The integration of bulk and single-cell sequencing data revealed the function of FKBP10 in the gastric cancer microenvironment
Highlight box
Key findings
• The overexpression of FKBP10 in inflammatory cancer-associated fibroblasts (iCAFs) may be associated with the invasion and metastasis of gastric cancer.
What is known and what is new?
• Upregulation of FKBP10 has been observed and is intimately linked to malignant phenotypes of the tumor.
• In this study, the results show that FKBP10 facilitate the invasion and metastasis of gastric cancer. FKBP10 is predominantly expressed in iCAFs. FKBP10 and FKBP10-coexpressed genes may guide cancer cells to their surroundings by mediating extracellular matrix remodeling, particularly through influencing collagen synthesis and secretion.
What is the implication, and what should change now?
• The results of this study promote the understanding of cell-specific FKBP10-dependent biological alterations in human gastric cancer, and render available clues to guide immune checkpoint blockade therapy decisions.
Introduction
Background
Surgical resection is the unique treatment option for patients with gastric cancer (1). Postoperative adjuvant chemotherapy is widely used in clinical practice, greatly improving the prognosis of patients (2-4). However, gastric cancer frequently progresses to an advanced or metastatic stage due to the lack of clinical manifestations (5). Distant spread of tumor cells is the most common recurrence pattern in patients with gastric cancer after surgery (6). Therefore, it is particularly vital to elucidate the molecular mechanism that triggers gastric cancer metastasis, which may provide a theoretical basis for the development of novel targeted therapies.
Rationale and knowledge gap
In gastric cancer and other cancers, component remodeling of the extracellular matrix (ECM) plays a crucial role in the regulation of tumor progression (7-12). FKBP10 is localized in the coarse endoplasmic reticulum and involved in collagen synthesis of organisms via biochemical processes (13). Dysfunction of FKBP10 affects the synthesis and secretion of ECM proteins, mediating the occurrence of diseases, including osteogenesis imperfect and idiopathic pulmonary fibrosis (IPF) (14-17). In addition, in some cancers, such as melanoma, lung cancer, renal cell carcinoma, colorectal cancer, and even gastric cancer, upregulation of FKBP10 has been observed and is intimately linked to malignant phenotypes of the tumor (18-22). Famously, the tumor microenvironment (TME) has become a critical element in determining the phenotype of cancer and affecting the effect of treatment (23-25). Nevertheless, the expression localization of FKBP10 in the TME of gastric cancer, as well as its corresponding functions, still remain largely unknown.
Objective
Emerging techniques and advances allow us to elucidate the causes and dysfunctional effects of FKBP10 aberrantly expressed in the TME of primary and metastatic gastric cancer (26). Herein, we found that FKBP10 promoted the invasion and metastasis of gastric cancer cells. To further illustrate the importance of the FKBP10 gene in gastric cancer progression, we combined public single-cell sequencing data for analysis. Unexpectedly, FKBP10 was concentrated in cancer-associated fibroblasts (CAFs), especially in inflammatory CAFs (iCAFs). The FKBP10-coexpressed genes (FCGs) in iCAFs were calculated, and the genes of the corresponding modules were analyzed by functional enrichment to evaluate the specific role of FCGs in the TME. Finally, based on bulk RNA sequencing data, we identified two distinct clusters using nine FCGs that correlated with patient prognosis and assessed the potential immunotherapeutic significance of FCGs through a series of bioinformatics analyses. We present this article in accordance with the MDAR reporting checklist (available at https:/tcr.amegroups.com/article/view/10.21037/tcr-23-1484/rc).
Methods
Cell culture and transfection
The gastric cancer cell lines (AGS and HGC-27) utilized in our study were purchased from Procell Biotech (Wuhan, China). Cells were routinely cultured in Dulbecco’s modified Eagle medium (DMEM; Wisent, Nanjing, China) at 37 ℃ in 5% CO2, supplemented with 10% fetal bovine serum (FBS; Wisent) and 100 U/mL penicillin streptomycin (Wisent). The small interfering RNA (siRNA) targeting FKBP10 was obtained from Sangon Biotech (Shanghai, China) with the sequences as follows: 5'-CCACACCUACAAUACCUAUAUTT-3'. And the siRNA targeting negative control (siNC) was used as the control group. When the cells in the six-well plate reached 80% confluence, we transfected according to the manufacturer’s protocol.
RNA extract and quantitative real-time polymerase chain reaction (qRT-PCR)
Total cellular RNA was isolated by cell/tissue total RNA isolation kit (Vazyme, Nanjing, China). cDNA was subsequently obtained using HiScript III RT SuperMix for qPCR (Vazyme). Finally, ChamQ Universal SYBR qPCR Master Mix (Vazyme) was used as a fluorescent dye for amplification. The specific primers were as follows: FKBP10-F, 5'-GTTCACCTCGCATGACTAC-3'; FKBP10-R, 5'-CCTCTCTCCCACACACAT-3'; ACTB-F, 5'-TGGCACCCAGCACAATGAA-3'; ACTB-R, 5'-CTAAGTCATAGTCCGCCTAGAAGCA-3'. The relative expression levels of messenger RNA (mRNA) were normalized to ACTB.
