A nomogram for predicting overall survival of breast cancer with regional lymph node metastasis in young women
Highlight box
Key findings
• In this study, a brand-new nomogram that predicts 2-, 3-, and 5-year overall survival (OS) in young breast cancer (YBC) patients with regional lymph node metastases (RLNMs) was successfully established and showed great clinical utility.
What is known and what is new?
• Compared to older breast cancer patients, YBC patients have a higher risk of recurrence and mortality, as well as a poorer prognosis for survival.
• This is the first practical and real-world nomogram based on clinicopathological variables from a large-scale database to predict OS in YBC patients with RLNMs.
What is the implication, and what should change now?
• The utilization of our nomogram will empower clinicians to identify potential high-risk YBC with RLNM subgroups with inferior survival outcomes, enabling them to make more sophisticated and personalized treatment and management decisions.
Introduction
According to Global Cancer Statistics 2022, breast cancer showed a slow growth over the last few years but ranked first among newly diagnosed cancers, constituting nearly 31% of all new cancer cases occurrences in females (1,2). Although the vast majority of breast cancer cases occur in middle-aged and elderly women, and the incidence of breast cancer tends to increase with age (3,4), a rise in incidence has been recorded among younger individuals in several countries (5-8). There is no set definition for “young women” in the field of breast oncology, but most of the literature refers to women aged ≤40 years (9,10). The European Society for Medical Oncology guidelines define young breast cancer (YBC) as those aged below 40 years, which is the most prevalent malignant tumor and the main cause of female cancer mortality in this age range, accounting for approximately 5–15% of all cases of invasive breast cancer (10-12). As a result, YBC has aroused growing attention in recent years. Previous research has indicated that as compared to older individuals, young people with breast cancer had more advanced stages, more aggressive tumor subtypes (such as triple-negative), worse outcomes, and a greater recurrence rate (9,10,13-15).
Till now, more than 26 counties around the world are running breast cancer screening programs, with the majority of these counties recommending biennial screening mammography for all women over 40 years old, for instance, the United States Preventive Services Task Force (USPSTF) recently announced new clinical recommendations that women aged 50 to 74 years should perform biennial screening (16,17). It is inevitable that a considerable number of young women often present to clinicians because of clinically significant symptomatology before normal screening programs, such as discharge from nipples, a lump that feels different from the other breast tissue, lumps found in lymph nodes located in the armpits and so forth. YBC patients identified with big tumors and regional lymph node metastasis (RLNM), unfortunately, are prone to a poor prognosis and unfavorable clinical outcomes (18,19). Notably, Abdel-Razeq et al. reported that YBC adults with the node-positive disease had worse 5-year overall survival (OS) than those with node-negative disease (81% vs. 93%, P=0.0006) (20). Gao et al. found that a high nodal tumor burden (>2 positive lymph nodes) was more likely to occur in young women diagnosed with breast cancer (21). Indeed, as a key part of the tumor-node-metastasis (TNM) staging system, the status of regional lymph nodes has been proven to be correlated with biological aggressiveness and a propensity for distant spread (22), which is critically important for surgeons to implement further treatment strategies for patients.
Nomograms in breast cancer are statistical tools used for prognosis, prediction, and decision-making. They utilize multiple factors, such as patient characteristics, tumor characteristics, and treatment variables, to provide personalized estimates for outcomes such as survival, recurrence, and response to therapy. These nomograms have gained attention due to their ability to provide individualized risk assessments and assist clinicians in making informed treatment decisions (23). Some common themes addressed in previous papers include the development and validation of nomograms for various breast cancer subtypes, the incorporation of novel biomarkers and imaging techniques into nomograms, and the evaluation of nomogram performance in different patient populations. Currently, there are just a few studies that particularly focus on the clinicopathologic features and prognosis of YBC patients with RLNM. Using the Surveillance, Epidemiology, and End Results (SEER) database, we intend to explore independent predictive variables for OS and further establish and validate a novel and feasible nomogram for YBC patients with RLNM, which would assist clinicians to identify potential high-risk subgroups with poorer survival outcomes and make better-personalized treatment and management decisions. We present this article in accordance with the TRIPOD reporting checklist (available at https://tcr.amegroups.com/article/view/10.21037/tcr-23-1825/rc).
Methods
Patients’ identification
The data of patients who received a diagnosis of YBC with RLNM between 2010 and 2015 were retrieved from the SEER 18 Cancer Registries (with additional treatment fields) using Surveillance Research Program, National Cancer Institute SEER*Stat software (https://seer.cancer.gov/seerstat/) version 8.3.9. The SEER database, which is sponsored by the National Cancer Institute and keeps cancer information for nearly 30% of the population of the U.S., was used in this retrospective cohort research (24). Our SEER Research Data Use Agreement had already been signed, allowing us to access SEER data under the login “20864-Nov2020”. Because of anonymous patient data and free availability for institutional account holders and non-institutional users, informed permission of patients in the SEER database was not necessary. As a result, the Ethics Committee of the Second Affiliated Hospital of Guangzhou Medical University waived ethics approval for this study. The study was conducted in accordance with the Declaration of Helsinki (as revised in 2013).
The inclusion criteria were as follows: (I) pathology-confirmed invasive breast cancer; (II) female patients aged 18 and 40 years; (III) patients diagnosed between January 2010 and December 2015; (IV) histology records were confirmed as 8500/3, 8520/3, 8522/3, 8523/3, and 8524/3 according to the International Classification of Diseases of Oncology, 3rd Edition (ICD-0-3) codes; and (V) patients diagnosed with RLNM. The exclusion criteria were as follows: (I) patients who had unknown information on significant variables including race, grade, marital status, T stage, molecular subtype, surgery, and distant metastasis status; (II) bilateral or site unspecified concerning laterality; (III) survival time shorter than 1 month after diagnosis; and (IV) breast cancer was not the first and only malignant tumor. This cohort research eventually enrolled 6,424 eligible patients, of whom 4,497 were assigned at random to the training group and 1,927 to the validation cohort (Figure 1).
