CXCL family-related classification predicts prognosis and response to immunotherapy in patients with head and neck squamous cell carcinoma based on TCGA and GEO databases
Highlight box
Key findings
• We have established a CXCL family-based gene clustering and risk score to predict the prognosis and immunotherapy efficacy of head and neck squamous cell carcinoma (HNSCC).
What is known and what is new?
• The CXCL family is an important predictor of clinical outcomes in various cancers.
• This study is the first to analyze the association between CXCL family genes and overall survival and immune infiltration in HNSCC through consensus clustering analysis.
What is the implication, and what should change now?
• The research results of this article lay the foundation for developing treatment targets for patients with head and neck squamous cell lung cancer.
Introduction
Head and neck squamous cell carcinoma (HNSCC) is the sixth most prevalent malignant cancer globally (1). Although conventional treatment methods have achieved some success, the 5-year survival rate of patients in advanced disease stages remains quite poor (2); thus, new prognostic markers are needed to inform clinical treatment decisions for patients with HNSCC.
The cysteine X cysteine ligand (CXCL) family is involved in recruiting immune cells and strongly influences the onset and growth of malignancies (3,4). For instance, CXCL1, CXCL5, and CXCL16 promote the development of gastric and pulmonary cancers (5-8). Additionally, CXCL1 is essential in the formation and spread of the initially malignant tumor microenvironment (TME) in colorectal cancer (9-11). By triggering epithelial-mesenchymal transition and activating neutrophils, CXCL5 promotes the spread of gastric cancer (12). CXCL9, CXCL10, CXCL13, CXCL14, and CXCL17 can be utilized as prognostic biomarkers in HNSCC (13); however, the precise functions of CXCL family genes in this cancer remain unclear. In recent years, therapeutic strategies have been developed to target the TME because of its key role in controlling tumor growth and modifying the effectiveness of standard treatments (14-16). Changes in the composition of the immune microenvironment have important implications for immunotherapeutic effects in patients with HNSCC (17-19). Because the CXCL family is crucial for controlling the TME (20,21), understanding its roles can help predict HNSCC development and immunotherapy effects, which can greatly improve patient outcomes.
In this study, we developed a set of relevant classifications of the CXCL family which were used to analyse the TME in HNSCC. Further, we developed CXCL family ratings to predict the prognosis, immune infiltration, and therapy outcomes in the patients. This classification can be used to guide the treatment and improve the outcomes of patients with HNSCC. We present this article in accordance with the TRIPOD reporting checklist (available at https://tcr.amegroups.com/article/view/10.21037/tcr-23-1299/rc).
Methods
Datasets
We downloaded the RNA sequencing and matched clinicopathological data of 379 patients with HNSCC as the training set from The Cancer Genome Atlas (TCGA; https://portal.gdc.cancer.gov/). Additionally, we chose two datasets from the Gene Expression Omnibus (GEO; https://www.ncbi.nlm.nih.gov/geo/; datasets GSE41613 and GSE85446) for use as validation sets. The study was conducted in accordance with the Declaration of Helsinki (as revised in 2013).
Differential gene expression (DGE) analysis
To investigate differences in gene expression levels between the comparison and control groups, we used the t-test program in the R statistical environment (The R Project for Statistical Computing, Vienna, Austria). To determine the false discovery rate (FDR) of each gene, we utilized the “p.adjust” tool. The following cut-offs were used to identify differentially expressed genes (DEGs): P<0.05, FDR <0.05, and |fold-change| >1.5.
Functional enrichment analyses
The signalling pathways and biological processes of the cluster1 and cluster2 patient populations were compared using Gene Ontology (GO) and Kyoto Encyclopedia of Genes and Genomes (KEGG) pathway enrichment analyses. The annotated genes were subjected to enrichment analysis using the R “clusterprofiler” package (version 3.14.3), with the following parameters: minimum gene set =5, maximum gene set =5,000. Gene sets were considered statistically significant when P<0.05 and FDR <0.25.
