Multiomics combined with single-cell analysis shows that mitophagy-related genes could accurately predict the prognosis of patients with clear cell renal cell carcinoma
Highlight box
Key findings
• A prognostic model was established based on mitophagy-related genes, which can accurately predict the prognosis of clear cell renal cell carcinoma (ccRCC) patients.
What is known and what is new?
• Mitophagy has various effects on tumor progression, however, there have been limited studies investigating the impact of mitophagy-related genes on the prognosis of ccRCC patients.
• By utilizing mitophagy-related genes, a prediction model for the prognosis of ccRCC patients was successfully developed and validated.
What is the implication, and what should change now?
• This model is capable of effectively predicting the prognosis of ccRCC patients. Furthermore, the use of nomograms and immune-related analysis can be valuable tools in both diagnosing and treating ccRCC patients.
Introduction
There are many types of kidney tumors, of which renal cell carcinoma (RCC) accounts for approximately 90% (1). RCC is a very heterogeneous tumor with many subtypes, such as papillary RCC, clear cell RCC (ccRCC), and chromophotic RCC, of which ccRCC accounts for 60% to 80% (2-4). ccRCC has a high probability of metastasizing, having the most negative prognosis among all subtypes (1,5). In addition, even in clear cell carcinoma of the kidney, there is still a great deal of tumor heterogeneity (6). Therefore, further research on clear cell carcinoma types of the kidney is warranted to develop a more accurate treatment plan. Recently, the American Cancer Society estimated that the number of kidney and renal pelvis tumors would reach 81,800 by 2023, with an associated number of deaths of 14,890. The disease incidence of deaths in men is approximately twice that of women (7), which is higher than the 2022 estimates by the same organisation (8). Due to the advances in diagnostic technology since the 1990s, the incidence of renal clear cell carcinoma globally has gradually increased, while the mortality rate has decreased given that previously undiagnosed tumors are diagnosed at an early stage and receive timely treatment (1). Although various treatment modalities are rapidly advancing, the prognosis for patients with advanced tumors is still poor (6,9,10). Therefore, advancing the tools that allow a more personalised treatment plan is necessary.
Mitochondria are the energy-processing factories in living organisms that play an important role in cellular life activities (11). When mitochondria age or are exposed to external stimuli such as hypoxia and inflammation, apoptosis and cell death are triggered (12,13). Mitophagy, the removal of damaged mitochondria, is important for maintaining stable cell function and ensuring energy supply. Many researchers have recently studied the relationship between mitophagy and tumor occurrence and metastasis (14). The expression of the ISG15 and ISGylation protein in pancreatic cancer stem cells is necessary to maintain their metabolic plasticity. By inhibiting the expression of ISG15, the mitophagy of pancreatic cancer stem cells can be dysregulated, inhibiting the occurrence and metastasis of pancreatic cancer (15). The mitochondrial autophagy-related genes PINK1 and PARK2 are also independent prognostic markers for survival in patients with RCC and are associated with tumor aggressiveness (16). Furthermore, MAPK1/3 kinase-dependent ULK1 degradation can lead to the attenuation of mitophagy, promoting bone metastasis in breast cancer (17). In ovarian cancer, inducing mitophagy can inhibit the progression of chemotherapy-resistant ovarian cancer (18). Therefore, research on the development of new tumor treatment modalities for mitophagy is gaining increasing popularity (19-21). In addition, RCC is essentially a metabolic disease characterised by the reprogramming of energy metabolism (22-26). In particular, metabolic fluxes are induced by glycolysis and mitochondrial bioenergetics, while OxPhox and lipid metabolism are impaired (27-29). Therefore, we hypothesised that mitophagy is an important regulator of the metabolism of ccRCC cells. However, little is known about the role of mitophagy-related genes in patients with ccRCC.
With the study of the mechanisms associated with mitochondrial autophagy, we have discovered its important role in tumor progression. The development of treatments that target the mechanism of mitophagy has benefited many cancer patients. However, the role of mitophagy-related genes in renal clear cell carcinoma remains unknown. Therefore, this study aimed to obtain the expression profiles of mitophagy-related genes and explore the influence of mitochondrial autophagy-related genes on the prognosis of patients with renal clear cell carcinoma using bioinformatics. We present this article in accordance with the TRIPOD reporting checklist (available at https://tcr.amegroups.com/article/view/10.21037/tcr-23-1765/rc).