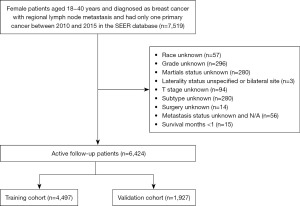
Variable definition and outcome
In the analysis, the following demographic and clinicopathological information were obtained from each eligible patient: age at diagnosis for breast cancer (between 18 and 40 years), race (White, Black, and other), primary site (central, inner, outer, overlapping and other of breast), marital status at diagnosis (married and unmarried), grade (grade I: well differentiated, grade II: moderately differentiated, grade III: poorly differentiated, grade IV: undifferentiated), laterality (left and right orientation of primary tumor), histology [invasive ductal carcinoma (IDC), invasive lobular carcinoma (ILC), and other], therapeutic regimen (including surgery, radiation and chemotherapy), molecular subtype [luminal A: hormone receptor (HR)+/human epidermal growth factor receptor 2 (HER2)−, luminal B: HR+/HER2+, HER2 enriched: HR−/HER2+, triple negative: HR−/HER2−], derived American Joint Committee on Cancer (AJCC) stage 7th edition (I, II, III, and IV), derived AJCC T stage (T1, T2, T3, and T4), derived AJCC N stage (N1, N2, and N3), bone metastasis (no and yes), liver metastasis (no and yes), lung metastasis (no and yes), survival status and survival time. In the SEER database, derived AJCC T stage and N stage typically refer to the pathological staging, which is determined based on the pathological analysis results of the surgically resected tissue. The main endpoint of our study was a patient’s OS, which was typically calculated as the interval from diagnosis to death for any reason or, in the case of still-living patients, until the last follow-up.
Statistical analysis
The statistical software programs R (version 4.1.2; https://www.r-project.org/) and Free Statistics software (version 1.5) were used to conduct all of the analyses. Using the caret package in R, the eligible participants were randomly split into a training set and a validation set, conforming to a frequently-used 7:3 ratio. Categorical variables, shown as counts and percentages, were examined using the chi-squared test or Fisher’s exact test, while non-normally distributed continuous variables, such as age, were assessed by the rank-sum test and given as medians with quartiles. Univariate Cox regression analyses were performed in the training group to look for possible OS risk factors, and statistically significant variables (P<0.05) were chosen to conduct multivariate Cox regression analysis, for the purpose of determining the independent prognostic variables for OS. Results were presented as hazard ratios and 95% confidence intervals (CIs). After that, a prognostic nomogram using the rms package was created to estimate the patients’ 2-, 3-, and 5-year OS using independent prognostic indicators in multivariate analysis in the training set. Harrell’s concordance index (C-index), as well as the area under the curve (AUC) of a time-dependent receiver operating characteristic (ROC) curve, were carried out to assess predictive discrimination of our nomogram model (25). They both fell between the ranges of 0.5 and 1.0, where 0.5 indicating no predicted discrimination and 1.0 indicating excellent discriminative ability. Subsequently, calibration curves (with 1,000 bootstrapping) were plotted to see if the nomogram-predicted and actual survival probabilities were consistent. To examine the nomogram’s clinical utility, we also generated decision curve analyses (DCAs) using the ggDCA package, which is a plot of net benefit against threshold probability (26). The overall score of each patient served as the basis for the creation of a risk classification system. Patients in both cohorts were split into two groups based on their median risk scores: the high- and the low-risk groups. The log-rank test was applied to Kaplan-Meier survival curves to show the OS differences between the two groups. All P values were always two-tailed, and statistical significance was considered as a value less than 0.05.
Results
Patients’ features
Based on the inclusion and exclusion criteria, 6,424 eligible patients from the SEER database were eventually enrolled in this study from 2010 to 2015 and then were split into the training set (n=4,497) and the validation set (n=1,927) in a 7:3 ratio at random. Figure 1 presents the flowchart of patient selection. The median age at diagnosis of the overall population was 36.0 years. A considerable proportion of patients (71.8%) were White race in the training set, while 72.4% were in the validation set. Meanwhile, according to the degree of differentiation, grade III (poorly differentiated) (57.5%) accounted for the foremost percentage, followed by grade II and grade I (moderately and well differentiated) (only 36.8% and 5.2% respectively). Moreover, the number of people diagnosed with IDC in histology was 3,991 (88.7%) in the training group and 1,720 (89.3%) in the test group. In addition, luminal A breast cancer was more frequently found in young patients, whereas HER2 enriched subtype took up a minimal part, only 7.0%. Regarding the AJCC stage 7th edition and T stage, there were approximately half of the patients considered as stage II and T2, respectively. Notably, the whole patient follow-up period lasted 41 months on average (with a range of 1 to 83 months). Table 1 provides an overview of the demographics and clinicopathologic characteristics of the two groups.
