A four-gene signature predicts overall survival of patients with esophageal adenocarcinoma
Highlight box
Key findings
• We present a novel signature that incorporates four important prognostic genes (ALAD, ABLIM3, IL17RB and IFI6) to predict the overall survival (OS) for esophageal adenocarcinoma (EAC) patients.
What is known and what is new?
• Prognostic assessment is a crucial step in the management of patients with EAC, yet there are no globally reliable and reproducible prognostic markers that can identify esophageal cancer patients at high risk of mortality.
• The signature identified in this study exhibited a strong correlation with patients OS. Multivariate Cox regression analysis further indicated that its prognostic ability was independent of commonly used clinical features and may serve as an independent prognostic biomarker.
What is the implication, and what should change now?
• Our work is a supplement to the identification of molecular prognostic biomarkers in the field of EAC. Next, a large scale multicenter trial is necessary to validate its reliability and applicability in clinical practice.
Introduction
Among cancers, esophageal cancer ranks seventh in terms of incidence and sixth in mortality. It was estimated that there would be 604,100 new esophageal cancer cases and 544,076 deaths worldwide in 2020 (1). Esophageal cancer has two major histological subtypes: esophageal adenocarcinoma (EAC) and esophageal squamous cell carcinoma (ESCC). ESCC accounts for about 90% of esophageal cancer cases worldwide, while EAC cases have been progressively increasing and comprise the majority of esophageal cancer cases in the United States and some western European countries (2,3). Owing to the lack of adequate diagnostic methods, esophageal cancer is usually detected at an advanced stage. For patients diagnosed during 2006 to 2012 in the United States, the 5-year relative survival rate was only 18%, which was much lower than that of many other solid tumors (4). Thus, it is imperative to establish reliable and reproducible prognostic markers that can identify esophageal cancer patients at high risk of mortality.
Currently, the strongest clinical prognostic factor in patients with EAC is the tumor, node, metastasis (TNM) stage (5). The 5-year survival rates decrease substantially from 80.5% in the small proportion of EAC patients with stage I tumors, to 45.1%, 17.6% and, 2.1% for patients with stage II, III, and IV tumors, respectively (3). However, the adequacy for TNM stage to predict prognosis of EAC is questioned repeatedly with limited ability to stratify patients who are stage-matched and nonetheless present considerable variations in clinical outcomes (6). Cancers are heterogeneous at the molecular and genetic levels (7,8). Clearly, the ideal staging system would consider the unique molecular mechanisms underlying EAC development and progression and correlate prognosis with specific tumor biomarkers.
There have been some studies focused on finding molecular prognostic factors of EAC. A recent study, assessing the effect of human epidermal growth factor receptor 2 (HER2) heterogeneity on survival among HER2-amplified EACs, indicated that HER2 heterogeneity was independently prognostic for both disease-free survival (DFS, P=0.025) and overall survival (OS, P=0.026) (9). Another study showed that low antigen-presenting molecule human leukocyte antigen DR (HLA-DR) expression in leading edge tumor epithelium was an independent predictor of poor survival, associated with a 2.8-fold increase in disease-associated death (P=0.023) (10). Using gene expression analysis and array-comparative genomic hybridization arrays, Goh et al. and Peters et al. have identified eight biologically relevant molecular targets associated with EAC prognosis, including TRIM44, SIRT2, EGFR, PAPSS2, NEIL2, WT1, MTMR9 and DCK (11,12). Further study has established and validated that the immunohistochemical panel consisting of EGFR, TRIM44 and SIRT2 was independently associated with OS and provided additional prognostic information to current survival predictors, such as TNM stage (13). Unfortunately, clinical useful molecular biomarkers for EAC prognosis are still scarce. Further study is needed to obtain accurate prognostic information for improving patient staging and management decisions.
