Construction and validation of a joint diagnosis model based on random forest and artificial intelligence network for hepatitis B-related hepatocellular carcinoma
Highlight box
Key findings
• A diagnostic model of hepatitis B virus (HBV)-related hepatocellular carcinoma (HCC) was established, and the model showed better efficiency in distinguishing HBV-related HCC from other non-cancerous with HBV individuals.
What is known and what is new?
• In the previous studies, differentially expressed genes and the association pathways involved in HBV-induced HCCs were identified through integrated bioinformatics analysis using multiple datasets. Other types of diagnostic and predictive models for HBV-related HCC have also been established previously.
• Based on Gene Expression Omnibus (GEO) expression data, a diagnostic model of early HBV-related HCC was established.
What is the implication, and what should change now?
• The findings give a deeper and more comprehensive understanding of the occurrence and progression of HCC and its association with HBV and a valuable reference for the early screening and directions for improving the clinical efficacy of HBV-related HCC.
Introduction
Hepatocellular carcinoma (HCC) is the most prevalent primary liver cancer (90%) and the fourth leading cause of cancer-related death worldwide (1). By 2025, it is estimated to threat the health of more than 0.8 million people annually, with Chinese patients accounting for more than half of the global HCC burden (2). Variations in the incidence rate for HCC globally are attributed to diversity in risk factors. The prevalence of hepatitis B and C virus infections, especially the hepatitis B virus (HBV), is responsible for the highest incidence of HCC in East Asia and sub-Saharan Africa, with HBV-induced HCCs accounting for ~60% of cases in Asia and Africa (1,3). Chronic HBV infection leads to persistent liver damage and impaired regeneration, a well-known driving force of liver fibrogenesis and carcinogenesis (4). Therefore, there is an urgent need to identify reliable diagnostic markers to distinguish HBV-related HCC from other non-cancerous individuals with HBV.
Conventionally, early clinical diagnosis of HCC is depended on clinical symptoms, serum alpha-fetoprotein (AFP) and imaging findings in patients with chronic hepatitis or cirrhosis. Despite significant improvement in the prevention, monitoring, early screening, diagnosis and therapy of HCC over the past decade, the prognosis for the vast majority of HCC patients is typically poor. In addition, most patients lack obvious clinical symptoms in the early stage of HCC, and tumor that located deep within the abdominal cavity makes early diagnosis even more challenging. This highlights the importance of cancer research in identifying effective biomarkers, which are an attractive alternative for surveillance and early diagnosis of HCC because of its objectivity and reproducibility. Previous studies have identified a few potential diagnostic markers for HBV-related HCC, including AFP, Des-Gamma Carboxy Prothrombin (DCP), Golgi Protein Complex 73 (GPC 73), Osteopontin (OPN), cell-free/circulating tumor DNA, tumor-associated microRNAs and extracellular vesicles (5-11).
Machine learning (ML), unlike traditional statistical methods, is not rule-based programming but rather learning from examples. ML is an emerging discipline based on the intersection of statistics and mathematical sciences. It builds a statistical model from learning from large massive datasets data to achieve accurate prediction and to guide future research efforts (12,13). The random forest (RF), an innovative and highly effective supervised ML algorithm, uses several different prediction features in the training samples to effectively classify unknown samples by constructing a series of decision trees (13). RF classifier is an integrated approach consisting of multiple decision trees that are independent of each other. Each decision tree processes samples and predicts output labels, and the final output of the model is determined by the class that receives the most votes from the individual trees (14). As RFs overcomes the common problem of over-fitting through the use of bootstrap aggregation, it appears to be more accurate in prediction than other algorithms (15). Another supervised ML algorithm is artificial neural network (ANN), which is based on the functioning of biological neural networks. A neural network is composed of a large number of nodes (or neurons) connected to each other. The connection between each two nodes represents a weighted value for the signal passing through the connection, which is called the weight (16). Usually, these neurons are grouped in layers and process data in each layer, which are then passed forward to the next layers. Finally, the last layer responsible for making decisions and outputting results. The ANN is used to build a model of the complex relationship between input and output data and thus revealing the patterns (17). Compared to conventional programming, neural networks are available to deal with problems that algorithms could not solve, or the available solutions are too complex (17). ANN models are widely used in disease diagnosis, classification, prediction, and survival analysis because of their ability to handle linear and nonlinear relationship of data (18). It is well acknowledged that carcinogenesis and progression of HCC are closely related to mutation of genes, overexpression of various oncogenes and inactivation of tumor suppressor genes (19). With the rapid development in sequencing technology, huge volumes of gene expression profiling data related to cancer are generated for the identification of novel differential genes and diagnostic and prognostic biomarkers. In the previous studies, differentially expressed genes (DEGs) and the association pathways involved in HBV-induced HCCs were identified through integrated bioinformatics analysis using multiple datasets.
In this study, three datasets were merged. The RF algorithm was then used to identify the key genes expressed in HBV-related HCC, and ANNs constructed a genetic diagnostic model of HBV-related HCC. Finally, immune cell infiltration between HBV-related HCC samples and non-cancerous samples with HBV was evaluated. We present this article in accordance with the TRIPOD reporting checklist (available at https://tcr.amegroups.com/article/view/10.21037/tcr-23-1197/rc).
