Preliminary exploration of the potential biological functions and prognosis values of RAB4B in pan-cancer combing with experimental validation in BLCA
Highlight box
Key findings
• Ras-related protein Rab-4B (RAB4B) may be a promising biomarker for tumor immunotherapy.
What is known and what is new?
• RAB4B can predict the prognosis of pan-cancers.
• These study findings offer insights for a better understanding of the prevention and treatment of tumors and provide the foundation for precision medicine.
What is the implication, and what should change now?
• No specific mechanism of the relationship between RAB4B and tumor prognosis was elucidated. Future studies should be conducted using more pan-cancer datasets to validate the current findings, thus establishing the prognosis model based on clinical data and RAB4B.
Introduction
In most countries, cancer is predicted to overtake cardiovascular disease in the 21st century, posing a serious obstacle to increasing life expectancy (1). Although surgery, chemotherapy, and radiotherapy are the most recommended conventional cancer treatment strategies, they are associated with adverse effects, which impact the quality of patients’ life. Therefore, there is still much room for improvement on our idealized anti-cancer targets. Intriguingly, it has been well-documented that immune manipulation works as a basis for cancer treatment (2). With the advent of breakthroughs in immune checkpoint blockade (ICB) therapy, cancer immunotherapy has become a key treatment strategy for cancer, which has yielded significant success, and age-standardized 5-year relative survival rates for most cancers have increased substantially in recent years (3-5). However, a clinical study has shown that although checkpoint inhibition immunotherapies have achieved significant survival benefits in certain populations, approximately 40–60% of patients do not benefit from these therapies (6). Therefore, the exploration of predictive biomarkers for immunotherapy efficacy is urgent.
Ras-related protein Rab-4B (RAB4B), a small GTPase in the RAS superfamily, has been linked to various processes such as glucose homeostasis, synaptic homeostasis, and adaptive immunity (7-10). RAB4B is considered a key protein in T cells, which contributes to the control of the white adipose tissue amplification and insulin sensitivity by regulating fat Th17/Treg balance (7). RAB4B has also been implicated in tumorigenesis, and its dysregulation has been associated with cancer in various organs (11). Research has shown that RAB4 can promote tumor proliferation by controlling protein hydrolysis and mesenchymal invasion processes (12). Besides, MIA-RAB4B was found to be specifically upregulated in colorectal polyps, and was considered to function as a competitive endogenous RNA to titrate away micro-RNA 24, causing the up-regulation of its other target oncogenes (13). It has also been shown that RAB4B plays a role in hepatocellular carcinoma and cholangiocarcinogenesis (14). Immunological study has shed light on the molecular link between RAB4B transcriptional activation and major histocompatibility complex class II genes, further demonstrating the essential functions of RAB4B in mediating endocytic recycling, thereby enhancing the antigen presentation capacity of antigen-presenting cells (9). Nevertheless, it is still unknown whether RAB4B has a role in human pan-cancers and whether it is predictive for immunotherapy.
Using RAB4B as a prognostic marker in pan-cancers, we conducted a comprehensive analysis. The analytical workflow of the research is described in Figure 1. The findings demonstrated that RAB4B is a reliable biomarker for predicting the outcome of pan-cancer patients and their response to immunotherapy. We present this article in accordance with the REMARK reporting checklist (available at https://tcr.amegroups.com/article/view/10.21037/tcr-23-1840/rc).
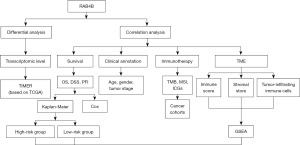
Methods
Sample information and the expression profiles of RAB4B gene in human cancers
Data associated with 33 cancers (as shown in Table 1) were downloaded from the University of California Santa Cruz Xena (https://xena.ucsc.edu/). With Practical Extraction and Report Language (PERL), expression matrices of RAB4B were extracted from all samples. Our analysis and visualization of the RAB4B expression levels in a variety of cancer types were conducted by the R package “ggpubr”. By converting all expression data to log2, all expression data were normalized.
Table 1
Abbreviations | Full name |
---|---|
ACC | Adrenocortical carcinoma |
BLCA | Bladder urothelial carcinoma |
BRCA | Breast invasive carcinoma |
CESC | Cervical squamous cell carcinoma |
CHOL | Cholangiocarcinoma |
COAD | Colon adenocarcinoma |
DLBC | Lymphoid neoplasm diffuse large B-cell lymphoma |
ESCA | Esophageal carcinoma |
GBM | Glioblastoma multiforme |
LGG | Brain lower grade glioma |
HNSC | Head and neck squamous cell carcinoma |
KICH | Kidney chromophobe |
KIRC | Kidney renal clear cell carcinoma |
KIRP | Kidney renal papillary cell carcinoma |
LAML | Acute myeloid leukemia |
LIHC | Liver hepatocellular carcinoma |
LUAD | Lung adenocarcinoma |
LUSC | Lung squamous cell carcinoma |
MESO | Mesothelioma |
OV | Ovarian serous cystadenocarcinoma |
PAAD | Pancreatic adenocarcinoma |
PCPG | Pheochromocytoma and paraganglioma |
PRAD | Prostate adenocarcinoma |
READ | Rectum adenocarcinoma |
SARC | Sarcoma |
SKCM | Skin cutaneous melanoma |
STAD | Stomach adenocarcinoma |
TGCT | Testicular germ cell tumors |
THCA | Thyroid carcinoma |
THYM | Thymoma |
UCEC | Uterine corpus endometrial carcinoma |
UCS | Uterine carcinosarcoma |
UVM | Uveal melanoma |
TCGA, The Cancer Genome Atlas.