With the development of microarray and sequencing technology, high-throughput data grow rapidly. Public databases such as Gene Expression Omnibus (GEO, https://www.ncbi.nlm.nih.gov/geo/) database and The Cancer Genome Atlas (TCGA, http://cancergenome.nih.gov/) provide us enormous expression data of multiple cancer types including esophageal cancer (14-17). Thus, it appears reasonable and practicable to determine prognosis-related biomarkers through bioinformatical ways. Recently, a number of studies have successfully used the in silico data to identify potential prognostic signature in esophageal cancer. A previous study by Fan et al. identified from TCGA an eight-long non-coding RNA signature for OS prediction of esophageal cancer (18). Another 8-long non-coding RNA signature from GSE53625 was identified to incorporate with age and pathologic stage for predicting 3- and 5-year survival probability of patients with ESCC (19). Furthermore, recent studies paid more attention to constructing prognostic signatures involved in specific biological process, such as autophagy (20), lactic acid metabolism (21), necroptosis (22,23) and ferroptosis (24), which explained their functional association with prognostic outcomes better. However, all the above analysis was conducted in single ESCC cohort or the total esophageal cohort regardless of subtypes, suggesting that the EAC public data still need a more sufficient utilization.
In this study, we used public high-throughput data from TCGA and GEO databases and identified a four-gene signature comprised of ALAD, ABLIM3, IL17RB and IFI6 as a predictor of OS in EAC patients. This signature exhibited a strong correlation with patient OS and may serve as an independent prognostic biomarker. The results obtained provided a base for further validation of this molecular signature as a prognostic biomarker in EAC patients. We present this article in accordance with the TRIPOD reporting checklist (available at https://tcr.amegroups.com/article/view/10.21037/tcr-23-1798/rc).
Methods
Gene expression profiles of EAC
Gene expression profiles of GSE13898 and GSE26886 were downloaded from GEO. The GSE13898 data set included 64 primary EAC tissues and 28 surrounding non-cancerous fresh frozen tissues. The GSE26886 data set included 21 specimens of adenocarcinoma patients and 19 biopsies of normal esophageal squamous epithelium. The preprocessed level 3 RNA sequencing data and corresponding clinical information of esophageal cancer patients were obtained from TCGA database, which included 80 EAC tissues and 11 adjacent tissues. For GSE13898, normalized data were provided directly by the authors. For GSE26886, the analysis of raw probe-level data (.CEL file) was performed using the robust multiarray average algorithm RMA in the Affy package of R (25) after background correction and quantile normalization, and the expression values were then obtained. The averages of the probe sets of values were calculated as the expression values for the same gene with multiple probe sets (26). For TCGA profiles, row counts data were normalized by ‘‘edgeR’’ R package to obtain the normalized expression data (27). The study was conducted in accordance with the Declaration of Helsinki (as revised in 2013).
Differentially expressed genes (DEGs) screening between EAC tissues and non-cancerous tissues
For GEO profiles, DEGs between EAC tissues and non-cancerous tissues were identified through the “Limma” package in R software (28). Genes with |log2 (fold change)| >1 and P<0.05 were considered to be differentially expressed. For TCGA profiles, the R package of “edgeR” was employed to identify the DEGs following the same threshold (27). The DEGs from each data set were then intersected to obtain common DEGs for next analysis.
Identification of DEGs related prognostic signature and Kaplan-Meier analysis
The “survival” R package was used to evaluate the association between DEGs and patient OS by univariate Cox proportional hazards regression analysis. Only those DEGs with P value <0.05 were reserved for a stepwise multivariate Cox proportional hazards regression analysis tested by Akaike information criterion (AIC) to identify the prognostic model with the best performance. Then, the survival risk score (SRS) for each patient was calculated by summing the mRNA expression level of each gene multiplied with its corresponding coefficient from the multivariate Cox regression analysis. Using the median risk score as the cutoff value, patients were divided into high-risk and low-risk groups. Kaplan-Meier method was used to generate the OS curves, and log-rank test was employed to compare the differences between high-risk and low-risk patient groups. Time-dependent receiver operating characteristic (ROC) curves were generated to detect the prognostic power of the risk score model, using “survivalROC” R package.
Multivariate Cox regression analysis and stratification analysis
To assess whether the survival prediction ability of the DEGs signature was independent of other clinical or pathological factors of patients with EAC, multivariate Cox proportional hazards regression analysis was carried out. The included variates covered clinical or pathological factors which had been identified to be significant in the univariate Cox regression analysis. For clinical features with P value <0.05 in multivariate Cox regression analysis, stratification analysis was further performed to determine whether the DEGs signature exhibit prognostic value within the same clinical feature.
