A novel gene signature related to fatty acid metabolism predicts prognosis, immune landscape, and drug sensitivity in early-stage lung squamous cell carcinoma
Highlight box
Key findings
• Fatty acid metabolism-related genes (FAMRGs)-based signature: a novel signature based on FAMRGs comprising nine genes demonstrated robust predictive capabilities for prognosis in early-stage lung squamous cell carcinoma (LUSC).
• Prognostic superiority: the signature exhibited superior prognostic capacity compared to conventional clinicopathological features in The Cancer Genome Atlas-LUSC dataset and was validated across six independent Gene Expression Omnibus-LUSC datasets.
• Immunological associations: the signature showed a significant association with immune cell infiltration, human leukocyte antigen genes expression, and immune checkpoint genes, suggesting links between fatty acid metabolism (FAM) and the tumor immune microenvironment.
• Therapeutic relevance: the signature demonstrated potential sensitivity to chemo-/target-therapy, highlighting its clinical relevance for personalized treatment in early-stage LUSC.
What is known and what is new?
• While dysregulation of FAM in tumorigenesis is established, this study pioneers a comprehensive analysis of FAMRGs in early-stage LUSC. Existing knowledge underscores the importance of metabolic alterations in cancer.
• The identified nine-gene signature introduces a novel prognostic tool, surpassing conventional approaches and revealing intricate connections between FAM and the tumor immune microenvironment.
What is the implication, and what should change now?
• The FAMRGs-based signature presents a transformative approach for prognostic prediction and personalized treatment in early-stage LUSC. Clinicians should integrate this signature into risk assessment protocols, and researchers should further explore the identified genes for potential therapeutic targeting. The study underscores the significance of FAM in LUSC, urging a paradigm shift in clinical strategies toward FAMRGs as predictive targets for tailored interventions.
Introduction
Lung cancer stands as a foremost contributor to global cancer-related morbidity and mortality (1). The disparate survival rates between early-stage and advanced lung cancer diagnoses emphasize the criticality of early intervention in prognosis (2). Among the various pathologic types, lung squamous cell carcinoma (LUSC) ranks as the second most prevalent, accounting for approximately 30% of all lung cancer cases (3). In contrast to lung adenocarcinoma (LUAD), LUSC exhibited a more unfavorable prognosis and lacks effective therapeutic targets (3-5). Consequently, an in-depth comprehension of the molecular biology underlying early-stage LUSC and identification of reliable prognostic markers and clinical treatment strategies are pressing needs.
Dysregulation of cellular metabolism stands as a hallmark of cancer (6). Fatty acid (FA) metabolic reprogramming has garnered significant attention in recent years (7). FA metabolism (FAM) plays a pivotal role in cancer’s metabolic reprogramming by not only augmenting the uptake of exogenous FAs and facilitating de novo biosynthesis to sustain rapid cancer cell proliferation but also by acting as secondary messengers in oncogenic signal transduction and serving as an alternate energy source for cellular fuel generation (6,8). Previous research has demonstrated that alterations in FAM and aberrant expression of FAM-related genes (FAMRGs) closely correlate with tumor survival and treatment resistance (8-10). For example, oxoglutarate dehydrogenase-like (OGDHL) was found to be significantly downregulated in clear cell renal cell carcinoma. The upregulation of OGDHL expression effectively inhibited cancer growth and metastasis both in vitro and in vivo (11). Yang et al. showed that RGS2 was highly expressed in gastric cancer and may contribute to immune rejection, which was closely associated with a poor prognosis (12). Furthermore, a study by Li et al. demonstrated that sterol regulatory element binding transcription factor 1 (SREBF1) as a central mediator linking tumor protein 63 (TP63) with FAM, which regulates the biosynthesis of FAs, was essential for viability and migration in squamous cell carcinomas (SCCs), and its overexpression was associated with poor survival in SCC patients (13). However, the characteristics of FAM alteration and its potential as a biomarker for cancer prognosis and treatment response in early-stage LUSC require further exploration. In light of this, our study employs a bioinformatic approach to establish a robust gene signature rooted in FAM for prognostication. This signature aims to offer valuable insights into clinical therapeutic strategies for early-stage LUSC. We present this article in accordance with the TRIPOD reporting checklist (available at https://tcr.amegroups.com/article/view/10.21037/tcr-23-1640/rc).
Methods
Selection of FAMRGs
FAMRGs were curated through the Kyoto Encyclopedia of Genes and Genomes (KEGG) database (https://www.kegg.jp/) and the Molecular Signature Database (MSigDB) (https://www.gsea-msigdb.org/gsea/msigdb). A total of 394 FAMRGs were extracted from 14 lipid metabolism-related gene sets of the KEGG database, and 1,634 FAMRGs were extracted from 18 FAMRG sets of the gene set enrichment analysis (GSEA) database. After removing the overlapping genes, a consolidated list of 1,651 genes was compiled. The detailed information of gene sets is provided in table available at https://cdn.amegroups.cn/static/public/tcr-23-1640-1.xlsx. The study was conducted in accordance with the Declaration of Helsinki (as revised in 2013).
