Identification and verification of anoikis-related gene markers to predict the prognosis of patients with bladder cancer and assist in the diagnosis and treatment of bladder cancer
Highlight box
Key findings
• A prognostic model based on anoikis-related genes was established to stably predict the prognosis of bladder cancer patients.
What is known and what is new?
• Anoikis affects various processes of tumor progression, but few studies have explored the impact of anoikis-related genes on the prognosis of bladder cancer patients.
• Based on anoikis-related genes, a prediction model for the prognosis of bladder cancer patients was established and validated.
What is the implication, and what should change now?
• This model can predict the prognosis of bladder cancer patients. In addition, immune-related analysis and drug susceptibility analysis are helpful for personalized treatment of bladder cancer patients.
Introduction
The recurrence rate and mortality rate of bladder cancer are extremely high, because smoking and other factors make men to be four-time more vulnerable to bladder cancer than women (1,2). Of patients who had undergone surgery still face relapse after the surgery. early surgical treatment is still advocated to prolong survival (3). Unfortunately, up to 17% of surgical patients still relapse after surgery. The most effective methods for recurrent or metastatic bladder cancer are chemotherapy and immunotherapy (4,5). However, the 5-year survival rate and median survival of bladder cancer are still difficult to achieve satisfactory results (6-8). With the development of bioinformatics technology, more and more literature shows that genes can be used as biomarkers for prognosis prediction of bladder cancer (9-13).
Anoikis is an important physiological process in the body, which helps clean up aging and abnormal cells, maintain vitality and maintain homeostasis (14). Anoikis (Greek for “homeless”) is a type of apoptosis (15,16), Its function is mainly to remove abnormally adherent cells (17). When the malignant tumor metastasis, it will trigger anoikis, and kill the displaced malignant tumor cells (18). In addition, related studies have also found that in diabetes, cardiovascular disease and infectious diseases, it can also trigger anoikis, remove abnormal cells, and maintain homeostasis (19,20). At present, it has been found that tumor cells can achieve distant metastasis of tumors through the resistance mechanism of anoikis (21). No related studies have shown that the mechanism of apoptosis plays a key role in lung cancer and breast cancer metastasis (22,23). However, there have been no studies in bladder cancer.
Through previous studies, we have found that the mechanism of anoikis is closely related to the development of tumors. Combined with bioinformatics, the relationship between anoikis-related genes and bladder cancer is expected to offer new diagnostic indicators and provide reference for clinicians to make diagnosis and treatment plans. In this study, we constructed a prognostic model of bladder cancer anoikis-related genes by bioinformatics techniques. Integrating the scoring of the model formula with the clinical information, we created a nomogram. By using nomograms, accurate results for survival rates for bladder cancer patients can be easily calculated. We present this article in accordance with the TRIPOD reporting checklist (available at https://tcr.amegroups.com/article/view/10.21037/tcr-23-1770/rc).
Methods
Acquisition of data
The study was conducted in accordance with the Declaration of Helsinki (as revised in 2013). We downloaded raw bladder cancer data from The Cancer Genome Atlas (TCGA) database and Gene Expression Omnibus (GEO) database through formal channels. Data were organized through R (4.2.1) and Perl (Strawberry Edition). TCGA data and GEO data were normalized using SVA packages to remove batch effects. The external validation data were from the GSE32894 dataset of the GEO database. The immunohistochemistry (IHC) results of the model-associated genes were queried by the HPAanalyze The GeneCards website was used to obtain anoikis-related genes (https://www.genecards.org/).
Screening of prognosis-related differential genes
We searched through the GeneCards website and obtained 801 anoikis-related genes. The differential gene was obtained via the limma package [false discovery rate (FDR) <0.05, |log fold change (FC)| >0.585] of R software (4.2.2). The differential genes obtained from the screening were used for univariate independent prognostic analysis (P<0.05). Through a series of screenings, we obtained prognostically relevant differential genes.
Model construction and validation
Least absolute shrinkage and selection operator (LASSO) regression was used to screen model-associated genes from prognosis-related differential genes. By cross-validation, we obtained the genes that are best suited for model building Cox regression model (COX) regression is used to build the model and output model formulas. All included bladder cancer samples were scored by model formula. All included bladder cancer patients were randomly divided into two equal parts by the createDataPartition function in the caret package, half as the Train group (the median risk score of the Train group patients were divided into high and low risk groups) and half as the Test group (the Test group was used for internal validation). Patients GSE32894 dataset were used for external validation of the model. Then, the accuracy of the model is tested by progression-free survival analysis and receiver operating characteristic (ROC) curves. Univariate and multivariate independent prognostic analysis was then used to assess whether the risk score could be used as an independent prognostic factor.