Methods
Figure 1 shows the research framework of this study. The study was conducted in accordance with the Declaration of Helsinki (as revised in 2013).
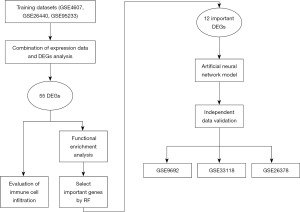
Gene expression data
Gene expression profiles of Gene Expression Omnibus (GEO) Series (GSE)19665 (https://www.ncbi.nlm.nih.gov/geo/query/acc.cgi?acc=GSE19665) (20), GSE55092 (https://www.ncbi.nlm.nih.gov/geo/query/acc.cgi?acc=GSE55092) (21), GSE121248 (https://www.ncbi.nlm.nih.gov/geo/query/acc.cgi?acc=GSE121248) (22), GSE17548 (https://www.ncbi.nlm.nih.gov/geo/query/acc.cgi?acc=GSE17548) (23), GSE104310 (https://www.ncbi.nlm.nih.gov/geo/query/acc.cgi?acc=GSE104310), GSE44074 (https://www.ncbi.nlm.nih.gov/geo/query/acc.cgi?acc=GSE44074) (24), and GSE136247 (https://www.ncbi.nlm.nih.gov/geo/query/acc.cgi?acc=GSE136247) (25) were obtained from the GEO database of the National Centre for Biotechnology Information (https://www.ncbi.nlm.nih.gov/geo/) (raw data are available at https://cdn.amegroups.cn/static/public/tcr-23-1197-1.xlsx, Table 1). The seven datasets were divided into two groups: the training set, which including GSE19665, GSE55092, and GSE121248, and the remaining datasets were classified into the test set to verify the performance of the model. As previously described, the process of converting gene probe IDs to gene symbols was done using A Perl language command. The normalisation between arrays function was used to normalise the gene expression data, and the gene expression data were averaged when multiple probes correspond to a gene. Subsequently, the expression data of the three datasets in training sets were merged and used for the following analysis, the batch effect from the different datasets was removed, and the common genes were finally obtained. The gene expression data with a larger value were subjected to log2 transformation in the limma R package.
Table 1
Dataset ID | Sample | Platform | Non-cancerous samples with HBV | HBV-related HCC | Classification | Country | Reference |
---|---|---|---|---|---|---|---|
GSE19665 | HCC (HBV) | GPL570 | 5 | 5 | Training sets | Japan | Deng et al. 2010, (20) |
GSE55092 | HCC (HBV) | GPL570 | 91 | 49 | Training sets | USA | Melis et al. 2014, (21) |
GSE121248 | HCC (HBV) | GPL570 | 37 | 70 | Training sets | Singapore | Wang et al. 2007, (22) |
GSE17548 | HCC (HBV) | GPL570 | 11 | 10 | Test sets | Turkey | Yildiz et al. 2013, (23) |
GSE104310 | HCC (HBV) | GPL16791 | 7 | 9 | Test sets | China | Yun et al. 2021, not published |
GSE44074 | HCC (HBV) | GPL13536 | 36 | 34 | Test sets | Japan | Ueda et al. 2013, (24) |
GSE136247 | HCC (HBV) | GPL17586 | 19 | 26 | Test sets | France | Cerapio et al. 2021, (25) |
GEO, Gene Expression Omnibus; HBV, hepatitis B virus; HCC, hepatocellular carcinoma.
Identification of DEGs and enrichment analyses
The limma R package v.3.5.2 in R software was used to identify DEGs. The DEGs were selected based on the cut-off criterion that adjusted P value <0.05 and |log2FC| >2. Metascape (http://metascape.org/gp/#/main/step1), a common integrated portal, contains functional enrichment, interactome analysis, gene annotation and membership search to provide a comprehensive gene list annotation and analysis resource for users to grasp biological characteristics (26). In present study, Gene Ontology (GO) and Kyoto Encyclopedia of Genes and Genomes (KEGG) pathway enrichment analyses were executed using Metascape (https://metascape.org/gp/index.html#/main/step1) online tool. P<0.05 was considered statistically significant.
RF screening for important genes
The RF software package (v.4.1.3) was used to filter out important variables and create an RF model that contributed most to the prediction of HBV-related HCC. First, the average model miscalculation rate of all genes based on out-of-band data was calculated. The best variable number for the binary tree at the node was set to 6, and 2000 was chosen as the best number of trees contained in the RF (27). Based on the point with the smallest error, the best RF model was then built, and the candidate genes for HBV-related HCC diagnosis were determined using the mean decrease Gini. Finally, for the subsequent model construction, the genes with a significance score greater than 4 were chosen as disease-specific genes.
Subsequently, scores were assigned to the expression data of the selected DEGs using the following rules: If an upregulated gene’s log FC value for a sample was greater than the gene’s median expression value across all samples, its score was automatically assigned as 1; otherwise, it was set to 0. If the log FC of the downregulated gene was greater than the mean expression value, its score was automatically assigned as 0; otherwise, it was set to 1. The heatmap of the selected DEGs was drawn to show their expression in the merged dataset.