Data acquisition
Gene expression data and clinical survival information for patients diagnosed with stage I–II LUSC were retrieved from The Cancer Genome Atlas (TCGA) database (https://portal.gdc.cancer.gov/) and the Gene Expression Omnibus (GEO) database (https://www.ncbi.nlm.nih.gov/geo). For our study, we incorporated one RNA-seq dataset (TCGA-LUSC) and six microarray datasets (GSE73403, GSE74777, GSE50081, GSE37745, GSE30219, and GSE7157010), all with overall survival (OS) data. The TCGA-LUSC data served as the training cohort, comprising 396 cases, while the validation cohorts consisted of independent datasets from GSE73403, GSE74777, GSE50081, GSE37745, GSE30219, and GSE7157010, totaling 543 GEO-LUSC cases. Detailed clinical information for the cohorts is provided in Table 1.
Table 1
Characteristics | Datasets | ||||||
---|---|---|---|---|---|---|---|
TCGA (n=396) | GSE157010 (n=235) | GSE0081 (n=43) | GSE37745 (n=55) | GSE30219 (n=57) | GSE74777 (n=107) | GSE73403 (n=46) | |
Age (years) | |||||||
≤65 | 147 | 93 | 15 | 20 | 33 | 56 | 30 |
>65 | 244 | 142 | 28 | 35 | 24 | 51 | 16 |
Unknown | 5 | – | – | – | – | – | – |
Gender | |||||||
Male | 290 | 153 | 25 | 38 | 53 | 96 | 44 |
Female | 106 | 82 | 18 | 17 | 4 | 11 | 2 |
Smoking | |||||||
No | 84 | – | – | – | – | 3 | – |
Yes | 303 | – | – | – | – | 104 | – |
Unknown | 9 | – | – | – | – | – | – |
T stage | |||||||
T1 | 109 | 63 | 10 | – | 49 | 26 | 3 |
T2 | 248 | 139 | 33 | – | 6 | 65 | 34 |
T3 | 39 | 31 | – | – | 2 | 16 | 9 |
Unknown | – | 2 | – | – | – | – | – |
N stage | |||||||
N0 | 300 | – | 27 | – | 52 | 72 | 34 |
N1 | 96 | – | 16 | – | 5 | 33 | 12 |
Unknown | – | – | – | – | – | 2 | – |
Stage | |||||||
Stage I | 237 | – | 27 | 40 | – | 54 | 25 |
Stage II | 159 | – | 16 | 15 | – | 53 | 21 |
OS status | |||||||
Dead | 160 | 119 | 17 | 42 | 39 | 42 | 15 |
Alive | 236 | 116 | 26 | 13 | 18 | 65 | 31 |
Recurrence status | |||||||
Recurrence | 47 | – | 11 | 12 | 18 | 28 | – |
No-recurrence | 209 | – | 31 | 13 | 38 | 79 | – |
Unknown | 140 | – | 1 | 30 | 1 | – | – |
TCGA, The Cancer Genome Atlas; OS overall survival.
Consensus clustering analysis and functional enrichment analyses
To identify potential prognostic FAMRGs for early-stage LUSC, we initially conducted univariate Cox regression analysis of OS in the TCGA-LUSC dataset, focusing on genes with a P value <0.05. Subsequently, unsupervised clustering was performed to explore the molecular classification of LUSC based on these prognostic FAMRGs, utilizing the “ConsensusClusterPlus” R package with 1,000 iterations to enhance stability (14). The obtained molecular classification was visualized through principal component analysis (PCA), offering a comprehensive overview of the identified clusters. Kaplan-Meier (K-M) analysis was then performed to compare the survival outcomes between these clusters. Functional enrichment analysis of Gene Ontology (GO) pathways was performed using Cytoscape plug-in ClueGO to elucidate the biological processes and pathways associated with the identified FAMRGs in the context of LUSC (15).
Risk model construction and validation
The prognostic FAMRGs were selected for further analysis using the least absolute shrinkage and selection operator (LASSO) algorithm. LASSO analysis facilitated variable selection and shrinkage, determining the optimal λ value and screen prognosis risk factors based on the “glmnet” R package (16). Subsequently, genes identified in the LASSO regression were incorporated into the multivariate Cox regression analysis, establishing the FAMRGs signature for survival prediction. The signature was based on the relative expression and LASSO Cox coefficient of individual genes as follows:
Patients were categorized into high- and low-risk groups based on the optimal risk score. K-M analysis and receiver operating characteristic (ROC) curve analysis assessed model accuracy and stability. Furthermore, the prognostic value of FAMRGs signature was validated in independent cohorts, including GSE73403, GSE74777, GSE50081, GSE37745, GSE30219, and GSE7157010.