Bladder urothelial carcinoma (BLCA) tissues were surgically resected from patients at the First Affiliated Hospital of Guangxi Medical University from December 2022 to March 2023. BLCA tissues and normal tissues (n=6) were collected from the same patients and rapidly stored in liquid nitrogen for further experiments. The study was conducted in accordance with the Declaration of Helsinki (as revised in 2013). The study was approved by the Ethics Review Committee of the First Affiliated Hospital of Guangxi Medical University (No. 2022-E388-01) and informed consent was taken from all the patients.
Correlation analysis of RAB4B expression with survival and clinicopathology characteristics in pan-cancers
A correlation analysis between the RAB4B expression and overall survival (OS), disease-specific survival (DSS), and progression-free interval (PFI) were performed in 33 cancers using Kaplan-Meier curves. The correlation between RAB4B and OS in different cancer types was further analyzed using the Kaplan-Meier plotter (https://kmplot.com/). Hazard ratios (HRs) and 95% confidence intervals (95% CIs) were calculated using univariate Cox analysis and visualized by the R package “forestplot”. Subsequently, a multivariate Cox analysis was conducted using R package “survival” to determine whether RAB4B is an independent prognostic factor in pan-cancer. R packages “limma” and “ggpubr” were used to analyze the correlation between RAB4B expression and clinicopathological features.
Tumor mutational burden (TMB) and microsatellite instability (MSI) are correlated with RAB4B expression in pan-cancers
“Masked Somatic Mutation” data were downloaded from The Cancer Genome Atlas (TCGA) database (https://portal.gdc.cancer.gov/) and processed with VarScan2. A Perl script was then used to integrate data from the samples that contained TMB or MSI. Then R package “fmsb” was used to analyze the correlation between RAB4B expression and TMB or MSI.
Tumor microenvironment (TME) in pan-cancers tissues and their relationship with RAB4B expression
The ESTIMATE algorithm was utilized to calculate immune and stromal scores to predict tumor purity and the presence of stromal/immune cells in pan-cancers tissues. Immune cell infiltrations were assessed using the CIBERSORT algorithm. With the help of R packages “ggplot2”, “ggpubr”, and “ggExtra”, we analyzed the correlation between RAB4B expression and TME.
Co-expression of immune-related gene and associated pathways in pan-cancers
Using the R package “limma” and the packages “reshape2” and “RColorBrewer”, we analyzed the correlation. Gene Ontology (GO) and Kyoto Encyclopedia of Genes and Genomes (KEGG) gene sets were downloaded from the gene set enrichment analysis (GSEA) website (https://www.gsea-msigdb.org/gsea/downloads.jsp).
RNA extraction and quantitative reverse transcription polymerase chain reaction (qRT-PCR)
According to the manufacturer’s protocol, total RNAs were isolated using an Axygen reagent (CORNING, Nanning, China). The primers sequences used for qRT-PCR were obtained from Sangon Biotech (Shanghai, China) as below (5'-3'): RAB4B forward-ATGGCTGAGACCTACGACTTC, reverse-CGCCGATTGTGTGGTTGGA. β-actin forward-GTCATTCCAAATATGAGATGCGT, reverse-GCTATCACCTCCCCTGTGTG. Relative expression levels were calculated according to the 2−ΔΔCt method. Statistical analyses were performed with GraphPad Prism.
Immunohistochemical (IHC) staining
IHC staining was performed after deparaffinization in xylene and rehydration in graded ethanol. The antigen was retrieved with sodium citrate buffer (pH 6.0) at 95 ℃ for 20 minutes. A 3% H2O2 solution was used to quench endogenous peroxidase activity and a 1% bovine serum albumin buffer was used to block non-specific binding. After that, sections were incubated with the primary antibodies overnight at 4 ℃ and then exposed to secondary antibodies for 40 minutes at room temperature after going through several washes. 3,3-diaminobenzidine tetrahydrochloride (DAB) was then applied to the sections. Image J software was used to analyze the intensity of staining. The slices were finally observed under the microscope, followed by optical density (OD) measurement by Image J software. The relative expression of RAB4B was presented as average OD.
Western blotting
Total tissue protein was extracted with powerful RIPA lysate buffer (Vazyme Biotech, Nanjing, China) containing protease inhibitors (Beyotime, Beijing, China), and the protein concentration was then quantified. After adding the 5× sodium dodecyl sulfate-polyacrylamide gel (SDS-PAGE) protein loading buffer, the protein sample was placed in a 100 ℃ metal bath for 10 minutes. The proteins were separated by 12% SDS-PAGE electrophoresis and then transferred to the polyvinylidene fluoride (PVDF) membrane (Beyotime). The membrane was sealed with 5% skimmed milk for 1 hour and then washed thrice for 5 minutes in Tris Buffered Saline with Tween 20 (TBST). After that, the membrane was incubated with primary antibody at 4 ℃ overnight, then washed in TBST, and incubated with secondary antibody at room temperature for 1 hour. Finally, the target stripe signals were detected using the HRP substrate (Merck Millipore, USA) and analyzed using Image J software and GraphPad Prism. The antibodies were provided in Table 2.
Table 2
Antibody | Company | Host | Dilution |
---|---|---|---|
RAB4B | CUSABIO Technology, Houston, USA | Rabbit | 1:2,500 |
β-actin | Proteintech, Wuhan, China | Mouse | 1:5,000 |
Goat anti-rabbit | Thermo Scientific, Shanghai, China | Goat | 1:10,000 |
Goat anti-mouse | Proteintech, Wuhan, China | Goat | 1:5,000 |
RAB4B, Ras-related protein Rab-4B.