Neural network to build the disease classification model
The R software package neural net (v.1.44.2) were available to develop an ANN model of the important variables. The weight of each gene was obtained and five hidden layers were set as the model parameters to build a classification model of HBV-related HCC through the obtained gene score. The model accuracy results were obtained for HBV-related HCC samples and non-cancerous samples with HBV in the training set, and the receiver operating characteristic (ROC) software package was used to calculate the areas under the ROC curves (AUCs) classification performance verification results.
Validation of the predictive model
Four independent datasets (GSE17548, GSE104310, GSE44074, GSE136247) were used to verify the accuracy of the ANN model for classifying samples (HBV-related HCC or non-cancerous samples with HBV), and the ROC curves for each dataset were drawn using the pROC software package separately. At the same time, the optimal threshold in the ROC curve and the sensitivity and specificity in classifying cancer and normal samples under this threshold were calculated.
Evaluation of immune cell infiltration
The normalised gene expression data from the merged dataset was available to evaluate the abundance of immune infiltrates in all samples through the CIBERSORT algorithm. The percentages of 22 infiltrating immune cell types were calculated and output with the cutoff criterion that P value <0.05, and their correlations were displayed in a correlation heatmap drawn by the “corrplot” package (28). The ratios of infiltrating immune cells in non-cancerous liver tissues from HBV patients and HBV-related HCC tissues were visualised by a histogram, and the difference was shown by violin diagrams.
Statistical analysis
The limma R package v.3.5.2 in R software was used to identify DEGs. The DEGs were selected based on the cut-off criterion that adjusted P value <0.05 and |log2FC| >2. The performance of ANN model was evaluated using ROCs, and the AUC, sensitivity, and specificity were determined. The correlation between 22 infiltrating immune cell types was assessed by calculating the Pearson correlation coefficient.
Results
Identification of DEGs in HCC
The samples in all datasets were strictly screened, and the samples without chronic HBV infection were excluded. GSE19665, GSE55092, and GSE121248 gene expression data were merged as a training dataset for subsequent analysis. A total of 133 non-cancerous liver tissues with HBV and 124 HBV-related HCC tissues were included in present analysis. As shown in the volcano graph (Figure 2A), 116 genes were identified as DEGs according to the cut-off criterion that adjusted P value <0.05 and |log2FC| >2 (Table S1, Figure S1). Figure 2B shows a heatmap of the top 10 up- and downregulated genes.
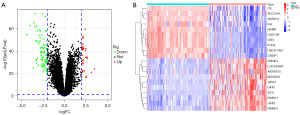
Functional enrichment analysis of DEGs in the training dataset
To further investigate the biological functions of the 116 DEGs, GO analysis and KEGG pathway enrichment analysis were performed using online database Metascape. As previously described (29), the GO analysis consisted of three functional groups, namely, the biological process (BP) group, the cellular component (CC) group and the molecular function (MF) group. The results of GO analysis exhibited that the DEGs were particularly enriched in the BP (Figure 3A, Table S2), including monocarboxylic acid metabolic process, response to bacterium, response to peptide, regulation of growth, and cellular response to xenobiotic stimulus. For the CC (Figure 3B), the DEGs were mainly enriched in collagen-containing extracellular matrix, spindle, external side of plasma membrane, blood microparticle, and basolateral plasma membrane. In the MF (Figure 3C), the DEGs were principally enriched in oxidoreductase activity, protein homodimerization activity, carbohydrate binding, amide binding, and lipid transporter activity.

The results of KEGG pathway enrichment analysis revealed that the DEGs were particularly enriched in bile secretion, cytokine-cytokine receptor interaction, caffeine metabolism, tryptophan metabolism, and steroid hormone biosynthesis (Figure 3D, Table S3).
RF screening for DEGs
All 116 DEGs were included in the RF classifier. Figure 4A shows the relationship between the model error and the number of decision trees. The final model showed a stable error when the number of decision trees was 2000. Therefore, the RF model was built with 2000 trees as the parameter of the final model. Genes with an importance score are shown in Figure 4B; genes with an importance score greater than 4 were selected as the candidate genes for subsequent analysis. Finally, nine genes were selected, DNA topoisomerase II alpha (TOP2A), C-type lectin domain family 1 member B (CLEC1B), BUB1 mitotic checkpoint serine/threonine kinase B (BUB1B), ficolin 2 (FCN2), C-X-C motif chemokine ligand 14 (CXCL14) and cyclase associated actin cytoskeleton regulatory protein 2 (CAP2) being the most important, followed by ficolin 3 (FCN3), kynurenine 3-monooxygenase (KMO) and cadherin related family member 2 (CDHR2). As shown in Figure 4C, CAP2, TOP2A, BUB1B were upregulated in HBV-related HCC samples, while KMO, CDHR2, CXCL14, FCN2, CLEC1B were upregulated in non-cancerous liver tissue with HBV.
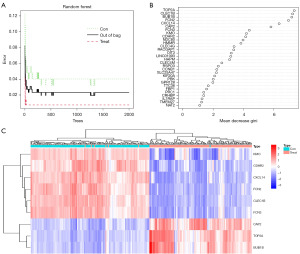
Construction of the ANN model
Expression data for these nine genes in each sample were assigned a score of 1 or 0. Based on these nine important variables, an ANN model was constructed and used to distinguish HBV-related HCC tissues and non-cancerous liver tissue with HBV in 257 samples of the merge datasets (Figure 5A). As a result, the model could correctly predict 132 cases in the HBV-related HCC group with 99.2% (132/133) accuracy and 120 cases in the non-cancerous with HBV group with 96.8% (120/124) accuracy. The AUC of the model in the training dataset were close to 1 (average AUC >0.99), showing the highly stable of the model in diagnosing HBV-related HCC (Figure 5B).