Nomogram
A nomogram was constructed for risk assessment and predict 1-, 3-, and 5-year OS probabilities for individual early-stage LUSC patients by using gender, age, T stage, N stage, tumor-node-metastasis (TNM) stage, and risk score. The “survival” and “rms” R packages facilitated this process (17).
Gene set variation analysis (GSVA)
We also performed GSVA to elucidate relevant signaling pathways and molecular mechanisms in high- and low-risk groups using the hallmark gene set (c2.cp.kegg.v7.4.symbols.gmt) from the MSigDB database (18).
Tumor immune infiltration analysis
To investigate the immune landscape influenced by FAMRGs-based signature in LUSC patients, we conducted a comprehensive analysis, including the assessment of tumor immune cell infiltration, analysis of immune functions, and examination the expression of immune regulatory genes across different risk groups. We utilized the ESTIMATE algorithm to calculate immune scores, stromal scores, and ESTIMATE scores between high- and low-risk groups using “estimate” R package (19). Additionally, we employed the single simple GSEA (ssGSEA) algorithm to assess the immune function analysis, human leukocyte antigen (HLA) genes expression, and immune checkpoint genes expression based on “gsva” R package (20).
Drug response prediction
Furthermore, we performed drug response in enrolled cases using Genomics of Drug Sensitivity in Cancer (GDSC) database. Half-maximal inhibitory concentration (IC50) was calculated using “pRRophetic” R package for estimating drug sensitivity (21).
Statistical analysis
All statistical analyses were conducted using R language (version 4.2.1). The Wilcoxon test compared two groups, while the Kruskal-Wallis’s test compared more than two groups. K-M analysis with log-rank test assess OS, and Cox hazard regression analysis identified independent prognostic factors. P<0.05 was considered significant.
Results
Identification of FAMRGs and functional annotation
The study’s flowchart is illustrated in Figure 1. A total of 396 and 543 patients with early-stage LUSC were selected from training (TCGA dataset) and validation (GEO datasets) cohorts, respectively. Initially, we cross-verified RNA expression in the TCGA, GEO, and FAMRGs datasets, identifying 1,390 co-expressed genes for further investigation (https://bioinformatics.psb.ugent.be/webtools/Venn/) (Figure 2A). Subsequently, a univariate Cox regression analysis was applied to the TCGA-LUSC dataset, identifying 343 prognostic FAMRGs with a corrected P value of regression analysis less than 0.05 (table available at https://cdn.amegroups.cn/static/public/tcr-23-1640-2.xlsx). We then grouped 343 prognostic FAMRGs using unsupervised clustering analysis, employing the Elbow method to determine the optimal number of clusters. The result, k=2, revealed a distinct distribution pattern between cluster 1 and cluster 2 (Figure 2B,2C). Moreover, patients in cluster 1 showed better OS than patients in cluster 2 (P=0.000042) (Figure 2D). Additionally, the GO analyses based on ClueGO indicated that these prognostic FAMRGs predominantly participated in positive regulation of lipid kinase activity (46.88%), phosphatidylinositol phosphate biosynthetic process (28.12%), positive regulation of protein phosphorylation (18.75%), and acyl-coenzyme A (CoA) metabolic process (6.25%) (P<0.01) (Figure 3A,3B).
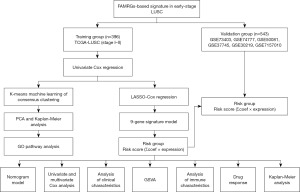
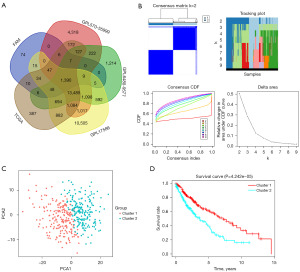
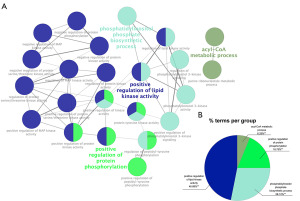
Construction and validation of a FAMRGs prognostic signature
To identify the most powerful FAMRGs for establishing the prognostic signature, we applied the 343 prognostic FAMRGs to LASSO regression analysis, resulting in 21 genes for further analysis (Figure 4A,4B). Subsequently, these 21 genes underwent multivariate Cox regression analysis, identifying the nine most robust FAMRGs (ACOT11, APOH, BMX, CYP2R1, DPEP3, FABP6, FADS2, GLYATL2, and THRSP) for model construction (Figure 4C). The risk score model was calculated using the formula: risk score = 0.253993 × ACOT11 + 0.107299 × APOH + 0.094113 × BMX + 0.204989 × CYP2R1 + 0.091726 × DPEP3 + 0.066066 × FABP6 + 0.098061 × FADS2 + (−0.07792) × GLYATL2 + 0.108707 × THRSP. In the TCGA cohort, patients were classified into high- (n=197) and low-risk (n=198) groups based on the optimized cutoff risk score. The distribution of risk scores and survival status is shown in Figure 4D, with high-risk scores patients exhibiting higher survival risk and shorter survival time. The expression heatmap of nine genes between the subgroups is depicted in Figure 4E. The time-dependent ROC curve was utilized to evaluate the performance of the model. The results showed that the area under the curve (AUC) values for predicting 1-, 3-, and 5-year OS were 0.700, 0.735, and 0.732, respectively [hazard ratio (HR) =4.26; 95% confidence interval (CI): 3.15–5.75; P<0.0001], indicating the robustness of the model (Figure 4F). Moreover, K-M survival analysis revealed that patients with high-risk scores showed worse OS, disease-specific survival (DSS), progression-free interval (PFI), and disease-free interval (DFI) than patients with low-risk scores (P<0.001) (Figure 4G-4J).