Gene Set Enrichment Analysis (GSEA) software
We utilized GSEA software (version 3.0; http://software.broadinstitute.org/gsea/index.jsp) to analyse the associated pathways and biological mechanisms, and to separate the samples into two groups depending on the expression of the CXCL family genes. We used the following parameters based on the gene expression profiles and phenotypic groupings: minimum gene set =5, maximum gene set =5,000, resampling =1,000, P<0.05, FDR <0.25.
Immune landscape among CXCL family gene subsets
Immuno-Oncology Biological Research (IOBR) is a computational tool that can utilize multiple omics data to promote exploration of immune oncology, reveal tumor immune interactions, and accelerate precision immunotherapy (22). Based on the expression profile, we used the R “iobr” tool and utilized two methods, estimate (23) and mcpcounter (24), to score immune-infiltrating cells.
Somatic mutation analysis
We detected gene mutations in the 379 patients with HNSCC using the R “maftools” function and presented the results in a waterfall diagram.
Consensus clustering
ConsensusClusterPlus (25) was used for cluster analysis, which involved resampling 80% of the samples ten times and employing agglomerative paired clustering with 1-Pearson correlation distances. Using an empirical cumulative distribution function plot, the ideal number of clusters was identified.
Construction of the CXCL family risk signature
We combined data on gene expression, survival time, and survival status using the R “glmnet” package and performed regression analysis using the least absolute shrinkage and selection operator (LASSO) Cox technique. In addition, we also set a 10-fold cross validation to obtain the optimal model, we set the Lambda value to 0.03 and ultimately obtained four genes. Additionally, we determined the best risk score cut-off value using the R “maxstat” test and set the minimum and maximum numbers of grouped samples as >25% and <75%, respectively. The patients were divided into high- and low-risk groups based on these criteria.
Creation and verification of a multifactor nomogram
We combined data on the survival time, survival status, and six features of patients with HNSCC using the R “rms” package. We then performed Cox analysis to create nomograms and assess the prognostic importance of these variables in HNSCC.
Survival analysis
We analysed prognostic differences between several groups of samples using the “survfit” function of the R survival package and utilized the log-rank test method to determine the significance of these differences.
Drug sensitivity analysis
We used “oncoPredict” to examine the medication sensitivity of clinically treated low- and high- risk patients. The filter conditions were P<0.05 and |log fold-change| >1.
Statistical analysis
We used Student t-test, Wilcoxon test, or one-way analysis of variance (ANOVA) for group comparison. The statistical significance was set to P<0.05, and the significance levels were expressed as *P<0.05, **P<0.01, ***P<0.001, and ****P<0.0001. Non significant differences were represented as ‘ns’.
Data processing
The technical methods provided by the Sangerbox toolkit (26) were used to process the data.
Results
Consensus clustering identifies subtypes in patients with HNSCC
Using the STRING database, we conducted protein-protein interaction network analysis to detect connections between CXCL family genes Figure 1A). We further investigated CXCL family gene expression in HNSCC using consensus clustering (Figure 1B-1E). Combined with the cumulative distribution curve, area under the distribution curve, and consistency analysis results of sample clustering, robust clustering was observed when k=2; this result indicated that patients could be divided into two categories, cluster1 (C1; 179 patients) and cluster2 (C2; 200 patients), which can be interpreted as different HNSCC subtypes. CXCL genes showed different expression patterns in the two subtypes (Figure 1F). Although some CXCL family genes showed higher expression in C2 than in C1, CXCL9, CXCL10, CXCL11, and CXCL13 expression levels were higher in C1 than in C2. The two subtypes were also linked to various clinical outcomes according to survival analysis. The prognosis of patients with the C1 subtype was generally better than that of patients with the C2 subtype (Figure 1G).