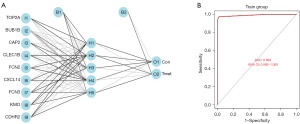
Validation of the ANN model
Four independent datasets (GSE17548, GSE104310, GSE44074, GSE136247) were used to verify the performance of the ANN model to classify samples (HBV-related HCC tissues or non-cancerous liver tissues with HBV). As a result, the model could correctly predict 23 cases in the HBV-related HCC group with 88.5% (23/26) accuracy and 19 cases in the non-cancerous with HBV group with 100% (19/19) accuracy in GSE136247, 9 cases in the HBV-related HCC group with 90% (9/10) accuracy and 9 cases in the non-cancerous with HBV group with 81.8% (9/11) accuracy in GSE17548, 23 cases in the HBV-related HCC group with 88.5% (23/26) accuracy and 17 cases in the non-cancerous with HBV group with 89.5% (17/19) accuracy in GSE104310, and 26 cases in the HBV-related HCC group with 76.5% (26/34) accuracy and 26 cases in the non-cancerous with HBV group with 72.2% (26/36) accuracy in GSE44074. The AUCs of the model in the test dataset were 1 [95% confidence interval (CI): 1–1], 0.927 (95% CI: 0.791–1), 0.921 (95% CI: 0.738–1) and 0.833 (95% CI: 0.725–0.918), respectively (Figure 6).
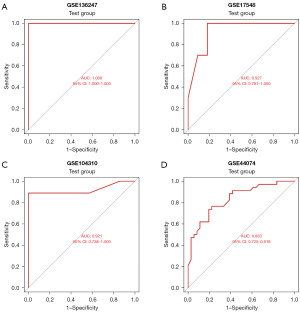
Immune cell infiltration results
A total of 133 cases of non-cancerous liver tissues from HBV patients and 124 cases of HBV-related HCC tissues were selected for the immune cell infiltration analysis. Based on the cut-off criterion that P<0.05, 38 cases of HBV-related HCC tissues and 31 cases of non-cancerous liver tissues from HBV patients were selected for CIBERSORT analysis. First, the percentages of 22 kinds of immune cells in each sample were visualised in a histogram (Figure 7A). The correlations of 22 kinds of infiltrating immune cells between HBV-related HCC tissues and non-cancerous liver tissue with HBV were analysed (Figure 7B). For example, T follicular helper cells were positively correlated with T cells CD8+ and macrophages M1. Natural killer (NK) cells resting were positively associated with neutrophils and T cells CD4 naïve. The Wilcoxon test was used to detect significantly different immune cell infiltrates between HBV-related HCC tissues and non-cancerous liver tissue with HBV. The results that presented 12 types (B cells naive, B cells memory, plasma cells, T cells CD8, T cells CD4 memory resting, Tregs, T cells gamma delta, NK cells resting, NK cells activated, Macrophages M0, Dendritic cells activated, Mast cells activated) of immune cells with P<0.05 are shown in a violin diagram in Figure 7C.
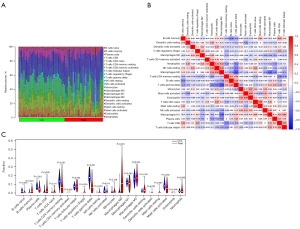
Discussion
This study aimed to establish an effective diagnostic model for HBV-related HCC based on gene expression data from GEO. The three datasets in the training group were from different countries, using the same sequencing platform, which minimised the effect of confounding factors to some extent, 116 DEGs were identified in the merged dataset formed from three HBV-related HCC datasets. Nine important candidate DEGs were acquired through the RF classifier, and a neural network model was created. Four independent datasets were used to verify the classification (HBV-related liver cancer or non-cancerous liver tissues with HBV) efficiency of the model, and the AUC (1, 0.927, 0.921, 0.833) efficiency showed excellent. Four independent datasets from different countries and regions were used to assess the performance of this diagnostic model, increasing the stability, usefulness and credibility of this model. The immune cell infiltration result shows that the percentages of 12 types of immune cells were significantly different between HBV-related HCC tissues and non-cancerous liver tissue with HBV.
RF and ANN are different types of algorithms. RF is an ensemble decision tree approach in which each decision tree processes a sample and predicts an output label. Decision trees in an ensemble are independent. ANN is composed of many layers of nodes that carry the signal and process it to make the final decision (30). An ANN model for the diagnosis and screening of HBV-related HCC was constructed based on nine important genes from RFs. Of these nine genes, TOP2A and BUB1B have been extensively studied in HCC (31-35). KMO (36,37), CDHR2 (38), CLEC1B (39), CXCL14 (40) and FCN2 (41) were significantly decreased in HCC tissues (or) and cell lines, overexpression of these genes exhibited tumor-inhibitory effects towards HCC (36,37), including inhibiting tumor formation and the growth of subcutaneous tumors, suppresseing proliferation, migration and invasion of HCC cells, epithelial-mesenchymal transition (EMT) and induced apoptosis. FCN3 expression was significantly lower in HCC tissues than in normal tissues (42). However, more in vitro and in vivo experiments are needed to further confirm its effect on HCC. KMO (37), CXCL14 (43), CAP2 (44) and FCN3 (45) were prognostic markers in HCC, and the combination of PD-L1high and CLEC1Blow expression has been shown to predict worse outcomes (46).