Methods
Access to research data using public databases
Using “mitophagy” as the keyword, we retrieved 5,655 mitophagy-related genes (https://www.genecards.org/) from the searchable GeneCards database. In addition, the expression, mutation, and copy data required for analysis were retrieved from The Cancer Genome Atlas (TCGA) database (https://portal.gdc.cancer.gov/). The GSE29609 dataset from the Gene Expression Omnibus (GEO) database (https://www.ncbi.nlm.nih.gov/geo/) was combined with renal clear cell carcinoma data from the TCGA database for prognostic modelling and internal validation. The clear cell renal carcinoma cohort from the International Cancer Genome Consortium (ICGC) (https://dcc.icgc.org/) was used for external validation, while the data from the E-MTAB-1980 cohort provided additional external validation. The GSE40435 and GSE53757 datasets were utilised to validate the model at the gene expression level. In addition, protein expression data were obtained from the University of Alabama at Birmingham Cancer Data Analysis Portal (UALCAN) database (https://ualcan.path.uab.edu/index.html), commonly used for analysing cancer omics data (30,31). The Human Protein Atlas (HPA) (https://www.proteinatlas.org/) provided immunohistochemistry data, while the GSE159115 dataset was used for single-cell analysis (32,33). Finally, the acquisition and analysis of single-cell data were achieved using the Tumor Immune Single-cell Hub (TISCH) (http://tisch.comp-genomics.org/) database (34). The study was conducted in accordance with the Declaration of Helsinki (as revised in 2013).
Preliminary screening of genes using R software and data standardisation
First, we obtained the expression profiles of mitophagy-related genes in patients with clear cell carcinoma of the kidney in the TCGA database using the limma package. The TCGA cohort contained a total of 542 tumor samples as well as 72 normal samples. After differential analysis of expression profiles, we obtained 762 differential genes [log fold change (FC) >1, false discovery rate (FDR) <0.05]. To ensure that the results can be extrapolated to a larger population, the data from this study were drawn from multiple databases. To make data from different databases comparable, we standardised the data from the TCGA, GEO, ICGC, and E-MTAB-1980 using the SVA package. After standardising the gene expression data, we eliminated the samples with incomplete survival information after combining it with clinical information. Finally, we included samples from 759 patients with tumors, including 529 oncology patients from TCGA database, 38 from the GSE29609 dataset, 91 from the ICGC database, and 101 from the E-MTAB-1980 cohort. In addition, using independent prognostic analysis, we screened 340 prognostic-related genes from 762 differential genes. The genes obtained were further screened for mitophagy-related gene model signatures (MRGMS) which were used to build the mitophagy-related gene model (MRGM).
MRGM construction with prognostically associated differential mitophagy-related genes
We combined the TCGA and GSE29609 datasets to form a merged cohort, which was randomly divided into a M (model) and T (test) cohorts (1:1) using the createDataPartition function in the caret package. The samples in the M cohort were used to construct the MRGM. We applied least absolute shrinkage and selection operator (LASSO) regression and cross-validation to obtain the best MRGMS for building the model. After deriving the MRGM formula, each tumor sample obtained an MRGM score. The 759 samples were divided into two groups based on the median patient sample score of the M cohort (model cohort), which was greater than the median value in the H group (high risk group) and less than the median value in the L group (low risk group). Tumor samples from the T cohort (Test cohort) were used internally to verify the accuracy of the MRGM predictions. To ensure that MRGM can accurately predict the prognosis of patients with clear cell carcinoma of the kidney, we performed external verification of MRGM using the ICGC cohort. The E-MTAB-1980 cohort provided an additional external validation. Using principal component analysis (PCA), we observed whether MRGMS can distinguish between patients in groups H and L. The survival analyses of patients in groups H and L in all cohorts enabled us to determine whether the model accurately predicted the prognosis of patients with clear cell carcinoma of the kidney. The area under the receiver operating characteristic (ROC) curve reflected the predictive performance of the MRGM. In addition, the distribution map of survival status provided a direct indication of the ability of the MRGM to predict ccRCC prognosis.
Analysis of MRGMS using multi-omics data
We further explored MRGMS using the multi-omics data. Using the mutation data from TCGA, we explored the mutation of MRGMS in renal clear cell carcinoma and produced a waterfall plot for visualisation. In addition, we located the MRGMS on chromosomes and analysed the copy data. Next, we selected the GSE40435 and GSE53757 datasets to externally verify the differential expression of MRGMS at the gene expression level. In addition, using the protein expression data of renal clear cell carcinoma in the UALCAN database, we performed external verification of MRGMS expression at the protein expression level. Finally, we queried the immunohistochemistry of MRGMS in the HPA database.
Integration of common clinical indicators for building a nomogram
Nomograms are widely used in clinical practice because they can accurately predict the survival rate of patients. Therefore, we combined common clinical indicators with MRGM scores to construct a nomogram. Calibration curves and decision curve analysis (DCA) curves were used to verify the predictive power of nomograms. The area under the ROC curve was utilised to recognise the ability of a nomogram to predict prognosis.
Channel enrichment using gene set variation analysis (GSVA) and other methods
Through GSVA enrichment, we analysed the enrichment of Kyoto Encyclopedia of Genes and Genomes (KEGG) pathways in patients with different MRGM scores. Next, we examined the genes that were expressed differently in patients in groups H and L. Based on the difference analysis, we determined the enrichment of Gene Ontology (GO) pathways in patients with different risk groups.