Statistical analysis
R software (v 4.1.2), Image J software (v 1.8), and GraphPad Prism (v 9.4) were applied for all statistical analyses and diagram drawings. The Wilcox rank sum test was used to analyze the expression profiles of RAB4B. Univariate and multivariate Cox regression analysis were used to calculate the HR and P value among clinicopathological features. To determine the survival times in patients with high- and low-level expressing genes, a Kaplan-Meier curve analysis was performed. P<0.05 was used as the significance cut-off.
Results
RAB4B is aberrantly expressed in pan-cancers
To elucidate the expression characteristics of RAB4B. The Tumor Immune Estimation Resource (TIMER) database was used to analyze the expression levels of RAB4B. Results showed that RAB4B was significantly increased in BLCA, breast invasive carcinoma (BRCA), cholangiocarcinoma (CHOL), colon adenocarcinoma (COAD), esophageal carcinoma (ESCA), head and neck squamous cell carcinoma (HNSC), kidney renal clear cell carcinoma (KIRC), liver hepatocellular carcinoma (LIHC), and uterine corpus endometrial carcinoma (UCEC) tissues compared with normal adjacent tissues (Figure 2A), with the results from the TCGA database manifesting similar trends (Figure 2B). Collectively, these results suggested that RAB4B was aberrantly expressed in pan-cancers.
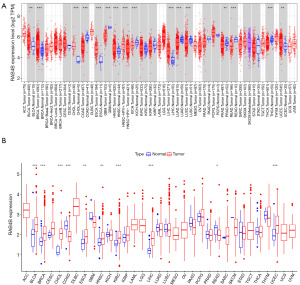
Relationship between RAB4B expression and prognosis of pan-cancers
Following that, survival metrics were analyzed about RAB4B expression. As shown in Figure 3A-3C, high RAB4B expression was positively correlated with the prognosis of four different cancer types, including BLCA (OS: P<0.001; DSS: P=0.002; PFI: P=0.003), pancreatic adenocarcinoma (PAAD) (OS: P=0.003; DSS: P=0.001; PFI: P<0.001), skin cutaneous melanoma (SKCM) (OS: P=0.013; DSS: P=0.008), and kidney renal papillary cell carcinoma (KIRP) (DSS: P=0.040). Conversely, high RAB4B expression had a negative effect on the prognosis of three other types of cancer, which included KIRC (OS: P<0.001; DSS: P<0.001; PFI: P=0.008), brain lower grade glioma (LGG) (OS: P=0.035; DSS: P=0.035), and uveal melanoma (UVM) (OS: P=0.007; DSS: P=0.020). To further confirm the relationship between RAB4B and prognosis of pan-cancer, we analyzed the correlation between RAB4B and OS in pan-cancer using the online tool Kaplan-Meier plotter. The results also demonstrate a positive correlation between high expression of RAB4B and prognosis in BLCA, KIRP, PAAD, pheochromocytoma and paraganglioma (PCPG), cervical squamous cell carcinoma (CESC), and BRCA, while showing a negative correlation with prognosis in KIRC (Figure 3D).
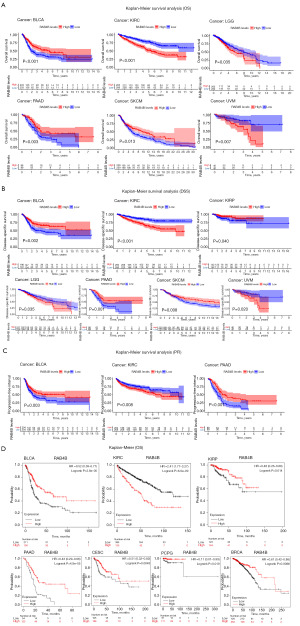
Univariate Cox analysis showed that high RAB4B expression played a negative prognostic role in KIRC (OS: HR =2.372, 95% CI: 1.807–3.114, P<0.001; DSS: HR =2.818, 95% CI: 2.025–3.920, P<0.001; PFI: HR =1.768, 95% CI: 1.314–2.378, P<0.001), LGG (OS: HR =1.593, 95% CI: 1.113–2.282, P=0.011; DSS: HR =1.552, 95% CI: 1.054–2.285, P=0.026; PFI: HR =1.366, 95% CI: 1.024–1.822, P=0.034), and UVM (OS: HR =4.494, 95% CI: 1.872–10.787, P<0.001; DSS: HR =3.632, 95% CI: 1.459–9.041, P=0.006), whereas, high RAB4B expression played a protective prognostic role in BLCA (OS: HR =0.612, 95% CI: 0.465–0.806, P<0.001; DSS: HR =0.559, 95% CI: 0.398–0.784, P<0.001; PFI: HR =0.629, 95% CI: 0.478–0.828, P<0.001), BRCA (OS: HR =0.686, 95% CI: 0.509–0.925, P=0.014), CESC (OS: HR =0.473, 95% CI: 0.284–0.787, P=0.004; DSS: HR =0.454, 95% CI: 0.253–0.815, P=0.008; PFI: HR =0.544, 95% CI: 0.330–0.899, P=0.018), PAAD (OS: HR =0.528, 95% CI: 0.366–0.762, P<0.001; DSS: HR =0.430, 95% CI: 0.283–0.654, P<0.001; PFI: HR =0.487, 95% CI: 0.341–0.694, P<0.001), PCPG (OS: HR =0.201, 95% CI: 0.046–0.886, P=0.034; DSS: HR =0.109, 95% CI: 0.016–0.748, P=0.024), SKCM (OS: HR =0.769, 95% CI: 0.609–0.972, P=0.028; DSS: HR =0.725, 95% CI: 0.561–0.937, P=0.014), and HNSC (DSS: HR =0.662, 95% CI: 0.450–0.973, P=0.036) (Figure 4A-4C).