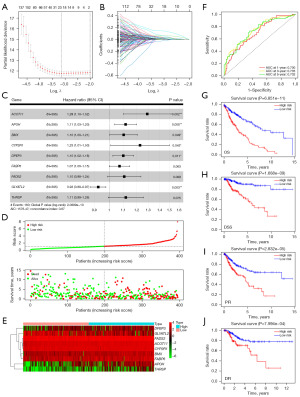
Furthermore, six independent GEO datasets (GSE73403, GSE74777, GSE50081, GSE37745, GSE30219, and GSE7157010) were used to validate the performance of the model. Each cohort was classified into a high-risk group and a low-risk group according to the optimistic cutoff value of the risk score. The results demonstrated that patients with high-risk scores exhibit worse OS than patients with low-risk scores in the six GEO datasets (GSE74777, P=0.0276; GSE37745, P=0.0352; GSE157010, P=0.0367; GSE50081, P=0.0133; GSE73403, P=0.0061; GSE30219, P=0.082) (Figure 5A-5F), indicating that the signature based on FAMRGs can accurately predict the prognosis of early-stage LUSC.
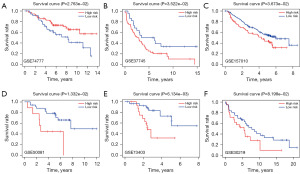
As the FAMRGs-based signature having undergone robust validation in independent cohorts, we further carried out univariate and multivariate Cox regression analysis to assess its viability as an independent prognostic predictor for early-stage LUSC patients in the TCGA-LUSC cohort. Notably, the risk score derived from the signature emerged as an independent indicator in both univariate (HR =1.740; 95% CI: 1.509–2.007; P<0.001) and multivariate Cox analyses (HR =1.722; 95% CI: 1.494–1.983; P=0.001) even after adjusting for various clinical features such as age, gender, stage, and smoking status (Figure 6A,6B). To facilitate risk assessment and predict 1-, 3-, and 5-year OS probabilities for individual LUSC patients, we constructed a personalized scoring nomogram. This nomogram seamlessly integrated the risk score with other pertinent clinicopathological features, including age, gender, T stage, N stage, and TNM stage. An illustrative example of the nomogram’s application in predicting survival probability for a patient is depicted in Figure 6C. The calibration curves were employed to validate the nomogram’s performance, revealing a high degree of consistency between the actual 1-year (green line), 3-year (blue line), and 5-year (red line) OS predictions and the predicted survival (45° line) (Figure 6D). Additionally, ROC analysis was performed to compare the predictive abilities of risk score, nomogram, and other clinicopathological features in predicting OS. The results demonstrated that the AUC value of the risk score was 0.721, while the AUC of the nomogram was 0.690, surpassing the performance of other parameters (Figure 6E). These findings affirm that both risk score and nomogram exhibit remarkable predictive capabilities for early-stage LUSC, with the risk score performed better.
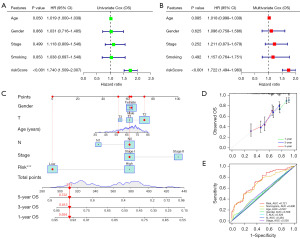
Furthermore, we assessed the association of FAMRGs-based signature with clinicopathological features. The distribution of clinicopathological features and gene expression level between high- and low-risk groups is shown in Figure 7A. Boxplots were used to assess differences in risk scores among different clinical parameters. The results showed that there were no significant differences in risk scores based on age, gender, smoking status, and T stage. Contrary to our conjectures, patients with lower TNM stage (P=0.0087) and less lymph node metastasis (P=0.0085) had higher risk scores (Figure 7B-7G).