Table 1
Variables | Total (n=6,424) | Training cohort (n=4,497) | Validation cohort (n=1,927) |
---|---|---|---|
Age (years), median (IQR) | 36.0 (33.0, 39.0) | 36.0 (33.0, 39.0) | 37.0 (33.0, 39.0) |
Race, n (%) | |||
White | 4,624 (72.0) | 3,228 (71.8) | 1,396 (72.4) |
Black | 981 (15.3) | 686 (15.3) | 295 (15.3) |
Other† | 819 (12.7) | 583 (13.0) | 236 (12.2) |
Marital status, n (%) | |||
No | 2,324 (36.2) | 1,636 (36.4) | 688 (35.7) |
Yes | 4,100 (63.8) | 2,861 (63.6) | 1,239 (64.3) |
Primary site, n (%) | |||
Central | 258 (4.0) | 187 (4.2) | 71 (3.7) |
Inner | 861 (13.4) | 608 (13.5) | 253 (13.1) |
Outer | 2,733 (42.5) | 1,914 (42.6) | 819 (42.5) |
Overlapping | 1,443 (22.5) | 1,033 (23.0) | 410 (21.3) |
Other‡ | 1,129 (17.6) | 755 (16.8) | 374 (19.4) |
Grade, n (%) | |||
I | 334 (5.2) | 233 (5.2) | 101 (5.2) |
II | 2,367 (36.8) | 1,664 (37.0) | 703 (36.5) |
III | 3,692 (57.5) | 2,578 (57.3) | 1,114 (57.8) |
IV | 31 (0.5) | 22 (0.5) | 9 (0.5) |
Laterality, n (%) | |||
Left | 3,263 (50.8) | 2,276 (50.6) | 987 (51.2) |
Right | 3,161 (49.2) | 2,221 (49.4) | 940 (48.8) |
Histology, n (%) | |||
IDC | 5,711 (88.9) | 3,991 (88.7) | 1,720 (89.3) |
ILC | 195 (3.0) | 136 (3.0) | 59 (3.1) |
Other§ | 518 (8.1) | 370 (8.2) | 148 (7.7) |
Surgery, n (%) | |||
No | 388 (6.0) | 272 (6.0) | 116 (6.0) |
Yes | 6,036 (94.0) | 4,225 (94.0) | 1,811 (94.0) |
Radiation, n (%) | |||
No | 2,193 (34.1) | 1,546 (34.4) | 647 (33.6) |
Yes | 4,231 (65.9) | 2,951 (65.6) | 1,280 (66.4) |
Chemotherapy, n (%) | |||
No | 563 (8.8) | 394 (8.8) | 169 (8.8) |
Yes | 5,861 (91.2) | 4,103 (91.2) | 1,758 (91.2) |
Subtype, n (%) | |||
Luminal A | 3,747 (58.3) | 2,627 (58.4) | 1,120 (58.1) |
Luminal B | 1,241 (19.3) | 878 (19.5) | 363 (18.8) |
HER2 enriched | 450 (7.0) | 305 (6.8) | 145 (7.5) |
TNBC | 986 (15.3) | 687 (15.3) | 299 (15.5) |
AJCC stage 7th edition, n (%) | |||
I | 335 (5.2) | 245 (5.4) | 90 (4.7) |
II | 3,356 (52.2) | 2,364 (52.6) | 992 (51.5) |
III | 2,311 (36.0) | 1,589 (35.3) | 722 (37.5) |
IV | 422 (6.6) | 299 (6.6) | 123 (6.4) |
T stage, n (%) | |||
T1 | 1,744 (27.1) | 1,238 (27.5) | 506 (26.3) |
T2 | 3,232 (50.3) | 2,265 (50.4) | 967 (50.2) |
T3 | 1,085 (16.9) | 745 (16.6) | 340 (17.6) |
T4 | 363 (5.7) | 249 (5.5) | 114 (5.9) |
N stage, n (%) | |||
N1 | 4,604 (71.7) | 3,219 (71.6) | 1,385 (71.9) |
N2 | 1,165 (18.1) | 813 (18.1) | 352 (18.3) |
N3 | 655 (10.2) | 465 (10.3) | 190 (9.9) |
Bone metastasis, n (%) | |||
No | 6,168 (96.0) | 4,309 (95.8) | 1,859 (96.5) |
Yes | 256 (4.0) | 188 (4.2) | 68 (3.5) |
Liver metastasis, n (%) | |||
No | 6,306 (98.2) | 4,415 (98.2) | 1,891 (98.1) |
Yes | 118 (1.8) | 82 (1.8) | 36 (1.9) |
Lung metastasis, n (%) | |||
No | 6,351 (98.9) | 4,445 (98.8) | 1,906 (98.9) |
Yes | 73 (1.1) | 52 (1.2) | 21 (1.1) |
†, other: defined as American Indian/Alaska Native, Asian/Pacific Islander; ‡, other: defined as axillary tail of breast, nipple and breast, NOS; §, other: defined as invasive duct mixed with lobular carcinoma, invasive lobular mixed with other types of carcinomas, invasive duct mixed with other types of carcinomas. YBC, young breast cancer; RLNM, regional lymph node metastasis; IQR, interquartile range; IDC, invasive ductal carcinoma; ILC, invasive lobular carcinoma; HER2, human epidermal growth factor receptor 2; TNBC, triple-negative breast cancer; AJCC, American Joint Committee on Cancer; NOS, not otherwise specified.
Risk factors associated with OS
To distinguish the independent risk variables of OS in YBC patients with RLNM, univariate and multivariate Cox regression analyses were carried out. Age, race, marital status, grade, histology, surgery, radiation, subtype, AJCC stage 7th edition, T stage, N stage, bone metastasis, liver metastasis, and lung metastasis were all shown to be possibly linked with OS (all P<0.05) (Table 2). Further analysis was conducted using multivariate Cox regression analysis based on the above risk factors. We found that Black race likely had worse survival than White race (hazard ratio, 1.28; 95% CI: 1.04–1.57; P=0.018), while other races represented a better prognosis (hazard ratio, 0.69; 95% CI: 0.50–0.94; P=0.018). Similarly, grade, histology, surgery, radiation, subtype, AJCC stage 7th edition, T stage and N stage were ultimately regarded as independent predicted variables of OS in our target population.
