Construction of a prognosis model of head and neck squamous cell carcinoma pyroptosis and an analysis of immuno-phenotyping based on bioinformatics
Highlight box
Key findings
• We identified some new markers of cell pyroptosis for predicting the prognosis of head and neck squamous cell carcinoma (HNSCC) patients.
What is known, and what is new?
• Cell pyroptosis has the dual effect of promoting and inhibiting tumors.
• We studied pyroptosis-related-gene (PRG) markers that may promote and inhibit HNSCC and examined their relationship with immunity.
What is the implication, and what should change now?
• These PRG markers may provide new targets for the treatment of HNSCC, but they need to be validated in further experiments.
Introduction
Head and neck squamous cell carcinoma (HNSCC) is the sixth most common cancer worldwide (1). Research has shown that typical risk factors for HNSCC include smoking and excessive drinking (2). In addition, human immunodeficiency virus (HIV) (3), exposure to radiation (4), salted food (5), and poor oral hygiene (6) have also been shown to increase the risk of HNSCC.
At present, most HNSCC is accompanied by cervical lymph node metastasis, and radical treatment is carried out by a combination of surgery, radiotherapy and chemotherapy (7). However, recurrent disease and metastatic tumors are generally considered incurable and have a poor prognosis. Thus, more effective treatments, such as targeted therapies, for patients with advanced HNSCC need to be explored. To the best of our knowledge, no effective biomarker has yet been found (8).
Pyroptosis is a newly discovered form of pyroptosis triggered by pro-inflammatory signals (9) and inflammatory caspases (1, 4, and 5) after the activation of classical or non-classical inflammatory body pathways. Research has shown that the exogenous activation of pyroptosis can trigger powerful anti-tumor effects (10).
The occurrence and development of tumors depend on aberrations in oncogenes, tumor suppressor genes, and the tumor microenvironment (TME) (10). It has been suggested that the TME score could serve as a prognostic biomarker for HNSCC. In particular, naive B cells, regulatory T cells, and follicular T cells in patients with high immunosuppressive HNSCC were associated with improved outcomes, while neutrophils and activated mast cells in patients with low immunosuppressive HNSCC were associated with poorer outcomes (11).
In conclusion, pyroptosis is known to play an important role in both tumor and anti-tumor processes. However, anti-tumor effects require a combination of multiple immune factors and multiple pathways. Thus, a comprehensive understanding of the characteristics of pyroptosis-mediated TME immune infiltration may provide important insights into the TME of HNSCC and assist in the prediction of immune therapy responses. We present this article in accordance with the TRIPOD reporting checklist (available at https://tcr.amegroups.com/article/view/10.21037/tcr-23-922/rc).
Methods
Downloaded data
We use R version (x64 4.1.1) for data analysis. And we obtained the mutation data, RNA sequencing (RNA-seq) data, and corresponding clinical information of HNSCC and normal patients from The Cancer Genome Atlas (TCGA) database (https://portal.gdc.cancer.gov/repository). We also downloaded the clinical information of HNSCC patients from the Gene Expression Omnibus (GEO) database (GSE41613) (https://www.ncbi.nlm.nih.gov). In addition, the copy number data of HNSCC patients were downloaded from https://xena.ucsc.edu/. This study was conducted in accordance with the Declaration of Helsinki (as revised in 2013).
Comprehensive analysis of the pyroptosis-related genes (PRGs) in TCGA database
Genes reported to be related to pyroptosis in the literature were selected (12). The RNA-seq data from TCGA database were used to identify the differentially expressed genes (DEGs) between the normal tissues and tumor tissues; genes with P values <0.05 were selected. The results were visualized, and the DEGs were visualized in a box graph. In addition, a protein-protein interaction (PPI) map of the DEGs was generated using the STRING website (https://string-db.org/). Before any comparisons, the expression data in TCGA database were normalized to fragments of millions of bases per thousand values. “Limma” package was used to identify the DEGs with P values <0.05. The significance levels of the DEGs are indicated as follows: * indicates the P value is <0.05, ** indicates the P value is <0.01, and *** indicates the P value is <0.001. The mutation data related to the HNSCC patients from TCGA data were downloaded, and the data were visualized in a waterfall diagram using the “maftools” package. Based on the copy data, each variation frequency was statistically plotted in a heatmap, and a copy number circle chart was drawn after the start- and the end-genes of the copy data were sorted into files with Perl.
Classification and immunoassays of pyroptotic typing
First, the clinical data in TCGA and GEO databases were integrated, and data on the expression of the PRGs were extracted. Second, the survival of the patients with the 52 PRGs was analyzed using R’s “survival” package, and the statistically significant DEGs in terms of overall survival (OS) between clusters A and B were selected. Third, R’s “rcolorbrewer” package was used to generate a prognostic network diagram for these genes.
To explore the relationship between the significantly expressed PRGs and the HNSCC subtypes, a consistent cluster analysis was performed on the data of all 626 HNSCC patients integrated from TCGA and GEO databases. The K values with the highest intracluster correlation and the lowest intercluster correlation were selected and typed by increasing the cluster variable (k) from 2 to 10. We then drew the survival curve. The gene expressions and clinical characteristics, including tumor stage, gender, age, and sample typing, of all the HNSCC patients in TCGA and GEO databases were combined and heatmaps were drawn using the “pheatmap” package from R. The Gene Set Variation Analysis (GSVA) package of R was used for the GSVA and the single-sample Gene-Set Enrichment Analysis (ssGSEA). Moreover, R’s “limma” package was used to conduct the principal component analysis (PCA) on the samples.
DEGs and functional enrichment analysis of pyroptotic typing
The “limma” package of R was used to analyze the difference between pyroptotic clusters A and B, and a logfcfilter <0.585 and an adjusted P value filter <0.05 were the filter conditions used to screen for DEG. A Venn diagram was drawn with the Venn diagram package. The Gene Ontology (GO) analysis and Kyoto Encyclopedia of Genes and Genomes (KEGG) analysis of these DEGs were carried out with “clusterprofiler” package.