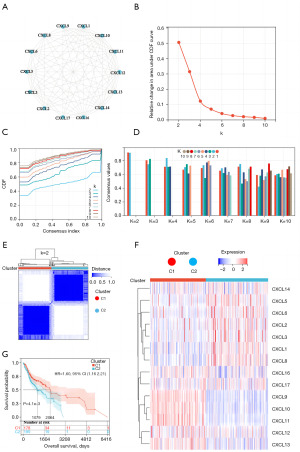
Functional enrichment and TME infiltration in several HNSCC subtypes
To assess the variations in gene expression between the C1 and C2 subtypes, we used the t-test function in R; we selected 731 DEGs for further analysis (Figure 2A,2B). Using the KEGG and GO databases, we conducted functional enrichment analyses of the DEGs. C1 was enriched in immunological pathways compared to C2 (Figure 2C,2D), including the T cell, natural killer cell, and B cell signalling pathways. Finally, we performed GSEA to identify pathways that were differentially represented in C1 versus C2 (Figure 2E-2M). The T cell and natural killer cell pathways were among the top nine enriched pathways and played important roles in the immune microenvironment. These findings suggested that the T cell pathway and natural killer cell pathway are more enriched in the C1 subtype than in the C2 subtype in GSEA enrichment. This indicates that the C1 subtype plays a more important role in the immune microenvironment.
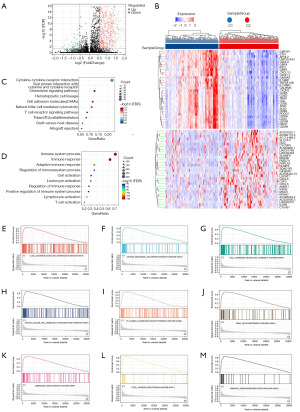
Mutational landscapes of the two subtypes of HNSCC
The two subtypes of HNSCC, C1 and C2, exhibited different somatic mutation profiles (Figure 3A). The most common mutations were in TP53, TTN, FAT1, CDKN2A, and muc16, and occurred at different frequencies between the subtypes. Furthermore, we investigated CXCL family mutations and found that C1 had higher CXCL13, CXCL3, and CXCL14 mutation frequencies than did C2 (Figure 3B). In contrast, C2 had higher CXCL1, CXCL6, CXCL10, CXCL12, and other genetic mutation frequencies than did C1.
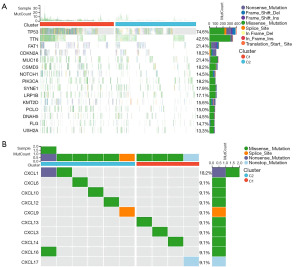
Mutational landscapes of the TME in different HNSCC subtypes
The importance of the CXCL family of genes in the TME is increasingly supported by available data. Further investigation of the compositional differences in the TME revealed that C1 had a higher immunological score (Figure 4A-4C) but lower purity than C2 (Figure 4D). Using the R “mcpcounter” package, we estimated variations in the proportions of invading immune cell types across the C1 and C2 subtypes; immune cell infiltration in the 379 patients with HNSCC is shown in Figure 4E. Additionally, we analysed the expression of 10 different immune cell types in the C1 and C2 subtypes; we discovered that, aside from endothelial cells and fibroblasts, patients with the C1 subtype had higher levels of the other eight immune cell types than patients with the C2 subtype (Figure 4F). Finally, we investigated the differences in immune checkpoints and human leukocyte antigen (HLA) cells between C1 and C2. Eight immune checkpoints and 19 HLAs were upregulated in patients with the C1 subtype (Figure 4G,4H). Thus, patients with the C1 subtype were more likely to develop hot tumors, whereas those with the C2 subtype were more likely to develop cold tumors.
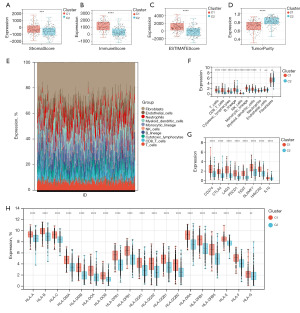
CXCL family gene univariate regression analysis
Through univariate regression analysis of the CXCL family, we found that CXCL13, CXCL17, CXCL9, and CXCL8 can serve as independent prognostic factors of HNSCC (Figure 5).
Differential expression and survival analysis of CXCL13, CXCL17, CXCL8, and CXCL9
CXCL13, CXCL8, and CXCL9 were lowly expressed in normal tissue and highly expressed in cancer tissue in patients with HNSCC, whereas CXCL17 was lowly expressed in normal tissue and highly expressed in cancer tissue (Figure 6A-6D). This was also true for patients with paired HNSCCs (Figure 6E-6H). Patients with HNSCC who overexpressed CXCL13, CXCL17, or CXCL9 genes had a better prognosis, whereas those with CXCL8 overexpression had a worse prognosis (Figure 6I-6L).