Multiomic analysis of model-related genes
Multiomics data is used for deeper mining of model-related genes. First, copies of model-related genes were obtained from the original data using Perl (strawberry version). The copy data of the model-related genes were then visualized by histogram and circle chart. Then the waterfall plot is used to show the mutation status of the model-related gene, and the heat map is used to show the co-mutation of the model-related gene. Finally, in order to screen for more valuable biomarkers from nine model-related genes, we first screened genes with more than 3-fold differences in expression in tumor and normal tissues by differential analysis (|logFC| >1.5). Next, we used the HPAanalyze package to query the IHC of the differential genes in the Human Protein Atlas (HPA) database.
Construction and accuracy verification of nomograms
Linemaps were constructed to more accurately predict survival in bladder cancer patients. First, we integrated the patient’s risk score with common clinical information to construct a nomogram, and used the ninth bladder cancer patient in the TCGA-bladder cancer cohort as an example to demonstrate the use of nomogram. Next, we use the calibration curve to verify the reliability of the nomogram by comparing the survival rate of actual patients with the nomogram prediction of patient survival. Independent prognostic analysis is used to see if nomograms can be used as an independent factor in predicting prognosis in patients with bladder cancer. By plotting the ROC curve to observe the area under the curve, we can further understand the ability of nomogram to predict prognosis. The decision curve analysis (DCA) curve is also used to confirm the accuracy of the prediction nomogram prediction results.
Pathway enrichment analysis
Pathway enrichment analysis was used to gain a deeper understanding of the mechanism underlying the onset and progression of bladder cancer. First, gene set enrichment analysis (GSEA) and gene set variation analysis (GSVA) analyses were performed to observe the enrichment of patient pathways across different risk groups. The gene expressions of patients in distinct risk groups were then subjected to differential analysis. Based on the findings of differential analysis, conventional pathway enrichment analysis was performed on differential genes.
Immune correlation analysis of patients at different risk levels
To develop a more accurate treatment plan, the correlation between immune cell infiltration and risk scores was investigated, followed by additional research into the sensitivity of immunotherapy. First, the expression levels of 47 genes associated with immune checkpoints in various groups were analyzed. By analyzing the tumor microenvironment, we had a general understanding of the infiltration of immune cells and matrix cells surrounding the tumor tissue. The correlation between the filtration of immune cells surrounding tumor tissue and the risk scores was then analyzed on existing immunoassay platforms. Subsequently, the sensitivity of patients in various risk groups to immunotherapy was predicted using Tumor Immune Dysfunction and Exclusion (TIDE) websites.
Drug sensitivity analysis
The oncoPredict package was utilized for drug sensitivity analysis, allowing for targeted drug administration based on the results of the analysis.
Statistical analysis
The statistical analysis was performed using R software (version 4.1.2). The chi-square test was used to analyse differences in patients, with Kaplan-Meier analysis and log-rank analysis used to assess patients’ overall survival (OS) and progression-free survival (PFS). The Wilcoxon test was used to check the deviation between the components. A P value <0.05 was considered statistically significant.
Results
A total of 146 prognosis-related differential genes were obtained
The process flowchart of this study depicts the entire process of this study (Figure 1). Differential analysis gave a total of 635 genes differentially expressed in tumor and normal tissues. Then, 313 genes with significant differences in expression based on |logFC| >0.585 and FDR <0.05 were obtained and displayed using heat map and volcano map (Figure S1). An independent univariate prognostic analysis was conducted on the 313 differential genes, and 146 prognosis-related differential genes were subsequently screened from these genes.
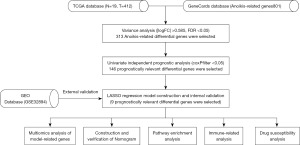
Construction of prognostic scoring model and validation of accuracy
The 146 differential prognosis-related genes were used for LASSO regression (Figure 2A). Cross-validation results indicated that the scoring model constructed with 2–15 genes contained the fewest errors (Figure 2B). After a series of screenings, 9 genes (KLF12, INHBB, CASP6, TGFBR3, FASN, TPM1, OGT, RAC3, and ID4) were utilized in the model’s construction, and the model’s formula was output. Risk score = EXP[(KLF12*0.38) + (INHBB*0.30) + (CASP6*−0.38) + (TGFBR3*−0.15) + (FASN*0.49) + (TPM1*0.24) + (OGT*−0.53) + (RAC3*0.23) + (ID4*−0.22)] (the result of risk coefficient was rounded up to two decimal places). This formula was applied to all patients included in this study, and the median risk score of patients in the Train group was used to stratify these patients. Principal component analysis (PCA) demonstrated that the risk score effectively distinguished between patients belonging to distinct risk groups (Figure 2C). Results from both univariate and multivariate independent prognostic analyses indicated that the risk score was an independent factor unaffected by other variables (Figure 2D,2E). The model’s accuracy could be assessed in greater detail using the survival curve and ROC curve. The survival analysis results of the entire cohort, the Train cohort, and the Test cohort revealed that there were significant differences in survival between different risk groups (P<0.001), and that the area under the curve (AUC) of 1-, 3-, and 5-year survival were all greater than 0.65 (Figure 3A-3C). External data were utilized to validate the accuracy of this model’s predictions (Figure 3D,3E). In the multivariate ROC curve, we discovered that the risk score had the highest prediction accuracy (Figure 3F).