Scratch assay and invasion assay
Transwell chambers and Matrigel were purchased from Corning to evaluate the migration and invasion abilities of gastric cancer cells. Cells were diluted with serum-free medium, and then, 100 µL single-cell suspension (5×105 cells) was planted into the upper chamber, and 600 µL medium containing 20% FBS was added to the lower chamber. 24 hours later, cells were fixed with pure methanol for 20 minutes and stained with 0.1% crystal violet for 15 minutes. Pictures were obtained by microscope and the number of cells invaded or metastasized were assessed.
Cells with stable condition were planted into six-well plates and scratched with a 200 µL tip after the cell confluency reached 90%. After washing three times with phosphate buffered solution (PBS), the cells were cultured in a medium containing 2% serum for 24 hours, then the scratch width was observed under the microscope.
Processing and analysis of single-cell sequencing data
The GSE163558 (26) dataset from the Gene Expression Omnibus (GEO) (https://ncbi.nlm.nih.gov/geo/query/acc.cgi?acc=GSE163558) was retrieved. Single-cell transcriptome data from six gastric cancer patients with nine samples were selected, incorporating three primary tumor samples (PT1, PT2, and PT3), two liver metastasis samples (Li1 and Li2), two lymph nodes metastasis samples (LN1 and LN2), one peritoneal metastasis sample (P1), and one ovary metastasis sample (O1). Downstream principal component analysis (PCA) and t-distributed stochastic neighbor embedding (t-SNE) analysis were performed by the Seurat R package (version 4.2.0). The cell filtration criteria were set to cells with <200 genes, >5,000 genes, or >20% mitochondrial genes, resulting in 40,667 cells. After normalizing the gene expression matrix using “LogNormalize” method, 2,000 hypervariable genes (HVGs) were identified through the “FindVariableFeatures” function of the Seurat package. The appropriate principal components (PCs) were determined by the functions of “JackStraw” and “ScoreJackStraw”. Eventually, 20 PCs were used for subsequent analysis. The “FindClusters” and “FindAllMarkers” functions of Seurat divided the cells into 21 clusters and confirmed the marker genes for each cluster. Based on previous literature reports, cell types were identified via the marker genes in each cluster (26-28). The “scCustomize” package was applied to visualize the expression of FKBP10 in the TME. In addition, we further validated the expression of FKBP10 using the paired tumor-adjacent samples from nine patients in the GSE183904 dataset obtained from the GEO database, following the preprocessing steps as described (28). We utilized the “monocle3” package to perform pseudotime trajectory analysis of the fibroblasts in PT2 samples to observe the expression changes of FKBP10. By conducting the Wilcoxon rank-sum test, we compared the iCAFs of patients with metastatic tumor and patients with primary tumor, and further identified differentially expressed genes (DEGs). In order to identify statistically significant DEGs in the field of statistics, a cutoff point of Bonferroni-corrected p-values less than 0.05 was utilized. To elucidate the biological effects of iCAFs in tumor progression, we performed gene ontology (GO) and Kyoto Encyclopedia of Genes and Genomes (KEGG) enrichment analysis on these DEGs using the “clusterProfiler” package. The “hdWGCNA” package was used to establish FCG networks and functional modules associated with iCAFs in PT2 samples.
Bulk sequencing data acquisition and investigation
Bulk RNA sequencing data and clinical data of stomach adenocarcinoma from The Cancer Genome Atlas (TCGA-STAD; https://portal.gdc.cancer.gov/) were acquired through the “TCGAbiolinks” package. After the non-tumor samples were rejected, 346 gastric cancer samples were retained according to the overall survival (OS) time ≥10 days. Kaplan-Meier analysis of OS with FCGs from single-cell data results was performed by “survival” and “survminer” packages, resulting in nine prognosis-related FCGs. Based on the above nine FCG expression matrices, 346 samples were divided into two distinct clusters using non-negative matrix factorization (“NMF” package). The Tumor Immune Dysfunction and Exclusion (TIDE) algorithm was applied to evaluate differences in immune checkpoint blockade (ICB) therapy responses among the two clusters (29). A single-sample gene set enrichment analysis (ssGSEA) was introduced via “GSVA” package to measure the infiltration abundance of 28 immune cell types in the TME between the two clusters. After calculating the DEGs of the two clusters by “DESeq2” package, GSEA for GO and KEGG terms were conducted using “clusterProfiler” package. The University of ALabama at Birmingham CANcer data analysis Portal (https://ualcan.path.uab.edu/index.html) (UALCAN) was used to analyze FKBP10 in different types of gastric cancer samples.
Statistical analysis
All analyses were implemented by R software package (version 4.2.1) and GraphPad Prism 9.1.0. Student’s t-test and one-way analysis of variance (ANOVA) were applied to compare the data from different groups.
Ethical statement
The study was conducted in accordance with the Declaration of Helsinki (as revised in 2013).
Results
FKBP10 promotes invasion and migration of gastric cancer cells
Our previous study had shown that FKBP10 was highly expressed in gastric cancer tissue and was connected with a poor prognosis (19). To further clarify the effects of FKBP10 on tumor biological behavior, we conducted an experimental analysis. The knockdown efficiency of FKBP10 was verified by RT-qPCR (Figure 1A). The transwell assay demonstrated that the migration and invasion abilities of HGC-27 and AGS cells decreased apparently with the knockdown of FKBP10 (Figure 1B). Scratch assays of HGC-27 and AGS cells revealed that the wound healing rate in the siFKBP10 group was significantly reduced compared with the control group (Figure 1C,1D). These results implied the catalytic role of FKBP10 in tumor metastasis.