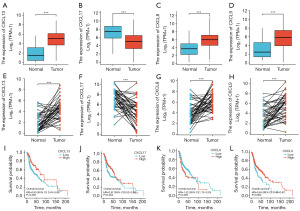
Risk model based on the CXCL family
To create a risk prediction model for patients with HNSCC, we used the R software package glmnet to integrate survival time, survival status, and gene expression data, and conducted regression analysis using the lasso box method. In addition, we also set a 10-fold cross validation to obtain the optimal model. We set the Lambda value to 0.0282483463007567 and ultimately obtained four genes. The model formula constructed is: RiskScore =0.0262776278846678 × CXCL1 + 0.022842939330026 × CXCL8 − 0.071226203344226 × CXCL13 − 0.0465917389070204 × CXCL17 (Figure 7A,7B). We also evaluated the relationships between survival status, risk scores, and risk genes. With an increase in risk variables, the survival rate of patients dramatically decreased. CXCL1 and CXCL8 were risk factors, whereas CXCL13 and CXCL17 were protective factors (Figure 7C). We used the R software package maxstat (Maximally selected rank statistics with sever P value approximations version: 0.7–25) to calculate the optimal cutoff value for RiskScore. We set the minimum group sample size to be greater than 25% and the maximum group sample size to be less than 75%, and ultimately obtained the optimal cutoff value of −0.272929657980261. Based on this, patients were divided into high and low groups, and further analyzed the prognostic differences between the two groups using the survival function of the R software package, we evaluated the significance of prognostic differences between different groups of samples using the logrank test method, and ultimately observed significant prognostic differences (P=5.9e−5) and higher risk scores were linked to poorer prognoses in the patients with HNSCC (Figure 7D).
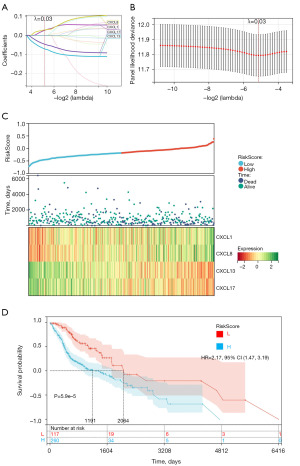
Risk factor-related clinical three-line table
In the TCGA dataset, we compared the clinical data of high- and low-risk patients and found substantial disparities between them in the T, M, and N stages (Table 1).
Table 1
Characteristic | High risk score (n=248) | Low risk score (n=107) | P |
---|---|---|---|
Event | 0.517 | ||
Alive | 143 (40.3) | 57 (16.1) | |
Dead | 105 (29.6) | 50 (14.1) | |
Sex | 0.464 | ||
Female | 64 (18.0) | 23 (6.5) | |
Male | 184 (51.8) | 84 (23.7) | |
T stage | <0.001 | ||
1 | 20 (5.6) | 1 (0.3) | |
2 | 90 (25.4) | 14 (3.9) | |
3 | 52 (14.6) | 38 (10.7) | |
4 | 86 (24.2) | 54 (15.2) | |
N stage | <0.001 | ||
0 | 175 (49.3) | 0 (0) | |
1 | 53 (14.9) | 2 (0.6) | |
2 | 20 (5.6) | 100 (28.2) | |
3 | 0 (0) | 5 (1.4) | |
M stage | 0.008 | ||
0 | 248 (69.9) | 103 (29.0) | |
1 | 0 (0) | 4 (1.1) | |
OS (days) | 695 [385.5, 1,334] | 548 [355.5, 1,028.5] | 0.062 |
Age (years) | 61 [54, 70] | 59 [52, 66] | 0.011 |
Data are presented as n (%) or median [IQR]. OS, overall survival; IQR, interquartile range.