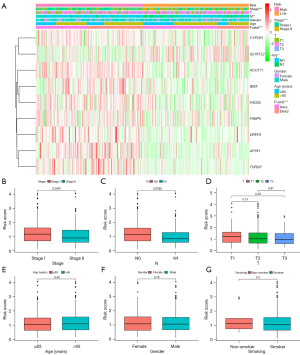
GSVA
GSVA was employed to analyze the differences in metabolic processes between the high-risk and low-risk groups. As shown in Figure 8, the metabolic pathways in apoptosis, NOD-like receptor signaling pathway, glycosphingolipid biosynthesis ganglio series, complement and coagulation cascades, glycosaminoglycan degradation, other glycan degradation, focal adhesion, lysosome, and N-glycan biosynthesis were enriched in the high-risk group. In contrast, the metabolic pathways in linoleic acid metabolism, glycosphingolipid biosynthesis-lacto and neolacto series, starch and sucrose metabolism, pentose and glucoronate interconversions, ascorbate and aldarate metabolism, and drug metabolism other enzymes were enriched in the low-risk group.
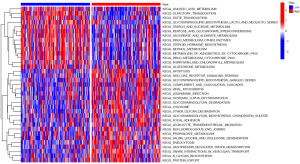
Immune infiltration analysis and immunomodulators expression in LUSC patients
Previous research has linked high immune cell infiltration in lung cancer to a poor prognosis (22). Therefore, we evaluated the infiltration levels of various immune cells on TCGA-LUSC samples by ESTIMATE algorithm analysis. We observed that elevated stromal score, immune score, and ESTIMATE score in the high-risk group compare to the low-risk group, indicating increased immune cell infiltration in the high-risk group (Figure 9A-9C). The immune function analysis further revealed that a significant increase in C-C chemokine receptor (CCR), HLA, parainflammation, type-II-interferon (INF)-response, major histocompatibility complex (MHC)-class-I, and type-I-INF-response in the high-risk group (Figure 9D). Additionally, we conducted a comparative analysis of the expression levels of immune regulatory molecules, including HLA genes and eight immune checkpoint genes between different risk groups. The results showed that high-risk group exhibited higher expression of HLA genes and several immune checkpoint genes such as CTLA4, HAVCR2, LAG3, PDCD1, PDCD1LG2, SIGLEC15, and TIGIT (Figure 9E,9F). These results illuminate the possibility that early-stage LUSC patients with high-risk scores may manifest a more robust response to therapies targeting the mentioned immune checkpoints. Furthermore, considering the central role of HLA molecules in immunity, variation at the HLA loci could differentially affect the response to immune checkpoint inhibitors (ICIs) (23). For example, the loss of heterozygosity at the HLA-I locus (HLA-LOH) was associated with immune resistance in non-small cell lung cancer (NSCLC) (24). Consequently, enhancing the expression of HLA may hold the potential to augment T immune cell activities and improve the response to immunotherapy in NSCLC (25). These findings contribute to our understanding of the immune landscape mediated by FAMRGs in LUSC and provide potential avenues for therapeutic intervention.

Signature predict the response to chemotherapy and targeted therapy
Since GSVA revealed an enrichment of drug metabolism pathway in the low-risk group, we hypothesized that our FAMRGs-based signature may influence the response to anti-tumor treatment in LUSC. We utilized the GDSC database for drug response prediction. Our results demonstrated that patients with low-risk scores exhibited heightened sensitivity to several antineoplastic drugs (gemcitabine, paclitaxel, and erlotinib) relevant to LUSC. The IC50 values for gemcitabine, paclitaxel, and erlotinib were significantly lower in the low-risk group compared to the high-risk group (Figure 10A-10F, P<0.001).
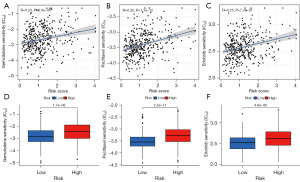
Discussion
FAs are essential for synthesizing complex lipids critical for cancer cell proliferation (7). FAs metabolism in cancer cells is influenced not only by intracellular oncogenic signals but also by various factors in the tumor immune microenvironment, such as lipids, cells, cytokines, growth factors, DNA, RNA, and nutrients (6). Emerging evidence highlights the role of FAs metabolism in immune escape and immune tolerance, involving immune checkpoint molecules, antigen presentation loss, and lipid metabolic reprogramming (26,27). Understanding the interplay between FAM and immune cell function could reveal valuable markers for tumor prognosis and treatment. However, while studies on lung cancer metabolism are primarily focused on adenocarcinoma, research on SCC remains limited. Our study aimed to uncover the unique FAM characteristics in early-stage LUSC and its association with prognosis, tumor immunity, and therapeutic response.
