Identification and validation of mitophagy-related signatures as a novel prognostic model for colorectal cancer
Highlight box
Key findings
• A comprehensive analysis of key modulators of mitophagy involved in the progression and prognosis of colorectal cancer (CRC) could guide clinical judgment and provide patients with new therapeutic alternatives.
What is known and what is new?
• Mitophagy is associated with CRC tumorigenesis.
• Specific roles of different mitophagy pathways in human cancers are clarified in order to propose mitotic modulators for anti-cancer therapies.
What is the implication, and what should change now?
• We have developed and validated mitophagy-related signatures that can not only predict the survival outcomes of CRC patients but also reflect the immune status of CRC. Prospective clinical trials and mechanistic explorations are required to validate the current results.
Introduction
According to recent global cancer statistics, colorectal cancer (CRC) is the third most common malignancy of the digestive system in terms of incidence and mortality (1,2). CRC is frequently discovered at an advanced stage because patients at the early stage rarely experience obvious symptoms. Despite the major advancements in surgical treatment, chemotherapy, and biologic therapy in recent years, metastatic CRC has a poor prognosis, with a 5-year survival rate of less than 20% (3,4). Therefore, the molecular mechanisms of CRC development need to be understood in order to find new biomarkers for CRC early detection and prognosis evaluation.
Mitophagy is a conserved cellular process that plays an essential role in maintaining cellular homeostasis by precisely removing dysfunctional mitochondria. Mitochondria are major organelles in cells that play an important role in energy production, redox homeostasis, and apoptosis. It has now been well described that mitochondria sustain tumor growth and cancer progression (5). The biological relevance is further shown by the association of mitophagy deficit with a number of clinical diseases, including neurodegeneration, heart failure, aging, and cancer. Since accumulation of dysfunctional mitochondria is involved in tumorigenesis, its function has been associated with tumor suppression (6,7). The mitochondrially targeted PINK1/Parkin and BNIP3/NIX/FUNDC1 pathways are well-established synergistic mechanisms for the disposal of dysfunctional mitochondria, executing ubiquitin-dependent mitophagy (8,9). In hepatocellular carcinoma (HCC), PINK1 mutations or depletion are associated with poor clinical outcomes. PINK1 knockdown reduces the levels of oxidative stress, mitochondrial mass, and mitochondrial cristae and promotes the growth of HCC cells (10). In gastric cancer, the loss of PINK is correlated with the poorer overall survival (OS) in advanced stages. Under both normoxic and hypoxic environments, PINK1 loss promotes gastric cancer cell proliferation and migration by inhibiting mitophagy, producing mitochondrial reactive oxygen species (mtROS), stabilizing hypoxia inducible factor 1 (HIF-1), and facilitating the Warburg effect (11).
The degradation of damaged mitochondria and the recycling of metabolic precursors by mitophagy could improve cell survival and prevent cell death in several cancer types. A study showed that melanoma development was inhibited in Parkin-KO mice through the maintenance of MFN2 levels and the suppression of Parkin’s ability to ubiquitinate, suggesting a pro-tumor role of Parkin-dependent mitophagy (12). BNIP3 has been found to be downregulated in pancreatic cancers but upregulated in breast ductal carcinoma (13,14). A high level of FUNDC1 expression is related with poor prognosis in cervical cancer (15). A growing number of studies suggest the regulatory role of mitophagy in tumorigenesis and drug resistance, and that it may be a promising anti-cancer strategy (16-18). These studies have also demonstrated the different roles of different mitophagy pathways in tumorigenesis. Therefore, we need to further clarify the unique involvement of distinct mitophagy pathways in human malignancies in order to propose effective mitotic modulators for anticancer therapies.
To date, some studies have suggested that mitophagy is implicated in CRC tumorigenesis. Pharmacological inhibition of the AMPK signaling cascade stimulates mitophagy and abrogates colon cancer cell proliferation (19). Mitophagy protein PINK1 suppresses colon tumor growth by metabolic reprogramming and reducing acetyl-CoA production (20). DJ-1 inhibition is a promising therapeutic approach for the treatment of CRC, as DJ-1 induces mitophagy which promotes tumor progression (21). In addition, another study showed that mitochondria-targeted drugs to stimulate mitophagy provide an attractive approach for therapeutic intervention in colon cancer (19). The majority of studies have focused on the stimulation of mitophagy by anti-cancer drugs and its relationship with drug resistance. However, the role of mitophagy in CRC progression remains poorly understood. Therefore, a comprehensive analysis of key modulators of mitophagy involved in the progression and prognosis of CRC can potentially guide clinical judgment and provide patients with new therapeutic alternatives.