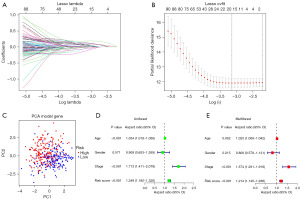
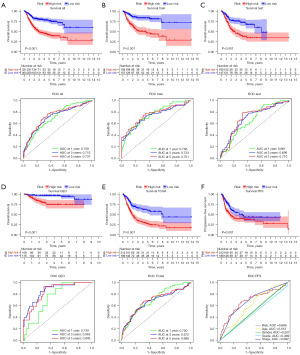
Multiomics analysis of model-related genes
Analysis of the model-related genes’ copy number revealed that approximately 88% of model-related genes increased, while the copy number of TGFBR3 decreased (Figure 4A). TGFBR3 was located on chromosome 1, while the circle plot displayed the locations of other model-related genes (Figure 4B). From the analysis of the mutation data of model-related genes, about 55% of model-related genes were confirmed to have mutations (FASN had the highest mutation frequency), and nearly all the mutations were missense mutations (Figure 4C). Four genes screened were found to be significantly differentially expressed between tumor and normal tissues (FASN, RAC3, TPM1, and TGFBR3) (Figure 4D). We found consistency between the IHC results of FASN, RAC3, and TGFBR3 and the gene expression results by analyzing the statistical results, but no significant differences between the IHC results of TPM1 in normal tissue and tumor tissue. Then, the detailed IHC of FASN, RAC3, and TGFBR3 was displayed (Figure 4E).
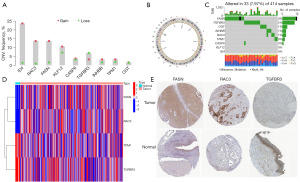
Construction and verification of nomogram
To accurately predict the survival rate of patients with bladder cancer, a nomogram was developed by integrating risk scores with clinical data, and the method of using the nomogram was demonstrated in the ninth patient of the cohort (Figure 5A). The calibration curve revealed that the nomogram’s prediction results were almost identical to the actual results (Figure 5B). Independent univariate and multivariate prognostic analysis confirmed that the predictive ability of the nomogram was unaffected by other variables (Figure 5C,5D). The largest AUC (AUC =0.757) was observed for the nomogram in the ROC curve, indicating that the nomogram had superior prediction effect (Figure 5E); the DCA curve also confirmed the accuracy of the nomogram’s prediction results (Figure 5F).
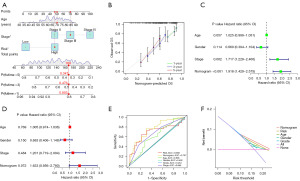
Pathway enrichment analysis
Metabolic pathways were enriched in the low-risk group, whereas tumor-related pathways were enriched in the high-risk group. GSEA analysis revealed that pathways including ascorbate-and-aldarate, drug-metabolism-cytochrome-p450, metabolism-of-xenobiotics-by cytochrome-p45, pentose-and-glucuronate-interconversions, and retinol-metabolism were enriched in the low-risk group (Figure 6A). Several pathways were enriched in the high-risk group, including cell-adhesion-molecules-cams, cytokine-cytokine-receptor-interaction, extracellular matrix (ECM)-receptor-interaction, focal-adhesion, and regulation-of-actin-cytoskeleton (Figure 6B). According to GSEA analysis, cellular-hormone-metabolic-process, cellular-response-to-xenobiotic-stimulus, monocarboxylic-acid-catabolic-process, xenobiotic-catabolic-process, and xenobiotic-metabolic-process were all associated with biological processes (Figure 6C). Interestingly, the majority of enriched pathways in the high-risk group were associated with cellular components (Figure 6D).
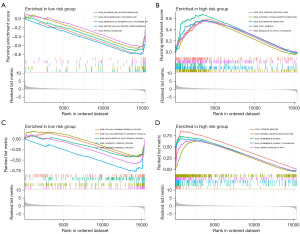
Patients with lower risk scores were more suitable to receive immunotherapy
First, the differential analysis of immune checkpoint-related genes revealed that 76% of immune checkpoint-related genes were significantly expressed differently in patients from different risk groups (Figure S2). Following this, tumor microenvironment analysis revealed statistically significant differences in the filtration of ECM and immune cells between risk groups (Figure 7A). The analysis of seven platforms (TIMER, CIBERSORT, CIBERSORTABS, XCELL, QUANTISEQ, EPIC, and MCP-counter) led to the following generalization: the correlation coefficient of 54% of immune cells with the model score, was greater than 0, and 46% were negatively correlated with the risk scores (Figure 7B). We reached the same conclusion with the TIDE platform’s analysis results (Figure 7C).