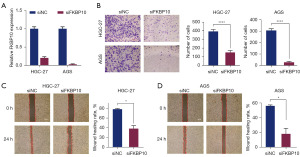
Single-cell atlas of primary and metastatic gastric cancer
In order to further elucidate the cause of abnormally high expression of FKBP10 in tumor tissues and the mechanism of regulating tumor invasion and migration, we mined single-cell transcriptome data for subsequent analysis. Based on the single-cell RNA sequencing (scRNA-seq) dataset of GSE163558, the gene expression profiles of 51308 cells were obtained from nine gastric cancer samples, including three primary tumor samples (PT1, PT2, and PT3) containing 15,729 cells and six metastatic samples containing 35,579 cells. After quality control, we extracted 40,667 cells, of which 12,014 cells originated from primary tumor samples and another 28,653 cells from metastatic samples. The characteristics of each sample after filtration are shown in Figure 2A. nFeature_RNA represents the total number of genes, nCount_RNA represents the number of RNA expressed, the more nCount_RNA, the higher nFeature_RNA, and the correlation coefficient was 0.83, which indicates that our samples are qualified and available (Figure 2B). Then, we obtained highly variable genes (HVGs) that expressed significant differences between cells, and the top ten genes are demonstrated in Figure 2C. IGLC2, IGHA1, and IGKC are the top three HVGs responsible for encoding allogeneic immunoglobulins (30). PCA discerned all 20 PCs and visualized them through ElbowPlot (Figure 2D). Twenty-one clusters were identified using 20 PCs, and the top five marker genes are exhibited in Figure 2E. After removing the fifth low-quality cluster, these 20 clusters were annotated into ten cell subsets based on previous research (Figure 3A). There was distinct heterogeneity in the proportion of cell subsets among samples (Figure 3B). Bubble chart was used to show the expression levels of cell type marker genes (Figure 3C). The composition ratio of the intergroup cell cluster is shown in Figure 3D. Figure 3E shows the proportion of each cell cluster in an independent sample. Compared with the primary tumor group, the T cell cluster and B cell cluster in the metastatic tumor group increased significantly, while the myeloid cell cluster and epithelial cell cluster were clearly reduced.
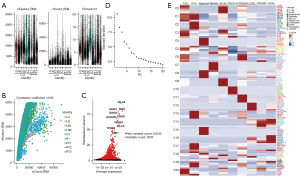
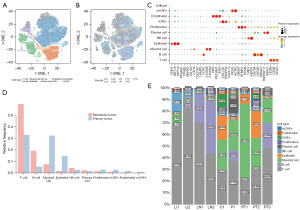
Expression and function of FKBP10 in the TME
FKBP10 was principally expressed in iCAFs, myo-CAFs (mCAFs), and Edothelial, with specificity between different groups (Figure 4A,4B). In primary tumor samples, FKBP10 expression was mainly detected in PT2 sample, while in metastatic tumor samples, FKBP10 expression was primarily identified in O1 sample (Figure 4C). In addition, to further validate the expression pattern of FKBP10 in the TME, we performed cell annotation on the GSE183904 dataset (Figure 4D) and found that FKBP10 was predominantly highly expressed on the iCAFs of tumor samples (Figure 4E). Due to the small number of cells in the O1 sample, which was prone to bias, we subsequently extracted 317 iCAFs and 111 mCAFs from the PT2 sample to perform pseudo-time analysis. Figure 4F,4G depicted the developmental trajectories of fibroblasts based on cell-type and pseudotime, respectively. FKBP10 was stably expressed during the developmental trajectory of iCAFs (Figure 4H).
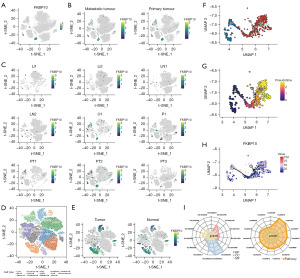
Since FKBP10 was primarily expressed in iCAFs, we explored the DEGs between primary tumor samples and metastatic tumor samples in iCAFs (table available at https://cdn.amegroups.cn/static/public/tcr-23-1484-1.xlsx). By applying GO and KEGG enrichment analysis to these DEGs (Figure 4I), we discovered that iCAFs were mainly involved in biological processes such as ECM organization (GO:0030198), focal adhesion (GO:0005925, hsa04510), CXCR chemokine receptor binding (GO:0045236), IL-17 signaling pathway (hsa04657), and TNF signaling pathway (hsa04668). To further explore the potential function of FKBP10 in the TME, we performed high-dimensional weighted gene co-expression network analysis (hdWGCNA) of iCAFs in PT2 samples, constructed a co-expression network, co-expression module (Figure 5A). Then, the eigengene-based connectivity (kME) was calculated to determine the hub genes within each module (Figure 5B). After identifying FKBP10 as one of the key genes in iCAFs-M16, we proceeded to analyze this module. As shown in Figure 5C, epithelial cells and proliferative cells exhibited higher harmonized module eigengenes (hMEs). Figure 5D illustrates the co-expression network of the top 25 FCGs of kME in iCAFs-M16. Finally, GO and KEGG enrichment analysis on the 86 hub genes (table available at https://cdn.amegroups.cn/static/public/tcr-23-1484-1.xlsx) of iCAFs-M16 were carried out. KEGG pathway analysis revealed enrichment of protein processing in the endoplasmic reticulum, focal adhesion, and pathways in cancer (Figure 5E). Enriched in these GO terms were connected with the ECM (Figure 5F), including collagen fibril organization (GO:0030199), ECM organization (GO:0030198), collagen-containing ECM (GO:0062023), dolichyl-diphosphooligosaccharide-protein glycotransferase activity (GO:0004579).