Association between risk scores and the TME
We comprehensively evaluated the relationship between the risk score and TME. T cells, CD8 T cells, cytotoxic lymphocytes, B cell lineage, natural killer cells, monocytic lineage, and myeloid dendritic cells were negatively associated with risk score (Figure 8A-8G). Moreover, we found that the most common subtype among high-risk patients was C2, whereas that for low-risk patients was C1 (Figure 8H).
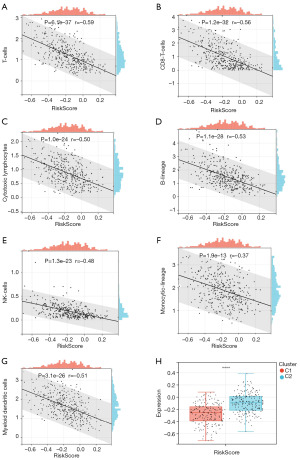
Creation of multivariate nomograms to predict survival
We combined data on the survival time, survival status, and six factors using the R “rms” package; we then utilized the Cox method to create nomograms to predict the survival of patients with HNSCC at one, three, and five years (Figure 9A). A calibration curve for the patient nomogram was drawn. Patient age, M stage, and risk score affected patient survival status at all time-points (Figure 9B). Further, we used multivariate regression analysis to predict patient prognosis. Patients with high-risk scores had a poor prognosis, which was supported by the results of receiver operating characteristic (ROC) analysis (Figure 9C). Finally, our prediction model was validated using two GEO datasets, gse41613 and gse85446 (Figure 9D,9E).
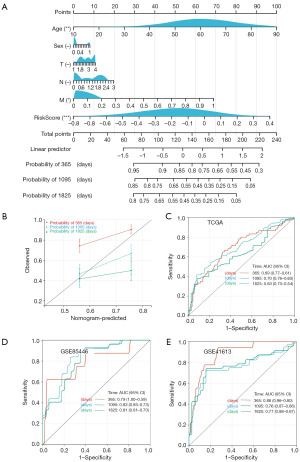
Drug sensitivity test of 379 HNSCC patients
We used “oncoPredict” to perform drug sensitivity analysis on samples from the low- and high-risk groups (Figure 10A-10C). Three drugs showing large differences in drug sensitivity were selected by screening the filter conditions with thresholds of P<0.05 and |log fold-change| >1.0.
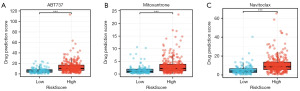
Discussion
The mucosal epithelial cells of the oral cavity, pharynx, and larynx are the primary source of most head and neck cancers. Smoking and alcohol misuse are typically linked to oral and laryngeal malignancies, whereas human papillomavirus infection, particularly human papillomavirus-16, is increasingly assumed to cause pharyngeal cancer (27). Programmed death 1 (PD-1) is a receptor protein on the surface of T cells in immune cells, which interacts with a protein PD-L1 expressed on the surface of tumor cells. PD-L1, full name programmed cell death ligand 1, also known as programmed cell death ligand 1 (28). With advancements in immunotherapy, patients with HNSCC can obtain good treatments, specifically those receiving PD-1 and PD-L1 immunotherapy (29). Cells secrete small molecules known as chemokines; increasing evidence indicates that in various malignancies, CXC chemokines regulate the TME to direct tumor cell proliferation, invasion, and metastasis (30-33). CXCL family genes in HNSCC and their effect on the TME have not been widely examined. In this study, we divided 379 patients with HNSCC into two subtypes, C1 and C2, through consensus clustering. In addition, we explored differences in the prognosis and immunotherapeutic responses of patients between these subtypes. Previous studies have only focused on the influence of a single gene from the CXCL family on HNSCC prognosis (34,35). Here, we developed a new set of typing techniques to explore the effects of CXCL genes on patients with different HNSCC subtypes. This new typing system can guide decisions on immunotherapy treatment plans in patients with HNSCC.