In this study, we developed a FAMRGs-based prognostic signature for early-stage LUSC based on the TCGA dataset. First, we identified 343 prognosis-related genes from a pool of 1,390 FAMRGs in the TCGA-LUSC cohort through univariate Cox regression analysis. Based on unsupervised clustering, these 343 prognosis FAMRGs can be classified into two clusters (cluster 1 and cluster 2) and significantly different OS were observed between the two clusters. Concurrently, these prognostic FAMRGs were found to be predominantly involved in regulation of lipid kinase activity and various metabolic pathways. To select the most robust candidate genes for constructing the FAMRGs-based prognostic signature, we employed LASSO Cox regression analysis and multivariate regression analysis. Ultimately, nine genes (ACOT11, APOH, BMX, CYP2R1, DPEP3, FABP6, FADS2, GLYATL2, and THRSP) were identified as the most reliable contributors to established signature. Notably, these genes were predominantly involved in regulating tumor lipid metabolism and progression. For instance, ACOT11, a FA-CoA metabolic enzyme, was proved as an oncogene in lung cancer, which could bind CSE1L to promote cell proliferation, migration, and invasion (28). FA-binding protein 6 (FABP6), a bile acid carrier protein, was overexpressed in colorectal cancer, bladder cancer, and glioma (29). Inhibition of FABP6 reduced tumor cell invasion and angiogenesis by reducing MMP-2 and VEGF in human glioblastoma cells (30). Kothapalli et al. showed that as a dominant FA desaturase (FAD), FADS2 upregulated in various cancer cells including melanoma, prostate, liver, and lung cancer, which could accelerate lipid metabolic activity and tumor invasiveness (31). In ovarian cancer, inhibition of FADS2 directly downregulated GPX4 and the GSH/GSSG ratio, disrupted cellular/mitochondrial redox balance, and then leaded to iron-mediated lipid peroxidation and mitochondrial dysfunction in ascites-derived ovarian cancer cells (32). Another study indicated that FADS2 mediated resistance to PI3K/mTOR inhibitors in NSCLC (33). These findings suggest that the genes within this signature could potentially impact the prognosis of LUSC by regulating tumor lipid metabolism. The predictive performance of the prognostic signature was validated across six independent GEO-LUSC datasets. Furthermore, even after adjusting for clinicopathological confounders in multivariate Cox regression analysis, the signature maintained its accurate prognostic predictive capacity. To enhance the practical application, we developed a scoring nomogram as a visual tool for predicting survival in individual LUSC patients.
Considering the potential link between FAMRGs and immune escape, immune tolerance, and antigen presentation loss, as well as the crucial role of tumor immune microenvironment in tumorigenesis, prognosis, and response to immunotherapy (34-36), we investigated tumor immune cell infiltration and immunomodulators expression in different risk groups. Our investigation demonstrated an upregulation of immune-related genes, particularly those associated with HLA and immune checkpoint genes, in the high-risk group. Notably, immune functions such as CCR, HLA, parainflammation, type-II-INF-response, MHC-class-I, and type-I-INF-response were markedly elevated in this cohort. These findings strongly suggest intrinsic differences in tumor immunogenicity between FAMRGs-defined risk groups and FAMRGs may play a pivotal role in shaping the tumor immune microenvironment. Furthermore, through GSVA, we identified the NOD-like receptor signaling pathway as a major pathway in the high-risk group. Previous research has highlighted the involvement of this pathway in inflammation-associated tumorigenesis, angiogenesis, cancer cell stemness, and chemoresistance (37). Based on these findings, we propose a hypothesis that FAMRGs may contribute to early-stage LUSC tumorigenesis by regulating the tumor immune microenvironment particularly through the modulation of NOD-like receptor signaling pathway.
In addition, GSVA analysis unveiled an enrichment of drug metabolism pathway in the low-risk group. We used GDSC for drug sensitivity analysis. Our results indicated that enhanced effectiveness of gemcitabine, paclitaxel, and erlotinib in early-stage LUSC patients with low-risk scores. These findings underscore the potential of our FAMRGs-based signature as a valuable tool for predicting and stratifying patient responses to specific chemotherapeutic and targeted therapeutic agents. The identification of such predictive signature holds promise for guiding personalized treatment approaches in LUSC patients, thereby enhancing the effectiveness and precision of therapeutic interventions.
Although the FAMRGs-based signature proves effective as a prognostic marker and emerges as a potential therapeutic target in early-stage LUSC, this study still had some limitations. Firstly, our study was based on some retrospective datasets, and a prospective study to validate the utility of this FAMRGs-based signature will be necessary. Secondly, the conclusion drawn from our integrated analysis of clinical samples obtained from public databases, and the findings need validated through new methodologies and the inclusion of fresh specimens. Thirdly, while we indirectly assessed the ability to predict responses to immune, chemo, and targeted therapies, a more in-depth exploration of the underlying mechanisms of the signature in predicting treatment responses is warranted.
Conclusions
This study establishes a robust prognostic signature based on nine FAMRGs, providing a reliable tool for predicting prognosis, delineating immune landscape, and offering profound insights for the development of personalized treatment strategies in early-stage LUSC patients.
Acknowledgments
We thank the Department of Oncology, the Affiliated Cancer Hospital of Nanjing Medical University, Nanjing, China, for providing computing resources and bioinformatics support. We also thank TCGA and GEO for their research network.