Functional enrichment analysis
The Gene Ontology (GO) annotation analysis was conducted using the Database for Annotation, Visualization, and Integrated Discovery (DAVID) (29) to obtain enriched GO terms of biological processes with P<0.05 as the threshold value. Pathway enrichment analysis was performed using KOBAS 3.0 (http://kobas.cbi.pku.edu.cn/), which is the first hypergeometric distribution-based examination software to evaluate the significance of enrichment of pathways (30). P<0.05 was also set as the threshold value.
Statistical analysis
All statistical analyses were conducted using Graphpad Prism 5.0 (GraphPad Software Inc., San Diego, CA, USA), SPSS (IBM Statistics, Armonk, NY, USA) and R 3.5.0 (R Foundation for Statistical Computing, Vienna, Austria).
Results
Identification of DEGs between EAC tissues and non-cancerous tissues
According to the cutoff criteria, 2,330 (861 upregulated, 1,469 downregulated), 3,545 (1,892 upregulated, 1,653 downregulated), and 3,740 (1,413 upregulated, 2,327 downregulated) differentially expressed genes were identified from GSE13898, GSE26886 and TCGA, respectively (available online: https://cdn.amegroups.cn/static/public/tcr-23-1798-1.xls). Among them, 336 genes showed the same trend of change in these three data sets, including 145 upregulated genes and 191 downregulated genes in EAC tissues compared with normal or paracancerous tissues.
Establishment of DEGs related prognostic model to predict OS of patients with EAC
By performing univariate Cox proportional hazards regression analysis using TCGA follow-up data, 14 OS related DEGs (available online: https://cdn.amegroups.cn/static/public/tcr-23-1798-1.xls) were identified with P value <0.05, and they were used for a stepwise multivariate Cox proportional hazards regression analysis. Finally, a four-gene predictive signature with the best explanatory and informative efficacy tested by AIC was established, which included ALAD, ABLIM3, IL17RB and IFI6. Kaplan-Meier curves of the four prognostic genes using median expression level as the cutoff value are shown in Figure 1. As described previously, the prognostic model was defined as the linear combination of the expression levels of these four genes weighted by their individual coefficient from the multivariate Cox regression analysis. The formula of SRS was as follows: SRS = (−0.0336 × ALAD mRNA expression value) + (−0.0485 × ABLIM3 mRNA expression value) + (0.0101 × IL17RB mRNA expression value) + (−0.0004 × IFI6 mRNA expression value). ALAD, ABLIM3 and IFI6 showed negative coefficients, indicating that they were protective prognostic factors, while IL17RB was considered to be an unfavorable prognostic factor with a positive coefficient.
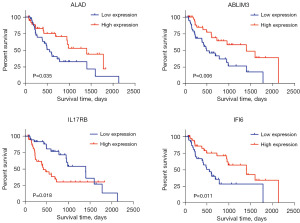
Kaplan-Meier analysis and ROC curve indicated good performance of the four-gene signature in predicting the OS of EAC
According to the established prognostic model, SRS of each patient was calculated, and the distribution of SRSs and survival status are shown in Figure 2A,2B. Using a median risk score of −1.5425 as the cutoff value, a set of 40 patients with SRSs >−1.5425 were assigned into the high-risk group, while the other 40 patients were assigned into the low-risk group. The OS for patients in high-risk group was significantly poorer than those in low-risk group (P=4.92e−05<0.0001), with a much shorter median survival time (480 vs. 1599 days for high-risk vs. low-risk, Figure 2C). Time-dependent ROC analysis showed that the prognostic signature performed well for EAC OS prediction, as the areas under the curves (AUCs) of the time-dependent ROC curve were 0.804, 0.792 and 0.695 for 1-, 3- and 5-year survival prediction, respectively (Figure 2D).