The TME is extremely complex and contains tumor cells and the cellular elements surrounding them, including lymphocytes, tumor vasculature, and immune cells that have infiltrated the tumor (36-38). Notably, CXCLs recruit immune cells. For example, CXCL10 can regulate CD8+ T cell responses during chronic infection (39); CXCL1, CXCL22, and CXCL8 are crucial for changing the immune microenvironment in colon cancer (40); and CXCL9 controls the recruitment of stem-like CD8+ T cells that express CXCR3 (41,42). Based on these reports, we used the TCGA database to establish an HNSCC prognostic model that utilized CXCL family typing. The large differences in prognosis and immune cell infiltration between patients with the C1 and C2 subtypes are reflected in our model. Our classification system can also determine whether a tumor is hot or cold, which can guide immunotherapy choices. For improved clinical applications, we gave each patient with HNSCC in our study a risk factor score. Patients with low-risk factors had a good prognosis, high immune cell infiltration ratio, and good drug sensitivity. Future treatments for people with HNSCC can benefit from this paradigm.
However, our model had some limitations. For instance, we only considered three publicly available datasets (TCGA data, GSE41613, and GSE85446), and more accurate clinical patient data were lacking. In future studies, we would need to collect additional clinical data to further verify our prognostic model.
Conclusions
We developed an accurate prognosis prediction model by examining the effect of CXCL genes on the prognosis, immunological microenvironment, and treatment sensitivity of patients with HNSCC. Our findings emphasize the clinical importance of the CXCL family type in this disease, which is helpful for predicting the outcomes of and customizing immunotherapy in patients with HNSCC.
Acknowledgments
We thank the TCGA and GEO databases for providing the data used in this study. The data used can be found at TCGA (https://gdc.cancer.gov/) and GEO (https://www.ncbi.nlm.nih.gov/geo/; datasets GSE41613 and GSE85446) databases.
Funding: This work was supported by
Footnote
Reporting Checklist: The authors have completed the TRIPOD reporting checklist. Available at https://tcr.amegroups.com/article/view/10.21037/tcr-23-1299/rc
Peer Review File: Available at https://tcr.amegroups.com/article/view/10.21037/tcr-23-1299/prf
Conflicts of Interest: All authors have completed the ICMJE uniform disclosure form (available at https://tcr.amegroups.com/article/view/10.21037/tcr-23-1299/coif). The authors have no conflicts of interest to declare.
Ethical Statement: The authors are accountable for all aspects of the work in ensuring that questions related to the accuracy or integrity of any part of the work are appropriately investigated and resolved. The study was conducted in accordance with the Declaration of Helsinki (as revised in 2013).
Open Access Statement: This is an Open Access article distributed in accordance with the Creative Commons Attribution-NonCommercial-NoDerivs 4.0 International License (CC BY-NC-ND 4.0), which permits the non-commercial replication and distribution of the article with the strict proviso that no changes or edits are made and the original work is properly cited (including links to both the formal publication through the relevant DOI and the license). See: https://creativecommons.org/licenses/by-nc-nd/4.0/.
References
- Sung H, Ferlay J, Siegel RL, et al. Global Cancer Statistics 2020: GLOBOCAN Estimates of Incidence and Mortality Worldwide for 36 Cancers in 185 Countries. CA Cancer J Clin 2021;71:209-49. [Crossref] [PubMed]
- Ferris RL, Blumenschein G Jr, Fayette J, et al. Nivolumab for Recurrent Squamous-Cell Carcinoma of the Head and Neck. N Engl J Med 2016;375:1856-67. [Crossref] [PubMed]
- Wu L, Awaji M, Saxena S, et al. IL-17-CXC Chemokine Receptor 2 Axis Facilitates Breast Cancer Progression by Up-Regulating Neutrophil Recruitment. Am J Pathol 2020;190:222-33. [Crossref] [PubMed]
- Chen S, Yang Y, He S, et al. Review of biomarkers for response to immunotherapy in HNSCC microenvironment. Front Oncol 2023;13:1037884. [Crossref] [PubMed]