Table 2
Variables | Univariate analysis | Multivariate analysis | |||
---|---|---|---|---|---|
Hazard ratio (95% CI) | P value | Hazard ratio (95% CI) | P value | ||
Age | 0.96 (0.94–0.97) | <0.001*** | 0.98 (0.96–1) | 0.052 | |
Race | |||||
White | 1 (ref.) | 1 (ref.) | |||
Black | 1.79 (1.47–2.17) | <0.001*** | 1.28 (1.04–1.57) | 0.018* | |
Other† | 0.64 (0.47–0.87) | 0.005** | 0.69 (0.50–0.94) | 0.018* | |
Marital status | |||||
No | 1 (ref.) | 1 (ref.) | |||
Yes | 0.68 (0.58–0.8) | <0.001*** | 0.89 (0.74–1.06) | 0.189 | |
Primary site | |||||
Central | 1 (ref.) | ||||
Inner | 1.18 (0.72–1.93) | 0.515 | |||
Outer | 1.08 (0.68–1.71) | 0.738 | |||
Overlapping | 1.37 (0.86–2.19) | 0.181 | |||
Other‡ | 1.23 (0.76–1.99) | 0.392 | |||
Grade | |||||
I | 1 (ref.) | 1 (ref.) | |||
II | 3.16 (1.39–7.17) | 0.006** | 2.52 (1.11–5.74) | 0.028* | |
III | 7.58 (3.39–16.96) | <0.001*** | 4.11 (1.82–9.32) | 0.001*** | |
IV | 8.78 (2.68–28.78) | <0.001*** | 5.21 (1.57–17.28) | 0.007** | |
Laterality | |||||
Left | 1 (ref.) | ||||
Right | 1.03 (0.87–1.21) | 0.746 | |||
Histology | |||||
IDC | 1 (ref.) | 1 (ref.) | |||
ILC | 0.77 (0.45–1.3) | 0.325 | 1.24 (0.72–2.14) | 0.441 | |
Other§ | 0.55 (0.38–0.8) | 0.002** | 0.62 (0.42–0.92) | 0.016* | |
Surgery | |||||
No | 1 (ref.) | 1 (ref.) | |||
Yes | 0.27 (0.21–0.35) | <0.001*** | 0.67 (0.5–0.9) | 0.007** | |
Radiation | |||||
No | 1 (ref.) | 1 (ref.) | |||
Yes | 0.77 (0.65–0.91) | 0.003** | 0.76 (0.63–0.91) | 0.003** | |
Chemotherapy | |||||
No | 1 (ref.) | ||||
Yes | 1.3 (0.94–1.8) | 0.114 | |||
Subtype | |||||
Luminal A | 1 (ref.) | 1 (ref.) | |||
Luminal B | 0.73 (0.56–0.96) | 0.027* | 0.55 (0.41–0.73) | <0.001*** | |
HER2 enriched | 1.15 (0.81–1.65) | 0.43 | 0.68 (0.47–0.98) | 0.039* | |
TNBC | 3.61 (3.01–4.33) | <0.001*** | 2.77 (2.26–3.4) | <0.001*** | |
AJCC stage 7th edition | |||||
I | 1 (ref.) | 1 (ref.) | |||
II | 4.37 (1.62–11.79) | 0.004** | 2.5 (0.91–6.9) | 0.076 | |
III | 11.71 (4.37–31.43) | <0.001*** | 3.55 (1.25–10.11) | 0.018* | |
IV | 33.58 (12.39–90.99) | <0.001*** | 7.49 (2.5–22.48) | <0.001*** | |
T stage | |||||
T1 | 1 (ref.) | 1 (ref.) | |||
T2 | 2.24 (1.72–2.91) | <0.001*** | 1.62 (1.24–2.12) | <0.001*** | |
T3 | 3.78 (2.84–5.03) | <0.001*** | 1.92 (1.38–2.67) | <0.001*** | |
T4 | 8.57 (6.24–11.79) | <0.001*** | 3.00 (2.08–4.31) | <0.001*** | |
N stage | |||||
N1 | 1 (ref.) | 1 (ref.) | |||
N2 | 2.16 (1.77–2.63) | <0.001*** | 1.67 (1.26–2.21) | <0.001*** | |
N3 | 4.08 (3.34–4.99) | <0.001*** | 2.34 (1.8–3.04) | <0.001*** | |
Bone metastasis | |||||
No | 1 (ref.) | 1 (ref.) | |||
Yes | 4.43 (3.46–5.65) | <0.001*** | 1.23 (0.83–1.82) | 0.312 | |
Liver metastasis | |||||
No | 1 (ref.) | 1 (ref.) | |||
Yes | 4.51 (3.14–6.47) | <0.001*** | 1.28 (0.83–1.98) | 0.272 | |
Lung metastasis | |||||
No | 1 (ref.) | 1 (ref.) | |||
Yes | 7.97 (5.47–11.6) | <0.001*** | 1.24 (0.79–1.93) | 0.351 |
†, other: defined as American Indian/Alaska Native, Asian/Pacific Islander; ‡, other: defined as axillary tail of breast, nipple and breast, NOS; §, other: defined as infiltrating duct mixed with lobular carcinoma, infiltrating lobular mixed with other types of carcinomas, infiltrating duct mixed with other types of carcinomas. *, P≤0.05; **, P≤0.01; ***, P≤0.001. OS, overall survival; YBC, young breast cancer; RLNM, regional lymph node metastasis; CI, confidence interval; IDC, invasive ductal carcinoma; ILC, invasive lobular carcinoma; HER2, human epidermal growth factor receptor 2; TNBC, triple-negative breast cancer; AJCC, American Joint Committee on Cancer; NOS, not otherwise specified.