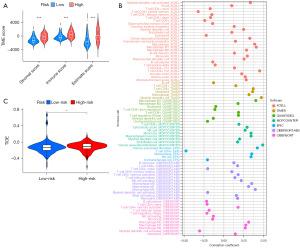
Patients with higher risk scores were more sensitive to common chemotherapeutic drugs
The results of the drug sensitivity analysis revealed that patients with higher risk scores were more sensitive to common chemotherapeutic drugs (foretinib, taselisib, cisplatin, staurosporine, trametinib, dasatinib, entospletinib, talazoparib, and 5-fluorouracil) (Figure 8). These results could help in providing more precise treatments for patients with bladder cancer at different risk levels.
Discussion
Bladder cancer is a significant threat to public health and safety. Previous research has demonstrated a strong link between anoikis and tumor progression. With the rapidly expanding of the bioinformatics field, we utilized anoikis-related genes in this study to identify significant biomarkers. Using LASSO regression, a stable prognosis model was established, and a nomogram was created to accurately predict the survival rate of patients with bladder cancer. We screened nine model-related genes in the present study, including KLF12, INHBB, CASP6, TGFBR3, FASN, TPM1, OGT, RAC3, and ID4.
Study indicates that CASP6 is primarily responsible for encoding the Caspase-6 protein, and its expression is negatively correlated with tumor development (24). In this study, we also discovered that patients with bladder cancer who expressed a greater number of CASP6 genes had a better prognosis. ID4, a negative transcription factor, was linked to poor breast cancer prognosis and metastasis as early as 2005 (25). ID4 expression was shown to be higher in non-invasive bladder cancer but lower in invasive bladder cancer, according a study published in 2018 (26). In other words, the higher the ID4 expression, the better the prognosis of the patient, which was confirmed in our study. INHBB is a heterodimer glycoprotein belonging to the transforming growth factor β (TGFβ) family (27). The expression of INHBB in tumors is not completely consistent. Few studies have discovered reduced expressions of INHBB in renal cell carcinoma, pancreatic cancer, and lung cancer (28,29). And one study even considers it to be a tumor-inhibiting factor (30). Despite this, a large number of studies have demonstrated elevated INHBB expression in rectal cancer, endometrial cancer, adrenocortical carcinoma, oral squamous cell carcinoma, and placental tumor (28,31,32). In this study, we discovered that high INHBB expression was associated with a poor prognosis in patients with bladder cancer; similar findings have been reported in other study on gastric cancer (33). KLF12 is a member of the Krüppel-like factors (KLFs) family (34). Some studies found that KLF12 was abnormally expressed in a variety of tumors (35-37). In addition, KLF12 plays a crucial role in the occurrence and progression of bladder cancer (38,39). Multiple tumors, including lung cancer, liver cancer, and prostate cancer, have been shown to express OGT at high levels (40-42). Rozanski et al. reported in 2012 that OGT mRNA expression was detected in the urine of patients with bladder cancer, with the expression level correlating to the malignancy of bladder cancer (43). In 2018, Wang et al. knocked out OGT and discovered that bladder cancer cells were more sensitive to chemotherapeutic drugs (44). In recent years, emphasis has been placed on the role of OGT in bladder cancer, and numerous studies have again confirmed the role of OGT in the occurrence and progression of bladder cancer (45,46). In 2022, Lee et al. experimentally confirmed that OGT expression in patients with bladder cancer could be considered a potential therapeutic target (47). TGFBR3 inhibits the development of tumors such as renal cancer, lung cancer, and liver cancer, and has been considered a tumor-inhibiting factor (48-50). Encouragingly, Chen et al. discovered in 2022 that TGFBR3 was negatively correlated with the progression and metastasis of bladder cancer (51). The results of our study confirmed this conclusion. TPM1, a type of tropomyosin (52,53), is abundant in numerous cell types (54). One study indicates that TPM1—a cancer suppressor gene—is expressed at low levels in a variety of tumor cells (55). The findings regarding this gene and bladder cancer are encouraging.