In this study, we aimed to identify a mitophagy-related signature of CRC patients based on the gene expression profiles in The Cancer Genome Atlas (TCGA) and Gene Expression Omnibus (GEO) databases. A total of 27 differentially expressed mitophagy-related genes were identified between tumor samples and normal tissues. A prognostic signature including ATG12, MAP1LC3B, TOMM22, and TOMM40 was constructed which showed moderate predictive ability for the OS of CRC patients. Moreover, we tried to explore the association between mitophagy regulators and immune microenvironment by gene and functional enrichment analysis. We developed and validated a mitophagy score (mtscore) model to predict prognosis and evaluate treatment sensitivity and therapeutic compounds. This mitophagy-related signature is not only a viable potential therapeutic target, but it may also be an indication of immune dysregulation within the tumor microenvironment and a reliable prognostic biomarker for CRC. We present this article in accordance with the TRIPOD reporting checklist (available at https://tcr.amegroups.com/article/view/10.21037/tcr-23-785/rc).
Methods
Database search and data collection
The TCGA database (https://portal.gdc.cancer.gov/) was used to download the RNA sequencing and the corresponding clinical data of CRC patients. A total of 616 CRC samples and 51 normal case samples were included in the study. The collected clinical data included age, gender, and survival prognosis. Somatic mutation data of CRC patients were downloaded and the process of visualizing somatic mutations was performed using maftools package of R (22). Copy number variation (CNV) data of CRC patients were also downloaded and visualized at UCSC Xena (https://xena.ucsc.edu/). In addition, RNA-seq data and clinical survival information of the GSE17536 (23) and GSE39582 (24) dataset for CRC samples were obtained from the GEO (https://www.ncbi.nlm.nih.gov/geo/). Excluded from this analysis were samples with incomplete data on gender, age, survival time, survival status, and pathological grading. The three datasets were normalized separately, combined and de-batch effected using the combat algorithm of the surrogate variable analysis (SVA) package. The final expression matrix was obtained for a total of 1,152 patients. This study was conducted in accordance with the Declaration of Helsinki (as revised in 2013).
Identification of mitophagy genes in CRC
A total of 27 genes associated with mitophagy were downloaded from the Pathway Unification database (https://pathcards.genecards.org/), including ATG12, ATG5, CSNK2A1, CSNK2A2, CSNK2B, FUNDC1, MAP1LC3A, MAP1LC3B, MFN1, MFN2, MTERF3, PGAM5, PINK1, PRKN, RPS27A, SQSTM1, SRC, TOMM20, TOMM22, TOMM40, TOMM5, TOMM70, UBA52, UBB, UBC, ULK1, and VDAC1. All of these 27 genes were evaluated in TCGA-colon adenocarcinoma (COAD) data for expression and mutation information.
Correlation analysis of mitophagy-related genes and immune characteristics
In order to estimate the number of specific infiltrating immune cells and the activity of specific immune response, single-sample gene set enrichment analysis (ssGSEA) (25) was conducted. Each sample was given an enrichment score to indicate the absolute enrichment of that set of genes. The list of genes in the infiltrating immune cell gene set was obtained from a previous study (26) and the immune response gene set was obtained from the ImmPort database (http://www.immport.org) (27). This enrichment score represented the absolute enrichment in immune cell abundance and immune response. Spearman correlation analysis was conducted to examine the relationship between mitochondrial autophagy-related genes and the proportion of immune cells and immune response activity.
Unsupervised clustering analysis of mitophagy modification patterns of CRC
Different mitochondrial autophagy modification patterns were identified based on the expression of 27 mitochondrial autophagy regulatory genes. Clustering was performed using the R package ConsensusClusterPlus (28) and the number of clusters and robustness were evaluated. Principal component analysis (PCA) further validated the expression patterns of mitochondrial autophagy regulators under different modification patterns. Kruskal test was used to compare mitochondrial autophagy regulatory gene expression, immune infiltrating cell abundance scores, and immune response scores for different modification patterns.