Establishment and validation of a nomogram for OS
A unique nomogram was created to estimate the 2-, 3-, and 5-year OS for YBC patients with RLNM in the training cohort using prognostic variables such as race, grade, histology, surgery, radiation, subtype, AJCC stage 7th edition, T stage, and N stage that were statistically significant in the multivariable analysis (Figure 2). Patients could acquire specific points of selected risk factors and the points were added together to obtain the corresponding 2-, 3-, and 5-year survival probabilities. Patients with higher scores had a shorter life expectancy, according to the nomogram.
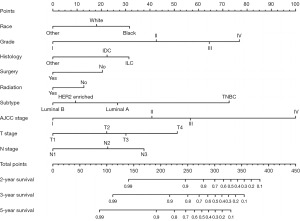
A Harrell’s C-index of 0.786 (95% CI: 0.767–0.805) and 0.791 (95% CI: 0.760–0.822) were obtained from the training and test groups, respectively. Figure 3 depicts the time-dependent ROC for expected 2-, 3-, and 5-year OS. The AUC of the two cohorts did not differ substantially for 2-year (training vs. validation: 0.837 vs. 0.825), 3-year (training vs. validation: 0.795 vs. 0.796), and 5-year (training vs. validation: 0.761 vs. 0.767) OS prediction, suggesting the discriminative ability of the model was generally good. Furthermore, the calibration plots of training and validation cohorts could be observed in Figure 4, indicating remarkable coordination between predicted results and actual survival outcomes. Moreover, DCA curves of 2-, 3-, and 5-year OS in both training and validation cohorts assessed the net benefit of nomogram-assisted decisions at different threshold probabilities, which displayed that our prediction model had a larger net benefit and satisfactory clinical utility (Figure 5). All of above results demonstrated that the nomogram we constructed was a practical clinical tool for estimating survival in YBC patients diagnosed with RLNM.
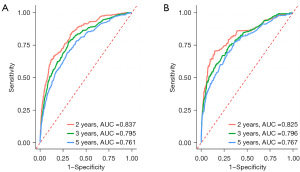
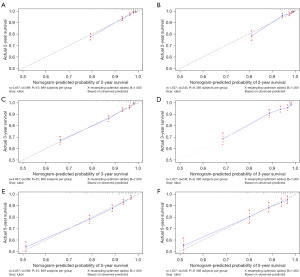
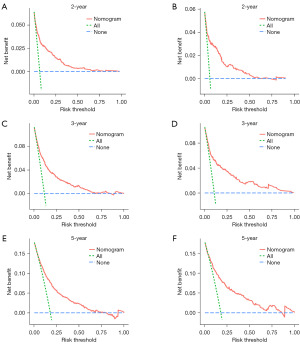
Risk stratification system of patients
Finally, using the total points computed by the nomogram, we created a risk classification system. Two risk groups of YBC patients with RLNM were generated: low risk (training: n=2,251; validation: n=935) and high risk (training: n=2,246; validation: n=992). The OS of the various groups in the training set was clearly segregated according to the risk classification model, as shown by Kaplan-Meier curves (P<0.0001, Figure 6A). Significant OS disparities were also observed in the validation group (P<0.0001, Figure 6B), showing that patients with a low-risk score had a better outcome than those with a high-risk score.
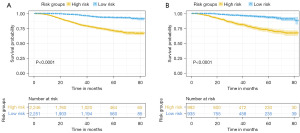
Discussion
For young patients diagnosed with breast cancer, tumor metastasis can result in a poorer prognosis. It is generally acknowledged that breast cancer mainly has three routes of metastasis, including local invasion, lymphatic as well as hematogenous metastasis, among which lymphatic metastasis is the most common pathway of metastasis. The mechanism of lymph node metastasis (LNM) in YBC has not been fully elucidated. According to certain research, the expression of matrix metalloproteinase-9 (MMP-9) was positively connected with LNM in YBC patients, and tumor invasiveness, rather than lymphangiogenesis played a significant role in LNM among YBC patients (27,28). The management of this particular population requires a systematic and multidisciplinary approach and takes account of several specific issues, such as genetic counseling, fertility preservation, reemployment preparation, psychological and sexual distress and so forth, which are crucial to carry out individually targeted treatments (3,29,30). Since the treatments and managements are usually complicated and burdensome, particular attention should be directed to this group. Although numerous nomograms have previously been reported to predict survival in YBC patients (31-35), a reliable and specialized nomogram to predict prognosis in YBC patients with RLNM has yet to be created. Herein, as far as we know, this was the first practical and real-world nomogram based on clinicopathological variables from a large-scale database to predict OS in this unusual group.