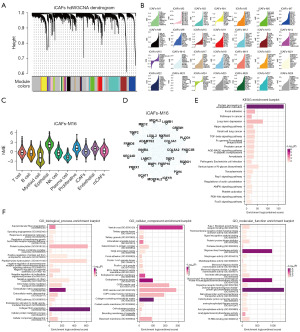
Identification of gastric cancer subtypes based on single-cell data results
Then, we selected 86 hub genes (Table S1) from iCAFs-M16 and performed Kaplan-Meier analysis, and obtained nine genes, namely, FKBP10, BCAT1, FNDC3B, SEC23A, CCND1, INHBA, ADAM10, TCEAL9, and COL5A2 (Table S2). Based on the expression of these genes, the TCGA-STAD cohort (n=346) was divided into two clusters (Figure 6A) by NMF with the appropriate rank value of 2. Kaplan-Meier analysis demonstrated that patients in cluster 2 had a worse prognosis than those in cluster 1 (Figure 6B). The TIDE algorithm was applied to predict the immunotherapy response effect of patients in clusters, and the proportion of responders in cluster 2 was lower than cluster 1 (Figure 6C). Figure 6D reveals that the TIDE score, Dysfunction score, exclusion score in cluster 2 were significantly increased, indicating that the immune escape potential of patients in cluster 2 was elevated, and the efficacy of immune checkpoint inhibitors may be terrible. Meanwhile, the CAF score in cluster 2 was distinctly multiplied, which was consistent with single-cell sequencing results, implying that FKBP10 and FCGs mainly played a role in CAFs. Figure 6E shows the difference distribution of 28 immune cell types between the two distinct clusters. Cluster 2 had significantly lower T cell infiltration abundance (including activated CD4 T cells, activated CD8 T cells, central memory CD4 T cells) compared to cluster 1, whereas immunosuppressive cell subsets were obviously evaluated [including regulatory T cells, macrophages, myeloid-derived suppressor cells (MDSCs)]. To confirm the gene expression signatures between the two clusters, we explored DEGs with a total of 585 up-regulated and 45 down-regulated DEGs preserved (table available at https://cdn.amegroups.cn/static/public/tcr-23-1484-2.xlsx). Besides, we conducted GSEA on these DEGs and visualized them with a ridge map, which was basically consistent with the results of single-cell sequencing data analysis, principally enriched in the ECM-related pathways (Figure 6F). Ultimately, by utilizing the corresponding clinical data (table available at https://cdn.amegroups.cn/static/public/tcr-23-1484-3.xlsx) and gene expression data, we had further validated the expression of FKBP10 in tumors. Consistent with previous findings, FKBP10 exhibited high expression in various types of tumor tissues (Figure S1).
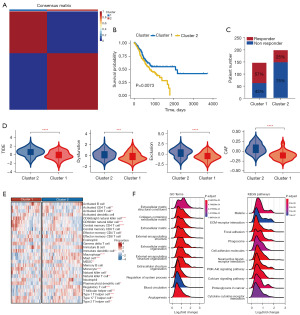
Discussion
Key findings
The TME is a dynamic system coordinated by cellular communication, which is an indispensable component to promote tumor progression and metastasis (24). While studies have demonstrated that targeting FKBP10 may be a prospective strategy for the treatment of gastric cancer (31,32), our research concentrates on exploring the function of FKBP10 in the TME. In the present study, we found that FKBP10 was involved in the invasion and migration of gastric cancer. FKBP10 was predominantly expressed in CAFs, especially in iCAFs. Importantly, FKBP10 and FCGs may guide cancer cells to their surroundings by mediating ECM remodeling, particularly through influencing collagen synthesis and secretion. Eventually, the NMF algorithm based on nine FCGs (FKBP10, BCAT1, FNDC3B, SEC23A, CCND1, INHBA, ADAM10, TCEAL9, and COL5A2) achieved satisfactory clustering effect, and significantly disparate immune activity and immunotherapy response in the two clusters were observed.