Differentially expressed gene (DEG) screening
To analyze the effect of different mitochondrial autophagy subtypes on CRC, differential gene analysis was performed using the R package “limma” (29) to screen for significant DEGs in different mitochondrial autophagy subtypes. A Padj value <0.01 and log2-fold change (FC) >0.5 were used to screen DEGs. Genes with log2FC >0.5 and Padj <0.01 were up-regulated expression, whereas genes with log2FC <−0.5 and Padj <0.01 were down-regulated expression.
Functional and pathway enrichment analysis of DEGs
A common method for conducting large-scale functional enrichment studies of genes, including biological process (BP), molecular function (MF), and cellular component (CC), is Gene Ontology (GO) functional annotation analysis. The Kyoto Encyclopedia of Genes and Genomes (KEGG) is a widely used database that contains information about genomes, biological pathways, diseases, and drugs. GO functional annotation analysis and KEGG pathway enrichment analysis of DEGs among mitochondrial autophagic subtypes were performed using the R package clusterProfiler (30), and a P value <0.05 was considered statistically significant.
Construction of a mtscore model
To quantify the mitochondrial autophagy modification patterns in individual CRC patients, we constructed a mitochondrial autophagy scoring scheme using PCA. First, DEGs among different mitochondrial autophagy modification clusters were analyzed by univariate Cox regression models, and the genes with significant prognostic impact were selected to cluster the samples to construct the mitochondrial autophagy score. A consensus clustering algorithm was used to determine the number and stability of gene clusters. Subsequently, we constructed a mitochondrial autophagy-related gene model using PCA, and extracted principal components 1 and 2 from the model. In addition, the PCA algorithm could effectively reduce data dimensionality and preserve the original data information to a large extent. We defined the mitochondrial autophagy score using a method similar to that previously reported in the literature (31):
Where it is the expression of exogenous genes associated with the mitochondrial autophagy phenotype.
Validation of mtscores
To validate the reliability and clinical application of the mitochondrial autophagy score, a nomogram was constructed using gender, disease classification, and mitochondrial autophagy score to individually predict the 1-, 3-, and 5-year survival rates of patients. Calibration curves were plotted to show the predictive performance of the nomogram. The R package Survminer and ReGlot were used to calculate and plot the graph.
Statistical analysis
All data processing and analysis in this study were conducted using R software (Version 4.2.0; The R Foundation for Statistical Computing, Vienna, Austria). Continuous variables were presented as mean ± standard deviation. The comparison between two groups was performed using the Wilcoxon rank-sum test. For comparisons involving three or more groups, we employed the Kruskal-Wallis test. Either the chi-square test or Fisher’s exact test was utilized to assess the statistical significance between two categorical variables. Spearman correlation analysis was conducted to calculate the correlation coefficients between different variables, with a P value <0.05 considered statistically significant.
Results
Landscape of mitophagy regulators in CRC
We analyzed genomic mutations and messenger RNA (mRNA) to identify the expression of mitophagy regulators in CRC. First, we performed a comprehensive analysis of mitophagy regulators expression in CRC and normal tissues from TCGA data. We obtained 27 mitophagy regulators in this study. Among the 27 genes, only MFN2, UBB, PINK1, and PRKN were significantly downregulated in CRC samples compared to normal samples, whereas most of other genes were significantly upregulated (Figure 1A,1B). PRKN and PINK1 had a significantly higher proportion of CNV in the 27 genes when compared to normal samples (Figure 1C). Waterfall plots showed low frequency of mutations of mitophagy regulators, the frequency was 16.75%. ULK1 exhibited the highest mutation frequency (Figure 1D). The localization of CNV alterations in mitophagy regulators on chromosomes are shown in Figure 1E.
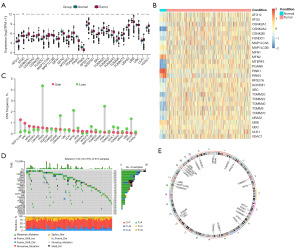
Expression correlation and prognostic analysis of mitophagy regulators
We used the COMBAT algorithm in the SVA package to merge the 3 data sets and remove the batch effect. The PCA plots before (Figure 2A) and after (Figure 2B) data merging showed that the batch effect was well removed.