In this study, we found that race, grade, histology, surgery, radiation, molecular subtype, AJCC stage 7th edition, T stage as well as N stage were independent predicting variables to predict OS in YBC patients with RLNM in accordance with the outcomes of multivariate Cox regression analysis. To be specific, those patients of Black race, larger tumor sizes, poorer differentiation, triple-negative breast cancer (TNBC) subtype, higher stage, more lymph nodes involvement and without active therapies had a poorer prognosis. In terms of Black race, what Walsh et al. (36) found was partially consistent with our results, which showed that Black women had more nodal disease compared with White counterparts (41.1% vs. 32%, P<0.001), and thus had an increasing hazard of OS and disease-free survival (DFS). Besides, an observational study in the U.S. by Iqbal and colleagues reported that the risk of a Black woman with small-sized breast cancer tumors presenting with nodal metastases was higher than for a non-Hispanic White woman (24.1% vs. 18.4%, respectively, P<0.001) (37). The possible reason may be that young Black patients have inadequate awareness of the disease, less financial support, or differences in tumor biology, leading to a delay in seeking medical attention and a worse prognosis (14,38). On the other hand, molecular subtypes are determined to be closely correlated with OS among YBC patients with RLNM and TNBC tumors accounting for a large proportion, which is similar to previously reported studies (19,39). Azim et al. (40) highlighted the prognostic value of stroma-related gene signatures (such as genes like DCN and PLAU) in the estrogen receptor (ER)−/HER2− subtype among patients aged 40 years or less. Further research is needed to develop treatments to target the stroma and microenvironment for the TNBC subgroup in young women. Additionally, pathological types were considered to be an important prognostic variable in predicting OS in the present study, while laterality and tumor primary sites were not thought to be OS predictors. In contrast, Liu and colleagues determined in another study that in young patients diagnosed with early-stage breast cancer, the inner placement of the original tumor was linked to a poorer cancer-specific survival (CSS) (35). The discrepancies might be explained by differences in patients’ inclusion criteria and primary outcomes.
To integrate and visualize diverse prognostic biologic and clinical variables we confirmed above, a novel medical nomogram that generates probabilities of 2-, 3-, and 5-year OS was subsequently developed for a particular group. Currently, nomograms are commonly used in oncology for their ability to estimate the individualized risk of a clinical event and impact all aspects of cancer treatments and care (41). The nomogram’s performance was typically evaluated using discrimination, calibration, and clinical applicability in both the training and validation sets. The discrimination ability was measured via the C-index and AUC of a receiver operating curve. As shown above, all the values of C-index and AUC in both cohorts were higher than 0.70, which demonstrated that our nomogram had enough discriminating power. Furthermore, in both two groups, the calibration plots revealed a high accordance between the estimated survival rates and the actual survival rates that we observed. The final aspect of evaluating nomogram performance is clinical utility, which looks at whether decisions made with the use of nomograms result in better patient outcomes. DCA curve introduced by Vickers and Elkin is a novel tool that assesses the clinical utility of nomogram based on threshold probability (42), which displayed in the present study suggested our nomogram can assist improve patients outcomes for a wide range of threshold probability. Hence, the nomogram established in this study can fulfill our desire for accurate individualized estimates of OS in YBC patients with RLNM.
Inevitably, certain limitations remain in our current study. To begin, our study is clearly a retrospective cohort study that is inevitably biased by patients’ selection in the SEER database. Second, there is a lack of some proven prognostic factors such as BRCA1/2 gene (43,44), body mass index (BMI) (45,46) and family history (47) in the SEER database, all of which have been found to be highly linked to poorer results for patients diagnosed with breast cancer. Third, we cannot operate further research on the roles of systematic treatments in YBC patients with RLNM, for the reason that detailed information on specific chemotherapy regimens and endocrine therapy was not accessible in the SEER database. Fourth, a younger age is linked to a higher chance of recurrence. Unfortunately, data on disease recurrence is unavailable in the SEER database. As a result, it was unable to evaluate the recurrence risk of YBC patients with RLNM in this study. Fifth, a limitation of the study is the relatively short follow-up period, which may impede a comprehensive understanding of long-term outcomes and disease progression in breast cancer patients. Last but not least, although internal validation had been performed to evaluate the performance of our nomogram, further external validations using other cohorts with the exception of the SEER program are needed to validate model performance. What is more, it is equally urgent to conduct prospective research and enroll more patients to obtain tailored treatment strategies for patients in the future.
Conclusions
In summary, using a large-scale cancer registration database (SEER database), we determined nine risk variables including race, grade, histology, surgery, radiation, molecular subtype, AJCC stage 7th edition, T stage as well as N stage as the independent prognostic variables in the prediction of OS of YBC patients with RLNM. And a brand-new nomogram that predicting 2-, 3-, and 5-year OS in this particular group was successfully established and displayed great discrimination and calibration ability in both training and validation cohorts, which can function as an effective tool for clinicians to distinguish people at high risk and provide suitable individualized treatments.
Acknowledgments
All authors would like to thank SEER for the open access to the database.
Funding: None.
Footnote
Reporting Checklist: The authors have completed the TRIPOD reporting checklist. Available at https://tcr.amegroups.com/article/view/10.21037/tcr-23-1825/rc
Peer Review File: Available at https://tcr.amegroups.com/article/view/10.21037/tcr-23-1825/prf
Conflicts of Interest: All authors have completed the ICMJE uniform disclosure form (available at https://tcr.amegroups.com/article/view/10.21037/tcr-23-1825/coif). The authors have no conflicts of interest to declare.
Ethical Statement: The authors are accountable for all aspects of the work in ensuring that questions related to the accuracy or integrity of any part of the work are appropriately investigated and resolved. The study was conducted in accordance with the Declaration of Helsinki (as revised in 2013). Because of anonymous patient data and free availability for institutional account holders and non-institutional users, informed permission of patients in the SEER database was not necessary. As a result, the Ethics Committee of the Second Affiliated Hospital of Guangzhou Medical University waived ethics approval for this study.