Construction of prognostic model and risk-score analysis
The statistically significant (P<0.05) DEGs related to prognosis from 1,003 DEGs were screened using the “survival” package of R. Prognosis-related DEGs were then consistently clustered. By increasing the cluster variable (k) from 2 to 10, the k value with the highest correlation was selected within the groups, and the k value with the lowest correlation was selected between the groups, and the samples were then typed, and the survival curve was drawn. In addition, a heatmap of the DEGs was drawn with R’s “pheatmap” package using the gene expression profiles and clinical features of all the HNSCC patients in TCGA and GEO databases, including tumor stage, gender, age, PRGs (hereinafter referred to as PRG clusters), and differential prognosis-related clusters of PRG clusters (hereinafter referred to as gene clusters). To screen out the pyroptosis-related DEGs, which are different from each gene cluster, R’s “limma” package was used to obtain 37 pyroptosis-related DEGs under the conditions of sd (expclu [, I]) <0.001 and P value <0.05. A box diagram of the above 37 DEGs was visualized with “ggpubr” package, and a PPI diagram of DEGs was made based on the PPIs.
Among the 37 prognosis-related DEGs, nine survival-related genes were further screened by a least absolute shrinkage and selection operator (LASSO)-Cox regression model, and the correlation coefficients of these genes were obtained. The risk prognostic model was constructed using these nine genes and the related risk coefficients. All the samples were divided into training and test groups with R on the condition that y=rt [, 2], P=0.5, list=F. Patients from both groups were used to construct prognostic models, and the risk score for each patient was obtained based on the formulas and correlation coefficients. The median value of the risk score in the training group was taken as the cut-off value, and the patients in the two groups were divided into high- and low-risk groups, respectively. A Sanggi diagram was drawn by observing the construction process of the prognostic model using the “global” package of R. Time-dependent receiver operating characteristic (ROC) curves were used to evaluate the predictive power of the model for 1-, 3-, and 5-year survival, and the survival curves of the high- and low-risk groups were analyzed.
R’s “limma” package was used to analyze the differences in the risk scores between each PRG clusters and gene clusters, and the results were drawn in a box diagram using “ggpubr” package. Next, R’s “limma” package was used to analyze the risk of the PRGs, and the pyroptosis-related DEGs were identified as high and low risk (P<0.05), and a box diagram was drawn with the “ggpubr” package.
Construction of the nomogram combined with clinical factors
After screening the clinical information, an alignment diagram was drawn using the “RMS” package of R. Based on the multivariate regression analysis, gender, age group, tumor stage, risk value, total score, linear predictive value, the 1-, 3-, and 5-year survival rate were comprehensively analyzed. The line segments with scales were drawn on the same plane according to the scale to mark the relationship between the various variables in the model. In addition, R was used to define the function of the risk curve, and a risk curve and survival state diagram were drawn for the training and test groups under the condition of bioriskplot = function (inputfile = null, project = null). Finally, a risk heatmap was plotted using the “heatmap” package. This model was validated using the GSE41613 and GSE31056 data sets, and risk scores were calculated using the same formula as that employed for TCGA and GEO (GSE41613) patient analyses.
Immune-cell and TCIA analysis
R was used to calculate the relative contents of the T cells, cluster of differentiation (CD)4+T cells, and B cells in each sample. Each content was equal to 1. All the immune cells were then cycled to determine the correlation between the risk score and the immune cells. Next, R’s “ggplot” package was used to draw a scatter diagram and a correlation heatmap of the immune cells with P values <0.05. Moreover, R’s “estimate” package was used to score the mechanism cells and immune cells of each sample, and the “ggplot2” package was used to draw the violin diagram.
Data from The Cancer Imaging Archive (TCIA; http://tcia.at/) were downloaded. TCIA data were used to validate the performance of predicting anti-PD-1 and anti-cytotoxic T lymphocyte antigen 4 (CTLA4) responses. TCIA prediction scores were assessed using “limma” and “ggpubr” packages in R.
Mutation analysis of high- and low-risk groups
Using R’s “maftools” package, data on the differences in the mutations associated with the PRGs in the high- and low-risk groups were analyzed, summarized, analyzed, annotated, and visualized. The “include” function was then used to draw a mutation waterfall graph for the mutation data, which is displayed according to the risk grouping. Next, R’s “ggpubr” package was used to analyze the correlations between the high-risk and low-risk groups, and a box graph and a correlation graph were drawn.
Drug-sensitivity analysis
Using the drug information downloaded from R and taking a P value <0.01 as the filtering condition, the differences in drug sensitivity between the high-risk and low-risk groups were analyzed using the “prophetic” package, and a box diagram was drawn.
Statistical analysis
OS refers to the interval from the date of diagnosis to the date of death. Survival curves were plotted based on the Kaplan-Meier log-rank test results. The prognostic value for 1-, 2-, and 3-year OS was assessed using the ROC curves. All the statistical analyses were performed using R version 4.1.1. Statistical significance was set at P<0.05.
Results
Correlation analysis of the PRGs in TCGA database
The RNA-seq data of 501 HNSCC patients and 44 normal patients, the clinical information of 528 HNSCC patients, and the mutation data of 508 HNSCC patients were extracted from TCGA database. After the literature search (12), 52 PRGs were identified (Table S1). A waterfall diagram of the genes related to cell-death mutations (plotted with R) is shown in Figure 1A. As Figure 1A shows, a total of 506 mutations were found in the sample data, of which 380 mutations were PRGs. Of these, tumor protein P53 (TP53) had the highest mutation rate at 66%. This indicated that mutations in TP53 were closely related to the development of HNSCC tumors, and tumors might be caused by changes in the immune microenvironment of mutant TP53 (13).