FASN is essential for the development and occurrence of tumors; 95% of fatty acids in tumor cells are synthesized by FASN (56,57). FASN, an important gene associated with tumors, is detectable in numerous tumor cells (58-60). FASN overexpression is associated with a poor prognosis in patients with bladder cancer; when FASN expression is inhibited, tumor metastasis and recurrence may be prevented, and patients’ sensitivity to chemotherapeutic drugs may be improved (61-65). This suggests that FASN will likely provide a new treatment target for bladder cancer as the subject of additional research. Multiple tumors are associated with RAC3, a member of the Rho GTP enzyme subfamily (66-68). Its high expression in patients with bladder cancer is frequently correlated with poor prognosis (69). Recent research indicates that RAC3 can predict the prognosis of patients with bladder cancer (70). Multiple findings indicate that additional research on RAC3 may produce unexpected results.
In recent years, numerous studies on tumor immunotherapy have been conducted. The results of this study revealed that immunotherapy may be more appropriate for low-risk group patients with bladder cancer. The correlation analysis revealed that risk scores were negatively correlated with T-cells and positively correlated with M2 macrophages. Previous research has shown that a high level of M2 macrophage infiltration is associated with a poor prognosis, while a low level of T-cell infiltration may result in tumor cells that are resistant to immunotherapy (71,72). This is consistent with our findings.
Conclusions
In this study, we constructed a model that can accurately predict the prognosis of patients with bladder cancer. Low-risk patients are more likely to benefit from immunotherapy, whereas high-risk patients are more susceptible to chemotherapeutic drugs.
Acknowledgments
We sincerely thank public databases such as TCGA and GEO for providing platforms and meaningful data.
Funding: None.
Footnote
Reporting Checklist: The authors have completed the TRIPOD reporting checklist. Available at https://tcr.amegroups.com/article/view/10.21037/tcr-23-1770/rc
Peer Review File: Available at https://tcr.amegroups.com/article/view/10.21037/tcr-23-1770/prf
Conflicts of Interest: All authors have completed the ICMJE uniform disclosure form (available at https://tcr.amegroups.com/article/view/10.21037/tcr-23-1770/coif). The authors have no conflicts of interest to declare.
Ethical Statement: The authors are accountable for all aspects of the work in ensuring that questions related to the accuracy or integrity of any part of the work are appropriately investigated and resolved. The study was conducted in accordance with the Declaration of Helsinki (as revised in 2013).
Open Access Statement: This is an Open Access article distributed in accordance with the Creative Commons Attribution-NonCommercial-NoDerivs 4.0 International License (CC BY-NC-ND 4.0), which permits the non-commercial replication and distribution of the article with the strict proviso that no changes or edits are made and the original work is properly cited (including links to both the formal publication through the relevant DOI and the license). See: https://creativecommons.org/licenses/by-nc-nd/4.0/.
References
- Sung H, Ferlay J, Siegel RL, et al. Global Cancer Statistics 2020: GLOBOCAN Estimates of Incidence and Mortality Worldwide for 36 Cancers in 185 Countries. CA Cancer J Clin 2021;71:209-49. [Crossref] [PubMed]
- Siegel RL, Miller KD, Fuchs HE, et al. Cancer Statistics, 2021. CA Cancer J Clin 2021;71:7-33. [Crossref] [PubMed]
- Lenis AT, Lec PM, Chamie K, et al. Bladder Cancer: A Review. JAMA 2020;324:1980-91. [Crossref] [PubMed]
- Seiler R, Ashab HAD, Erho N, et al. Impact of Molecular Subtypes in Muscle-invasive Bladder Cancer on Predicting Response and Survival after Neoadjuvant Chemotherapy. Eur Urol 2017;72:544-54. [Crossref] [PubMed]
- Powles T, Eder JP, Fine GD, et al. MPDL3280A (anti-PD-L1) treatment leads to clinical activity in metastatic bladder cancer. Nature 2014;515:558-62. [Crossref] [PubMed]