Strengths and limitations & comparison with similar researches
Incorporating our previous research, there is substantial evidence that FKBP10 is abnormally expressed in many cancers and affects the malignant biological behavior of cancer cells (18-22). Consistent with these results, our experiment manifested that knockdown FKBP10 inhibited the invasion and metastasis of gastric cancer cells in vitro. Admittedly, the TME is composed of a variety of cell types, including cancer cells, immune cells (33,34), fibroblasts (35), tissue-resident cells (36), etc. Unlike existing studies, we attempted to hunt for the cell-specific expression of FKBP10 in the TME, hoping to decipher the ability of FKBP10 to mediate cancer cell invasion and migration. Remarkedly, FKBP10 is principally localized in CAFs, especially iCAFs, which is not only in accord with the literature reports (17,37), but also renders vital clues for the function of FKBP10 in the TME. The CAFs that express α-smooth-muscle-actin (αSMA) is an indispensable element of the tumor stroma in the TME (38,39). Studies have indicated that stroma contributes to the progression of tumors, and when tissues are exposed to the stromal environment of chronic inflammation for a lengthy term, the incidence of tumors increases accordingly, especially Helicobacter pylori gastritis connected with gastric cancer (40,41). What can be determined is that CAFs are engaged in the mutual communication between cancer and stroma, supporting tumor formation, progression, and metastasis (42-44). Compared with normal fibroblasts, CAFs isolated from cancer tissues accelerate angiogenesis (45), facilitate the transformation of non-tumorigenic epithelial cells into tumorigenic cells (46), and compel cancer cells to invade via heterogeneous cell-cell interactions (47). Consequently, we hypothesize that specific expression of FKBP10 in CAFs may actuate tumor invasion and migration through multiple pathways.
As a key component of the TME, the ECM not only supports cell adhesion and migration, but also regulates angiogenesis and immune factor activation by integrin family signaling (48,49). Excessive synthesis and deposition of ECM proteins by CAFs is the characteristic of cancer-associated stroma (42). In the current study, we unexpectedly found that FKBP10 and FCGs may affect collagen synthesis and secretion by iCAFs, which was the first exploration in the field of TME. Previous researches demonstrated that the deletion of FKBP10 in pulmonary fibroblasts, dermal fibroblasts, and bone could diminish cross-linking and secretion of collagen, which offers a potent proof for our findings (13-17). There is accumulating evidence that collagen is overexpressed in a variety of cancers and has a profound impact on tumor progression. Increasing the length of collagen can prolong the migration distance of breast cancer cells and fortify the hardness of the ECM, thereby accelerating cancer invasion (50). In squamous cell carcinoma of the head and neck, adenocarcinoma of the esophagus, and colorectal cancer, extended collagen fibers are associated with undesirable clinical outcome (51). Additionally, a recent study manifested that increases in the density, length, and width of collagen fibers could predict adverse outcomes in patients with gastric cancer (8). To sum up, we provide a novel perspective on how iCAF-expressed FKBP10 performs its biological function in the TME.
Explanations of findings & implications and actions needed
A synthetical understanding of the interactions between cancer cells and the immune system could contribute to the exploitation of innovative strategies for cancer treatment (52-54). Evading immune surveillance is one of the emerging features of cancer (55). On the one hand, when normal cells develop into malignant cells, cancer cells continue to evade anticancer immune responses and form tumors, on the other hand, immune surveillance by immune cells within the TME enables the immune system to identify potentially hazardous tumors in the body to impose restrictions on the occurrence and progression of tumors. To interrupt immune evasion, agents targeting ICB have revealed considerable clinical gains in metastatic melanoma, non-small cell lung cancer, and metastatic kidney cancer (56-58). At the same time, several prospective trials have demonstrated that ICB therapy provides an unprecedented survival benefit for patients with advanced gastric cancer (59-62). Although the efficacy of ICB therapy has been recognized, limited response rates have been found in clinical applications, which means that the identification of biomarkers that can predict the effectiveness of immunotherapy in cancer patients is imminent. In this study, we inputted nine prognosis-related FCGs (FKBP10, BCAT1, FNDC3B, SEC23A, CCND1, INHBA, ADAM10, TCEAL9, and COL5A2) obtained from hdWGCNA into the NMF algorithm, identified two clusters in the TCGA-STAD cohort, and the ICB therapy response ratio of cluster 1 was predicted by the TIDE algorithm to be higher. The function of several FCGs (FKBP10, BCAT1, CCND1, INHBA, ADAM10, and COL5A2) in gastric cancer progression has been confirmed (63-67). This is the first time to exposit these FCGs and immunotherapy in gastric cancer, which provides an integrated index for predicting the efficacy of ICB therapy in gastric cancer patients.
Conclusions
In brief, by integrating single-cell sequencing data with bulk sequencing data, our study reveals the characteristics and function of FKBP10 in the TME, promotes the understanding of cell-specific FKBP10-dependent biological alterations in human gastric cancer, and renders available clues to guide ICB therapy decisions.
Acknowledgments
Funding: This research was sponsored by
Footnote
Reporting Checklist: The authors have completed the MDAR reporting checklist. Available at https://tcr.amegroups.com/article/view/10.21037/tcr-23-1484/rc
Peer Review File: Available at https://tcr.amegroups.com/article/view/10.21037/tcr-23-1484/prf
Conflicts of Interest: All authors have completed the ICMJE uniform disclosure form (available at https://tcr.amegroups.com/article/view/10.21037/tcr-23-1484/coif). The authors have no conflicts of interest to declare.
Ethical Statement: The authors are accountable for all aspects of the work in ensuring that questions related to the accuracy or integrity of any part of the work are appropriately investigated and resolved. The study was conducted in accordance with the Declaration of Helsinki (as revised in 2013).