Exploration of new immunotherapy options using immune-related analysis
Using the CIBERSORT platform, we obtained data on immune cell infiltration for all samples. We analysed the association between immune cell infiltration and MRGMS and MRGM scores. Further, single sample gene set enrichment analysis (ssGSEA) enrichment analysis was applied to determine immune cells and immune-related functions. Finally, we explored the differential expression of immune checkpoint-associated genes in groups H and L.
Analysis of MRGMS using single-cell data
Using the TISCH database, we obtained and annotated the cell clusters in the GSE159115 dataset and observed the distribution of MRGMS in various cell lineages. Next, we analysed the differential expression of MRGMS in tumor and normal tissues in malignant tumor cell lineages.
Statistical analyses
All statistical analyses in this study were performed using R software (version 4.2.2). Unless otherwise stated, a P<0.05 was set as the significance value in this study.
Results
Research process and preliminary data processing
The research process is illustrated in the flowchart (Figure 1). We found 5,655 genes associated with mitophagy. Through differential analysis, we obtained 762 mitophagy-related significantly differentially expressed genes. Among them, 274 and 488 genes were highly and poorly expressed in tumor tissues, respectively. Next, using independent prognostic analysis, we screened out 340 prognostic-related genes from 762 differential genes.
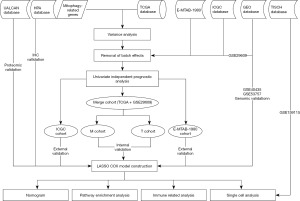
MRGM can stably predict patient prognosis in different cohorts
The LASSO regression as well as cross-validation analyses showed that 2-16 signatures were the most accurate to construct MRGMs (Figure 2A,2B). We identified eight genes as MRGMS (ATP1A1, IGFBP3, LIPA, PLG, IFI16, TUBB6, PSAT1, NOX4). Among these MRGMS, IFI16, TUBB6, and PSAT1, which were positively correlated with MRGM scores, while ATP1A1, IGFBP3, LIPA, PLG, and NOX4 were negatively correlated. The MRGMS were used to derive the following model formula: MRGM score = EXP [(ATP1A1 × −0.198081408034518) + (IGFBP3 × −0.649722900971) + (LIPA × −0.213274094277372) + (PLG × −0.154808702949903) + (IFI16 × 0.518561327288619) + (TUBB6 × 0.378853678087333) + (PSAT1 × 0.261673272955883) + (NOX4 × −0.177373744381922)]. The PCA plot shows that the mitophagy-related gene alone does not distinguish well between patients in the high H and L groups (Figure 2C). However, MRGMS allows for a good distinction between the two groups of patients (Figure 2D). The progression-free survival in group H was much lower than that in group L, and the difference between the two was statistically significant (Figure 2E). First, in the survival analysis curve of the pooled cohort, we can see that the prognosis is worse for the patients in group H (Figure 2F). In addition, the area under the ROC curve at 1-year, 3-year, and 5-year was higher than 0.7 (Figure 2G). Similar results of M and T cohorts were found, where the prognosis was better for patients in the L group and worse for patients with higher MRGM scores (Figure 2H-2K). In addition, the area under the ROC curve confirmed the predictive performance of the model. To extrapolate the model to a wider population, the ICGC and E-MTAB-1980 cohorts validated the model as external data. The survival analysis curve of the ICGC cohort showed a better prognosis and statistically significant difference in patients in the L group (Figure 2L). In addition, the area under the ROC curve of the ICGC cohort was greater than 0.65 (Figure 2M). External data from the ICGC cohort provided an accurate external validation for the results. Further, we provided an additional external validation using data from the E-MTAB-1980 cohort, from which we observed consistent results with the previous internal validation as well as the ICGC cohort external validation (Figure 2N,2O).
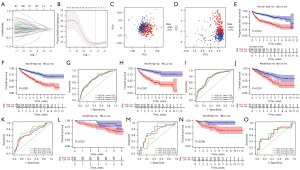
Multiomics data validation of MRGMS
In the TCGA database, we found four MRGMS (LIPA, IGFBP3, TUBB6, and IFI16) that were highly expressed in tumor tissues. PLG, ATP1A1, PSAT1, and NOX4 were poorly expressed in tumor tissue (Figure 3A). We reached consistent conclusions with the external GSE40435 and GSE53757 datasets (Figure 3B,3C). The results of the differential analysis of the protein expression data were also consistent with the gene expression data (Figure 3D). Finally, we queried the immunohistochemistry of six of the MRGMS in the HPA database. The immunohistochemistry of IFI16, LIPA, TUBB6, ATP1A1, PLG, and PSAT1 was also consistent with the gene expression (Figure 3E).