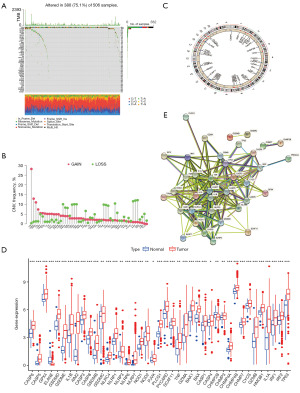
In addition, the copy number data of HNSCC patients were downloaded and their frequency variability was plotted (Figure 1B). Among the 52 PRGs, TP53, gasdermin C (GSDMC), and gasdermin D (GSDMD) had a much higher frequency of copy number increase than deletion, while glutathione peroxidase 4 (GPX4), interleukin 18 (IL18), and elastase, neutrophil expressed (ELANE) had a lower frequency of copy number increase than deletion. Figure 1C shows the locations of the HNSCC alterations in the genes on their respective chromosomes.
We also compared the messenger RNA (mRNA) expression levels between the HNSCC and normal tissues and found that the expression levels of most of the PRGs were positively correlated with the copy number variants, such as caspase 9 (CASP9) and absent in melanoma 2 (AIM2). However, in some copy number variants, such as ELANE, mRNA expression was downregulated (Figure 1D). Thus, while copy number variation explained many observed changes in the expression of PRGs, it was not the only factor involved in regulating mRNA expression. This indicated that copy number variation was involved in regulating the expression of mRNA and has some value in the prognosis of HNSCC (14).
The PPI network was used to explore the interactions among the PRGs, and the results are shown in Figure 1E. We found that there were significant differences in the genetic landscape and expression levels of the PRGs between the HNSCC and control samples, indicating the potential function of PRGs in the carcinogenesis of HNSCC.
Identification of pyroptosis subtypes in HNSCC
The clinical information of 98 patients with HNSCC was downloaded from the GEO database. The RNA-q (RNA sequencing) of 643 patients with HNSCC was integrated with the clinical data of 626 HNSCC patients from TCGA and GEO databases, the expression of the PRGs was extracted, and the survival of the above-mentioned 52 PRGs was analyzed with R “survival” package. Next, 27 genes [bcl2 antagonist/Killer 1 (BAK1), caspase 3 (CASP3), caspase 5 (CASP5), caspase 6 (CASP6), caspase 9 (CASP9), charged multivesicular body protein 4B (CHMP4B), charged multivesicular body protein 7 (CHMP7), cytochrome C, somatic (CYCS), GSDMC, granzyme A (GZMA), granzyme B (GZMB), high mobility group box 1 (HMGB1), interleukin 1 alpha (IL1A), interleukin 1 beta (IL1B), interleukin 6 (IL6), interferon regulatory factor 1 (IRF1), interferon regulatory factor 2 (IRF2), NLR family card domain containing 4 (NLRC4), NLR family pyrin domain containing 2 (NLRP2), NLR family pyrin domain containing 1 (NLRP1), NLR family pyrin domain containing 6 (NLRP6, nucleotide binding oligomerization domain containing 2 (NOD2), phospholipase C gamma 1 (PLCG1), SR-related CTD associated factor 11 (SCAF11), TIR domain containing adaptor protein (TIRAP), tumor necrosis factor (TNF) and TP53] were obtained, of which the survival rates of patients in PRG clusters A and B were statistically significant (Figure S1). The synthesis of PRG’ interactions, regulator linkages, and their prognostic value in patients with HNSCC was confirmed in the pyroptosis network (Figure 2A).
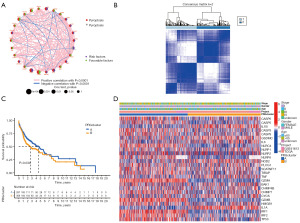
To explore the relationship between the expression of the 27 pyroptosis-related DEGs and HNSCC subtypes, a consistent cluster analysis on data of 626 HNSCC patients from TCGA and GEO databases. By increasing the clustering variable (k) from 2 to 10, it was found that when k=2, the intra-group correlation was the highest and the inter-group correlation was the lowest, which indicated that the 626 patients with HNSCC could be divided into two groups according to the 27 DEGs (Figure 2B). The survival score of patients with PRG cluster A was higher than that of those with PRG cluster B; that is, PRG cluster A was low risk and PRG cluster B was high risk. The heatmap shows the gene expression profiles and clinical features, including tumor stage (I, II, III, IV, or unknown), age (≤60 years old, >60 years old, or unknown), gender (male or female), database (GSE41613 or TCGA), and PRG cluster (A or B). Differences in the clinical features and OS between the two clusters were found (P<0.05), which provides preliminary evidence that these PRGs show significant effects on OS rates in HNSCC We observed that patients in Cluster A had a lower TNM (tumor node metastasis) stage (P<0.05) than those in Cluster B (Figure 2C,2D).
Characteristics of the TME in distinct subtypes
The “GSVA” package of R was used for the GSVA and ssGSEA. The GSVA results (Figure 3A) showed that tryptophan metabolism, the T-cell receptor signaling pathway, the B-cell receptor signaling pathway, Leishmania infection, the chemokine signaling pathway, primary immunodeficiency, natural killer cell mediated cytotoxicity, antigen processing and presentation, autoimmune thyroid disease, graft versus host disease, allograft rejection, type I diabetes, viral myocarditis, systemic lupus erythematosus, the intestinal immune network, asthma, cell-adhesion molecules, and the hematopoietic cell system for immunoglobulin A production were highly expressed in PRG cluster A and lowly expressed in PRG cluster B. Bladder cancer and nitrogen metabolism were highly expressed in PRG cluster B ,which suggests that bladder cancer and nitrogen metabolism may mutually inhibit HNSCC tumors. The ssGSEA results (Figure 3B) showed that the scores of CD56 and neutrophil PRG cluster B were higher than those of cluster A, and the other immune cells scored the opposite. Further, CD56 and neutrophils had tumor-promoting effects on HNSCC (15,16).