CAP2 was a valuable molecular marker in the histological diagnosis of early HCC (47), and its overexpression might be related to multistage hepatocarcinogenesis (48). In addition, CAP2 transcriptional levels were significantly suppressed in silibinin-treated HCC cells. Silibinin could be a potential therapeutic agent against HCC, particularly for HBV-related HCCs (49). These findings indicate that CAP2 may play a critical role in the carcinogenesis or progression of HBV-related HCC. CXCL14 was markedly suppressed in HBV-related HCC tissues, and its polymorphisms were associated with advanced-stage chronic HBV infection (50). FCN2 is active in hepatitis B infection (51), and ficolin-2 serum levels and FCN2 haplotypes contribute to the outcome of HBV infection in a Vietnamese cohort (51). FCN2 was implied, which was implied to play a crucial role in innate immunity against HBV infection.
Other types of diagnostic and predictive models for HBV-related HCC have also been established previously. ATP binding cassette subfamily B member 6 (ABCB6), importin 7 (IPO7), translocase of inner mitochondrial membrane 9 (TIMM9), frizzled class receptor 7 (FZD7), and acetyl-CoA acetyltransferase 1 (ACAT1), the five HBV-related genes were identified for constructing a prognostic model, which were capable of accurately differentiating HBV patients from non-HBV patients with HCC (52). Integrated analysis of the microbiome and host transcriptome revealed that six important microbial markers associated with the tumor immune microenvironment or bile acid metabolism showed good classification performance for discriminating 5-year survival and 2-year disease-free survival (53). LncRNA was also a potential diagnostic biomarker for HBV-related HCC, and AL356056.2, AL445524.1, TRIM52-AS1, AC093642.1, EHMT2-AS1, AC003991.1, AC008040.1, LINC00844 and LINC01018 were screened out by ML (54). Based on the data from the hospital authority data collaboration lab, 124,006 patients with chronic viral hepatitis (CVH) with complete data were included to build the models, and HCC ridge score (HCC-RS) from the ridge regression ML model accurately predicted HCC in patients with CVH (55). In addition, another study identified noninvasive biomarkers by applying a urinary proteomic strategy (56).
Infiltrating immune cells, a component of the tumor microenvironment, are involved in many processes, including tumor growth, invasion and metastasis. Accumulating evidence has shown that HCC tumors harbour a significant level of immune cell infiltration, and the status of immune cell infiltration and its characteristics are usually associated with different prognostic outcomes (57,58). The ratio of group 2 innate lymphoid cells (ILC2s) to ILC1s increased from non-tumor to tumor tissue in the majority of the HCC patients, and the high ILC2/ILC1 ratio were correlated to better patient survival rates (59). In this study, the density of B cells memory, T cells CD8, Tregs, NK cells resting, macrophages M0, dendritic cells activated in tumor tissues significantly increased compared with non-cancerous liver tissues with HBV. In contrast, the density of B cells naïve, plasma cells, T cells CD4 memory resting, T cells gamma delta, NK cells activated, mast cells activated in HBV-related HCC tissues significantly decreased. T cells, B cells, NK cells, macrophages and mast cells have been previously reported to be present in immune cell infiltrates of HCC and play essential roles in the development, prognosis and immunotherapy treatment of HCC. High densities of naïve B cells and plasma cells were associated with superior survival (60). The antitumor or tumor-promoting effects of tumor-infiltrating lymphocytes depend on the proportion of the lymphocyte subsets constituent in the tumor microenvironment, and T lymphocytes are the primary tumor-infiltrating lymphocytes (TILs) cells in HCC (61). The mechanism of mast cell activation in HCC is unclear, but its activation facilitates immune escape and resultant tumor growth (58). More importantly, HBV-specific CD8+ T cells, HBV-non-specific CD8+, CD4+ T, B and NK/NKT cells are all involved in the development of HBV-related HCC (62).
This study has some limitations. First, HCC exhibits high heterogeneity, which contains etiologic, geographic and molecular heterogeneity. Molecular heterogeneity can be further classified into interpatient, intertumor and intratumor heterogeneity (63). The HBV-related HCC diagnosis model using an ANN was solely based on gene expression data. Therefore, it is difficult to use a single model to accurately diagnose HCC at an early stage, although the model performed satisfactorily on the training and validation datasets. Second, the number of samples used for the construction and validation of this model was relatively small. Third, subsequent confirmatory experiments and clinical practice are needed to further monitor the accuracy and stability of the diagnostic model.
Conclusions
In conclusion, a combination of three datasets’ expression data was used to select important variables through RF. An ANN model was formulated for the early diagnosis and screening of HBV-related HCC. Finally, the ratio of infiltrating immune cells in non-cancerous liver tissues from HBV patients and HBV-related HCC tissues was assessed. The findings give a deeper and more comprehensive understanding of the occurrence and progression of HCC and its association with HBV and a valuable reference for the early screening and directions for improving the clinical efficacy of HBV-related HCC.