Funding: This study was supported by the grants from
Footnote
Reporting Checklist: The authors have completed the TRIPOD reporting checklist. Available at https://tcr.amegroups.com/article/view/10.21037/tcr-23-1640/rc
Peer Review File: Available at https://tcr.amegroups.com/article/view/10.21037/tcr-23-1640/prf
Conflicts of Interest: All authors have completed the ICMJE uniform disclosure form (available at https://tcr.amegroups.com/article/view/10.21037/tcr-23-1640/coif). The authors have no conflicts of interest to declare.
Ethical Statement: The authors are accountable for all aspects of the work in ensuring that questions related to the accuracy or integrity of any part of the work are appropriately investigated and resolved. The study was conducted in accordance with the Declaration of Helsinki (as revised in 2013).
Open Access Statement: This is an Open Access article distributed in accordance with the Creative Commons Attribution-NonCommercial-NoDerivs 4.0 International License (CC BY-NC-ND 4.0), which permits the non-commercial replication and distribution of the article with the strict proviso that no changes or edits are made and the original work is properly cited (including links to both the formal publication through the relevant DOI and the license). See: https://creativecommons.org/licenses/by-nc-nd/4.0/.
References
- Sung H, Ferlay J, Siegel RL, et al. Global Cancer Statistics 2020: GLOBOCAN Estimates of Incidence and Mortality Worldwide for 36 Cancers in 185 Countries. CA Cancer J Clin 2021;71:209-49. [Crossref] [PubMed]
- Siegel RL, Miller KD, Fuchs HE, et al. Cancer Statistics, 2021. CA Cancer J Clin 2021;71:7-33. [Crossref] [PubMed]
- Chen P, Liu Y, Wen Y, et al. Non-small cell lung cancer in China. Cancer Commun (Lond) 2022;42:937-70. [Crossref] [PubMed]
- Lau SCM, Pan Y, Velcheti V, et al. Squamous cell lung cancer: Current landscape and future therapeutic options. Cancer Cell 2022;40:1279-93. [Crossref] [PubMed]
- Tan AC, Tan DSW. Targeted Therapies for Lung Cancer Patients With Oncogenic Driver Molecular Alterations. J Clin Oncol 2022;40:611-25. [Crossref] [PubMed]
- Bian X, Liu R, Meng Y, et al. Lipid metabolism and cancer. J Exp Med 2021;218:e20201606. [Crossref] [PubMed]
- Koundouros N, Poulogiannis G. Reprogramming of fatty acid metabolism in cancer. Br J Cancer 2020;122:4-22. [Crossref] [PubMed]
- Butler LM, Perone Y, Dehairs J, et al. Lipids and cancer: Emerging roles in pathogenesis, diagnosis and therapeutic intervention. Adv Drug Deliv Rev 2020;159:245-93. [Crossref] [PubMed]
- Snaebjornsson MT, Janaki-Raman S, Schulze A. Greasing the Wheels of the Cancer Machine: The Role of Lipid Metabolism in Cancer. Cell Metab 2020;31:62-76. [Crossref] [PubMed]
- Munir R, Lisec J, Swinnen JV, et al. Too complex to fail? Targeting fatty acid metabolism for cancer therapy. Prog Lipid Res 2022;85:101143. [Crossref] [PubMed]
- Shi J, Miao D, Lv Q, et al. The m6A modification-mediated OGDHL exerts a tumor suppressor role in ccRCC by downregulating FASN to inhibit lipid synthesis and ERK signaling. Cell Death Dis 2023;14:560. [Crossref] [PubMed]
- Yang S, Sun B, Li W, et al. Fatty acid metabolism is related to the immune microenvironment changes of gastric cancer and RGS2 is a new tumor biomarker. Front Immunol 2022;13:1065927. [Crossref] [PubMed]
- Li LY, Yang Q, Jiang YY, et al. Interplay and cooperation between SREBF1 and master transcription factors regulate lipid metabolism and tumor-promoting pathways in squamous cancer. Nat Commun 2021;12:4362. [Crossref] [PubMed]
- Shi Y, Zhang L, Peterson CB, et al. Performance determinants of unsupervised clustering methods for microbiome data. Microbiome 2022;10:25. [Crossref] [PubMed]
- Lin J, Lu Y, Wang B, et al. Analysis of immune cell components and immune-related gene expression profiles in peripheral blood of patients with type 1 diabetes mellitus. J Transl Med 2021;19:319. [Crossref] [PubMed]
- Engebretsen S, Bohlin J. Statistical predictions with glmnet. Clin Epigenetics 2019;11:123. [Crossref] [PubMed]