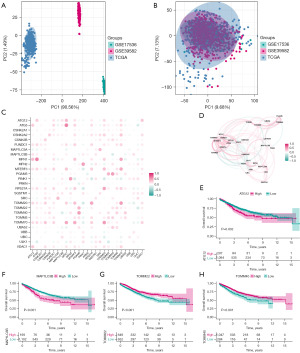
We performed a correlation analysis of the expression of mitophagy regulating genes. We used the corrr package to plot the correlation results as a heat map (Figure 2C) and a network graph (Figure 2D). Almost all mitophagy-related genes demonstrated strong positive correlations in CRC tissues. We analyzed the prognostic role of mitophagy-associated genes in CRC. The results showed that high expression of ATG12 (Figure 2E) and MAP1LC3B (Figure 2F) genes was associated with poor prognosis, whereas high expression of TOMM22 (Figure 2G) and TOMM40 (Figure 2H) genes predicted good prognosis.
Correlation between mitophagy regulators and immune cells and immune processes in CRC
To investigate the correlation between mitophagy regulators and immune microenvironment, we performed a correlation analysis between mitophagy regulators and immune cell infiltration and immune response gene sets. The results revealed the correlation between the abundance of 23 immune microenvironment infiltrating cells and the expression of mitophagy-related genes in CRC. MAP1LC3B, PINK1, UBB, and UBC were positively correlated with most of the immune cells, whereas CSNK2A2, FUNDC1, VDAC1, RPS27A, UBA52, and TOMM20 were negatively correlated with immune cells (Figure 3A). In immune process similar to immune microenvironment, MAP1LC3B, PINK1, UBB, and UBC were positively correlated with most of the immune process, whereas CSNK2A2, RPS27A, UBA52, and TOMM20 were negatively correlated with the immune process (Figure 3B). It is suggested that MAP1LC3B, PINK1, UBB, and UBC are positive regulators and CSNK2A2, RPS27A, UBA52, and TOMM20 are negative regulators of the immune microenvironment.
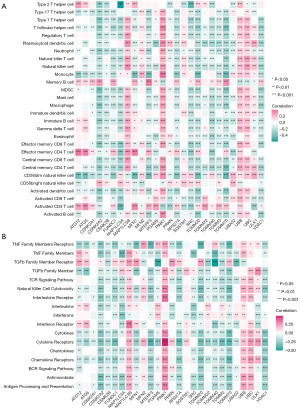
Mitophagy-related CRC modification patterns
To investigate mitophagy regulators’ modification pattern of CRC, we performed an unsupervised consensus clustering analysis based on the expression of 27 mitophagy regulators in tumor samples (Figure 4A-4C). The results showed that the clusters had good stability when K=2. PCA showed that the two mitophagy subtypes were well differentiated (Figure 4D). The gene expression pattern of mitophagy regulators was shown by using box line plot (Figure 4E) and heat map (Figure 4F). Next, we performed differential analysis of the two subtypes with GO and KEGG enrichment analyses for the differential genes. Figure S1A,S1B shows the results of DEG enrichment analysis between two mitophagy subtypes, which could mainly enrich to chromosomes and mitochondria and DNA pathway, indicating good clustering results. The differences in immune cell abundance and immune processes between the two subtypes are shown in Figure S1C,S1D. Cluster 2 had higher immune activity compared to cluster 1.
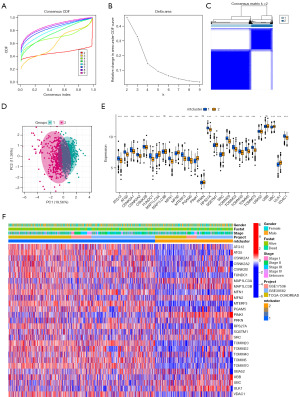
Construction of CRC subtypes with different mitophagy modification patterns
Although a consensus clustering algorithm based on 27 mitochondrial autophagy regulators classified colon cancer patients into two subtypes, the potential genetic changes and prognostic relevance in these phenotypes are not well understood. Based on the univariate Cox regression analysis of 873 DEGs in identified mitophagy clusters, we identified 305 survival-related genes as signature genes of mitophagy. An unsupervised consensus clustering analysis was then performed on colon cancer samples based on the expression of 305 mitophagy-related signature genes (Figure 5A-5C). The results showed that clustering had good stability when K=2. PCA analysis showed significant differences between the two mitophagy subtypes (Figure 5D), and the specificity of mitophagy-related signature gene expression between the two subtypes was shown by using boxplot (Figure 5E). Survival analysis showed better prognosis for subtype B (Figure 5F). The gene expression pattern of mitophagy regulators was shown using a heatmap of hierarchical clustering (Figure 5G). Figure S2 shows the variability in immune cell abundance and immune processes between the two subtypes. Subtype B exhibited higher immune activity.