Acknowledgments
Funding: This work was supported by
Footnote
Reporting Checklist: The authors have completed the TRIPOD reporting checklist. Available at https://tcr.amegroups.com/article/view/10.21037/tcr-23-1197/rc
Peer Review File: Available at https://tcr.amegroups.com/article/view/10.21037/tcr-23-1197/prf
Conflicts of Interest: All authors have completed the ICMJE uniform disclosure form (available at https://tcr.amegroups.com/article/view/10.21037/tcr-23-1197/coif). The authors have no conflicts of interest to declare.
Ethical Statement: The authors are accountable for all aspects of the work in ensuring that questions related to the accuracy or integrity of any part of the work are appropriately investigated and resolved. The study was conducted in accordance with the Declaration of Helsinki (as revised in 2013).
Open Access Statement: This is an Open Access article distributed in accordance with the Creative Commons Attribution-NonCommercial-NoDerivs 4.0 International License (CC BY-NC-ND 4.0), which permits the non-commercial replication and distribution of the article with the strict proviso that no changes or edits are made and the original work is properly cited (including links to both the formal publication through the relevant DOI and the license). See: https://creativecommons.org/licenses/by-nc-nd/4.0/.
References
- Hepatocellular carcinoma. Nat Rev Dis Primers 2021;7:7. [Crossref] [PubMed]
- Bray F, Ferlay J, Soerjomataram I, et al. Global cancer statistics 2018: GLOBOCAN estimates of incidence and mortality worldwide for 36 cancers in 185 countries. CA Cancer J Clin 2018;68:394-424. [Crossref] [PubMed]
- El-Serag HB. Epidemiology of viral hepatitis and hepatocellular carcinoma. Gastroenterology 2012;142:1264-1273.e1. [Crossref] [PubMed]
- Li J, Cheng L, Jia H, et al. IFN-γ facilitates liver fibrogenesis by CD161+CD4+ T cells through a regenerative IL-23/IL-17 axis in chronic hepatitis B virus infection. Clin Transl Immunology 2021;10:e1353. [Crossref] [PubMed]
- Pandyarajan V, Govalan R, Yang JD. Risk Factors and Biomarkers for Chronic Hepatitis B Associated Hepatocellular Carcinoma. Int J Mol Sci 2021;22:479. [Crossref] [PubMed]
- European Association for the Study of the Liver. Electronic address: easloffice@easloffice; . EASL Clinical Practice Guidelines on haemochromatosis. J Hepatol 2022;77:479-502. [Crossref]
- Bertino G, Neri S, Bruno CM, et al. Diagnostic and prognostic value of alpha-fetoprotein, des-γ-carboxy prothrombin and squamous cell carcinoma antigen immunoglobulin M complexes in hepatocellular carcinoma. Minerva Med 2011;102:363-71. [PubMed]
- Dai M, Chen X, Liu X, et al. Diagnostic Value of the Combination of Golgi Protein 73 and Alpha-Fetoprotein in Hepatocellular Carcinoma: A Meta-Analysis. PLoS One 2015;10:e0140067. [Crossref] [PubMed]
- Shang S, Plymoth A, Ge S, et al. Identification of osteopontin as a novel marker for early hepatocellular carcinoma. Hepatology 2012;55:483-90. [Crossref] [PubMed]
- Ahn JC, Teng PC, Chen PJ, et al. Detection of Circulating Tumor Cells and Their Implications as a Biomarker for Diagnosis, Prognostication, and Therapeutic Monitoring in Hepatocellular Carcinoma. Hepatology 2021;73:422-36. [Crossref] [PubMed]
- Beudeker BJB, Boonstra A. Circulating biomarkers for early detection of hepatocellular carcinoma. Therap Adv Gastroenterol 2020;13:1756284820931734. [Crossref] [PubMed]
- Greener JG, Kandathil SM, Moffat L, et al. A guide to machine learning for biologists. Nat Rev Mol Cell Biol 2022;23:40-55. [Crossref] [PubMed]
- Van Calster B, Wynants L. Machine Learning in Medicine. N Engl J Med 2019;380:2588. [Crossref] [PubMed]
- Inturi AR, Manikandan VM, Kumar MN, et al. Synergistic Integration of Skeletal Kinematic Features for Vision-Based Fall Detection. Sensors (Basel) 2023;23:6283. [Crossref] [PubMed]
- Zhao N, Charland K, Carabali M, et al. Machine learning and dengue forecasting: Comparing random forests and artificial neural networks for predicting dengue burden at national and sub-national scales in Colombia. PLoS Negl Trop Dis 2020;14:e0008056. [Crossref] [PubMed]
- Sampa MB, Hossain MN, Hoque MR, et al. Blood Uric Acid Prediction With Machine Learning: Model Development and Performance Comparison. JMIR Med Inform 2020;8:e18331. [Crossref] [PubMed]
- Azimi P, Mohammadi HR, Benzel EC, et al. Artificial neural networks in neurosurgery. J Neurol Neurosurg Psychiatry 2015;86:251-6. [Crossref] [PubMed]