Open Access Statement: This is an Open Access article distributed in accordance with the Creative Commons Attribution-NonCommercial-NoDerivs 4.0 International License (CC BY-NC-ND 4.0), which permits the non-commercial replication and distribution of the article with the strict proviso that no changes or edits are made and the original work is properly cited (including links to both the formal publication through the relevant DOI and the license). See: https://creativecommons.org/licenses/by-nc-nd/4.0/.
References
- Miller KD, Nogueira L, Devasia T, et al. Cancer treatment and survivorship statistics, 2022. CA Cancer J Clin 2022;72:409-36. [Crossref] [PubMed]
- Siegel RL, Miller KD, Fuchs HE, et al. Cancer statistics, 2022. CA Cancer J Clin 2022;72:7-33. [Crossref] [PubMed]
- Rossi L, Mazzara C, Pagani O. Diagnosis and Treatment of Breast Cancer in Young Women. Curr Treat Options Oncol 2019;20:86. [Crossref] [PubMed]
- Fu J, Wu L, Xu T, et al. Young-onset breast cancer: a poor prognosis only exists in low-risk patients. J Cancer 2019;10:3124-32. [Crossref] [PubMed]
- Huang J, Chan PS, Lok V, et al. Global incidence and mortality of breast cancer: a trend analysis. Aging (Albany NY) 2021;13:5748-803. [Crossref] [PubMed]
- Ellington TD, Miller JW, Henley SJ, et al. Trends in Breast Cancer Incidence, by Race, Ethnicity, and Age Among Women Aged ≥20 Years - United States, 1999-2018. MMWR Morb Mortal Wkly Rep 2022;71:43-7. Erratum in: MMWR Morb Mortal Wkly Rep 2022;71:156. [Crossref] [PubMed]
- Silva JDDE, de Oliveira RR, da Silva MT, et al. Breast Cancer Mortality in Young Women in Brazil. Front Oncol 2021;10:569933. [Crossref] [PubMed]
- Villarreal-Garza C, Lopez-Martinez EA, Muñoz-Lozano JF, et al. Locally advanced breast cancer in young women in Latin America. Ecancermedicalscience 2019;13:894. [Crossref] [PubMed]
- Hu X, Myers KS, Oluyemi ET, et al. Presentation and characteristics of breast cancer in young women under age 40. Breast Cancer Res Treat 2021;186:209-17. [Crossref] [PubMed]
- Eiriz IF, Vaz Batista M, Cruz Tomás T, et al. Breast cancer in very young women-a multicenter 10-year experience. ESMO Open 2021;6:100029. [Crossref] [PubMed]
- Paluch-Shimon S, Cardoso F, Partridge AH, et al. ESO-ESMO 4th International Consensus Guidelines for Breast Cancer in Young Women (BCY4). Ann Oncol 2020;31:674-96. [Crossref] [PubMed]
- Villarreal-Garza C, Platas A, Miaja M, et al. Young Women With Breast Cancer in Mexico: Results of the Pilot Phase of the Joven & Fuerte Prospective Cohort. JCO Glob Oncol 2020;6:395-406. [Crossref] [PubMed]
- Billena C, Wilgucki M, Flynn J, et al. 10-Year Breast Cancer Outcomes in Women ≤35 Years of Age. Int J Radiat Oncol Biol Phys 2021;109:1007-18. [Crossref] [PubMed]
- Walsh SM, Zabor EC, Flynn J, et al. Breast cancer in young black women. Br J Surg 2020;107:677-86. [Crossref] [PubMed]
- Kumar R, Abreu C, Toi M, et al. Oncobiology and treatment of breast cancer in young women. Cancer Metastasis Rev 2022;41:749-70. [Crossref] [PubMed]
- McGuire A, Brown JA, Malone C, et al. Effects of age on the detection and management of breast cancer. Cancers (Basel) 2015;7:908-29. [Crossref] [PubMed]
- Siu ALU.S. Preventive Services Task Force. Screening for Breast Cancer: U.S. Preventive Services Task Force Recommendation Statement. Ann Intern Med 2016;164:279-96. Erratum in: Ann Intern Med 2016;164:448. [Crossref] [PubMed]
- Kataoka A, Iwamoto T, Tokunaga E, et al. Young adult breast cancer patients have a poor prognosis independent of prognostic clinicopathological factors: a study from the Japanese Breast Cancer Registry. Breast Cancer Res Treat 2016;160:163-72. [Crossref] [PubMed]
- Sabiani L, Houvenaeghel G, Heinemann M, et al. Breast cancer in young women: Pathologic features and molecular phenotype. Breast 2016;29:109-16. [Crossref] [PubMed]
- Abdel-Razeq H, Almasri H, Abdel Rahman F, et al. Clinicopathological Characteristics And Treatment Outcomes Of Breast Cancer Among Adolescents And Young Adults In A Developing Country. Cancer Manag Res 2019;11:9891-7. [Crossref] [PubMed]
- Gao X, Luo W, He L, et al. Nomogram models for stratified prediction of axillary lymph node metastasis in breast cancer patients (cN0). Front Endocrinol (Lausanne) 2022;13:967062. [Crossref] [PubMed]
- Sopik V, Narod SA. The relationship between tumour size, nodal status and distant metastases: on the origins of breast cancer. Breast Cancer Res Treat 2018;170:647-56. [Crossref] [PubMed]
- Iasonos A, Schrag D, Raj GV, et al. How to build and interpret a nomogram for cancer prognosis. J Clin Oncol 2008;26:1364-70. [Crossref] [PubMed]