- Butt SU, Malik L. Role of immunotherapy in bladder cancer: past, present and future. Cancer Chemother Pharmacol 2018;81:629-45. [Crossref] [PubMed]
- Wen J, Yang T, Mallouk N, et al. Urinary Exosomal CA9 mRNA as a Novel Liquid Biopsy for Molecular Diagnosis of Bladder Cancer. Int J Nanomedicine 2021;16:4805-11. [Crossref] [PubMed]
- Pond GR, Agarwal A, Ornstein M, et al. Clinical Outcomes of Platinum-ineligible Patients with Advanced Urothelial Carcinoma Treated With First-line PD1/L1 Inhibitors. Clin Genitourin Cancer 2021;19:425-33. [Crossref] [PubMed]
- Zhu K, Liu X, Deng W, et al. Identification of a chromatin regulator signature and potential candidate drugs for bladder cancer. Hereditas 2022;159:13. [Crossref] [PubMed]
- Zhang Y, Xie Y, Feng Y, et al. Construction and verification of a prognostic risk model based on immunogenomic landscape analysis of bladder caner. Gene 2022;808:145966. [Crossref] [PubMed]
- Peng S, Ma S, Yang F, et al. Prognostic value and underlying mechanism of autophagy-related genes in bladder cancer. Sci Rep 2022;12:2219. [Crossref] [PubMed]
- Lu H, Wu J, Liang L, et al. Identifying a Novel Defined Pyroptosis-Associated Long Noncoding RNA Signature Contributes to Predicting Prognosis and Tumor Microenvironment of Bladder Cancer. Front Immunol 2022;13:803355. [Crossref] [PubMed]
- Jiang Y, Zeng Z, Xiong S, et al. New Prognostic Gene Signature and Immune Escape Mechanisms of Bladder Cancer. Front Cell Dev Biol 2022;10:775417. [Crossref] [PubMed]
- Green DR, Llambi F. Cell Death Signaling. Cold Spring Harb Perspect Biol 2015;7:a006080. [Crossref] [PubMed]
- Buchheit CL, Weigel KJ, Schafer ZT. Cancer cell survival during detachment from the ECM: multiple barriers to tumour progression. Nat Rev Cancer 2014;14:632-41. [Crossref] [PubMed]
- Frisch SM, Francis H. Disruption of epithelial cell-matrix interactions induces apoptosis. J Cell Biol 1994;124:619-26. [Crossref] [PubMed]
- Paoli P, Giannoni E, Chiarugi P. Anoikis molecular pathways and its role in cancer progression. Biochim Biophys Acta 2013;1833:3481-98. [Crossref] [PubMed]
- Kim YN, Koo KH, Sung JY, et al. Anoikis resistance: an essential prerequisite for tumor metastasis. Int J Cell Biol 2012;2012:306879. [Crossref] [PubMed]
- Haun F, Neumann S, Peintner L, et al. Identification of a novel anoikis signalling pathway using the fungal virulence factor gliotoxin. Nat Commun 2018;9:3524. [Crossref] [PubMed]
- Taddei ML, Giannoni E, Fiaschi T, et al. Anoikis: an emerging hallmark in health and diseases. J Pathol 2012;226:380-93. [Crossref] [PubMed]
- Zhang Y, Donaher JL, Das S, et al. Genome-wide CRISPR screen identifies PRC2 and KMT2D-COMPASS as regulators of distinct EMT trajectories that contribute differentially to metastasis. Nat Cell Biol 2022;24:554-64. [Crossref] [PubMed]
- Adeshakin FO, Adeshakin AO, Afolabi LO, et al. Mechanisms for Modulating Anoikis Resistance in Cancer and the Relevance of Metabolic Reprogramming. Front Oncol 2021;11:626577. [Crossref] [PubMed]
- Oudenaarden CRL, van de Ven RAH, Derksen PWB. Re-inforcing the cell death army in the fight against breast cancer. J Cell Sci 2018;131:jcs212563. [Crossref] [PubMed]
- Lee JW, Kim MR, Soung YH, et al. Mutational analysis of the CASP6 gene in colorectal and gastric carcinomas. APMIS 2006;114:646-50. [Crossref] [PubMed]
- Umetani N, Mori T, Koyanagi K, et al. Aberrant hypermethylation of ID4 gene promoter region increases risk of lymph node metastasis in T1 breast cancer. Oncogene 2005;24:4721-7. [Crossref] [PubMed]
- Morgan MJ, Fitzwalter BE, Owens CR, et al. Metastatic cells are preferentially vulnerable to lysosomal inhibition. Proc Natl Acad Sci U S A 2018;115:E8479-88. [Crossref] [PubMed]
- Zou G, Ren B, Liu Y, et al. Inhibin B suppresses anoikis resistance and migration through the transforming growth factor-β signaling pathway in nasopharyngeal carcinoma. Cancer Sci 2018;109:3416-27. [Crossref] [PubMed]
- Risbridger GP, Schmitt JF, Robertson DM. Activins and inhibins in endocrine and other tumors. Endocr Rev 2001;22:836-58. [Crossref] [PubMed]