- Renganathan V. Overview of artificial neural network models in the biomedical domain. Bratisl Lek Listy 2019;120:536-40. [Crossref] [PubMed]
- Nakonieczna S, Grabarska A, Kukula-Koch W. The Potential Anticancer Activity of Phytoconstituents against Gastric Cancer-A Review on In Vitro, In Vivo, and Clinical Studies. Int J Mol Sci 2020;21:8307. [Crossref] [PubMed]
- Deng YB, Nagae G, Midorikawa Y, et al. Identification of genes preferentially methylated in hepatitis C virus-related hepatocellular carcinoma. Cancer Sci 2010;101:1501-10. [Crossref] [PubMed]
- Melis M, Diaz G, Kleiner DE, et al. Viral expression and molecular profiling in liver tissue versus microdissected hepatocytes in hepatitis B virus-associated hepatocellular carcinoma. J Transl Med 2014;12:230. [Crossref] [PubMed]
- Wang SM, Ooi LL, Hui KM. Identification and validation of a novel gene signature associated with the recurrence of human hepatocellular carcinoma. Clin Cancer Res 2007;13:6275-83. [Crossref] [PubMed]
- Yildiz G, Arslan-Ergul A, Bagislar S, et al. Genome-wide transcriptional reorganization associated with senescence-to-immortality switch during human hepatocellular carcinogenesis. PLoS One 2013;8:e64016. [Crossref] [PubMed]
- Ueda T, Honda M, Horimoto K, et al. Gene expression profiling of hepatitis B- and hepatitis C-related hepatocellular carcinoma using graphical Gaussian modeling. Genomics 2013;101:238-48. [Crossref] [PubMed]
- Cerapio JP, Marchio A, Cano L, et al. Global DNA hypermethylation pattern and unique gene expression signature in liver cancer from patients with Indigenous American ancestry. Oncotarget 2021;12:475-92. [Crossref] [PubMed]
- Zhou Y, Zhou B, Pache L, et al. Metascape provides a biologist-oriented resource for the analysis of systems-level datasets. Nat Commun 2019;10:1523. [Crossref] [PubMed]
- Tian Y, Yang J, Lan M, et al. Construction and analysis of a joint diagnosis model of random forest and artificial neural network for heart failure. Aging (Albany NY) 2020;12:26221-35. [Crossref] [PubMed]
- Friendly M. Corrgrams: Exploratory Displays for Correlation Matrices. The American Statistician 2002;56:316-24. [Crossref]
- Xie S, Jiang X, Zhang J, et al. Identification of significant gene and pathways involved in HBV-related hepatocellular carcinoma by bioinformatics analysis. PeerJ 2019;7:e7408. [Crossref] [PubMed]
- Dey P. Artificial neural network in diagnostic cytology. Cytojournal 2022;19:27. [Crossref] [PubMed]
- Sha M, Cao J, Zong ZP, et al. Identification of genes predicting unfavorable prognosis in hepatitis B virus-associated hepatocellular carcinoma. Ann Transl Med 2021;9:975. [Crossref] [PubMed]
- Liao X, Yu T, Yang C, et al. Comprehensive investigation of key biomarkers and pathways in hepatitis B virus-related hepatocellular carcinoma. J Cancer 2019;10:5689-704. [Crossref] [PubMed]
- Chen X, Liao L, Li Y, et al. Screening and Functional Prediction of Key Candidate Genes in Hepatitis B Virus-Associated Hepatocellular Carcinoma. Biomed Res Int 2020;2020:7653506. [Crossref] [PubMed]
- Qiang R, Zhao Z, Tang L, et al. Identification of 5 Hub Genes Related to the Early Diagnosis, Tumour Stage, and Poor Outcomes of Hepatitis B Virus-Related Hepatocellular Carcinoma by Bioinformatics Analysis. Comput Math Methods Med 2021;2021:9991255. [Crossref] [PubMed]
- Yu M, Xu W, Jie Y, et al. Identification and validation of three core genes in p53 signaling pathway in hepatitis B virus-related hepatocellular carcinoma. World J Surg Oncol 2021;19:66. [Crossref] [PubMed]
- Shi Z, Gan G, Gao X, et al. Kynurenine catabolic enzyme KMO regulates HCC growth. Clin Transl Med 2022;12:e697. [Crossref] [PubMed]
- Jin H, Zhang Y, You H, et al. Prognostic significance of kynurenine 3-monooxygenase and effects on proliferation, migration, and invasion of human hepatocellular carcinoma. Sci Rep 2015;5:10466. [Crossref] [PubMed]
- Xia Z, Huang M, Zhu Q, et al. Cadherin Related Family Member 2 Acts As A Tumor Suppressor By Inactivating AKT In Human Hepatocellular Carcinoma. J Cancer 2019;10:864-73. [Crossref] [PubMed]
- Zhang G, Su L, Lv X, et al. A novel tumor doubling time-related immune gene signature for prognosis prediction in hepatocellular carcinoma. Cancer Cell Int 2021;21:522. [Crossref] [PubMed]
- Wang W, Huang P, Zhang L, et al. Antitumor efficacy of C-X-C motif chemokine ligand 14 in hepatocellular carcinoma in vitro and in vivo. Cancer Sci 2013;104:1523-31. [Crossref] [PubMed]