- Duggan MA, Anderson WF, Altekruse S, et al. The Surveillance, Epidemiology, and End Results (SEER) Program and Pathology: Toward Strengthening the Critical Relationship. Am J Surg Pathol 2016;40:e94-e102. [Crossref] [PubMed]
- Harrell FE Jr, Lee KL, Mark DB. Multivariable prognostic models: issues in developing models, evaluating assumptions and adequacy, and measuring and reducing errors. Stat Med 1996;15:361-87. [Crossref] [PubMed]
- Capogrosso P, Vickers AJ. A Systematic Review of the Literature Demonstrates Some Errors in the Use of Decision Curve Analysis but Generally Correct Interpretation of Findings. Med Decis Making 2019;39:493-8. [Crossref] [PubMed]
- Hao L, Zhang C, Qiu Y, et al. Recombination of CXCR4, VEGF, and MMP-9 predicting lymph node metastasis in human breast cancer. Cancer Lett 2007;253:34-42. [Crossref] [PubMed]
- Zhang ZQ, Han YZ, Nian Q, et al. Tumor Invasiveness, Not Lymphangiogenesis, Is Correlated with Lymph Node Metastasis and Unfavorable Prognosis in Young Breast Cancer Patients (≤35 Years). PLoS One 2015;10:e0144376. [Crossref] [PubMed]
- Martinez-Cannon BA, Barragan-Carrillo R, Villarreal-Garza C. Young Women with Breast Cancer in Resource-Limited Settings: What We Know and What We Need to Do Better. Breast Cancer (Dove Med Press) 2021;13:641-50. [Crossref] [PubMed]
- Tichy JR, Lim E, Anders CK. Breast cancer in adolescents and young adults: a review with a focus on biology. J Natl Compr Canc Netw 2013;11:1060-9. [Crossref] [PubMed]
- Gong Y, Ji P, Sun W, et al. Development and Validation of Nomograms for Predicting Overall and Breast Cancer-Specific Survival in Young Women with Breast Cancer: A Population-Based Study. Transl Oncol 2018;11:1334-42. [Crossref] [PubMed]
- Lin H, Zhang F, Wang L, et al. Use of clinical nomograms for predicting survival outcomes in young women with breast cancer. Oncol Lett 2019;17:1505-16. [PubMed]
- Sun Y, Li Y, Wu J, et al. Nomograms for prediction of overall and cancer-specific survival in young breast cancer. Breast Cancer Res Treat 2020;184:597-613. [Crossref] [PubMed]
- Cui X, Song D, Li X. Construction and Validation of Nomograms Predicting Survival in Triple-Negative Breast Cancer Patients of Childbearing Age. Front Oncol 2021;10:636549. [Crossref] [PubMed]
- Liu R, Xiao Z, Hu D, et al. Cancer-Specific Survival Outcome in Early-Stage Young Breast Cancer: Evidence From the SEER Database Analysis. Front Endocrinol (Lausanne) 2022;12:811878. [Crossref] [PubMed]
- Walsh SM, Zabor EC, Stempel M, et al. Does race predict survival for women with invasive breast cancer? Cancer 2019;125:3139-46. [Crossref] [PubMed]
- Iqbal J, Ginsburg O, Rochon PA, et al. Differences in breast cancer stage at diagnosis and cancer-specific survival by race and ethnicity in the United States. JAMA 2015;313:165-73. [Crossref] [PubMed]
- Ruddy KJ, Gelber S, Tamimi RM, et al. Breast cancer presentation and diagnostic delays in young women. Cancer 2014;120:20-5. [Crossref] [PubMed]
- Partridge AH, Hughes ME, Warner ET, et al. Subtype-Dependent Relationship Between Young Age at Diagnosis and Breast Cancer Survival. J Clin Oncol 2016;34:3308-14. [Crossref] [PubMed]
- Azim HA Jr, Michiels S, Bedard PL, et al. Elucidating prognosis and biology of breast cancer arising in young women using gene expression profiling. Clin Cancer Res 2012;18:1341-51. [Crossref] [PubMed]
- Balachandran VP, Gonen M, Smith JJ, et al. Nomograms in oncology: more than meets the eye. Lancet Oncol 2015;16:e173-80. [Crossref] [PubMed]
- Vickers AJ, Elkin EB. Decision curve analysis: a novel method for evaluating prediction models. Med Decis Making 2006;26:565-74. [Crossref] [PubMed]
- Guzmán-Arocho YD, Rosenberg SM, Garber JE, et al. Clinicopathological features and BRCA1 and BRCA2 mutation status in a prospective cohort of young women with breast cancer. Br J Cancer 2022;126:302-9. [Crossref] [PubMed]
- Bakkach J, Mansouri M, Derkaoui T, et al. Contribution of BRCA1 and BRCA2 germline mutations to early onset breast cancer: a series from north of Morocco. BMC Cancer 2020;20:859. [Crossref] [PubMed]
- Lee J, Kim H, Bae SJ, et al. Association of Body Mass Index With 21-Gene Recurrence Score Among Women With Estrogen Receptor-Positive, ERBB2-Negative Breast Cancer. JAMA Netw Open 2022;5:e2243935. [Crossref] [PubMed]
- Wang K, Wu YT, Zhang X, et al. Clinicopathologic and Prognostic Significance of Body Mass Index (BMI) among Breast Cancer Patients in Western China: A Retrospective Multicenter Cohort Based on Western China Clinical Cooperation Group (WCCCG). Biomed Res Int 2019;2019:3692093. [Crossref] [PubMed]
- McCarthy AM, Liu Y, Ehsan S, et al. Validation of Breast Cancer Risk Models by Race/Ethnicity, Family History and Molecular Subtypes. Cancers (Basel) 2021;14:45. [Crossref] [PubMed]