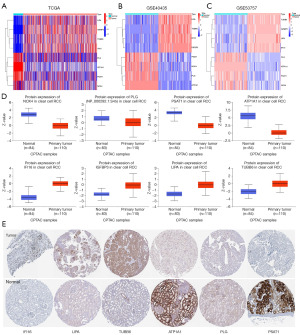
Nomograms can accurately predict patient survival
Using the age, tumor stage, MRGM score, and other information, we accurately predicted the survival rate of patients. For example, the 6th patient in the M cohort had a 1-year survival rate of 0.975, a 3-year survival rate of 0.928, and a 5-year survival rate of 0.881 (Figure 4A). As the duration of the disease increased, the survival rate of the patient gradually decreased, which was consistent with the clinical observations. The calibration curve showed that the predicted results of the nomogram were almost identical to the actual results (Figure 4B). The area under the ROC curve of the nomogram was 0.872, where the larger the area under the ROC curve, the higher the prediction efficiency of the nomogram (Figure 4C). In the DCA decision curve, we can determine that the predictive power of the nomogram was better than other clinical indicators (Figure 4D). Both univariate and multivariate independent prognostic analyses demonstrated that predicting the survival of patients with clear cell renal carcinoma using the nomogram did not depend on other indicators (Figure 4E,4F).
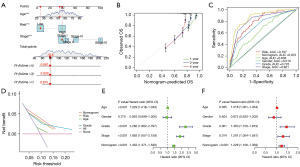
Pathway enrichment analysis
The heat map of the GSVA enrichment analysis showed that the top 50 pathways were predominantly enriched in patients in group L. Only 11 pathways were enriched in patients in group H (Figure 5A). From the GO enrichment analysis, we selected the top 8 pathways to plot circles. On the left side of the circle plot were genes enriched in the pathway, which were connected to the corresponding pathway by a curve. Genes were arranged from top to bottom according to the size of the LogFC value. On the right side of the circle plot, the enrichment pathway was shown, and the larger the area, the more genes were enriched in the pathway. For example, the largest area of the external encapsulating structure organisation and leukocyte migration pathway means that the genes enriched in these two pathways were the largest. In addition, we can observe that in GO results, the three genes were mainly closely related to the biological process (Figure 5B). Finally, the top 10 pathways with the highest number of genes in the GO were shown as bubble and column plots (Figure 5C).
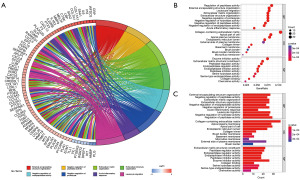
Immune-related analysis
In the ssGSEA analysis, 14 immune cell infiltrates differed between patients in groups H and L (Figure 6A). Further, 10 immune-related functions differed in patients in groups H and L (Figure 6B). Immune checkpoint inhibitors can thus offer new hope for patients with advanced clear cell carcinoma of the kidney. We observed significant differences in groups H and L of 36 immune checkpoint-associated genes, most of which were highly expressed in group H (Figure 6C).
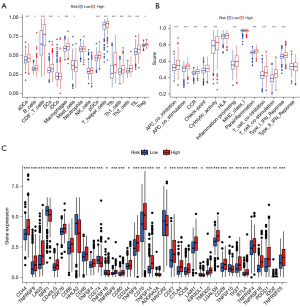
Single-cell data analysis
By clustering, single-cell data from the GSE159115 dataset were divided into 32 cell subtypes (Figure 7A). The annotation of single-cell data using the major lineage demonstrated that cells of subtype 11 and subtype 28 were annotated as CD8 T cells. Cells of subtypes 1, 10, 22, 27, and 29 were annotated as endothelial cells. Cells of subtypes 6, 8, 17, 21, 23, and 24 were annotated as epithelial cells. Cells of subtypes 9 and 31 were annotated as erythroblast cells. Cells of subtypes 0, 3, 7, 13, 14, 16, 19, and 26 were annotated as malignant cells. Cells of subtypes 4, 5, 12, 18, and 32 were annotated as mono/macro cells. Cells of subtypes 2, 15, 20, and 30 were annotated as pericytes. Cells of subtype 25 were annotated as plasma cells (Figure 7B). A total of 1,247 CD8 T cells, 3,798 endothelial cells, 5,477 epithelial cells, 82 erythroblasts, 9,027 malignant cells, 4,730 mono/macro cells, 3,059 pericytes, and 249 plasma cells were obtained from the GSE159115 dataset (Figure 7C). In addition, through the scale column chart, we observed the proportion of various cells in each sample (Figure 7D). Next, we analysed whether MRGMS was expressed differently between tumor and normal tissues in the malignancy lineage cells such as immune, malignant, and stromal cells. The results showed that the expression of ATP1A1, IGFBP3, LIPA, NOX4, PLG, and PSAT1 in immune cells was significantly different between tumor and normal tissues. In the malignant cells, ATP1A1, IFI16, IGFBP3, LIPA, NOX4, and TUBB6 expression differed significantly. In the stromal cells, ATP1A1, IFI16, IGFBP3, NOX4, TUBB6, and PSAT1 expression differed significantly (Figure 7E).