- Yang G, Liang Y, Zheng T, et al. FCN2 inhibits epithelial-mesenchymal transition-induced metastasis of hepatocellular carcinoma via TGF-β/Smad signaling. Cancer Lett 2016;378:80-6. [Crossref] [PubMed]
- Wang S, Song Z, Tan B, et al. Identification and Validation of Hub Genes Associated With Hepatocellular Carcinoma Via Integrated Bioinformatics Analysis. Front Oncol 2021;11:614531. [Crossref] [PubMed]
- Lin T, Zhang E, Mai PP, et al. CXCL2/10/12/14 are prognostic biomarkers and correlated with immune infiltration in hepatocellular carcinoma. Biosci Rep 2021;41:BSR20204312. [Crossref] [PubMed]
- Fu J, Li M, Wu DC, et al. Increased Expression of CAP2 Indicates Poor Prognosis in Hepatocellular Carcinoma. Transl Oncol 2015;8:400-6. [Crossref] [PubMed]
- Lai X, Wu YK, Hong GQ, et al. A Novel Gene Signature Based on CDC20 and FCN3 for Prediction of Prognosis and Immune Features in Patients with Hepatocellular Carcinoma. J Immunol Res 2022;2022:9117205. [Crossref] [PubMed]
- Hu K, Wang ZM, Li JN, et al. CLEC1B Expression and PD-L1 Expression Predict Clinical Outcome in Hepatocellular Carcinoma with Tumor Hemorrhage. Transl Oncol 2018;11:552-8. [Crossref] [PubMed]
- Sakamoto M, Mori T, Masugi Y, et al. Candidate molecular markers for histological diagnosis of early hepatocellular carcinoma. Intervirology 2008;51:42-5. [Crossref] [PubMed]
- Shibata R, Mori T, Du W, et al. Overexpression of cyclase-associated protein 2 in multistage hepatocarcinogenesis. Clin Cancer Res 2006;12:5363-8. [Crossref] [PubMed]
- Ghasemi R, Ghaffari SH, Momeny M, et al. Multitargeting and antimetastatic potentials of silibinin in human HepG-2 and PLC/PRF/5 hepatoma cells. Nutr Cancer 2013;65:590-9. [Crossref] [PubMed]
- Lin Y, Chen BM, Yu XL, et al. Suppressed Expression of CXCL14 in Hepatocellular Carcinoma Tissues and Its Reduction in the Advanced Stage of Chronic HBV Infection. Cancer Manag Res 2019;11:10435-43. [Crossref] [PubMed]
- Hoang TV, Toan NL. Ficolin-2 levels and FCN2 haplotypes influence hepatitis B infection outcome in Vietnamese patients. PLoS One 2011;6:e28113. [Crossref] [PubMed]
- Ma K, Wu H, Ji L. Construction of HBV gene-related prognostic and diagnostic models for hepatocellular carcinoma. Front Genet 2023;13:1065644. [Crossref] [PubMed]
- Huang H, Ren Z, Gao X, et al. Integrated analysis of microbiome and host transcriptome reveals correlations between gut microbiota and clinical outcomes in HBV-related hepatocellular carcinoma. Genome Med 2020;12:102. [Crossref] [PubMed]
- Nong S, Chen X, Wang Z, et al. Potential lncRNA Biomarkers for HBV-Related Hepatocellular Carcinoma Diagnosis Revealed by Analysis on Coexpression Network. Biomed Res Int 2021;2021:9972011. [Crossref] [PubMed]
- Wong GL, Hui VW, Tan Q, et al. Novel machine learning models outperform risk scores in predicting hepatocellular carcinoma in patients with chronic viral hepatitis. JHEP Rep 2022;4:100441. [Crossref] [PubMed]
- Zhao Y, Li Y, Liu W, et al. Identification of noninvasive diagnostic biomarkers for hepatocellular carcinoma by urinary proteomics. J Proteomics 2020;225:103780. [Crossref] [PubMed]
- Zheng C, Zheng L, Yoo JK, et al. Landscape of Infiltrating T Cells in Liver Cancer Revealed by Single-Cell Sequencing. Cell 2017;169:1342-1356.e16. [Crossref] [PubMed]
- Rohr-Udilova N, Klinglmüller F, Schulte-Hermann R, et al. Deviations of the immune cell landscape between healthy liver and hepatocellular carcinoma. Sci Rep 2018;8:6220. [Crossref] [PubMed]
- Heinrich B, Gertz EM, Schäffer AA, et al. The tumour microenvironment shapes innate lymphoid cells in patients with hepatocellular carcinoma. Gut 2022;71:1161-75. [Crossref] [PubMed]
- Zhang Z, Ma L, Goswami S, et al. Landscape of infiltrating B cells and their clinical significance in human hepatocellular carcinoma. Oncoimmunology 2019;8:e1571388. [Crossref] [PubMed]
- Zheng X, Jin W, Wang S, et al. Progression on the Roles and Mechanisms of Tumor-Infiltrating T Lymphocytes in Patients With Hepatocellular Carcinoma. Front Immunol 2021;12:729705. [Crossref] [PubMed]
- Chen Y, Tian Z. HBV-Induced Immune Imbalance in the Development of HCC. Front Immunol 2019;10:2048. [Crossref] [PubMed]
- Dhanasekaran R. Deciphering Tumor Heterogeneity in Hepatocellular Carcinoma (HCC)-Multi-Omic and Singulomic Approaches. Semin Liver Dis 2021;41:9-18. [Crossref] [PubMed]