- Wang J, Zhanghuang C, Tan X, et al. A Nomogram for Predicting Cancer-Specific Survival of Osteosarcoma and Ewing's Sarcoma in Children: A SEER Database Analysis. Front Public Health 2022;10:837506. [Crossref] [PubMed]
- Hänzelmann S, Castelo R, Guinney J. GSVA: gene set variation analysis for microarray and RNA-seq data. BMC Bioinformatics 2013;14:7. [Crossref] [PubMed]
- Yoshihara K, Shahmoradgoli M, Martínez E, et al. Inferring tumour purity and stromal and immune cell admixture from expression data. Nat Commun 2013;4:2612. [Crossref] [PubMed]
- Charoentong P, Finotello F, Angelova M, et al. Pan-cancer Immunogenomic Analyses Reveal Genotype-Immunophenotype Relationships and Predictors of Response to Checkpoint Blockade. Cell Rep 2017;18:248-62. [Crossref] [PubMed]
- Geeleher P, Cox N, Huang RS. pRRophetic: an R package for prediction of clinical chemotherapeutic response from tumor gene expression levels. PLoS One 2014;9:e107468. [Crossref] [PubMed]
- Zuo S, Wei M, Wang S, et al. Pan-Cancer Analysis of Immune Cell Infiltration Identifies a Prognostic Immune-Cell Characteristic Score (ICCS) in Lung Adenocarcinoma. Front Immunol 2020;11:1218. [Crossref] [PubMed]
- Naranbhai V, Viard M, Dean M, et al. HLA-A*03 and response to immune checkpoint blockade in cancer: an epidemiological biomarker study. Lancet Oncol 2022;23:172-84. [Crossref] [PubMed]
- Schehr JL, Sethakorn N, Schultz ZD, et al. Analytical validation and initial clinical testing of quantitative microscopic evaluation for PD-L1 and HLA I expression on circulating tumor cells from patients with non-small cell lung cancer. Biomark Res 2022;10:26. [Crossref] [PubMed]
- Carl DL, Laube Y, Serra-Burriel M, et al. Comparison of Uptake and Prices of Biosimilars in the US, Germany, and Switzerland. JAMA Netw Open 2022;5:e2244670. [Crossref] [PubMed]
- Ma X, Xiao L, Liu L, et al. CD36-mediated ferroptosis dampens intratumoral CD8(+) T cell effector function and impairs their antitumor ability. Cell Metab 2021;33:1001-1012.e5. [Crossref] [PubMed]
- Lim SA, Wei J, Nguyen TM, et al. Lipid signalling enforces functional specialization of T(reg) cells in tumours. Nature 2021;591:306-11. [Crossref] [PubMed]
- Liang C, Wang X, Zhang Z, et al. ACOT11 promotes cell proliferation, migration and invasion in lung adenocarcinoma. Transl Lung Cancer Res 2020;9:1885-903. [Crossref] [PubMed]
- Lin CH, Chang HH, Lai CR, et al. Fatty Acid Binding Protein 6 Inhibition Decreases Cell Cycle Progression, Migration and Autophagy in Bladder Cancers. Int J Mol Sci 2022;23:2154. [Crossref] [PubMed]
- Pai FC, Huang HW, Tsai YL, et al. Inhibition of FABP6 Reduces Tumor Cell Invasion and Angiogenesis through the Decrease in MMP-2 and VEGF in Human Glioblastoma Cells. Cells 2021;10:2782. [Crossref] [PubMed]
- Kothapalli KSD, Park HG, Kothapalli NSL, et al. FADS2 function at the major cancer hotspot 11q13 locus alters fatty acid metabolism in cancer. Prog Lipid Res 2023;92:101242. [Crossref] [PubMed]
- Xuan Y, Wang H, Yung MM, et al. SCD1/FADS2 fatty acid desaturases equipoise lipid metabolic activity and redox-driven ferroptosis in ascites-derived ovarian cancer cells. Theranostics 2022;12:3534-52. [Crossref] [PubMed]
- Colombo M, Passarelli F, Corsetto PA, et al. NSCLC Cells Resistance to PI3K/mTOR Inhibitors Is Mediated by Delta-6 Fatty Acid Desaturase (FADS2). Cells 2022;11:3719. [Crossref] [PubMed]
- Hinshaw DC, Shevde LA. The Tumor Microenvironment Innately Modulates Cancer Progression. Cancer Res 2019;79:4557-66. [Crossref] [PubMed]
- Bader JE, Voss K, Rathmell JC. Targeting Metabolism to Improve the Tumor Microenvironment for Cancer Immunotherapy. Mol Cell 2020;78:1019-33. [Crossref] [PubMed]
- Genova C, Dellepiane C, Carrega P, et al. Therapeutic Implications of Tumor Microenvironment in Lung Cancer: Focus on Immune Checkpoint Blockade. Front Immunol 2022;12:799455. [Crossref] [PubMed]
- Liu P, Lu Z, Liu L, et al. NOD-like receptor signaling in inflammation-associated cancers: From functions to targeted therapies. Phytomedicine 2019;64:152925. [Crossref] [PubMed]