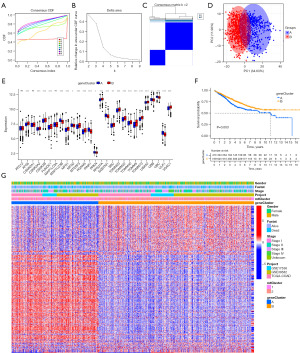
Calculation of mtscore
Although the above results confirm the role of mitophagy in regulating immune cell infiltration and prognosis, these analyses do not accurately predict the pattern of mitophagy modifications in individual cancer patients. To obtain a quantitative index of mitophagy modifications in CRC patients, we extracted the scores of principal components 1 and 2 to calculate the final mtscore. We plotted the Sankey diagram to demonstrate the process of mtscore construction (Figure 6A). The results showed that patients with high mtscore have a worse prognosis than those with low mtscore (Figure 6B). We compared the difference of mtscore in different outcome events, different mtclusters, and different geneclusters. The results showed that the patients in the group of dead, mtcluster 2, and genecluster B had a higher mtscore (Figure 6C-6E). There is a strong correlation between mtscore and immune cell abundance and immune process score, indicating that mtscore has a predictive effect on immune cell infiltration of tumor (Figure 6F,6G).
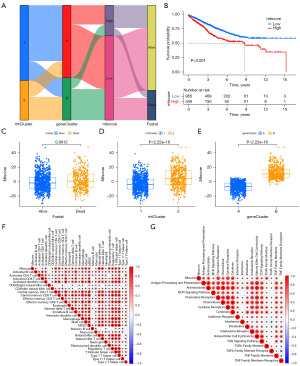
The clinical predictive role of mtscore
A nomogram could be a useful tool for quantitatively assessing individual risk in a clinical setting. We constructed a nomogram and evaluated its predictive effect on the prognosis of CRC patients (Figure 7). The nomogram was built with age, tumor stage, and mtscore incorporated estimating 1-, 3-, and 5-year OS for CRC patients (Figure 7A,7C). A calibration curve was used to validate the predictive accuracy of the nomogram (Figure 7B,7D). The results indicated that mtscore could be used as a novel effective clinical predictor and combined with other clinical characteristics to predict the prognosis of CRC patients.
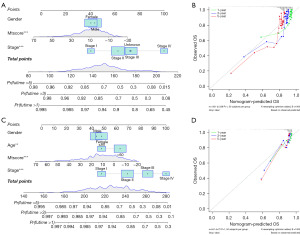
Discussion
Early detection and treatment of CRC have long been a great concern because it is one of the primary causes of cancer death. In the past decades, traditional treatments and combination chemotherapy had been proven ineffective in treating patients with recurrent and refractory CRC (32). Therefore, we need to further explore biomarkers associated with CRC, and provide a research base for clinical diagnosis and treatment.
There have been some CRC hub gene studies based on bioinformatics analyses, but very few studies targeting mitophagy (33,34). The role of mitophagy in inflammation, immunity, and tumor progression has gained widespread attention from researchers. The interactions among mitophagy regulators are complex; many previous studies have focused on individual regulators, leaving open questions about the overall characteristics of integrated roles of different mitophagy regulators. Therefore, the study of distinct mitophagy alteration patterns in CRC will help us to gain a better understanding of tumorigenesis and progression, as well as shed light on the development of novel therapeutic and prognostic strategies for CRC (35).
Previous studies had revealed that mitophagy has a role in tumor growth in some malignancies, such as HCC and breast cancer (36,37). Mitophagy is essential for maintaining cellular homeostasis by selectively removing dysfunctional mitochondria. However, at present the functions and immune implications of mitophagy in CRC have not been elucidated. In this study, we integratedly analyzed 2 microarray datasets from GEO and RNA sequencing data from TCGA. The results showed that almost all mitochondrial autophagy-related genes are abnormally expressed in CRC. We identified 21 upregulated genes and 5 downregulated genes between CRC tissues and normal tissues by bioinformatics method. Compared to normal tissues, PRKN and PINK1 with the high frequency of copy number losses showed significantly lower expression in CRC. This result is consistent with previous knowledge that the expression level of PINK1 was decreased in CRC compared to normal colorectal tissue and was regulated by reducing acetyl-CoA production (20). The functional enrichment analysis showed that DEGs were enriched in some BPs such as chromosome segregation, chromosome region, and DNA replication.