- Boelens MC, van den Berg A, Fehrmann RS, et al. Current smoking-specific gene expression signature in normal bronchial epithelium is enhanced in squamous cell lung cancer. J Pathol 2009;218:182-91. [Crossref] [PubMed]
- Stenvers KL, Findlay JK. Inhibins: from reproductive hormones to tumor suppressors. Trends Endocrinol Metab 2010;21:174-80. [Crossref] [PubMed]
- Robertson DM, Burger HG, Fuller PJ. Inhibin/activin and ovarian cancer. Endocr Relat Cancer 2004;11:35-49. [Crossref] [PubMed]
- Kita A, Kasamatsu A, Nakashima D, et al. Activin B Regulates Adhesion, Invasiveness, and Migratory Activities in Oral Cancer: a Potential Biomarker for Metastasis. J Cancer 2017;8:2033-41. [Crossref] [PubMed]
- Yu W, He G, Zhang W, et al. INHBB is a novel prognostic biomarker and correlated with immune infiltrates in gastric cancer. Front Genet 2022;13:933862. [Crossref] [PubMed]
- Simmen RC, Heard ME, Simmen AM, et al. The Krüppel-like factors in female reproductive system pathologies. J Mol Endocrinol 2015;54:R89-R101. [Crossref] [PubMed]
- Kim SH, Park YY, Cho SN, et al. Krüppel-Like Factor 12 Promotes Colorectal Cancer Growth through Early Growth Response Protein 1. PLoS One 2016;11:e0159899. [Crossref] [PubMed]
- Wang J, Pu J, Zhang Y, et al. DANCR contributed to hepatocellular carcinoma malignancy via sponging miR-216a-5p and modulating KLF12. J Cell Physiol 2019;234:9408-16. [Crossref] [PubMed]
- Yuan J, Kang J, Yang M. Long non-coding RNA ELF3-antisense RNA 1 promotes osteosarcoma cell proliferation by upregulating Kruppel-like factor 12 potentially via methylation of the microRNA-205 gene. Oncol Lett 2020;19:2475-80. [Crossref] [PubMed]
- Guo Y, Chen D, Su X, et al. The lncRNA ELF3-AS1 promotes bladder cancer progression by interaction with Krüppel-like factor 8. Biochem Biophys Res Commun 2019;508:762-8. [Crossref] [PubMed]
- Tang C, Wang M, Dai Y, et al. Krüppel-like factor 12 suppresses bladder cancer growth through transcriptionally inhibition of enolase 2. Gene 2021;769:145338. [Crossref] [PubMed]
- Gu Y, Mi W, Ge Y, et al. GlcNAcylation plays an essential role in breast cancer metastasis. Cancer Res 2010;70:6344-51. [Crossref] [PubMed]
- Zhu Q, Zhou L, Yang Z, et al. O-GlcNAcylation plays a role in tumor recurrence of hepatocellular carcinoma following liver transplantation. Med Oncol 2012;29:985-93. [Crossref] [PubMed]
- Krześlak A, Wójcik-Krowiranda K, Forma E, et al. Expression of genes encoding for enzymes associated with O-GlcNAcylation in endometrial carcinomas: clinicopathologic correlations. Ginekol Pol 2012;83:22-6. [PubMed]
- Rozanski W, Krzeslak A, Forma E, et al. Prediction of bladder cancer based on urinary content of MGEA5 and OGT mRNA level. Clin Lab 2012;58:579-83. [PubMed]
- Wang L, Chen S, Zhang Z, et al. Suppressed OGT expression inhibits cell proliferation while inducing cell apoptosis in bladder cancer. BMC Cancer 2018;18:1141. [Crossref] [PubMed]
- Jin L, Yuan F, Dai G, et al. Blockage of O-linked GlcNAcylation induces AMPK-dependent autophagy in bladder cancer cells. Cell Mol Biol Lett 2020;25:17. [Crossref] [PubMed]
- Chen Y, Liu J, Zhang W, et al. O-GlcNAcylation Enhances NUSAP1 Stability and Promotes Bladder Cancer Aggressiveness. Onco Targets Ther 2021;14:445-54. [Crossref] [PubMed]
- Lee HW, Kang MJ, Kwon YJ, et al. Targeted Inhibition of O-Linked β-N-Acetylglucosamine Transferase as a Promising Therapeutic Strategy to Restore Chemosensitivity and Attenuate Aggressive Tumor Traits in Chemoresistant Urothelial Carcinoma of the Bladder. Biomedicines 2022;10:1162. [Crossref] [PubMed]
- Nishida J, Miyazono K, Ehata S. Decreased TGFBR3/betaglycan expression enhances the metastatic abilities of renal cell carcinoma cells through TGF-β-dependent and -independent mechanisms. Oncogene 2018;37:2197-212. [Crossref] [PubMed]
- Huang JJ, Corona AL, Dunn BP, et al. Increased type III TGF-β receptor shedding decreases tumorigenesis through induction of epithelial-to-mesenchymal transition. Oncogene 2019;38:3402-14. [Crossref] [PubMed]