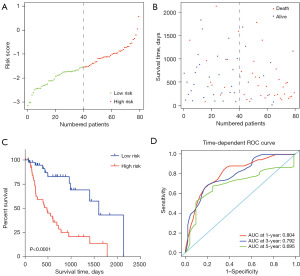
Survival prediction of the four-gene signature was independent of other clinicopathological factors
The main clinical and pathological factors of the enrolled 80 EAC patients are listed in Table 1. Univariate Cox regression analysis identified N classification, metastasis and tumor stage as well as the four-gene-defined SRS group as the candidate prognostic factors (Table 2). Multivariate Cox regression analysis demonstrated that only the four-gene signature maintained an independent prognostic factor after adjusting for the above factors (Table 2). Tumor stages was shown to be probably another independent prognostic factor with a P value of 0.07, which was close to 0.05. Therefore, stratification analysis was introduced to determine the independence of four-gene signature within the same tumor stage. Because of limited sample size of patients in stage I and IV (N=8 and 10), they were put together with stage II and III, respectively. For patients with stage I and II EAC, log-rank test showed that the four-gene signature could distinguish them with marginally significantly different survival (P=0.052, Figure 3A), and better predictive performance was observed for patients with stage III and IV EAC (P<0.001, Figure 3B). Thus, this four-gene signature might be able to help predict the survival of EAC patients independently.
Table 1
Clinical and pathological factors | Number of cases (%) |
---|---|
Age (years) | |
<60 | 26 (32.50) |
≥60 | 54 (67.50) |
Gender | |
Male | 69 (86.25) |
Female | 11 (13.75) |
Reflux history | |
Yes | 39 (48.75) |
No | 30 (37.50) |
Unavailable | 11 (13.75) |
Grade | |
G1+G2 | 29 (36.25) |
G3 | 25 (31.25) |
Unavailable | 26 (32.50) |
Tumor classification | |
T1+T2 | 30 (37.50) |
T3+T4 | 48 (60.00) |
Unavailable | 2 (2.50) |
Lymph node classification | |
N0 | 22 (27.50) |
N1+N2+N3 | 55 (68.75) |
Unavailable | 3 (3.75) |
Metastasis | |
M0 | 58 (72.50) |
M1 | 10 (12.50) |
Unavailable | 12 (15.00) |
Tumor stage | |
I+II | 35 (43.75) |
III+IV | 44 (55.00) |
Unavailable | 1 (1.25) |
Vital status | |
Alive | 41 (51.25) |
Dead | 39 (48.75) |
EAC, esophageal adenocarcinoma; TCGA, The Cancer Genome Atlas.
Table 2
Clinical and pathological factors | Univariate analysis | Multivariate analysis | |||
---|---|---|---|---|---|
HR (95% CI) | P value | HR (95% CI) | P value | ||
Age (<60, ≥60 years) | 0.708 (0.370–1.353) | 0.296 | |||
Gender (male, female) | 1.366 (0.476–3.922) | 0.562 | |||
Reflux history (no, yes) | 1.011 (0.468–2.181) | 0.978 | |||
Grade (G1+G2, G3) | 2.006 (0.957–4.206) | 0.065 | |||
Tumor classification (T1+T2, T3+T4) | 1.476 (0.731–2.980) | 0.277 | |||
Lymph node classification (N0, N1+N2+N3) | 3.413 (1.307–8.908) | 0.012 | 1.508 (0.479–4.745) | 0.482 | |
Metastasis (M0, M1) | 2.860 (1.273–6.423) | 0.011 | 1.335 (0.500–3.559) | 0.564 | |
Tumor stage (I+II, III+IV) | 2.786 (1.371–5.660) | 0.005 | 2.472 (0.923–6.621) | 0.072 | |
SRS group (low-risk, high-risk) | 4.374 (2.061–9.282) | <0.001 | 3.071 (1.341–7.033) | 0.008 |
HR, hazard ratio; CI, confidence interval; SRS, survival risk score.
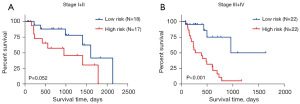
Functional enrichment analysis of the four-gene signature related gene sets
To explore the potential biological function of the four-gene signature, we screened protein-coding genes co-expressed with the four genes in the TCGA cohort. A total of 161 genes were identified to be co-expressed with at least one of the four genes (spearman correlation coefficient >0.50, P<0.05). GO analysis identified 29 significant biological process terms (P<0.05), and the results showed that these genes mainly clustered in cell proliferation related GO biological process, such as DNA replication, cell division, cell proliferation and G1/S transition of mitotic cell cycle (Figure 4A). Kyoto Encyclopedia of Genes and Genomes (KEGG) analysis returned 14 significant terms (P<0.05), of which the top two were DNA replication and cell cycle (Figure 4B). These results indicated that the four genes might be involved in the regulation of cancer cell development, thus affecting patients’ prognosis of OS.