We analyzed the prognostic role of mitophagy-related genes in CRC. The results indicated that high expression of ATG12 and MAP1LC3B is associated with poor prognosis of CRC, whereas high expression of TOMM22 and TOMM40 predicted a better prognosis. ATG12 expression was increased in radioresistant CRC specimens, indicating that it might serve as a potential therapeutic target in CRC treatment (38). The omega-3 fatty acid docosahexaenoic acid (DHA) is known for having anti-cancer properties. The basal level of autophagy and MAP1LC3B protein are possible indicators for DHA sensitivity in CRC cells (39). However, the importance of TOMM22 and TOMM40 in CRC remains unknown. Our findings may shed new light on the mitophagy that underpins CRC, as well as identify some critical genes.
A mtscore model was constructed based on 27 mitophagy regulators to estimate mitophagy accumulation, which was found to be a reliable prognostic indicator of CRC. The prognostic predictive ability of the mtscore was stable across subgroups stratified by gender, stage, and age. Nomograms based on independent factors were established to provide clinicians with more intuitive and easy-to-use prognostic models. The prognostic nomogram was developed as a feasible tool for predicting the probability of survival at 1-, 3-, and 5-years in patients with CRC.
Immune cell infiltrates in tumor immune microenvironment (TIME) are widely accepted to play an important role in cancer biology. Previous studies have shown that the degree of immune cell infiltration has a significant impact on CRC survival (40,41). Therefore, we investigated the association between mitophagy regulators and immune microenvironment by bioinformatics method. The results suggested that mitophagy regulators might interact with the TIME components and participate in various immune-related pathways, thus playing a crucial role in CRC progression.
There are several limitations in this study. Firstly, our study was mainly conducted by bioinformatics methods, lacking the validation of solid clinical specimens. Therefore, additional experiments are required to validate the results based on tumor samples and clinical data. Secondly, we explored the immune implications of mitophagy in CRC, however, the mechanism between mitophagy and the immune system is still not well understood. Thirdly, we just focused on the comparison of CRC tissues with matched normal tissue from the same patient; we will endeavor to include normal colorectal tissues from non-cancer patient samples to better reveal abnormalities of gene expression during tumorigenesis in further studies. In addition, a retrospective design was used for the study as opposed to a prospective one. Therefore, to validate the current conclusion, more investigations involving prospective clinical trials and mechanistic exploration are required.
Conclusions
We developed and validated mitophagy-related signatures that could not only predict the survival outcomes of CRC patients but also reflect the immune status of CRC. Additionally, we constructed and validated a mitophagy-associated risk score system, which might provide some useful insights to predict the prognosis of CRC patients and even assist their treatment in clinical practice.
Acknowledgments
Funding: This study was supported by grants from
Footnote
Reporting Checklist: The authors have completed the TRIPOD reporting checklist. Available at https://tcr.amegroups.com/article/view/10.21037/tcr-23-785/rc
Peer Review File: Available at https://tcr.amegroups.com/article/view/10.21037/tcr-23-785/prf
Conflicts of Interest: All authors have completed the ICMJE uniform disclosure form (available at https://tcr.amegroups.com/article/view/10.21037/tcr-23-785/coif). The authors have no conflicts of interest to declare.
Ethical Statement: The authors are accountable for all aspects of the work in ensuring that questions related to the accuracy or integrity of any part of the work are appropriately investigated and resolved. This study was conducted in accordance with the Declaration of Helsinki (as revised in 2013).
Open Access Statement: This is an Open Access article distributed in accordance with the Creative Commons Attribution-NonCommercial-NoDerivs 4.0 International License (CC BY-NC-ND 4.0), which permits the non-commercial replication and distribution of the article with the strict proviso that no changes or edits are made and the original work is properly cited (including links to both the formal publication through the relevant DOI and the license). See: https://creativecommons.org/licenses/by-nc-nd/4.0/.