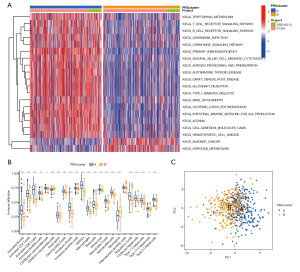
A PCA, also known as Karhunen-Loéve transformation, was conducted to explore and visualize the high-dimensional data set of the above 27 pyroptotic DEGs. The results showed that these DEGs were well differentiated in PRG clusters A and B (Figure 3C), indicating that patients could be successfully classified by the RPG clusters.
To further explore the differences in gene function and pathways between the subgroups of pyroptosis typing, “limma” R package was used with a logfcfilter <0.585 and an adjusted P valued filter <0.05 as the standard for extracting the DEGs. The 1003 DEGs screened were drawn on a Venn map. Based on these DEGs, GO and KEGG pathway analyses were carried out. The results showed that the DEGs were mainly related to the immune response, the chemokine-mediated signal pathway, and inflammatory cell chemotaxis (Figure S2A,S2B).
Construction of prognostic model and risk-score analysis
To explore the potential biological behavior of each mode of cell death, 355 prognosis-associated DEGs were screened from the 1,003 DEGs in the pyroptosis classification using the R “survival” package. To further verify this regulatory mechanism, we used consensus clustering algorithms to classify the patients into two genic subtypes based on prognostic genes; that is, gene subtypes A and B (Figure 4A). The Kaplan-Meier curve showed that patients with gene subtype B had poorer OS than those with gene subtype A (log-rank test, P=0.002; Figure 4B). In addition, the pattern of gene subtype B was associated with advanced TNM staging and an older age. There were significant differences in prognostic gene expression among the two gene subtypes, which is consistent with the expected results for the pyroptosis patterns (Figure 4C,4D). We also found that these genes and proteins interacted very closely (Figure S3A).
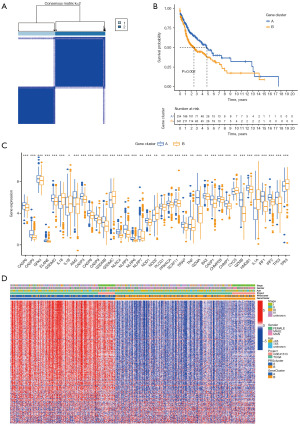
Construction and validation of the prognostic model
For the 37 statistically significant prognosis-related DEGs, the LASSO-Cox penalty regression model was used for dimensionality reduction. A LASSO regression graph was generated (Figure S3B), and Lambda was selected as the critical value (Figure S3C). Finally, the model was optimized using a stepwise regression method, and a prognostic model comprising nine survival-related genes [i.e., CTLA4, V-set and immunoglobulin domain containing 4 (VSIG4), heparin-binding-epidermal growth factor (HBEGF), AQP1, SCNN1D, argininosuccinate synthase 1 (ASS1), family 83 (FAM83), CDKN2A, and serine protease inhibitor Kazal 6 (SPINK6)] was constructed. The analytical concept scoring model was expressed as follows: risk score = (0.223 × CTLA4 exp.) + (0.238 × VSIG4 exp.) + (0.163 × HBEGF exp.) + (–0.331 × AQP1 exp.) + (–0.196 × SCNN1D exp.) + (0.219 × ASS1 exp.) + (–0.171 × FAM83 exp.) + (–0.086 × CDKN2A exp.) + (–0.096 × SPINK6 exp.).
All the samples were divided into the training group and the test group. The training and test group comprised 297 patients, respectively. There were 148 high-risk and 149 low-risk patients in the training group, and 149 high-risk and 148 low-risk patients in the testing group. In the training group (Figure 5A), the OS rate of the high-risk group was low, and the difference between the two groups was statistically significant, which showed that the model could predict the survival prognosis of patients with HNSCC. The areas under the ROC curve of the 1-, 3- and 5-year survival rates were 0.718, 0.758, and 0.780, respectively, indicating that the model had good predictive ability (Figure 5B). The survival and ROC curves of the test group also showed the predictability of the prognosis model (Figure 5C,5D). Moreover, R’s “ggalluvial” package was used to draw a Sanggi diagram (Figure S3D) to show the construction process of the prognostic model. We also analyzed all the samples and verified that the prognostic model had a good ability to predict the survival of HNSCC patients of HNSCC (Figure S3E,S3F).
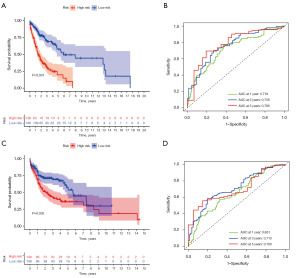
Construction of nomogram combined with clinical factors
The “limma” package of R was used to analyze the differences in the risk scores of the RPG clusters and gene clusters. The results showed that there was no difference in the risk scores of patients in the RPG clusters (P>0.05) (Figure S4A). However, the risk scores of patients differed in the gene clusters (P<0.05), and the risk score of the high-risk group was higher than that of the low-risk group. This indicated that a low-risk score may be closely related to immune-activation characteristics, while a high-risk score may be related to matrix-activation characteristics. More importantly, gene subtype B had a significantly higher risk score than gene subtype A.
We comprehensively analyzed the nomogram combined with the clinical factors (Figure 6). We identified 14 pyroptosis-related DEGs between the high- and low-risk groups (P<0.05) (Figure 6A). Among them, certain PRGs [i.e., CYCS, BAK1, CASP3, nucleotide binding oligomerization domain containing 1 (NOD1), protein kinase camp-activated catalytic subunit alpha (PRKACA), IL6, IL1A, glutathione peroxidase 4 (GPX4), and HMGB1] were upregulated in the high-risk group and downregulated in the low-risk group, and thus classified as high-risk PRGs. While other PRGs (i.e., IL18, GSDMC, NOD2, NLRP1, and PLCG1) were downregulated in the high-risk group and upregulated in the low-risk group, and thus classified as low-risk PRGs.
