. Acta Pharmacol Sin 2011;32:619-25. [Crossref] [PubMed]
- Ko JK, Choi CH, Kim YK, et al. The proteasome inhibitor MG-132 induces AIF nuclear translocation through down-regulation of ERK and Akt/mTOR pathway. Neurochem Res 2011;36:722-31. [Crossref] [PubMed]
- Zhou Q. BMS-536924, an ATP-competitive IGF-1R/IR inhibitor, decreases viability and migration of temozolomide-resistant glioma cells in vitro and suppresses tumor growth in vivo. Onco Targets Ther 2015;8:689-97. [Crossref] [PubMed]
- Nicolaides TP, Li H, Solomon DA, et al. Targeted therapy for BRAFV600E malignant astrocytoma. Clin Cancer Res 2011;17:7595-604. [Crossref] [PubMed]
- Dasgupta T, Olow AK, Yang X, et al. Erratum to: Survival advantage combining a BRAF inhibitor and radiation in BRAF V600E-mutant glioma. J Neurooncol 2016;126:395. [Crossref] [PubMed]
- Xu PF, Yang JA, Liu JH, et al. PI3Kβ inhibitor AZD6482 exerts antiproliferative activity and induces apoptosis in human glioblastoma cells. Oncol Rep 2019;41:125-32. [PubMed]