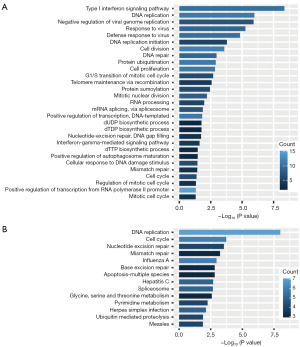
Discussion
In this study, we established a four-gene signature with good performance for prediction of OS in patients with EAC. Multivariate Cox regression analysis further indicated that its prognostic ability was independent of commonly used clinical features. Our work helps to identify patients with high risk of mortality who may need individualized therapeutic interventions.
Over the past couple of decades, substantial improvements of prognosis have been made in EAC, owing to several main reasons including earlier tumor diagnosis, better surgical and perioperative therapy and the application of neoadjuvant chemotherapy or chemoradiotherapy for locally advanced EAC (31-33). However, the above factors still contribute limitedly in improving prognosis with the current 5-year survival rate less than 20%. Achieving the goal of effective treatments remains challenging.
Tumor stage is the most important prognostic factor for EAC. Besides tumor stage, the tumor response to neoadjuvant chemotherapy or chemoradiotherapy is another important prognostic factor (34), which determines the tumor stage at the time of surgery. Other prognostic factors include patients’ performance status, comorbidities and quality of life (35). However, they are still inadequate for EAC prognosis prediction, and other kinds of prognostic factors are being under development.
Gene mutation and dysregulation are the intrinsic causes of cancer occurrence and progression (36), so the feasibility of gene as a prognostic marker cannot be ignored. Although some studies had been devoted to identifying genes associated with EAC prognosis, they mainly focused on known biomarkers in cancer, such as HER2 and EGFR (9,13). Considering the complexity of molecular context underlying EAC biology, there must be quite a few remaining genes worth mining. Undoubtedly, public high-throughput expression data provide us an excellent opportunity to find them. As previously mentioned, EAC patients are in urgent need of effective treatment. Another immense benefit for identifying such prognostic genes is that they might provide new therapy targets and help largely improve EAC treatment as well as patient survival. For EAC, molecular target therapy is now still at the early stage with limited options. Targeting HER2 has showed certain clinical efficacy in combination with chemotherapy, and more molecular targets and inhibitors are now being tested in clinical trials (37,38).
The bioinformatical analysis of EAC public data on prognosis is less advanced. A latest study identified RORA, KAT2B, CDC25B and ECT2 as the hub genes in esophageal cancer progression, which may participate the modulation of immune cell infiltration (39). However, they were identified through transcriptomic analysis of GEO data sets including ESCC, ECA and small cell carcinoma and are not specific biomarkers for EAC. Noticeably, we also hope that the prognostic genes would ideally account for the biology of EAC to add its potency to be developed as therapy targets. Therefore, the prognostic signature was constructed based on DEGs between cancer tissues and adjacent tissues. We ultimately got a signature consisting of four deregulated genes, namely ALAD, ABLIM3, IL17RB and IFI6, all of which were protective prognostic factors except for IL17RB. ALAD is an enzyme of delta-aminolevulinic acid dehydratase, which catalyzes the second step of heme synthesis (40). Recent studies reveal that ALAD is a favorable prognostic factor in patients with hepatocellular carcinoma (41), breast cancer (42) and clear cell renal cell carcinoma (43). Cell experiment shows that overexpression of ALAD could inhibit the proliferation and invasion of breast cancer cells by regulating transforming growth factor-beta (TGF-ꞵ) mediated epithelial-mesenchymal transition. ABLIM3, a member of the actin-binding LIM (abLIM) protein family, is rarely reported in cancer research, whereas its homologous protein ABLIM1, is considered to be a tumor suppressor in melanoma (44). IL-17RB is a member of the interleukin (IL)-17 receptor family, which can be activated by IL-17B and has been proved to be involved in inflammatory diseases and cancers (45). It has been reported that IL-17RB expression is significantly increased in gastric cancer tissues, indicating a poor prognosis of patients (46). In pancreatic cancer, overexpression of IL-17RB promotes cancer cell invasion via the ERK1/2 pathway and inversely correlates with progression-free survival (47). Two bioinformatical studies using TCGA data also identifies IL17RB as a poor prognosis factor of OS in EAC (48) and ESCA (49), which further corroborates the reliability of our study. IFI6 is a glycosylated protein induced by interferon and localizes at mitochondria. It exerts an anti-apoptotic function through inhibition of the depolarization of mitochondrial membrane potential and release of cytochrome c (50). In ESCC, IFI6 is involved in cell senescence, apoptosis as well as mitochondrial dysfunction (51,52). Moreover, the overexpression of IFI6 is reported to be correlated with poor clinical prognosis in ESCC. However, our study indicates that elevated IFI6 indicated a beneficial clinical outcome in EAC, suggesting a different role of IFI6 in ESCC and EAC. Generally, all these genes are far from being fully elucidated in EAC, and are worthy of future investigation on their functions in the biology process during EAC occurrence and development.