Open Access Statement: This is an Open Access article distributed in accordance with the Creative Commons Attribution-NonCommercial-NoDerivs 4.0 International License (CC BY-NC-ND 4.0), which permits the non-commercial replication and distribution of the article with the strict proviso that no changes or edits are made and the original work is properly cited (including links to both the formal publication through the relevant DOI and the license). See: https://creativecommons.org/licenses/by-nc-nd/4.0/.
References
- Cao Y, Liu H, Li H, et al. Association of O6-Methylguanine-DNA Methyltransferase Protein Expression With Postoperative Prognosis and Adjuvant Chemotherapeutic Benefits Among Patients With Stage II or III Gastric Cancer. JAMA Surg 2017;152:e173120. [Crossref] [PubMed]
- Cunningham D, Allum WH, Stenning SP, et al. Perioperative chemotherapy versus surgery alone for resectable gastroesophageal cancer. N Engl J Med 2006;355:11-20. [Crossref] [PubMed]
- Macdonald JS, Smalley SR, Benedetti J, et al. Chemoradiotherapy after surgery compared with surgery alone for adenocarcinoma of the stomach or gastroesophageal junction. N Engl J Med 2001;345:725-30. [Crossref] [PubMed]
- Sasako M, Sakuramoto S, Katai H, et al. Five-year outcomes of a randomized phase III trial comparing adjuvant chemotherapy with S-1 versus surgery alone in stage II or III gastric cancer. J Clin Oncol 2011;29:4387-93. [Crossref] [PubMed]
- Al-Batran SE, Homann N, Pauligk C, et al. Effect of Neoadjuvant Chemotherapy Followed by Surgical Resection on Survival in Patients With Limited Metastatic Gastric or Gastroesophageal Junction Cancer: The AIO-FLOT3 Trial. JAMA Oncol 2017;3:1237-44. [Crossref] [PubMed]
- Hohenberger P, Gretschel S. Gastric cancer. Lancet 2003;362:305-15. [Crossref] [PubMed]
- Provenzano PP, Inman DR, Eliceiri KW, et al. Collagen density promotes mammary tumor initiation and progression. BMC Med 2008;6:11. [Crossref] [PubMed]
- Zhou ZH, Ji CD, Xiao HL, et al. Reorganized Collagen in the Tumor Microenvironment of Gastric Cancer and Its Association with Prognosis. J Cancer 2017;8:1466-76. [Crossref] [PubMed]
- Lu P, Weaver VM, Werb Z. The extracellular matrix: a dynamic niche in cancer progression. J Cell Biol 2012;196:395-406. [Crossref] [PubMed]
- Pathak A, Kumar S. Transforming potential and matrix stiffness co-regulate confinement sensitivity of tumor cell migration. Integr Biol (Camb) 2013;5:1067-75. [Crossref] [PubMed]
- Tung JC, Barnes JM, Desai SR, et al. Tumor mechanics and metabolic dysfunction. Free Radic Biol Med 2015;79:269-80. [Crossref] [PubMed]
- Nyström H, Naredi P, Berglund A, et al. Liver-metastatic potential of colorectal cancer is related to the stromal composition of the tumour. Anticancer Res 2012;32:5183-91. [PubMed]
- Gjaltema RA, van der Stoel MM, Boersema M, et al. Disentangling mechanisms involved in collagen pyridinoline cross-linking: The immunophilin FKBP65 is critical for dimerization of lysyl hydroxylase 2. Proc Natl Acad Sci U S A 2016;113:7142-7. [Crossref] [PubMed]
- Lietman CD, Lim J, Grafe I, et al. Fkbp10 Deletion in Osteoblasts Leads to Qualitative Defects in Bone. J Bone Miner Res 2017;32:1354-67. [Crossref] [PubMed]
- Barnes AM, Cabral WA, Weis M, et al. Absence of FKBP10 in recessive type XI osteogenesis imperfecta leads to diminished collagen cross-linking and reduced collagen deposition in extracellular matrix. Hum Mutat 2012;33:1589-98. [Crossref] [PubMed]
- Knüppel L, Heinzelmann K, Lindner M, et al. FK506-binding protein 10 (FKBP10) regulates lung fibroblast migration via collagen VI synthesis. Respir Res 2018;19:67. [Crossref] [PubMed]
- Staab-Weijnitz CA, Fernandez IE, Knüppel L, et al. FK506-Binding Protein 10, a Potential Novel Drug Target for Idiopathic Pulmonary Fibrosis. Am J Respir Crit Care Med 2015;192:455-67. [Crossref] [PubMed]
- Cai HQ, Zhang MJ, Cheng ZJ, et al. FKBP10 promotes proliferation of glioma cells via activating AKT-CREB-PCNA axis. J Biomed Sci 2021;28:13. [Crossref] [PubMed]
- Liang L, Zhao K, Zhu JH, et al. Comprehensive evaluation of FKBP10 expression and its prognostic potential in gastric cancer. Oncol Rep 2019;42:615-28. [Crossref] [PubMed]