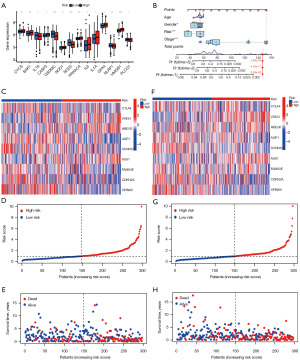
A multivariate Cox regression analysis was conducted to identify the key clinical factors, such as gender, age group, tumor stage, risk value, total score, linear predictive value, and the 1-, 3-, and 5-year OS rates, and the “RMS” R package was used to construct the nomogram (Figure 6B), and generate the 1-, 3-, and 5-year calibration curves (Figure S4B). As Figure S4B shows, the three curves are close to the grey line, indicating that the model is highly accurate at predicting the 1-, 3-, and 5-year survival rates of patients. All the above results further validated the accuracy of our model in assessing the prognosis of HNSCC patients.
Finally, the clinical and survival characteristics of the prognostic model were analyzed (Figure 6). The risk curve and survival state diagram of the test and training groups (Figure 6D,6E,6G,6H) were drawn. The results indicated that the prognostic model could predict the prognosis of HNSCC patients well, and similar results were found for the training' group. In addition, the risk heatmap of the survival-related genes in the test and training group (Figure 6C,6F) showed that low-risk genes, such as CTLA4, FAM83E, AQP1, SCNND, CDKN2A, and SPINK6, decreased as risk increased, while high-risk genes, such as VSIG4, HBEGF and ASS1 increased as risk decreased.
External risk signature validation
To further validate the risk signatures developed above, data pertaining to HNSCC patients from the GSE41613 and GSE31056 data sets were used. These patients were separated into high- and low-risk groups based on the median risk scores derived from TCGA and GEO merged data. We validated the prognostic model using the external data (Figure S5). Significant differences in OS were found between the low- and high-risk HNSCC groups (Figure S5D). The ROC curve analyses further confirmed the predictive accuracy of the model, with respective AUCs for 1-, 2-, and 3-year OS of 0.797, 0.818, and 0.811, respectively (Figure S5C). The distribution of the risk scores and survival status between the high- and low-risk groups is shown in Figure S5A,S5B. The results of the validation set demonstrate the ability of our model to predict prognosis’ in HNSCC.
Immune-cell and TCIA analysis
To further analyze the immune cells of the prognosis model, we analyzed the correlation between the survival-related genes and immune cells (Figure 7A). Figure S6A shows a graph displaying the correlations between the risk score of the patients and immune cells. Notably, B immature cells, dendritic cells, resting mast cells, plasma cells, CD4 memory T cells, CD4+T cells, auxiliary follicular T cells, and regulatory T cells were negatively correlated with the risk score of the patients. Conversely, M0 and M2 macrophages, activated mast cells, and quiescent natural killers cells were positively correlated with the risk score of the patients.
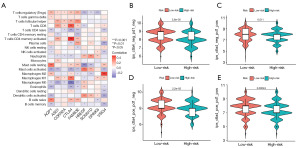
In addition, we analyzed the TME of each sample, including the stromal score, immune score, and Estimation of STromal and Immune cells in MAlignant Tumor tissues using Expression (ESTIMATE) score (Figure S6B). However, no differences in the stromal, immunological, and ESTIMATE scores were found between the high- and low-risk groups. The association between the risk score and immune cells suggests that there is a relationship between each immune cell. However, the results showed that the risk score was not correlated with the tumor immune microenvironment, which may be caused by the interaction between various immune pathways and cells.
We next investigated whether pyroptosis-related genetic signatures may contribute to the clinical benefit of immune checkpoint inhibitor therapy. TCIA analysis showed that the immune targets of both PD-1 or CTLA4 were effective in the treatment of HNSCC (Figure 7B-7E). These results suggest that better treatment options may be available for patients with HNSCC, such as single drug therapies and combination therapies (17).
Mutation analysis of high- and low-risk groups
The gene set used comprised all the PRGs (Table S1). There was a mutation difference between the high- and low-risk groups, such that the mutation rate of the low-risk group was lower than that of the high-risk group (Figure 8A-8C). In addition, the correlation between the tumor mutation load and risk score was analyzed, and a positive correlation was found between the risk score and tumor mutation load (Figure 8D). These results suggest that tumor mutations have a negative effect on prognosis.
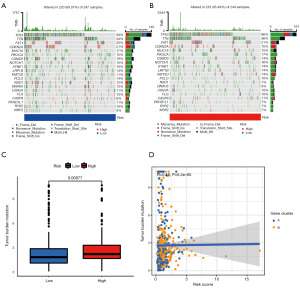
Next, we selected some chemotherapy drugs to evaluate the sensitivity of patients in the low- and high-risk groups to these drugs. Interestingly, we found that the semi-inhibitory concentrations of cisplatin, cytarabine, docetaxel, and sorafenib were lower in the high-risk group, while the semi-inhibitory concentrations of chemotherapy drugs, such as gemcitabine and gefitinib, were significantly lower in the low-risk group. In summary, these results indicate that the PRGs were associated with drug sensitivity (Figure S7A-S7Z).
Discussion
In this study, we analyzed the role of PRGs in HNSCC from a multivariate genomics perspective and constructed a prognostic model of HNSCC with multiple levels and structures. Finally, a prognostic model comprising nine survival-related genes (i.e., CTLA4, VSIG4, HB-EGF, AQP1, SCNN1D, ASS1, FAM83, CDKN2A, and SPINK6) was constructed using a univariate Cox regression analysis. We verified the feasibility of the model in several respects. In the model of the pyroptotic DEGs, CTLA4, FAM83E, AQP1, SCNN1D, CDKN2A, and SPINK6 were low-risk genes, while VSIG4, HBEGF, and ASS1 were high-risk genes. In our study, the correlation between prognostic models and immune cells and pathways was well demonstrated, but there was no difference in the analysis of TME. This may be related to the PPIs in the TME and the influence of multiple pathways, such that the ESTIMATE score and the immune environment had little effect on HNSCC.