References
- Siegel RL, Miller KD, Fuchs HE, et al. Cancer statistics, 2022. CA Cancer J Clin 2022;72:7-33. [Crossref] [PubMed]
- Sung H, Ferlay J, Siegel RL, et al. Global Cancer Statistics 2020: GLOBOCAN Estimates of Incidence and Mortality Worldwide for 36 Cancers in 185 Countries. CA Cancer J Clin 2021;71:209-49. [Crossref] [PubMed]
- Ladabaum U, Dominitz JA, Kahi C, et al. Strategies for Colorectal Cancer Screening. Gastroenterology 2020;158:418-32. [Crossref] [PubMed]
- Tauriello DV, Calon A, Lonardo E, et al. Determinants of metastatic competency in colorectal cancer. Mol Oncol 2017;11:97-119. [Crossref] [PubMed]
- Porporato PE, Filigheddu N, Pedro JMB, et al. Mitochondrial metabolism and cancer. Cell Res 2018;28:265-80. [Crossref] [PubMed]
- Ferro F, Servais S, Besson P, et al. Autophagy and mitophagy in cancer metabolic remodelling. Semin Cell Dev Biol 2020;98:129-38. [Crossref] [PubMed]
- Poole LP, Macleod KF. Mitophagy in tumorigenesis and metastasis. Cell Mol Life Sci 2021;78:3817-51. [Crossref] [PubMed]
- Bernardini JP, Lazarou M, Dewson G. Parkin and mitophagy in cancer. Oncogene 2017;36:1315-27. [Crossref] [PubMed]
- Zhu L, Wu W, Jiang S, et al. Pan-Cancer Analysis of the Mitophagy-Related Protein PINK1 as a Biomarker for the Immunological and Prognostic Role. Front Oncol 2020;10:569887. [Crossref] [PubMed]
- Kung-Chun Chiu D, Pui-Wah Tse A, Law CT, et al. Hypoxia regulates the mitochondrial activity of hepatocellular carcinoma cells through HIF/HEY1/PINK1 pathway. Cell Death Dis 2019;10:934. [Crossref] [PubMed]
- Xu Y, Lu J, Tang Y, et al. PINK1 deficiency in gastric cancer compromises mitophagy, promotes the Warburg effect, and facilitates M2 polarization of macrophages. Cancer Lett 2022;529:19-36. [Crossref] [PubMed]
- Lee YS, Jung YY, Park MH, et al. Deficiency of parkin suppresses melanoma tumor development and metastasis through inhibition of MFN2 ubiquitination. Cancer Lett 2018;433:156-64. [Crossref] [PubMed]
- Erkan M, Kleeff J, Esposito I, et al. Loss of BNIP3 expression is a late event in pancreatic cancer contributing to chemoresistance and worsened prognosis. Oncogene 2005;24:4421-32. [Crossref] [PubMed]
- Sowter HM, Ferguson M, Pym C, et al. Expression of the cell death genes BNip3 and NIX in ductal carcinoma in situ of the breast; correlation of BNip3 levels with necrosis and grade. J Pathol 2003;201:573-80. [Crossref] [PubMed]
- Hou H, Er P, Cheng J, et al. High expression of FUNDC1 predicts poor prognostic outcomes and is a promising target to improve chemoradiotherapy effects in patients with cervical cancer. Cancer Med 2017;6:1871-81. [Crossref] [PubMed]
- Guan Y, Wang Y, Li B, et al. Mitophagy in carcinogenesis, drug resistance and anticancer therapeutics. Cancer Cell Int 2021;21:350. [Crossref] [PubMed]
- Chi HC, Chen SL, Lin SL, et al. Thyroid hormone protects hepatocytes from HBx-induced carcinogenesis by enhancing mitochondrial turnover. Oncogene 2017;36:5274-84. [Crossref] [PubMed]
- Liu Y, Fu Y, Hu X, et al. Caveolin-1 knockdown increases the therapeutic sensitivity of lung cancer to cisplatin-induced apoptosis by repressing Parkin-related mitophagy and activating the ROCK1 pathway. J Cell Physiol 2020;235:1197-208. [Crossref] [PubMed]
- Boyle KA, Van Wickle J, Hill RB, et al. Mitochondria-targeted drugs stimulate mitophagy and abrogate colon cancer cell proliferation. J Biol Chem 2018;293:14891-904. [Crossref] [PubMed]
- Yin K, Lee J, Liu Z, et al. Mitophagy protein PINK1 suppresses colon tumor growth by metabolic reprogramming via p53 activation and reducing acetyl-CoA production. Cell Death Differ 2021;28:2421-35. [Crossref] [PubMed]
- Chen WT, Yang HB, Ke TW, et al. Serum DJ-1 Is a Biomarker of Colorectal Cancer and DJ-1 Activates Mitophagy to Promote Colorectal Cancer Progression. Cancers (Basel) 2021;13:4151. [Crossref] [PubMed]