- Ge Y, Xu A, Zhang M, et al. FK506 Binding Protein 10 Is Overexpressed and Promotes Renal Cell Carcinoma. Urol Int 2017;98:169-76. [Crossref] [PubMed]
- Olesen SH, Christensen LL, Sørensen FB, et al. Human FK506 binding protein 65 is associated with colorectal cancer. Mol Cell Proteomics 2005;4:534-44. [Crossref] [PubMed]
- Ramadori G, Ioris RM, Villanyi Z, et al. FKBP10 Regulates Protein Translation to Sustain Lung Cancer Growth. Cell Rep 2020;30:3851-3863.e6. [Crossref] [PubMed]
- Balkwill FR, Capasso M, Hagemann T. The tumor microenvironment at a glance. J Cell Sci 2012;125:5591-6. [Crossref] [PubMed]
- Quail DF, Joyce JA. Microenvironmental regulation of tumor progression and metastasis. Nat Med 2013;19:1423-37. [Crossref] [PubMed]
- Berraondo P, Umansky V, Melero I. Changing the tumor microenvironment: new strategies for immunotherapy. Cancer Res 2012;72:5159-64. [Crossref] [PubMed]
- Jiang H, Yu D, Yang P, et al. Revealing the transcriptional heterogeneity of organ-specific metastasis in human gastric cancer using single-cell RNA Sequencing. Clin Transl Med 2022;12:e730. [Crossref] [PubMed]
- Zhang M, Hu S, Min M, et al. Dissecting transcriptional heterogeneity in primary gastric adenocarcinoma by single cell RNA sequencing. Gut 2021;70:464-75. [Crossref] [PubMed]
- Kumar V, Ramnarayanan K, Sundar R, et al. Single-Cell Atlas of Lineage States, Tumor Microenvironment, and Subtype-Specific Expression Programs in Gastric Cancer. Cancer Discov 2022;12:670-91. [Crossref] [PubMed]
- Jiang P, Gu S, Pan D, et al. Signatures of T cell dysfunction and exclusion predict cancer immunotherapy response. Nat Med 2018;24:1550-8. [Crossref] [PubMed]
- Liu Y, Dong Y, Wu X, et al. Identification of Immune Microenvironment Changes and the Expression of Immune-Related Genes in Liver Cirrhosis. Front Immunol 2022;13:918445. [Crossref] [PubMed]
- Gong LB, Zhang C, Yu RX, et al. FKBP10 Acts as a New Biomarker for Prognosis and Lymph Node Metastasis of Gastric Cancer by Bioinformatics Analysis and in Vitro Experiments. Onco Targets Ther 2020;13:7399-409. [Crossref] [PubMed]
- Wang RG, Zhang D, Zhao CH, et al. FKBP10 functioned as a cancer-promoting factor mediates cell proliferation, invasion, and migration via regulating PI3K signaling pathway in stomach adenocarcinoma. Kaohsiung J Med Sci 2020;36:311-7. [Crossref] [PubMed]
- Gajewski TF, Schreiber H, Fu YX. Innate and adaptive immune cells in the tumor microenvironment. Nat Immunol 2013;14:1014-22. [Crossref] [PubMed]
- de Visser KE, Eichten A, Coussens LM. Paradoxical roles of the immune system during cancer development. Nat Rev Cancer 2006;6:24-37. [Crossref] [PubMed]
- Kalluri R. The biology and function of fibroblasts in cancer. Nat Rev Cancer 2016;16:582-98. [Crossref] [PubMed]
- Tabuso M, Homer-Vanniasinkam S, Adya R, et al. Role of tissue microenvironment resident adipocytes in colon cancer. World J Gastroenterol 2017;23:5829-35. [Crossref] [PubMed]
- Patterson CE, Schaub T, Coleman EJ, et al. Developmental regulation of FKBP65. An ER-localized extracellular matrix binding-protein. Mol Biol Cell 2000;11:3925-35. [Crossref] [PubMed]
- Shiga K, Hara M, Nagasaki T, et al. Cancer-Associated Fibroblasts: Their Characteristics and Their Roles in Tumor Growth. Cancers (Basel) 2015;7:2443-58. [Crossref] [PubMed]
- Quante M, Tu SP, Tomita H, et al. Bone marrow-derived myofibroblasts contribute to the mesenchymal stem cell niche and promote tumor growth. Cancer Cell 2011;19:257-72. [Crossref] [PubMed]
- Coussens LM, Werb Z. Inflammation and cancer. Nature 2002;420:860-7. [Crossref] [PubMed]
- Houghton J, Wang TC. Helicobacter pylori and gastric cancer: a new paradigm for inflammation-associated epithelial cancers. Gastroenterology 2005;128:1567-78. [Crossref] [PubMed]
- Kalluri R, Zeisberg M. Fibroblasts in cancer. Nat Rev Cancer 2006;6:392-401. [Crossref] [PubMed]
- Orimo A, Weinberg RA. Stromal fibroblasts in cancer: a novel tumor-promoting cell type. Cell Cycle 2006;5:1597-601. [Crossref] [PubMed]
- Attieh Y, Vignjevic DM. The hallmarks of CAFs in cancer invasion. Eur J Cell Biol 2016;95:493-502. [Crossref] [PubMed]
- Allinen M, Beroukhim R, Cai L, et al. Molecular characterization of the tumor microenvironment in breast cancer. Cancer Cell 2004;6:17-32. [Crossref] [PubMed]