Two types of typing were performed in this study: (I) the typing of PRG clusters; and (II) the typing of gene clusters. We analyzed the differences in the risk scores between the two types of models. The risk score was found to be an independent factor in the gene clusters, but the risk score was not found to be an independent factor in the PRG clusters. It may be that the risk score is not representative due to the small number of DEGs associated with pyroptosis.
The study showed that the occurrence and development of tumors depend on the mutations of carcinogenic and suppressor oncogenes, as well as the TME (10). However, in HNSCC, the effect of pyroptosis on the TME and prognosis is unclear. This study produced a prognostic model with nine PRGs (i.e., CTLA4, VSIG4, HBEGF, AQP1, SCNN1D, ASS1, FAM83, CDKN2A, and SPINK6), and found that it could predict the prognosis of HNSCC patients.
Protein CTLA4 is an important negative regulator of the immune response (18). It can cause the immune system to fail to kill tumor cells (19). In recent studies (20), some FAM83 members with sequence similarities have been shown to be significantly upregulated in a variety of human cancer types. One study showed that FAM83E regulates tumor progression (21). Further, the high expression of FAM83E has been shown to be related to better survival results. The AQP1 gene is located on chromosome 7p14 and encodes highly conserved transmembrane aquaporin to promote transcellular water transport. AQP1 plays a carcinogenic role in many types of solid cancer (22). In this prognostic model, AQP1 seemed to be a tumor suppressor gene that inhibited the growth of HNSCC; however, its mechanism in pyroptosis needs to be studied further. SCNN1D is the member of the ENaC/degenerin superfamily (23), which is widely expressed in non-epithelial cells and epithelial cells (24). In many tumors, SCNN1D is lowly expressed (25), which is consistent with our research conclusion. The CDKN2A gene encodes the proteins P14 adp ribosylation factor (ARF) and p16 INK4a, also known as p16 (26). In HNSCC, CDKN2A is mainly an inhibitor gene (27). A previous study showed that CDKN2A is significantly correlated with the level of pro-inflammatory factors in HNSCC samples and can be used as an independent prognostic factor (28). It may be related to the inflammatory response caused by pyroptosis (29). Human SPINK6 is a selective inhibitor of kallikrein-related peptidase in skin. SPINK6 has been shown to be associated with skin cancer and liver cancer cells (30), and to promote the metastasis of nasopharyngeal carcinoma through a secretory mechanism (31). Interestingly, we found that SPINK6 is a low-risk pyroptosis gene of HNSCC; however, the relationship between pyroptosis and SPINK6 needs to be further explored.
VSIG4 is specifically expressed in monocytes, macrophages, and dendritic cells (32). Recent studies have confirmed that VSIG4 is overexpressed in a variety of cancer cells and plays a role in cellular immune function and in promoting tumor progression as a carcinogenic oncogene (33,34). It is well known that NLRP3 inflammasome promotes inflammation or induces inflammatory pyroptosis (35). In this study, the prognosis of VSIG4 was positively correlated with macrophages. Consistent with the results of the present study, another study showed that VSIG4 initiates inhibitory signals to specifically weaken the expression of NLRP3 in macrophages and affects pyroptosis (36). We speculated that VSIG4 negatively regulates the expression of NLRP3 by upregulating the immune response of macrophages, affects pyroptosis, and thus promotes the occurrence of tumors. HBEGF is expressed under inflammatory and pathological conditions (37). Some studies have shown that fibroblasts from RA (rheumatoid arthritis) (38) and colon tumors (39) promote the occurrence of inflammation with macrophages. Our study showed that HBEGF, as a high-risk gene, is more meaningful for mast cells. ASS1 is a urea cycle enzyme that is essential in the conversion of nitrogen from ammonia and aspartic acid to urea (40). ASS1 has been shown to play an important role in inhibiting tumor cell proliferation, tumor cell migration, tumor invasion, and tumor angiogenesis (41). However, there may be significant differences in the expression levels of ASS1 in different tumor types (42-45). ASS1 was a high-risk gene for prognosis in HNSCC, and the mechanism is likely to be closely related to cell metabolism and the immune response (46).
Conclusions
Our comprehensive analysis of PRGs revealed that their extensive regulatory mechanisms affect the tumor immune interstitial microenvironment, clinicopathological characteristics, and patient prognosis. More importantly, the immune-related mechanisms underlying pyroptosis may be related to PD-1/PD-L1- or CTLA. Our study identified some novel genetic markers for predicting the prognosis of patients with HNSCC and provides an important foundation for future studies on the relationship between PRGs and immunity in HNSCC. However, due to the limitations of the database samples, we were unable to obtain the patients’ treatment plans and related information, and were thus unable to equilibrate the various samples. In addition, due to the lack of data, it was difficult to confirm whether these regulatory factors also play a corresponding role in the pyrolytic pathway of HNSCC. This question deserves further investigation. Further in vitro and in vivo experiments should be performed in the laboratory to test our hypothesis.
Acknowledgments
We sincerely acknowledge TCGA and GEO databases for providing their platforms and the contributors for uploading their meaningful data sets.
Funding: The study was funded by
Footnote
Reporting Checklist: The authors have completed the TRIPOD reporting checklist. Available at https://tcr.amegroups.com/article/view/10.21037/tcr-23-922/rc
Peer Review File: Available at https://tcr.amegroups.com/article/view/10.21037/tcr-23-922/prf
Conflicts of Interest: All authors have completed the ICMJE uniform disclosure form (available at https://tcr.amegroups.com/article/view/10.21037/tcr-23-922/coif). The authors have no conflicts of interest to declare.