Functional assessment of co-expressed genes of the prognostic signature showed that cell proliferation related biological processes and pathways were significantly enriched, including DNA replication, cell cycle and cell division, which were closely associated with tumor growth. Interestingly, type I interferon signaling pathway was most significantly clustered among GO biological processes, and we found that genes in this term were all co-expressed with IFI6. Type I interferon signaling pathway plays an essential role in tumor immune surveillance, and mediates antitumor effects against several tumor types (53). It is also essential for the full-blown efficacy of anticancer agents, with its activation reported to predict clinical responses to chemotherapy in patients with breast cancer (54). These might explain why the upregulation of IFI6 constitutes a positive prognostic factor in EAC patients.
There are some limitations in the present study. First, the results generated here were derived from a single cohort, of which the included patients could not represent all patient populations in terms of genetic diversity. In addition, many other elements add the heterogeneity of a population, such as treatments and dietary habits. It is therefore necessary to validate the present results in external cohorts. Second, the population is relatively small, and clinical information of some patients, including tumor grade and metastasis status, is incomplete, which further decrease the sample number in the Cox proportional hazard regression analysis for relevant clinical factors.
Conclusions
In conclusion, the current study has firstly employed the public TCGA database and identified a four-gene signature with potential prognostic value for OS of patients with EAC. Our work is a supplement to the identification of molecular prognostic biomarkers in the field of EAC. Next, more work is necessary to validate its reliability and applicability in clinical practice.
Acknowledgments
Funding: This study was supported by grants from
Footnote
Reporting Checklist: The authors have completed the TRIPOD reporting checklist. Available at https://tcr.amegroups.com/article/view/10.21037/tcr-23-1798/rc
Peer Review File: Available at https://tcr.amegroups.com/article/view/10.21037/tcr-23-1798/prf
Conflicts of Interest: All authors have completed the ICMJE uniform disclosure form (available at https://tcr.amegroups.com/article/view/10.21037/tcr-23-1798/coif). The authors have no conflicts of interest to declare.
Ethical Statement: The authors are accountable for all aspects of the work in ensuring that questions related to the accuracy or integrity of any part of the work are appropriately investigated and resolved. The study was conducted in accordance with the Declaration of Helsinki (as revised in 2013).
Open Access Statement: This is an Open Access article distributed in accordance with the Creative Commons Attribution-NonCommercial-NoDerivs 4.0 International License (CC BY-NC-ND 4.0), which permits the non-commercial replication and distribution of the article with the strict proviso that no changes or edits are made and the original work is properly cited (including links to both the formal publication through the relevant DOI and the license). See: https://creativecommons.org/licenses/by-nc-nd/4.0/.