- Hayward SW, Wang Y, Cao M, et al. Malignant transformation in a nontumorigenic human prostatic epithelial cell line. Cancer Res 2001;61:8135-42. [PubMed]
- Labernadie A, Kato T, Brugués A, et al. A mechanically active heterotypic E-cadherin/N-cadherin adhesion enables fibroblasts to drive cancer cell invasion. Nat Cell Biol 2017;19:224-37. [Crossref] [PubMed]
- Hynes RO, Naba A. Overview of the matrisome--an inventory of extracellular matrix constituents and functions. Cold Spring Harb Perspect Biol 2012;4:a004903. [Crossref] [PubMed]
- Hynes RO. The extracellular matrix: not just pretty fibrils. Science 2009;326:1216-9. [Crossref] [PubMed]
- Insua-Rodríguez J, Oskarsson T. The extracellular matrix in breast cancer. Adv Drug Deliv Rev 2016;97:41-55. [Crossref] [PubMed]
- Hanley CJ, Noble F, Ward M, et al. A subset of myofibroblastic cancer-associated fibroblasts regulate collagen fiber elongation, which is prognostic in multiple cancers. Oncotarget 2016;7:6159-74. [Crossref] [PubMed]
- Dong H, Strome SE, Salomao DR, et al. Tumor-associated B7-H1 promotes T-cell apoptosis: a potential mechanism of immune evasion. Nat Med 2002;8:793-800. [Crossref] [PubMed]
- Wang E, Monaco A, Monsurró V, et al. Antitumor vaccines, immunotherapy and the immunological constant of rejection. IDrugs 2009;12:297-301. [PubMed]
- Leach DR, Krummel MF, Allison JP. Enhancement of antitumor immunity by CTLA-4 blockade. Science 1996;271:1734-6. [Crossref] [PubMed]
- Hanahan D, Weinberg RA. Hallmarks of cancer: the next generation. Cell 2011;144:646-74. [Crossref] [PubMed]
- Hodi FS, O'Day SJ, McDermott DF, et al. Improved survival with ipilimumab in patients with metastatic melanoma. N Engl J Med 2010;363:711-23. [Crossref] [PubMed]
- Topalian SL, Hodi FS, Brahmer JR, et al. Safety, activity, and immune correlates of anti-PD-1 antibody in cancer. N Engl J Med 2012;366:2443-54. [Crossref] [PubMed]
- Choueiri TK, Escudier B, Powles T, et al. Cabozantinib versus Everolimus in Advanced Renal-Cell Carcinoma. N Engl J Med 2015;373:1814-23. [Crossref] [PubMed]
- Kang YK, Boku N, Satoh T, et al. Nivolumab in patients with advanced gastric or gastro-oesophageal junction cancer refractory to, or intolerant of, at least two previous chemotherapy regimens (ONO-4538-12, ATTRACTION-2): a randomised, double-blind, placebo-controlled, phase 3 trial. Lancet 2017;390:2461-71. [Crossref] [PubMed]
- Shitara K, Özgüroğlu M, Bang YJ, et al. Pembrolizumab versus paclitaxel for previously treated, advanced gastric or gastro-oesophageal junction cancer (KEYNOTE-061): a randomised, open-label, controlled, phase 3 trial. Lancet 2018;392:123-33. [Crossref] [PubMed]
- Fuchs CS, Doi T, Jang RW, et al. Safety and Efficacy of Pembrolizumab Monotherapy in Patients With Previously Treated Advanced Gastric and Gastroesophageal Junction Cancer: Phase 2 Clinical KEYNOTE-059 Trial. JAMA Oncol 2018;4:e180013. [Crossref] [PubMed]
- Bang YJ, Kang YK, Catenacci DV, et al. Pembrolizumab alone or in combination with chemotherapy as first-line therapy for patients with advanced gastric or gastroesophageal junction adenocarcinoma: results from the phase II nonrandomized KEYNOTE-059 study. Gastric Cancer 2019;22:828-37. [Crossref] [PubMed]
- Shu X, Zhan PP, Sun LX, et al. BCAT1 Activates PI3K/AKT/mTOR Pathway and Contributes to the Angiogenesis and Tumorigenicity of Gastric Cancer. Front Cell Dev Biol 2021;9:659260. [Crossref] [PubMed]
- Wang J, Zhang M, Hu X, et al. miRNA-194 predicts favorable prognosis in gastric cancer and inhibits gastric cancer cell growth by targeting CCND1. FEBS Open Bio 2021;11:1814-26. [Crossref] [PubMed]
- Qiu S, Li B, Xia Y, et al. CircTHBS1 drives gastric cancer progression by increasing INHBA mRNA expression and stability in a ceRNA- and RBP-dependent manner. Cell Death Dis 2022;13:266. [Crossref] [PubMed]
- Wang Y, Zhou N, Li P, et al. EphA8 acts as an oncogene and contributes to poor prognosis in gastric cancer via regulation of ADAM10. J Cell Physiol 2019;234:20408-19. [Crossref] [PubMed]
- Tan Y, Chen Q, Xing Y, et al. High expression of COL5A2, a member of COL5 family, indicates the poor survival and facilitates cell migration in gastric cancer. Biosci Rep 2021;41:BSR20204293. [Crossref] [PubMed]