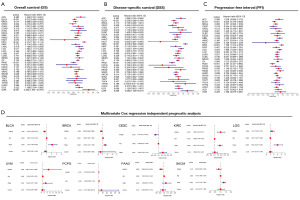
Based on the results of univariate Cox analysis, we conducted multivariate Cox analysis to assess the association of RAB4B with patient outcomes in various cancers (including BLCA, BRCA, CESC, KIRC, SKCM, LGG, PAAD, PCPG, and UVM), while controlling for potential confounding factors such as tumor histological stage, age, and gender. Our findings indicate that, with the exception of SKCM (HR =0.832, P=0.175), RAB4B serves as an independent prognostic factor for the remaining eight cancer types, including BLCA (HR =0.614, P=0.001), BRCA (HR =0.634, P=0.003), CESC (HR =0.547, P=0.032), KIRC (HR =1.908, P<0.001), LGG (HR =1.472, P=0.034), PAAD (HR =0.585, P=0.005), PCPG (HR =0.173, P=0.031), and UVM (HR =4.987, P=0.002) (Figure 4D). Overall, these results shed the light on the fact that the potential of RAB4B as a biomarker in predicting the survival outcome of various cancer patients, and its effect varies across tumor types.
Relevance of RAB4B expression to the clinicopathology of pan-cancers
Analysis revealed that RAB4B was highly expressed in stages I and II of BLCA, lung adenocarcinoma (LUAD), and testicular germ cell tumors (TGCT), but it was overexpressed in advanced stages of KIRC and SKCM (Figure 5A). RAB4B was highly expressed in patients aged ≥65 years with BRCA, ESCA, and rectum adenocarcinoma (READ). Conversely, its expression levels were decreased in patients aged ≥65 years with prostate adenocarcinoma (PRAD) and thymoma (THYM) (Figure 5B). Besides, RAB4B was highly expressed in male patients with glioblastoma multiforme (GBM) and sarcoma (SARC) and lowly expressed in male patients with SKCM (Figure 5C). Together, these results demonstrated that RAB4B was closely linked to multiple clinicopathological characteristics of cancer patients, further validating its crucial role as a biomarker reflecting the disease stages of pan-cancers.
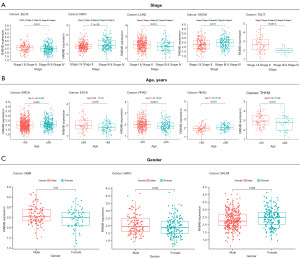
The expression of RAB4B in pan-cancers and its relationship to TMB or MSI
The relationship was analyzed to determine the efficacy of RAB4B as a potential biomarker for cancer immunotherapy. Results demonstrated that the expression levels of RAB4B were positively correlated with TMB in ESCA, while it was negatively correlated in THYM, stomach adenocarcinoma (STAD), PRAD, acute myeloid leukemia (LAML), and kidney chromophobe (KICH) (Figure 6A). Likewise, we were able to verify that RAB4B had a positive correlation with MSI in thyroid carcinoma (THCA), SKCM, LUAD, HNSC, lymphoid neoplasm diffuse large B-cell lymphoma (DLBC), and BRCA, while it was negatively correlated in TGCT, STAD, and GBM (Figure 6B). Based on these findings, RAB4B was found to be a reliable biomarker for predicting tumor response to immunotherapy.
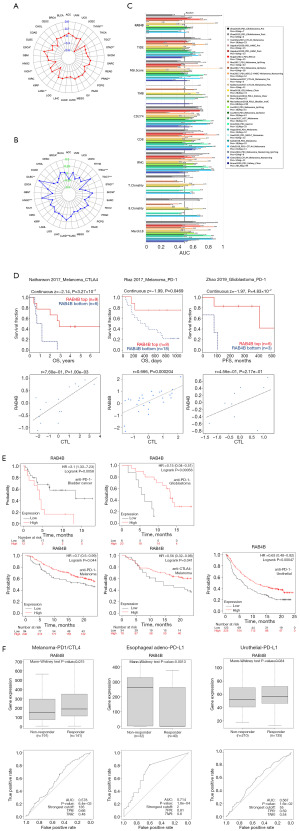
Furthermore, the tumor immune dysfunction and exclusion (TIDE) algorithm was used to determine whether RAB4B would be predictive as immunotherapy. Interestingly, the outcome revealed that the area under the receiver operating characteristic curve (AUC) for RAB4B was >0.5 in 10 of the 19 ICB sub-cohorts (Figure 6C). The predictive value of RAB4B was higher than that of TMB, T cell clonality, and B cell clonality, with AUC-values of >0.5 in eight, nine, and seven sub-cohorts, respectively. Besides, we also found that RAB4B expression levels were positively correlated with the survival outcome of in melanoma and GBM sub-cohorts treated with anti-programmed cell death protein 1 (PD-1) or anti-cytotoxic T-lymphocyte-associated protein 4 (CTLA4) (Figure 6D).