- Bian D, Chen Y. Bioinformatics Analysis of Prognostic Significance and Immune Characteristics of CXC Chemokine Family in Patients with Lung Adenocarcinoma. Comput Math Methods Med 2022;2022:3918926. [Crossref] [PubMed]
- Zhou Z, Xia G, Xiang Z, et al. A C-X-C Chemokine Receptor Type 2-Dominated Cross-talk between Tumor Cells and Macrophages Drives Gastric Cancer Metastasis. Clin Cancer Res 2019;25:3317-28. [Crossref] [PubMed]
- Zhou X, Fang D, Liu H, et al. PMN-MDSCs accumulation induced by CXCL1 promotes CD8(+) T cells exhaustion in gastric cancer. Cancer Lett 2022;532:215598. [Crossref] [PubMed]
- Su P, Jiang L, Zhang Y, et al. Crosstalk between tumor-associated macrophages and tumor cells promotes chemoresistance via CXCL5/PI3K/AKT/mTOR pathway in gastric cancer. Cancer Cell Int 2022;22:290. [Crossref] [PubMed]
- Wang D, Sun H, Wei J, et al. CXCL1 Is Critical for Premetastatic Niche Formation and Metastasis in Colorectal Cancer. Cancer Res 2017;77:3655-65. [Crossref] [PubMed]
- Zhou J, Xu W, Wu Y, et al. GPR37 promotes colorectal cancer liver metastases by enhancing the glycolysis and histone lactylation via Hippo pathway. Oncogene 2023;42:3319-30. [Crossref] [PubMed]
- Łukaszewicz-Zając M, Zajkowska M, Pączek S, et al. The Significance of CXCL1 and CXCR1 as Potential Biomarkers of Colorectal Cancer. Biomedicines 2023;11:1933. [Crossref] [PubMed]
- Mao Z, Zhang J, Shi Y, et al. CXCL5 promotes gastric cancer metastasis by inducing epithelial-mesenchymal transition and activating neutrophils. Oncogenesis 2020;9:63. [Crossref] [PubMed]
- Li Y, Wu T, Gong S, et al. Analysis of the Prognosis and Therapeutic Value of the CXC Chemokine Family in Head and Neck Squamous Cell Carcinoma. Front Oncol 2020;10:570736. [Crossref] [PubMed]
- Bejarano L, Jordāo MJC, Joyce JA. Therapeutic Targeting of the Tumor Microenvironment. Cancer Discov 2021;11:933-59. [Crossref] [PubMed]
- Kong J, Xu S, Zhang P, et al. CXCL1 promotes immune escape in colorectal cancer by autophagy-mediated MHC-I degradation. Hum Immunol 2023;84:110716. [Crossref] [PubMed]
- Adegoke NA, Gide TN, Mao Y, et al. Classification of the tumor immune microenvironment and associations with outcomes in patients with metastatic melanoma treated with immunotherapies. J Immunother Cancer 2023;11:e007144. [Crossref] [PubMed]
- Wang X, Guo J, Yu P, et al. The roles of extracellular vesicles in the development, microenvironment, anticancer drug resistance, and therapy of head and neck squamous cell carcinoma. J Exp Clin Cancer Res 2021;40:35.
- Shan F, Cillo AR, Cardello C, et al. Integrated BATF transcriptional network regulates suppressive intratumoral regulatory T cells. Sci Immunol 2023;8:eadf6717. [Crossref] [PubMed]
- Weed DT, Zilio S, McGee C, et al. The Tumor Immune Microenvironment Architecture Correlates with Risk of Recurrence in Head and Neck Squamous Cell Carcinoma. Cancer Res 2023;83:3886-900. [Crossref] [PubMed]
- Zhang W, Wang H, Sun M, et al. CXCL5/CXCR2 axis in tumor microenvironment as potential diagnostic biomarker and therapeutic target. Cancer Commun (Lond) 2020;40:69-80. [Crossref] [PubMed]
- Tokunaga R, Zhang W, Naseem M, et al. CXCL9, CXCL10, CXCL11/CXCR3 axis for immune activation - A target for novel cancer therapy. Cancer Treat Rev 2018;63:40-7. [Crossref] [PubMed]
- Zeng D, Ye Z, Shen R, et al. IOBR: Multi-Omics Immuno-Oncology Biological Research to Decode Tumor Microenvironment and Signatures. Front Immunol 2021;12:687975. [Crossref] [PubMed]
- Yoshihara K, Shahmoradgoli M, Martínez E, et al. Inferring tumour purity and stromal and immune cell admixture from expression data. Nat Commun 2013;4:2612. [Crossref] [PubMed]
- Becht E, Giraldo NA, Lacroix L, et al. Estimating the population abundance of tissue-infiltrating immune and stromal cell populations using gene expression. Genome Biol 2016;17:218. [Crossref] [PubMed]
- Wilkerson MD, Hayes DN. ConsensusClusterPlus: a class discovery tool with confidence assessments and item tracking. Bioinformatics 2010;26:1572-3. [Crossref] [PubMed]
- Shen W, Song Z, Zhong X, et al. Sangerbox: A comprehensive, interaction-friendly clinical bioinformatics analysis platform. iMeta 2022;1:e36.