- Mayakonda A, Lin DC, Assenov Y, et al. Maftools: efficient and comprehensive analysis of somatic variants in cancer. Genome Res 2018;28:1747-56. [Crossref] [PubMed]
- Smith JJ, Deane NG, Wu F, et al. Experimentally derived metastasis gene expression profile predicts recurrence and death in patients with colon cancer. Gastroenterology 2010;138:958-68. [Crossref] [PubMed]
- Marisa L, de Reyniès A, Duval A, et al. Gene expression classification of colon cancer into molecular subtypes: characterization, validation, and prognostic value. PLoS Med 2013;10:e1001453. [Crossref] [PubMed]
- Hänzelmann S, Castelo R, Guinney J. GSVA: gene set variation analysis for microarray and RNA-seq data. BMC Bioinformatics 2013;14:7. [Crossref] [PubMed]
- Zhang B, Wu Q, Li B, et al. m(6)A regulator-mediated methylation modification patterns and tumor microenvironment infiltration characterization in gastric cancer. Mol Cancer 2020;19:53. [Crossref] [PubMed]
- Bhattacharya S, Dunn P, Thomas CG, et al. ImmPort, toward repurposing of open access immunological assay data for translational and clinical research. Sci Data 2018;5:180015. [Crossref] [PubMed]
- Wilkerson MD, Hayes DN. ConsensusClusterPlus: a class discovery tool with confidence assessments and item tracking. Bioinformatics 2010;26:1572-3. [Crossref] [PubMed]
- Ritchie ME, Phipson B, Wu D, et al. limma powers differential expression analyses for RNA-sequencing and microarray studies. Nucleic Acids Res 2015;43:e47. [Crossref] [PubMed]
- Yu G, Wang LG, Han Y, et al. clusterProfiler: an R package for comparing biological themes among gene clusters. OMICS 2012;16:284-7. [Crossref] [PubMed]
- Sun M, Xie M, Zhang T, et al. m(6)A Methylation Modification Patterns and Tumor Microenvironment Infiltration Characterization in Pancreatic Cancer. Front Immunol 2021;12:739768. [Crossref] [PubMed]
- Li J, Ma X, Chakravarti D, et al. Genetic and biological hallmarks of colorectal cancer. Genes Dev 2021;35:787-820. [Crossref] [PubMed]
- Xu S, Liu D, Cui M, et al. Identification of Hub Genes for Early Diagnosis and Predicting Prognosis in Colon Adenocarcinoma. Biomed Res Int 2022;2022:1893351. [Crossref] [PubMed]
- Patil AR, Leung MY, Roy S. Identification of Hub Genes in Different Stages of Colorectal Cancer through an Integrated Bioinformatics Approach. Int J Environ Res Public Health 2021;18:5564. [Crossref] [PubMed]
- Onishi M, Yamano K, Sato M, et al. Molecular mechanisms and physiological functions of mitophagy. EMBO J 2021;40:e104705. [Crossref] [PubMed]
- Wang Y, Wang Z, Sun J, et al. Identification of HCC Subtypes With Different Prognosis and Metabolic Patterns Based on Mitophagy. Front Cell Dev Biol 2021;9:799507. [Crossref] [PubMed]
- Deng R, Zhang HL, Huang JH, et al. MAPK1/3 kinase-dependent ULK1 degradation attenuates mitophagy and promotes breast cancer bone metastasis. Autophagy 2021;17:3011-29. [Crossref] [PubMed]
- Hu JL, He GY, Lan XL, et al. Inhibition of ATG12-mediated autophagy by miR-214 enhances radiosensitivity in colorectal cancer. Oncogenesis 2018;7:16. [Crossref] [PubMed]
- Samdal H, Sandmoe MA, Olsen LC, et al. Basal level of autophagy and MAP1LC3B-II as potential biomarkers for DHA-induced cytotoxicity in colorectal cancer cells. FEBS J 2018;285:2446-67. [Crossref] [PubMed]
- Peng D, Wang L, Li H, et al. An immune infiltration signature to predict the overall survival of patients with colon cancer. IUBMB Life 2019;71:1760-70. [Crossref] [PubMed]
- Ge P, Wang W, Li L, et al. Profiles of immune cell infiltration and immune-related genes in the tumor microenvironment of colorectal cancer. Biomed Pharmacother 2019;118:109228. [Crossref] [PubMed]