Moreover, to further assess the predictive role of RAB4B in ICB therapy, we also investigated its prognostic significance in cancer immunotherapy using additional online databases, including Kaplan-Meier plotter and ROC plotter (https://www.rocplot.org/). The results demonstrate a positive correlation between RAB4B expression levels and survival time in melanoma patients treated with anti-PD-1 or anti-CTLA4 therapy. Additionally, a positive association was observed between RAB4B expression and survival time in patients with GBM treated with anti-PD-1 therapy, as well as in patients with urothelial carcinoma who received anti-programmed death-ligand 1 (PD-L1) therapy (Figure 6E,6F). The above results suggest that there is a correlation between RAB4B and the efficacy of ICB treatment, so it has the potential to be a predictor of immunotherapy.
Correlation between RAB4B expression and TME
Next, the correlation between RAB4B expression and TME was assessed across cancers. Based on the survival outcomes of patients with pan-cancers obtained from Kaplan-Meier curves, pan-cancers were classified into a high-risk group, including KIRC, LGG, and UVM, and a low-risk group, including BLCA, PAAD, SKCM, and KIRP. As shown in Figure 7A, RAB4B expression was positively correlated with the immune score of KIRP and the immune and stromal scores of SKCM, however, an adverse correlation was found between the stromal score of PAAD and RAB4B. Similarly, RAB4B was positively correlated with the immune score of KIRC and the immune and stromal scores of LGG and UVM (Figure 7B). Collectively, our data indicate that RAB4B may mediate the varying components in TME, thus influencing the survival of pan-cancer patients.
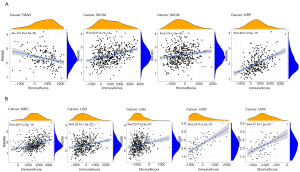
Relationship between RAB4B expression and immune cell infiltration
An examination of the correlation between RAB4B expression and tumor-infiltrating immune cells was conducted in pan-cancers to explore the relationship between RAB4B expression and immune cell infiltration. The findings revealed that RAB4B expression significantly positively correlated with CD8+ T cells, regulatory T cells (Tregs), and so forth, and negatively correlated with resting mast cells, resting memory CD4+ T cells, neutrophils, M2 Macrophages, M0 Macrophages, etc. (Figure 8A-8O).
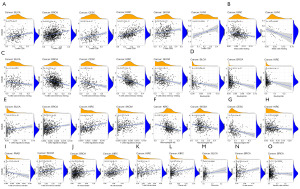
A positive correlation was found between RAB4B and several immune-related genes, including T cell immunoreceptors with immunoglobulin and ITIM domain (TIGIT), programmed cell death 1 (PDCD1), galectin-9 (LGALS9), lymphocyte-activation gene 3 (LAG3), killer-cell immunoglobulin-like receptor 2DL3 (KIR2DL3), and interleukin-10 receptor subunit beta (IL10RB), in SKCM, HNSC, LIHC, and TGCT, and this phenomenon was the most significant in SKCM (Figure 9). These results suggested that RAB4B was associated with immune microenvironment remodeling.
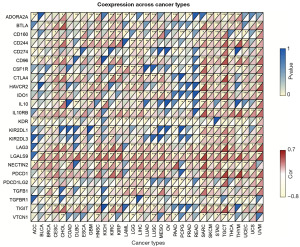
Analysis of the functional enrichment set of RAB4B in pan-cancers
GSEA analysis was performed to understand molecular mechanisms that regulate RAB4B in different tumor types. GO analysis showed that RAB4B negatively regulated biological processes such as epidermis development, keratinization, and skin development in BLCA. However, RAB4B positively regulated the above biological processes in PAAD, SKCM, KIRP, KIRC, LGG, and UVM. In addition, GO analysis revealed that RAB4B positively regulated molecular functions, including mRNA binding and olfactory receptor activity, in KIRP (Figure 10A). KEGG pathway analysis further demonstrated that RAB4B positively regulated several key immune cell-related pathways, including ascorbate and alternate metabolism, chemokine signaling pathway, cytokine-cytokine receptor interaction, and pentose and glucuronate interconversions, in BLCA, SKCM, LGG and UVM (Figure 10B). Overall, these results indicated that RAB4B positively regulated biological processes and immune cell-related pathways in most cancers.
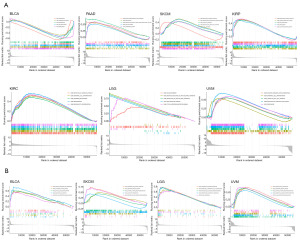
Differential expressions of RAB4B between BLCA and normal samples
According to the TCGA database, we found that RAB4B expression was significantly up-regulated in BLCA compared to para-cancer tissues (P<0.001; Figure 2). To further verify the differential expression of RAB4B in BLCA and normal adjacent tissues, we carried out IHC staining, qPCR, and western blotting. Our results indicated that compared to normal tissue, RAB4B was significantly up-regulated in cancerous tissue in BLCA patients (Figure 11A-11D).
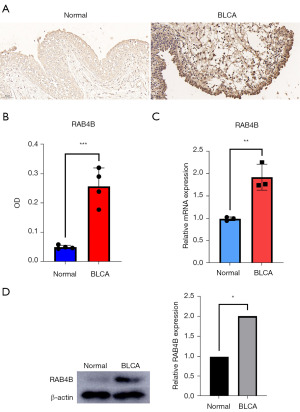
Discussion
To date, cancer remains a hot topic in the healthcare industry. An estimated 19.3 million new cancer cases and nearly 10 million cancer deaths were reported worldwide in 2020 alone, underscoring the burden of cancer (15). Socioeconomic status is strongly positively correlated with cancer incidence rate (16). An estimated 1.9 million new cancer cases and 608,570 cancer deaths occurred in the United States in 2021, corresponding to over 1,600 deaths per day (17). The advent of immunotherapy, which overcomes tumor-induced immunosuppression and enables the immune system to kill cancer cells, represents a paradigm shift in cancer treatment. In the wake of the approval of immune checkpoint inhibitors (ICIs) for the treatment of a wide range of cancers, including melanoma, non-small cell lung cancer, stomach cancer, HNSC, and renal cell carcinoma, immunotherapy is increasingly applied in clinical cancer treatments (18). Although immunotherapy has achieved better results than conventional and targeted therapies in some tumors, many patients do not respond to immunotherapy (19). Besides, it has been well documented that the incidence of adverse events associated with ICIs is between 54% and 76% (20). Therefore, the search for precision biomarkers is urgent, which may promote the use of precision medicine in cancer treatment.