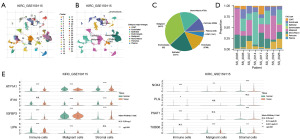
Discussion
We studied the prognostic effect of mitochondrial autophagy-related genes on patients with clear cell carcinoma of the kidney to construct a model. We used multiple datasets to verify the model to ensure the stability of prediction ability. The results of the multi-dataset validation show that the MRGM can stably predict the prognosis of patients in different datasets. In addition, nomograms and immuno-related analysis can assist clinicians in formulating accurate treatment plans. The LASSO regression as well as cross-validation provided eight MRGMS (ATP1A1, IGFBP3, LIPA, PLG, IFI16, TUBB6, PSAT1, NOX4). The multiomics data analysis showed that the MRGMS plays an important role in renal clear cell carcinoma. The results of the single-cell data analysis showed that the MRGMS was expressed differently between tumor and normal tissues in malignancy lineage cells such as immune cells, malignant cells, and stromal cells.
ATP1A1 can inhibit apoptosis and promote growth by encoding the α1 subunit of NKA (35). ATP1A1 is closely related to urinary diseases, such as the formation of kidney stones, a mutation leading to the worsening of aldosterone adenomas, and provides a prognostic predictive value in RCC (36-38). In addition, ATP1A1 can promote the growth of glioma stem cells (39). IFI16 can mediate the growth and metastasis of breast cancer and is also a modulator of breast cancer immunotherapy (40,41). IFI16 also plays a role in the treatment of gliomas (42). Similar to the results of this study, IGFBP3 can be used as a biomarker for prognosis prediction and guidance of treatment of various tumors (43-45). LIPA can be used as a viable therapeutic target for triple-negative breast cancer (46). Earlier bioinformatics studies found that NOX4 can be used as a tumor marker for predicting the prognosis of pancreatic and colon cancers (47,48). PLG has the potential to be a therapeutic target for patients with ccRCC (49). In addition, PLG plays an irreplaceable role in other tumors such as gastric, pancreatic, and liver cancers (50-52). Proteomic data have shown that TUBB6 expression in bladder cancer can predict a high risk of invasion as well as a poor prognosis (53). Similar conclusions were reached in our study of ccRCC. PSAT1 exhibits suppressive effects in a variety of tumors (54-57). In addition, ccRCC is one of the most immunoinvasive tumors (58). The activation of specific metabolic pathways plays an important role in regulating angiogenesis and inflammatory signalling (59). The characteristic microenvironment of tumors heavily influences disease biology and may influence response to systemic therapy (60-63). In addition, mitophagy plays a significant role in regulating immune cell infiltration and microbiota.
In this study, we verified the predictive ability of the model using the E-MTAB-1980 and ICGC cohorts as external data. In addition, we verified the expression patterns of model-related genes from the gene and protein expression levels using relevant databases. The validation results showed the reliability of the conclusions. However, this study was limited by the local patient cohort and experimental validation, which would make our conclusions more reliable.
Conclusions
Mitophagy-related genes are important for predicting the prognosis of clear cell carcinoma of the kidney and are conducive to the development of more personalised treatment plans for patients.
Acknowledgments
We want to thank the data contributors and curators of the TCGA, ICGC, GEO, HPA, UALCAN, and TISCH databases.
Funding: None.
Footnote
Reporting Checklist: The authors have completed the TRIPOD reporting checklist. Available at https://tcr.amegroups.com/article/view/10.21037/tcr-23-1765/rc
Peer Review File: Available at https://tcr.amegroups.com/article/view/10.21037/tcr-23-1765/prf
Conflicts of Interest: All authors have completed the ICMJE uniform disclosure form (available at https://tcr.amegroups.com/article/view/10.21037/tcr-23-1765/coif). The authors have no conflicts of interest to declare.
Ethical Statement: The authors are accountable for all aspects of the work in ensuring that questions related to the accuracy or integrity of any part of the work are appropriately investigated and resolved. The study was conducted in accordance with the Declaration of Helsinki (as revised in 2013).
Open Access Statement: This is an Open Access article distributed in accordance with the Creative Commons Attribution-NonCommercial-NoDerivs 4.0 International License (CC BY-NC-ND 4.0), which permits the non-commercial replication and distribution of the article with the strict proviso that no changes or edits are made and the original work is properly cited (including links to both the formal publication through the relevant DOI and the license). See: https://creativecommons.org/licenses/by-nc-nd/4.0/.