Ethical Statement: The authors are accountable for all aspects of the work in ensuring that questions related to the accuracy or integrity of any part of the work are appropriately investigated and resolved. The study was conducted in accordance with the Declaration of Helsinki (as revised in 2013).
Open Access Statement: This is an Open Access article distributed in accordance with the Creative Commons Attribution-NonCommercial-NoDerivs 4.0 International License (CC BY-NC-ND 4.0), which permits the non-commercial replication and distribution of the article with the strict proviso that no changes or edits are made and the original work is properly cited (including links to both the formal publication through the relevant DOI and the license). See: https://creativecommons.org/licenses/by-nc-nd/4.0/.
References
- Global Burden of Disease Cancer Collaboration. Global, Regional, and National Cancer Incidence, Mortality, Years of Life Lost, Years Lived With Disability, and Disability-Adjusted Life-years for 32 Cancer Groups, 1990 to 2015: A Systematic Analysis for the Global Burden of Disease Study. JAMA Oncol 2017;3:524-48. [Crossref] [PubMed]
- Leemans CR, Snijders PJF, Brakenhoff RH. The molecular landscape of head and neck cancer. Nat Rev Cancer 2018;18:269-82. [Crossref] [PubMed]
- Haase K, Piwonski I, Stromberger C, et al. Incidence and survival of HNSCC patients living with HIV compared with HIV-negative HNSCC patients. Eur Arch Otorhinolaryngol 2021;278:3941-53. [Crossref] [PubMed]
- Preston-Martin S, Thomas DC, White SC, et al. Prior exposure to medical and dental x-rays related to tumors of the parotid gland. J Natl Cancer Inst 1988;80:943-9. [Crossref] [PubMed]
- Yu MC, Yuan JM. Epidemiology of nasopharyngeal carcinoma. Semin Cancer Biol 2002;12:421-9. [Crossref] [PubMed]
- Guha N, Boffetta P, Wünsch Filho V, et al. Oral health and risk of squamous cell carcinoma of the head and neck and esophagus: results of two multicentric case-control studies. Am J Epidemiol 2007;166:1159-73. [Crossref] [PubMed]
- Ang KK, Chen A, Curran WJ Jr, et al. Head and neck carcinoma in the United States: first comprehensive report of the Longitudinal Oncology Registry of Head and Neck Carcinoma (LORHAN). Cancer 2012;118:5783-92. [Crossref] [PubMed]
- Hammerman PS, Hayes DN, Grandis JR. Therapeutic insights from genomic studies of head and neck squamous cell carcinomas. Cancer Discov 2015;5:239-44. [Crossref] [PubMed]
- Shen Y, Li X, Wang D, et al. Novel prognostic model established for patients with head and neck squamous cell carcinoma based on pyroptosis-related genes. Transl Oncol 2021;14:101233. [Crossref] [PubMed]
- Feng X, Luo Q, Zhang H, et al. The role of NLRP3 inflammasome in 5-fluorouracil resistance of oral squamous cell carcinoma. J Exp Clin Cancer Res 2017;36:81. [Crossref] [PubMed]
- Zhang J, Zhong X, Jiang H, et al. Comprehensive characterization of the tumor microenvironment for assessing immunotherapy outcome in patients with head and neck squamous cell carcinoma. Aging (Albany NY) 2020;12:22509-26. [Crossref] [PubMed]
- Song W, Ren J, Xiang R, et al. Identification of pyroptosis-related subtypes, the development of a prognosis model, and characterization of tumor microenvironment infiltration in colorectal cancer. Oncoimmunology 2021;10:1987636. [Crossref] [PubMed]
- Kong W, Han Y, Gu H, et al. TP53 mutation-associated immune infiltration and a novel risk score model in HNSCC. Biochem Biophys Rep 2022;32:101359. [Crossref] [PubMed]
- Chera BS, Kumar S, Beaty BT, et al. Rapid Clearance Profile of Plasma Circulating Tumor HPV Type 16 DNA during Chemoradiotherapy Correlates with Disease Control in HPV-Associated Oropharyngeal Cancer. Clin Cancer Res 2019;25:4682-90. [Crossref] [PubMed]
- Mandal R, Şenbabaoğlu Y, Desrichard A, et al. The head and neck cancer immune landscape and its immunotherapeutic implications. JCI Insight 2016;1:e89829. [Crossref] [PubMed]
- Hu S, Lu H, Xie W, et al. TDO2+ myofibroblasts mediate immune suppression in malignant transformation of squamous cell carcinoma. J Clin Invest 2022;132:e157649. [Crossref] [PubMed]
- Mei Z, Huang J, Qiao B, et al. Immune checkpoint pathways in immunotherapy for head and neck squamous cell carcinoma. Int J Oral Sci 2020;12:16. [Crossref] [PubMed]
- Schubert D, Bode C, Kenefeck R, et al. Autosomal dominant immune dysregulation syndrome in humans with CTLA4 mutations. Nat Med 2014;20:1410-6. [Crossref] [PubMed]
- Guan X, Wang Y, Sun Y, et al. CTLA4-Mediated Immunosuppression in Glioblastoma is Associated with the Infiltration of Macrophages in the Tumor Microenvironment. J Inflamm Res 2021;14:7315-29. [Crossref] [PubMed]
- Ma Z, Zhou Z, Zhuang H, et al. Identification of Prognostic and Therapeutic Biomarkers among FAM83 Family Members for Pancreatic Ductal Adenocarcinoma. Dis Markers 2021;2021:6682697. [Crossref] [PubMed]