In recent years, the role played by immune checkpoint genes (ICGs) in pan-cancers has been gradually revealed. ICGs may reveal the immune status of tumors and serve as a prognostic predictor of cancers (21,22). RAB4B has been implicated in processes such as the regulation of glucose homeostasis, synaptic homeostasis, and adaptive immunity (10). However, its function in pan-cancers has not yet been explored. The results of the present study showed that RAB4B is highly expressed in most tumor tissues in comparison to normal tissues when analyzed in pan-cancers databases. Interestingly, RAB4B was lowly expressed in COAD and READ. This proved that expression of RAB4B in tumor tissues was abnormal and showed an inconsistent trend in different tumors. Moreover, our analysis of RAB4B expression and survival metrics showed that high RAB4B expression was positively correlated with the prognosis of BLCA, PAAD, SKCM, and KIRP, while on the contrary, it was negatively correlated with the prognosis of KIRC, LGG, and UVM. In addition, our results indicated that RAB4B was highly expressed in phases I and II of BLCA, LUAD, and TGCT, and in advanced stages of KIRC and SKCM. This indicates that it is of great significance to preliminarily estimate the survival and prognosis of these cancer patients based on the expression level of RAB4B. Besides, RAB4B is associated with biological processes (epidermis development, keratinization, and skin development) and plays a key role in several immune cell-related pathways (ascorbate and alternate metabolism, chemokine signaling pathway, cytokine-cytokine receptor interaction, and pentose and glucuronate interconversions). Accordingly, RAB4B upregulation contributes to the development of hepatocellular carcinoma and cholangiocarcinogenesis (14). Collectively, our data indicated that RAB4B has the potential to provide new insights into the treatment and prognosis of pan-cancers.
As aforementioned, although immunotherapy has anticancer effects, not all patients benefit from this treatment. For decades, there has been an ongoing search for ideal biomarkers as predictors of response to PD-1/PD-L1 blockade across multiple tumor types (23). US Food and Drug Administration (FDA) approval for pre-treatment prediction of cancer immunotherapy using PD-L1 has been granted for several cancers, such as melanoma, gastric cancer, and bladder cancer, making it an effective predictive biomarker (24-26). Noteworthily, a previous study found that PD-L1 predicted tumors in 28.9% of cases for 15 approved tumor types and that it did not predict (53.3%) or was not tested in the remaining cases (27). This suggests that there is hope in the quest for reliable tumor biomarkers. TMB, the total number of mutations in each coding region of the tumor genome, can be used to predict ICI efficacy (28). Interestingly, a significantly longer survival period was observed in the high-TMB group in comparison with the low-TMB group, suggesting that TMB is related to the prognosis of BLCA, and it has emerged as a useful biomarker for multiple cancer types to identify patients who may benefit from immunotherapy (29,30). On the other hand, a study has shown that MSI, independent of tumor histology and completely dependent on tumor gene composition, is a pan-cancer biomarker for predicting immunotherapy response (31). A previous report found that high-MSI colorectal cancer patients were significantly more sensitive to ICIs than low-MSI patients (32). Nonetheless, there are numerous problems with these biomarkers (33). Thus, considerable attention has been given to ICGs, which link TMB and MSI and could be a reliable indicator to predict the effect of immunotherapy (34). Herein, we verified that RAB4B was associated with TMB in ESCA, THYM, STAD, PRAD, LAML, and KICH. RAB4B was found to be associated with MSI in THCA, SKCM, LUAD, HNSC, DLBC, BRCA, TGCT, STAD, and GBM. The results indicated that RAB4B has the potential to become an accurate biomarker for tumor immunotherapy, broadening the horizon for precision oncology.
TME, the complex multicellular environment in which tumorigenesis occurs, typically includes immune cells, stromal cells, extracellular matrix, and other secretory molecules, as well as blood and lymphatic vascular networks (35). It is well known that immune cell infiltration shows inconsistent prognostic value depending on the type of cancer. High immune cell infiltration indicated a worse prognosis in LGG and UVM. Contrarily, a favorable prognosis has been demonstrated in SKCM, which is consistent with the results obtained in this paper (36). Noteworthily, TIGIT and LAG3 exhibit high expression levels in the TME. A study has demonstrated a link between T-cell exhaustion and ICB efficacy (37). Herein, RAB4B was positively correlated with immune and stromal scores in most cancer patients. And RAB4B is associated with immune-related genes, such as TIGIT and LAG3. Notably, TIDE analysis results maintained that the AUC for RAB4B was >0.5 in 10 of the 19 ICB sub-cohorts and RAB4B had a better predictive value. Together, these findings indicate that RAB4B may be a potentially promising prognostic indicator and a predictive biomarker for immunotherapy in pan-cancers.