References
- Hsieh JJ, Purdue MP, Signoretti S, et al. Renal cell carcinoma. Nat Rev Dis Primers 2017;3:17009. [Crossref] [PubMed]
- Sung H, Ferlay J, Siegel RL, et al. Global Cancer Statistics 2020: GLOBOCAN Estimates of Incidence and Mortality Worldwide for 36 Cancers in 185 Countries. CA Cancer J Clin 2021;71:209-49. [Crossref] [PubMed]
- Comprehensive molecular characterization of clear cell renal cell carcinoma. Nature 2013;499:43-9. [Crossref] [PubMed]
- Davis CF, Ricketts CJ, Wang M, et al. The somatic genomic landscape of chromophobe renal cell carcinoma. Cancer Cell 2014;26:319-30. [Crossref] [PubMed]
- Vera-Badillo FE, Templeton AJ, Duran I, et al. Systemic therapy for non-clear cell renal cell carcinomas: a systematic review and meta-analysis. Eur Urol 2015;67:740-9. [Crossref] [PubMed]
- Motzer RJ, Jonasch E, Boyle S, et al. NCCN Guidelines Insights: Kidney Cancer, Version 1.2021. J Natl Compr Canc Netw 2020;18:1160-70. [Crossref] [PubMed]
- Siegel RL, Miller KD, Wagle NS, et al. Cancer statistics, 2023. CA Cancer J Clin 2023;73:17-48. [Crossref] [PubMed]
- Siegel RL, Miller KD, Fuchs HE, et al. Cancer statistics, 2022. CA Cancer J Clin 2022;72:7-33. [Crossref] [PubMed]
- Ochocki JD, Khare S, Hess M, et al. Arginase 2 Suppresses Renal Carcinoma Progression via Biosynthetic Cofactor Pyridoxal Phosphate Depletion and Increased Polyamine Toxicity. Cell Metab 2018;27:1263-1280.e6. [Crossref] [PubMed]
- Xu W, Atkins MB, McDermott DF. Checkpoint inhibitor immunotherapy in kidney cancer. Nat Rev Urol 2020;17:137-50. [Crossref] [PubMed]
- Song C, Pan S, Zhang J, et al. Mitophagy: A novel perspective for insighting into cancer and cancer treatment. Cell Prolif 2022;55:e13327. [Crossref] [PubMed]
- Rong Z, Tu P, Xu P, et al. The Mitochondrial Response to DNA Damage. Front Cell Dev Biol 2021;9:669379. [Crossref] [PubMed]
- Ploumi C, Daskalaki I, Tavernarakis N. Mitochondrial biogenesis and clearance: a balancing act. FEBS J 2017;284:183-95. [Crossref] [PubMed]
- Ferro F, Servais S, Besson P, et al. Autophagy and mitophagy in cancer metabolic remodelling. Semin Cell Dev Biol 2020;98:129-38. [Crossref] [PubMed]
- Alcalá S, Sancho P, Martinelli P, et al. ISG15 and ISGylation is required for pancreatic cancer stem cell mitophagy and metabolic plasticity. Nat Commun 2020;11:2682. [Crossref] [PubMed]
- Simon AG, Tolkach Y, Esser LK, et al. Mitophagy-associated genes PINK1 and PARK2 are independent prognostic markers of survival in papillary renal cell carcinoma and associated with aggressive tumor behavior. Sci Rep 2020;10:18857. [Crossref] [PubMed]
- Deng R, Zhang HL, Huang JH, et al. MAPK1/3 kinase-dependent ULK1 degradation attenuates mitophagy and promotes breast cancer bone metastasis. Autophagy 2021;17:3011-29. [Crossref] [PubMed]
- Meng Y, Qiu L, Zeng X, et al. Targeting CRL4 suppresses chemoresistant ovarian cancer growth by inducing mitophagy. Signal Transduct Target Ther 2022;7:388. [Crossref] [PubMed]
- Praharaj PP, Patro BS, Bhutia SK. Dysregulation of mitophagy and mitochondrial homeostasis in cancer stem cells: Novel mechanism for anti-cancer stem cell-targeted cancer therapy. Br J Pharmacol 2022;179:5015-35. [Crossref] [PubMed]
- Onishi M, Yamano K, Sato M, et al. Molecular mechanisms and physiological functions of mitophagy. EMBO J 2021;40:e104705. [Crossref] [PubMed]
- Panigrahi DP, Praharaj PP, Bhol CS, et al. The emerging, multifaceted role of mitophagy in cancer and cancer therapeutics. Semin Cancer Biol 2020;66:45-58. [Crossref] [PubMed]
- di Meo NA, Lasorsa F, Rutigliano M, et al. The dark side of lipid metabolism in prostate and renal carcinoma: novel insights into molecular diagnostic and biomarker discovery. Expert Rev Mol Diagn 2023;23:297-313. [Crossref] [PubMed]
- Lucarelli G, Loizzo D, Franzin R, et al. Metabolomic insights into pathophysiological mechanisms and biomarker discovery in clear cell renal cell carcinoma. Expert Rev Mol Diagn 2019;19:397-407. [Crossref] [PubMed]