- Cipriano R, Miskimen KL, Bryson BL, et al. Conserved oncogenic behavior of the FAM83 family regulates MAPK signaling in human cancer. Mol Cancer Res 2014;12:1156-65. [Crossref] [PubMed]
- Huo Z, Lomora M, Kym U, et al. AQP1 Is Up-Regulated by Hypoxia and Leads to Increased Cell Water Permeability, Motility, and Migration in Neuroblastoma. Front Cell Dev Biol 2021;9:605272. [Crossref] [PubMed]
- Hanukoglu I, Hanukoglu A. Epithelial sodium channel (ENaC) family: Phylogeny, structure-function, tissue distribution, and associated inherited diseases. Gene 2016;579:95-132. [Crossref] [PubMed]
- Zhao R, Ali G, Chang J, et al. Proliferative regulation of alveolar epithelial type 2 progenitor cells by human Scnn1d gene. Theranostics 2019;9:8155-70. [Crossref] [PubMed]
- Ji HL, Zhao RZ, Chen ZX, et al. δ ENaC: a novel divergent amiloride-inhibitable sodium channel. Am J Physiol Lung Cell Mol Physiol 2012;303:L1013-26. [Crossref] [PubMed]
- Cottone L, Eden N, Usher I, et al. Frequent alterations in p16/CDKN2A identified by immunohistochemistry and FISH in chordoma. J Pathol Clin Res 2020;6:113-23. [Crossref] [PubMed]
- Comprehensive genomic characterization of head and neck squamous cell carcinomas. Nature 2015;517:576-82. [Crossref] [PubMed]
- Yang J, Jiang Q, Liu L, et al. Identification of prognostic aging-related genes associated with immunosuppression and inflammation in head and neck squamous cell carcinoma. Aging (Albany NY) 2020;12:25778-804. [Crossref] [PubMed]
- Hsu SK, Li CY, Lin IL, et al. Inflammation-related pyroptosis, a novel programmed cell death pathway, and its crosstalk with immune therapy in cancer treatment. Theranostics 2021;11:8813-35. [Crossref] [PubMed]
- Ge K, Huang J, Wang W, et al. Serine protease inhibitor kazal-type 6 inhibits tumorigenesis of human hepatocellular carcinoma cells via its extracellular action. Oncotarget 2017;8:5965-75. [Crossref] [PubMed]
- Zheng LS, Yang JP, Cao Y, et al. SPINK6 Promotes Metastasis of Nasopharyngeal Carcinoma via Binding and Activation of Epithelial Growth Factor Receptor. Cancer Res 2017;77:579-89. [Crossref] [PubMed]
- Kim KH, Choi BK, Kim YH, et al. Extracellular stimulation of VSIG4/complement receptor Ig suppresses intracellular bacterial infection by inducing autophagy. Autophagy 2016;12:1647-59. [Crossref] [PubMed]
- Liao Y, Guo S, Chen Y, et al. VSIG4 expression on macrophages facilitates lung cancer development. Lab Invest 2014;94:706-15. [Crossref] [PubMed]
- Byun JM, Jeong DH, Choi IH, et al. The Significance of VSIG4 Expression in Ovarian Cancer. Int J Gynecol Cancer 2017;27:872-8. [Crossref] [PubMed]
- Miao EA, Rajan JV, Aderem A. Caspase-1-induced pyroptotic cell death. Immunol Rev 2011;243:206-14. [Crossref] [PubMed]
- Huang X, Feng Z, Jiang Y, et al. VSIG4 mediates transcriptional inhibition of Nlrp3 and Il-1β in macrophages. Sci Adv 2019;5:eaau7426. [Crossref] [PubMed]
- Bollée G, Flamant M, Schordan S, et al. Epidermal growth factor receptor promotes glomerular injury and renal failure in rapidly progressive crescentic glomerulonephritis. Nat Med 2011;17:1242-50. [Crossref] [PubMed]
- Kuo D, Ding J, Cohn IS, et al. HBEGF(+) macrophages in rheumatoid arthritis induce fibroblast invasiveness. Sci Transl Med 2019;11:eaau8587. [Crossref] [PubMed]
- He Z, Chen L, Chen G, et al. Interleukin 1 beta and Matrix Metallopeptidase 3 Contribute to Development of Epidermal Growth Factor Receptor-Dependent Serrated Polyps in Mouse Cecum. Gastroenterology 2019;157:1572-1583.e8. [Crossref] [PubMed]
- Rabinovich S, Adler L, Yizhak K, et al. Diversion of aspartate in ASS1-deficient tumours fosters de novo pyrimidine synthesis. Nature 2015;527:379-83. [Crossref] [PubMed]
- Huang HY, Wu WR, Wang YH, et al. ASS1 as a novel tumor suppressor gene in myxofibrosarcomas: aberrant loss via epigenetic DNA methylation confers aggressive phenotypes, negative prognostic impact, and therapeutic relevance. Clin Cancer Res 2013;19:2861-72. [Crossref] [PubMed]
- Delage B, Fennell DA, Nicholson L, et al. Arginine deprivation and argininosuccinate synthetase expression in the treatment of cancer. Int J Cancer 2010;126:2762-72. [Crossref] [PubMed]
- Yang TS, Lu SN, Chao Y, et al. A randomised phase II study of pegylated arginine deiminase (ADI-PEG 20) in Asian advanced hepatocellular carcinoma patients. Br J Cancer 2010;103:954-60. [Crossref] [PubMed]
- Szlosarek PW, Klabatsa A, Pallaska A, et al. In vivo loss of expression of argininosuccinate synthetase in malignant pleural mesothelioma is a biomarker for susceptibility to arginine depletion. Clin Cancer Res 2006;12:7126-31. [Crossref] [PubMed]
- Kobayashi E, Masuda M, Nakayama R, et al. Reduced argininosuccinate synthetase is a predictive biomarker for the development of pulmonary metastasis in patients with osteosarcoma. Mol Cancer Ther 2010;9:535-44. [Crossref] [PubMed]
- Mao Y, Shi D, Li G, et al. Citrulline depletion by ASS1 is required for proinflammatory macrophage activation and immune responses. Mol Cell 2022;82:527-541.e7. [Crossref] [PubMed]