Although our study yielded some key findings, it also has several limitations. For example, the pan-cancers database is too single, the research on prognosis is not in-depth enough, and no specific mechanism of the relationship between RAB4B and tumor prognosis was elucidated. Future studies should be conducted using more pan-cancer datasets to validate the current findings, thus establishing the prognosis model based on clinical data and RAB4B. Moreover, the P values we presented were not corrected for multiple comparisons through multiple tests, but our research results still have reference value and can serve as a basis for further research. In addition, in vivo and in vitro experiments are needed to elucidate the mechanisms by which RAB4B affects tumor prognosis. Finally, based on differential expression, prognostic correlation, sample collection feasibility, and other factors, only BLCA was selected for validation. Nevertheless, the validation of a single cancer is inadequate to reflect the relationship between RAB4B and the prognosis of multiple cancers, larger sample sizes and additional validation cohorts that incorporate more cancer types are imperatively warranted.
Conclusions
In summary, RAB4B can predict the prognosis of pan-cancers. Moreover, RAB4B may be a promising biomarker for tumor immunotherapy. These study findings offer insights for a better understanding of the prevention and treatment of tumors and provide the foundation for precision medicine.
Acknowledgments
The authors are grateful to the First Affiliated Hospital of Guangxi Medical University for their support of the present study.
Funding: This work was supported by
Footnote
Reporting Checklist: The authors have completed the REMARK reporting checklist. Available at https://tcr.amegroups.com/article/view/10.21037/tcr-23-1840/rc
Data Sharing Statement: Available at https://tcr.amegroups.com/article/view/10.21037/tcr-23-1840/dss
Peer Review File: Available at https://tcr.amegroups.com/article/view/10.21037/tcr-23-1840/prf
Conflicts of Interest: All authors have completed the ICMJE uniform disclosure form (available at https://tcr.amegroups.com/article/view/10.21037/tcr-23-1840/coif). The authors have no conflicts of interest to declare.
Ethical Statement: The authors are accountable for all aspects of the work in ensuring that questions related to the accuracy or integrity of any part of the work are appropriately investigated and resolved. The study was conducted in accordance with the Declaration of Helsinki (as revised in 2013). The study was approved by the Ethics Review Committee of the First Affiliated Hospital of Guangxi Medical University (No. 2022-E388-01) and informed consent was taken from all the patients.
Open Access Statement: This is an Open Access article distributed in accordance with the Creative Commons Attribution-NonCommercial-NoDerivs 4.0 International License (CC BY-NC-ND 4.0), which permits the non-commercial replication and distribution of the article with the strict proviso that no changes or edits are made and the original work is properly cited (including links to both the formal publication through the relevant DOI and the license). See: https://creativecommons.org/licenses/by-nc-nd/4.0/.
References
- Bray F, Laversanne M, Weiderpass E, et al. The ever-increasing importance of cancer as a leading cause of premature death worldwide. Cancer 2021;127:3029-30. [Crossref] [PubMed]
- Gotwals P, Cameron S, Cipolletta D, et al. Prospects for combining targeted and conventional cancer therapy with immunotherapy. Nat Rev Cancer 2017;17:286-301. [Crossref] [PubMed]
- Pardoll DM. The blockade of immune checkpoints in cancer immunotherapy. Nat Rev Cancer 2012;12:252-64. [Crossref] [PubMed]
- Miao Y, Wang J, Li Q, et al. Prognostic value and immunological role of PDCD1 gene in pan-cancer. Int Immunopharmacol 2020;89:107080. [Crossref] [PubMed]
- Zeng H, Chen W, Zheng R, et al. Changing cancer survival in China during 2003-15: a pooled analysis of 17 population-based cancer registries. Lancet Glob Health 2018;6:e555-67. [Crossref] [PubMed]
- Maleki Vareki S, Garrigós C, Duran I. Biomarkers of response to PD-1/PD-L1 inhibition. Crit Rev Oncol Hematol 2017;116:116-24. [Crossref] [PubMed]
- Gilleron J, Bouget G, Ivanov S, et al. Rab4b Deficiency in T Cells Promotes Adipose Treg/Th17 Imbalance, Adipose Tissue Dysfunction, and Insulin Resistance. Cell Rep 2018;25:3329-3341.e5. [Crossref] [PubMed]
- Hou L, Cai MJ, Liu W, et al. Small GTPase Rab4b participates in the gene transcription of 20-hydroxyecdysone and insulin pathways to regulate glycogen level and metamorphosis. Dev Biol 2012;371:13-22. [Crossref] [PubMed]
- Krawczyk M, Leimgruber E, Seguín-Estévez Q, et al. Expression of RAB4B, a protein governing endocytic recycling, is co-regulated with MHC class II genes. Nucleic Acids Res 2007;35:595-605. [Crossref] [PubMed]