- di Meo NA, Lasorsa F, Rutigliano M, et al. Renal Cell Carcinoma as a Metabolic Disease: An Update on Main Pathways, Potential Biomarkers, and Therapeutic Targets. Int J Mol Sci 2022;23:14360. [Crossref] [PubMed]
- De Marco S, Torsello B, Minutiello E, et al. The cross-talk between Abl2 tyrosine kinase and TGFβ1 signalling modulates the invasion of clear cell Renal Cell Carcinoma cells. FEBS Lett 2023;597:1098-113. [Crossref] [PubMed]
- Lasorsa F, Rutigliano M, Milella M, et al. Cancer Stem Cells in Renal Cell Carcinoma: Origins and Biomarkers. Int J Mol Sci 2023;24:13179. [Crossref] [PubMed]
- Lucarelli G, Rutigliano M, Sallustio F, et al. Integrated multi-omics characterization reveals a distinctive metabolic signature and the role of NDUFA4L2 in promoting angiogenesis, chemoresistance, and mitochondrial dysfunction in clear cell renal cell carcinoma. Aging (Albany NY) 2018;10:3957-85. [Crossref] [PubMed]
- Bombelli S, Torsello B, De Marco S, et al. 36-kDa Annexin A3 Isoform Negatively Modulates Lipid Storage in Clear Cell Renal Cell Carcinoma Cells. Am J Pathol 2020;190:2317-26. [Crossref] [PubMed]
- Lucarelli G, Rutigliano M, Loizzo D, et al. MUC1 Tissue Expression and Its Soluble Form CA15-3 Identify a Clear Cell Renal Cell Carcinoma with Distinct Metabolic Profile and Poor Clinical Outcome. Int J Mol Sci 2022;23:13968. [Crossref] [PubMed]
- Chandrashekar DS, Bashel B, Balasubramanya SAH, et al. UALCAN: A Portal for Facilitating Tumor Subgroup Gene Expression and Survival Analyses. Neoplasia 2017;19:649-58. [Crossref] [PubMed]
- Chandrashekar DS, Karthikeyan SK, Korla PK, et al. UALCAN: An update to the integrated cancer data analysis platform. Neoplasia 2022;25:18-27. [Crossref] [PubMed]
- Thul PJ, Lindskog C. The human protein atlas: A spatial map of the human proteome. Protein Sci 2018;27:233-44. [Crossref] [PubMed]
- Digre A, Lindskog C. The Human Protein Atlas-Spatial localization of the human proteome in health and disease. Protein Sci 2021;30:218-33. [Crossref] [PubMed]
- Sun D, Wang J, Han Y, et al. TISCH: a comprehensive web resource enabling interactive single-cell transcriptome visualization of tumor microenvironment. Nucleic Acids Res 2021;49:D1420-30. [Crossref] [PubMed]
- Aperia A. 2011 Homer Smith Award: To serve and protect: classic and novel roles for Na+, K+ -adenosine triphosphatase. J Am Soc Nephrol 2012;23:1283-90. [Crossref] [PubMed]
- Li Y, Lu X, Yu Z, et al. Meta-data analysis of kidney stone disease highlights ATP1A1 involvement in renal crystal formation. Redox Biol 2023;61:102648. [Crossref] [PubMed]
- Kobuke K, Oki K, Gomez-Sanchez CE, et al. ATP1A1 Mutant in Aldosterone-Producing Adenoma Leads to Cell Proliferation. Int J Mol Sci 2021;22:10981. [Crossref] [PubMed]
- He Y, Zhang P, Zhang D, et al. Combined assessment of low PGRMC1/positive ATP1A1 levels has enhanced prognostic value for renal cell carcinoma. Oncol Rep 2018;40:1467-76. [Crossref] [PubMed]
- Yu Y, Chen C, Huo G, et al. ATP1A1 Integrates AKT and ERK Signaling via Potential Interaction With Src to Promote Growth and Survival in Glioma Stem Cells. Front Oncol 2019;9:320. [Crossref] [PubMed]
- Ong LT, Lee WC, Ma S, et al. IFI16-dependent STING signaling is a crucial regulator of anti-HER2 immune response in HER2+ breast cancer. Proc Natl Acad Sci U S A 2022;119:e2201376119. [Crossref] [PubMed]
- Liu C, Li L, Hou G, et al. HERC5/IFI16/p53 signaling mediates breast cancer cell proliferation and migration. Life Sci 2022;303:120692. [Crossref] [PubMed]
- Gao Z, Xu J, Fan Y, et al. ARPC1B promotes mesenchymal phenotype maintenance and radiotherapy resistance by blocking TRIM21-mediated degradation of IFI16 and HuR in glioma stem cells. J Exp Clin Cancer Res 2022;41:323. [Crossref] [PubMed]
- Kuhn H, Frille A, Petersen MA, et al. IGFBP3 inhibits tumor growth and invasion of lung cancer cells and is associated with improved survival in lung cancer patients. Transl Oncol 2023;27:101566. [Crossref] [PubMed]
- Zhong Z, Xu X, Han S, et al. Comprehensive Analysis of Prognostic Value and Immune Infiltration of IGFBP Family Members in Glioblastoma. J Healthc Eng 2022;2022:2929695. [Crossref] [PubMed]