- Johnson DE, Burtness B, Leemans CR, et al. Head and neck squamous cell carcinoma. Nat Rev Dis Primers 2020;6:92. [Crossref] [PubMed]
- Tang Q, Chen Y, Li X, et al. The role of PD-1/PD-L1 and application of immune-checkpoint inhibitors in human cancers. Front Immunol 2022;13:964442. [Crossref] [PubMed]
- Solomon B, Young RJ, Rischin D. Head and neck squamous cell carcinoma: Genomics and emerging biomarkers for immunomodulatory cancer treatments. Semin Cancer Biol 2018;52:228-40. [Crossref] [PubMed]
- Struyf S, Proost P, Van Damme J. Regulation of the immune response by the interaction of chemokines and proteases. Adv Immunol 2003;81:1-44. [Crossref] [PubMed]
- Richmond A. Chemokine modulation of the tumor microenvironment. Pigment Cell Melanoma Res 2010;23:312-3. [Crossref] [PubMed]
- Ishimoto N, Park JH, Kawakami K, et al. Structural basis of CXC chemokine receptor 1 ligand binding and activation. Nat Commun 2023;14:4107. [Crossref] [PubMed]
- Shi T, Li X, Zheng J, et al. Increased SPRY1 expression activates NF-κB signaling and promotes pancreatic cancer progression by recruiting neutrophils and macrophages through CXCL12-CXCR4 axis. Cell Oncol (Dordr) 2023;46:969-85. [Crossref] [PubMed]
- Zhao Z, Ma Y, Lv J, et al. Expression of chemokine CXCL8/9/10/11/13 and its prognostic significance in head and neck cancer. Medicine (Baltimore) 2022;101:e29378. [Crossref] [PubMed]
- Dufies M, Grytsai O, Ronco C, et al. New CXCR1/CXCR2 inhibitors represent an effective treatment for kidney or head and neck cancers sensitive or refractory to reference treatments. Theranostics 2019;9:5332-46.
- Binnewies M, Mujal AM, Pollack JL, et al. Unleashing Type-2 Dendritic Cells to Drive Protective Antitumor CD4(+) T Cell Immunity. Cell 2019;177:556-571.e16. [Crossref] [PubMed]
- Zhang Z, Zeng X, Wu Y, et al. Cuproptosis-Related Risk Score Predicts Prognosis and Characterizes the Tumor Microenvironment in Hepatocellular Carcinoma. Front Immunol 2022;13:925618.
- Sathe A, Grimes SM, Lau BT, et al. Single-Cell Genomic Characterization Reveals the Cellular Reprogramming of the Gastric Tumor Microenvironment. Clin Cancer Res 2020;26:2640-53. [Crossref] [PubMed]
- Ozga AJ, Chow MT, Lopes ME, et al. CXCL10 chemokine regulates heterogeneity of the CD8(+) T cell response and viral set point during chronic infection. Immunity 2022;55:82-97.e8. [Crossref] [PubMed]
- Jia SN, Han YB, Yang R, et al. Chemokines in colon cancer progression. Semin Cancer Biol 2022;86:400-7. [Crossref] [PubMed]
- Marcovecchio PM, Thomas G, Salek-Ardakani S. CXCL9-expressing tumor-associated macrophages: new players in the fight against cancer. J Immunother Cancer 2021;9:e002045. [Crossref] [PubMed]
- Humblin E, Kamphorst AO. CXCR3-CXCL9: It's All in the Tumor. Immunity 2019;50:1347-9. [Crossref] [PubMed]