- Perrin L, Lacas-Gervais S, Gilleron J, et al. Rab4b controls an early endosome sorting event by interacting with the γ-subunit of the clathrin adaptor complex 1. J Cell Sci 2013;126:4950-62. [Crossref] [PubMed]
- Stephen AG, Esposito D, Bagni RK, et al. Dragging ras back in the ring. Cancer Cell 2014;25:272-81. [Crossref] [PubMed]
- Frittoli E, Palamidessi A, Marighetti P, et al. A RAB5/RAB4 recycling circuitry induces a proteolytic invasive program and promotes tumor dissemination. J Cell Biol 2014;206:307-28. [Crossref] [PubMed]
- Thean LF, Wong YH, Lo M, et al. Chromosome 19q13 disruption alters expressions of CYP2A7, MIA and MIA-RAB4B lncRNA and contributes to FAP-like phenotype in APC mutation-negative familial colorectal cancer patients. PLoS One 2017;12:e0173772. [Crossref] [PubMed]
- He H, Dai F, Yu L, et al. Identification and characterization of nine novel human small GTPases showing variable expressions in liver cancer tissues. Gene Expr 2002;10:231-42. [Crossref] [PubMed]
- Sung H, Ferlay J, Siegel RL, et al. Global Cancer Statistics 2020: GLOBOCAN Estimates of Incidence and Mortality Worldwide for 36 Cancers in 185 Countries. CA Cancer J Clin 2021;71:209-49. [Crossref] [PubMed]
- Lortet-Tieulent J, Georges D, Bray F, et al. Profiling global cancer incidence and mortality by socioeconomic development. Int J Cancer 2020;147:3029-36. [Crossref] [PubMed]
- Siegel RL, Miller KD, Fuchs HE, et al. Cancer Statistics, 2021. CA Cancer J Clin 2021;71:7-33. [Crossref] [PubMed]
- Billan S, Kaidar-Person O, Gil Z. Treatment after progression in the era of immunotherapy. Lancet Oncol 2020;21:e463-76. [Crossref] [PubMed]
- O'Donnell JS, Teng MWL, Smyth MJ. Cancer immunoediting and resistance to T cell-based immunotherapy. Nat Rev Clin Oncol 2019;16:151-67. [Crossref] [PubMed]
- Xu C, Chen YP, Du XJ, et al. Comparative safety of immune checkpoint inhibitors in cancer: systematic review and network meta-analysis. BMJ 2018;363:k4226. [Crossref] [PubMed]
- Hu FF, Liu CJ, Liu LL, et al. Expression profile of immune checkpoint genes and their roles in predicting immunotherapy response. Brief Bioinform 2021;22:bbaa176. [Crossref] [PubMed]
- Kather JN, Suarez-Carmona M, Charoentong P, et al. Topography of cancer-associated immune cells in human solid tumors. Elife 2018;7:e36967. [Crossref] [PubMed]
- Gong J, Chehrazi-Raffle A, Reddi S, et al. Development of PD-1 and PD-L1 inhibitors as a form of cancer immunotherapy: a comprehensive review of registration trials and future considerations. J Immunother Cancer 2018;6:8. [Crossref] [PubMed]
- Kaushik I, Ramachandran S, Zabel C, et al. The evolutionary legacy of immune checkpoint inhibitors. Semin Cancer Biol 2022;86:491-8. [Crossref] [PubMed]
- Huynh J, Patel K, Gong J, et al. Immunotherapy in Gastroesophageal Cancers: Current Evidence and Ongoing Trials. Curr Treat Options Oncol 2021;22:100. [Crossref] [PubMed]
- Roviello G, Catalano M, Santi R, et al. Immune Checkpoint Inhibitors in Urothelial Bladder Cancer: State of the Art and Future Perspectives. Cancers (Basel) 2021;13:4411. [Crossref] [PubMed]
- Davis AA, Patel VG. The role of PD-L1 expression as a predictive biomarker: an analysis of all US Food and Drug Administration (FDA) approvals of immune checkpoint inhibitors. J Immunother Cancer 2019;7:278. [Crossref] [PubMed]
- Yarchoan M, Hopkins A, Jaffee EM. Tumor Mutational Burden and Response Rate to PD-1 Inhibition. N Engl J Med 2017;377:2500-1. [Crossref] [PubMed]
- Wang C, Chen S, Li S, et al. A Prognostic Model for Predicting Tumor Mutation Burden and Tumor-Infiltrating Immune Cells in Bladder Urothelial Carcinoma. Front Genet 2022;13:708003. [Crossref] [PubMed]
- Chan TA, Yarchoan M, Jaffee E, et al. Development of tumor mutation burden as an immunotherapy biomarker: utility for the oncology clinic. Ann Oncol 2019;30:44-56. [Crossref] [PubMed]
- Chang L, Chang M, Chang HM, et al. Microsatellite Instability: A Predictive Biomarker for Cancer Immunotherapy. Appl Immunohistochem Mol Morphol 2018;26:e15-21. [Crossref] [PubMed]
- Le DT, Uram JN, Wang H, et al. PD-1 Blockade in Tumors with Mismatch-Repair Deficiency. N Engl J Med 2015;372:2509-20. [Crossref] [PubMed]
- Ramos-Casals M, Brahmer JR, Callahan MK, et al. Immune-related adverse events of checkpoint inhibitors. Nat Rev Dis Primers 2020;6:38. [Crossref] [PubMed]
- Chen S, Su X, Mo Z. KCNN4 is a Potential Biomarker for Predicting Cancer Prognosis and an Essential Molecule that Remodels Various Components in the Tumor Microenvironment: A Pan-Cancer Study. Front Mol Biosci 2022;9:812815. [Crossref] [PubMed]
- Bejarano L, Jordāo MJC, Joyce JA. Therapeutic Targeting of the Tumor Microenvironment. Cancer Discov 2021;11:933-59. [Crossref] [PubMed]
- Zuo S, Wei M, Wang S, et al. Pan-Cancer Analysis of Immune Cell Infiltration Identifies a Prognostic Immune-Cell Characteristic Score (ICCS) in Lung Adenocarcinoma. Front Immunol 2020;11:1218. [Crossref] [PubMed]
- Zheng L, Qin S, Si W, et al. Pan-cancer single-cell landscape of tumor-infiltrating T cells. Science 2021;374:abe6474. [Crossref] [PubMed]