- Ding TY, Peng YH, Hong CQ, et al. Serum insulin-like growth factor binding protein 3 as a promising diagnostic and prognostic biomarker in esophagogastric junction adenocarcinoma. Discov Oncol 2022;13:128. [Crossref] [PubMed]
- Liu X, Viswanadhapalli S, Kumar S, et al. Targeting LIPA independent of its lipase activity is a therapeutic strategy in solid tumors via induction of endoplasmic reticulum stress. Nat Cancer 2022;3:866-84. [Crossref] [PubMed]
- Zhao X, He Y, Pan Y, et al. Integrated clinical analysis and data mining assessed the impact of NOX4 on the immune microenvironment and prognosis of pancreatic cancer. Front Oncol 2023;13:1044526. [Crossref] [PubMed]
- Yang X, Yu Y, Wang Z, et al. NOX4 has the potential to be a biomarker associated with colon cancer ferroptosis and immune infiltration based on bioinformatics analysis. Front Oncol 2022;12:968043. [Crossref] [PubMed]
- Peng L, Cao Z, Wang Q, et al. Screening of possible biomarkers and therapeutic targets in kidney renal clear cell carcinoma: Evidence from bioinformatic analysis. Front Oncol 2022;12:963483. [Crossref] [PubMed]
- Qu X, Zhang L, Li S, et al. m(6) A-Related Angiogenic Genes to Construct Prognostic Signature, Reveal Immune and Oxidative Stress Landscape, and Screen Drugs in Hepatocellular Carcinoma. Oxid Med Cell Longev 2022;2022:8301888. [Crossref] [PubMed]
- Shi H, Duan J, Chen Z, et al. A prognostic gene signature for gastric cancer and the immune infiltration-associated mechanism underlying the signature gene, PLG. Clin Transl Oncol 2023;25:995-1010. [Crossref] [PubMed]
- Kou YQ, Yang YP, Pan ZJ, et al. Prognostic-Related Biomarkers in Pancreatic Ductal Adenocarcinoma Correlating with Immune Infiltrates Based on Proteomics. Med Sci Monit 2023;29:e938785. [Crossref] [PubMed]
- Kim B, Jung M, Moon KC, et al. Quantitative proteomics identifies TUBB6 as a biomarker of muscle-invasion and poor prognosis in bladder cancer. Int J Cancer 2023;152:320-30. [Crossref] [PubMed]
- Li S, Yang H, Li W, et al. ADH1C inhibits progression of colorectal cancer through the ADH1C/PHGDH /PSAT1/serine metabolic pathway. Acta Pharmacol Sin 2022;43:2709-22. [Crossref] [PubMed]
- Wang M, Yue S, Yang Z. Downregulation of PSAT1 inhibits cell proliferation and migration in uterine corpus endometrial carcinoma. Sci Rep 2023;13:4081. [Crossref] [PubMed]
- Luo MY, Zhou Y, Gu WM, et al. Metabolic and Nonmetabolic Functions of PSAT1 Coordinate Signaling Cascades to Confer EGFR Inhibitor Resistance and Drive Progression in Lung Adenocarcinoma. Cancer Res 2022;82:3516-31. [Crossref] [PubMed]
- Jiang J, Chen HN, Jin P, et al. Targeting PSAT1 to mitigate metastasis in tumors with p53-72Pro variant. Signal Transduct Target Ther 2023;8:65. [Crossref] [PubMed]
- Vuong L, Kotecha RR, Voss MH, et al. Tumor Microenvironment Dynamics in Clear-Cell Renal Cell Carcinoma. Cancer Discov 2019;9:1349-57. [Crossref] [PubMed]
- Netti GS, Lucarelli G, Spadaccino F, et al. PTX3 modulates the immunoflogosis in tumor microenvironment and is a prognostic factor for patients with clear cell renal cell carcinoma. Aging (Albany NY) 2020;12:7585-602. [Crossref] [PubMed]
- Lasorsa F, di Meo NA, Rutigliano M, et al. Immune Checkpoint Inhibitors in Renal Cell Carcinoma: Molecular Basis and Rationale for Their Use in Clinical Practice. Biomedicines 2023;11:1071. [Crossref] [PubMed]
- Ghini V, Laera L, Fantechi B, et al. Metabolomics to Assess Response to Immune Checkpoint Inhibitors in Patients with Non-Small-Cell Lung Cancer. Cancers (Basel) 2020;12:3574. [Crossref] [PubMed]
- Lucarelli G, Netti GS, Rutigliano M, et al. MUC1 Expression Affects the Immunoflogosis in Renal Cell Carcinoma Microenvironment through Complement System Activation and Immune Infiltrate Modulation. Int J Mol Sci 2023;24:4814. [Crossref] [PubMed]
- Lasorsa F, Rutigliano M, Milella M, et al. Cellular and Molecular Players in the Tumor Microenvironment of Renal Cell Carcinoma. J Clin Med 2023;12:3888. [Crossref] [PubMed]