- Zhang S, Sun WY, Wu JJ, et al. Decreased expression of the type III TGF-β receptor enhances metastasis and invasion in hepatocellullar carcinoma progression. Oncol Rep 2016;35:2373-81. [Crossref] [PubMed]
- Chen X, Wang P, Ou T, et al. KLF16 Downregulates the Expression of Tumor Suppressor Gene TGFBR3 to Promote Bladder Cancer Proliferation and Migration. Cancer Manag Res 2022;14:465-77. [Crossref] [PubMed]
- Liu G, Zhao X, Zhou J, et al. Long non-coding RNA MEG3 suppresses the development of bladder urothelial carcinoma by regulating miR-96 and TPM1. Cancer Biol Ther 2018;19:1039-56. [Crossref] [PubMed]
- Zheng Q, Safina A, Bakin AV. Role of high-molecular weight tropomyosins in TGF-beta-mediated control of cell motility. Int J Cancer 2008;122:78-90. [Crossref] [PubMed]
- Gunning P, O'Neill G, Hardeman E. Tropomyosin-based regulation of the actin cytoskeleton in time and space. Physiol Rev 2008;88:1-35. [Crossref] [PubMed]
- Wu H, Jiang W, Ji G, et al. Exploring microRNA target genes and identifying hub genes in bladder cancer based on bioinformatic analysis. BMC Urol 2021;21:90. [Crossref] [PubMed]
- Jones SF, Infante JR. Molecular Pathways: Fatty Acid Synthase. Clin Cancer Res 2015;21:5434-8. [Crossref] [PubMed]
- Ookhtens M, Kannan R, Lyon I, et al. Liver and adipose tissue contributions to newly formed fatty acids in an ascites tumor. Am J Physiol 1984;247:R146-53. [PubMed]
- Cai Y, Wang J, Zhang L, et al. Expressions of fatty acid synthase and HER2 are correlated with poor prognosis of ovarian cancer. Med Oncol 2015;32:391. [Crossref] [PubMed]
- Walter K, Hong SM, Nyhan S, et al. Serum fatty acid synthase as a marker of pancreatic neoplasia. Cancer Epidemiol Biomarkers Prev 2009;18:2380-5. [Crossref] [PubMed]
- Uddin S, Siraj AK, Al-Rasheed M, et al. Fatty acid synthase and AKT pathway signaling in a subset of papillary thyroid cancers. J Clin Endocrinol Metab 2008;93:4088-97. [Crossref] [PubMed]
- Humbert M, Seiler K, Mosimann S, et al. Reducing FASN expression sensitizes acute myeloid leukemia cells to differentiation therapy. Cell Death Differ 2021;28:2465-81. [Crossref] [PubMed]
- Gruslova A, McClellan B, Balinda HU, et al. FASN inhibition as a potential treatment for endocrine-resistant breast cancer. Breast Cancer Res Treat 2021;187:375-86. [Crossref] [PubMed]
- Shahid M, Kim M, Jin P, et al. S-Palmitoylation as a Functional Regulator of Proteins Associated with Cisplatin Resistance in Bladder Cancer. Int J Biol Sci 2020;16:2490-505. [Crossref] [PubMed]
- Tao T, Su Q, Xu S, et al. Down-regulation of PKM2 decreases FASN expression in bladder cancer cells through AKT/mTOR/SREBP-1c axis. J Cell Physiol 2019;234:3088-104. [Crossref] [PubMed]
- Abdelrahman AE, Rashed HE, Elkady E, et al. Fatty acid synthase, Her2/neu, and E2F1 as prognostic markers of progression in non-muscle invasive bladder cancer. Ann Diagn Pathol 2019;39:42-52. [Crossref] [PubMed]
- Lee CF, Carley RE, Butler CA, et al. Rac GTPase Signaling in Immune-Mediated Mechanisms of Atherosclerosis. Cells 2021;10:2808. [Crossref] [PubMed]
- Rosenberg BJ, Gil-Henn H, Mader CC, et al. Phosphorylated cortactin recruits Vav2 guanine nucleotide exchange factor to activate Rac3 and promote invadopodial function in invasive breast cancer cells. Mol Biol Cell 2017;28:1347-60. [Crossref] [PubMed]
- Hodge RG, Ridley AJ. Regulating Rho GTPases and their regulators. Nat Rev Mol Cell Biol 2016;17:496-510. [Crossref] [PubMed]
- Wang L, Shi J, Liu S, et al. RAC3 Inhibition Induces Autophagy to Impair Metastasis in Bladder Cancer Cells via the PI3K/AKT/mTOR Pathway. Front Oncol 2022;12:915240. [Crossref] [PubMed]
- Zheng W, Zhang J, Song Q, et al. Rac Family Small GTPase 3 Correlates with Progression and Poor Prognosis in Bladder Cancer. DNA Cell Biol 2021;40:469-81. [Crossref] [PubMed]
- Rizvi NA, Hellmann MD, Snyder A, et al. Cancer immunology. Mutational landscape determines sensitivity to PD-1 blockade in non-small cell lung cancer. Science 2015;348:124-8. [Crossref] [PubMed]
- Fridman WH, Zitvogel L, Sautès-Fridman C, et al. The immune contexture in cancer prognosis and treatment. Nat Rev Clin Oncol 2017;14:717-34. [Crossref] [PubMed]