References
- Bray F, Ferlay J, Soerjomataram I, et al. Global cancer statistics 2018: GLOBOCAN estimates of incidence and mortality worldwide for 36 cancers in 185 countries. CA Cancer J Clin 2018;68:394-424. [Crossref] [PubMed]
- Bollschweiler E, Wolfgarten E, Gutschow C, et al. Demographic variations in the rising incidence of esophageal adenocarcinoma in white males. Cancer 2001;92:549-55. [Crossref] [PubMed]
- Vial M, Grande L, Pera M. Epidemiology of adenocarcinoma of the esophagus, gastric cardia, and upper gastric third. Recent Results Cancer Res 2010;182:1-17. [PubMed]
- Siegel RL, Miller KD, Jemal A. Cancer Statistics, 2017. CA Cancer J Clin 2017;67:7-30. [Crossref] [PubMed]
- Lagarde SM, ten Kate FJ, Reitsma JB, et al. Prognostic factors in adenocarcinoma of the esophagus or gastroesophageal junction. J Clin Oncol 2006;24:4347-55. [Crossref] [PubMed]
- Talsma K, van Hagen P, Grotenhuis BA, et al. Comparison of the 6th and 7th Editions of the UICC-AJCC TNM Classification for Esophageal Cancer. Ann Surg Oncol 2012;19:2142-8.
- Navin N, Kendall J, Troge J, et al. Tumour evolution inferred by single-cell sequencing. Nature 2011;472:90-4. [Crossref] [PubMed]
- Gerlinger M, Rowan AJ, Horswell S, et al. Intratumor heterogeneity and branched evolution revealed by multiregion sequencing. N Engl J Med 2012;366:883-92. [Crossref] [PubMed]
- Yoon HH, Shi Q, Sukov WR, et al. Adverse prognostic impact of intratumor heterogeneous HER2 gene amplification in patients with esophageal adenocarcinoma. J Clin Oncol 2012;30:3932-8. [Crossref] [PubMed]
- Dunne MR, Michielsen AJ, O'Sullivan KE, et al. HLA-DR expression in tumor epithelium is an independent prognostic indicator in esophageal adenocarcinoma patients. Cancer Immunol Immunother 2017;66:841-50. [Crossref] [PubMed]
- Goh XY, Rees JR, Paterson AL, et al. Integrative analysis of array-comparative genomic hybridisation and matched gene expression profiling data reveals novel genes with prognostic significance in oesophageal adenocarcinoma. Gut 2011;60:1317-26. [Crossref] [PubMed]
- Peters CJ, Rees JR, Hardwick RH, et al. A 4-gene signature predicts survival of patients with resected adenocarcinoma of the esophagus, junction, and gastric cardia. Gastroenterology 2010;139:1995-2004.e15. [Crossref] [PubMed]
- Ong CA, Shapiro J, Nason KS, et al. Three-gene immunohistochemical panel adds to clinical staging algorithms to predict prognosis for patients with esophageal adenocarcinoma. J Clin Oncol 2013;31:1576-82. [Crossref] [PubMed]
- Cui Y, Guo W, Li Y, et al. Pan-cancer analysis identifies ESM1 as a novel oncogene for esophageal cancer. Esophagus 2021;18:326-38. [Crossref] [PubMed]
- Zhao J, Guo C, Ma Z, et al. Identification of a novel gene expression signature associated with overall survival in patients with lung adenocarcinoma: A comprehensive analysis based on TCGA and GEO databases. Lung Cancer 2020;149:90-6. [Crossref] [PubMed]
- Zhang R, Gao X, Zuo J, et al. STMN1 upregulation mediates hepatocellular carcinoma and hepatic stellate cell crosstalk to aggravate cancer by triggering the MET pathway. Cancer Sci 2020;111:406-17. [Crossref] [PubMed]
- Cui K, Liu C, Li X, et al. Comprehensive characterization of the rRNA metabolism-related genes in human cancer. Oncogene 2020;39:786-800. [Crossref] [PubMed]
- Fan Q, Liu B. Identification of a RNA-Seq Based 8-Long Non-Coding RNA Signature Predicting Survival in Esophageal Cancer. Med Sci Monit 2016;22:5163-72. [Crossref] [PubMed]
- Zhang J, Ling X, Fang C, et al. Identification and validation of an eight-lncRNA signature that predicts prognosis in patients with esophageal squamous cell carcinoma. Cell Mol Biol Lett 2022;27:39. [Crossref] [PubMed]
- Chen F, Gong E, Ma J, et al. Prognostic score model based on six m6A-related autophagy genes for predicting survival in esophageal squamous cell carcinoma. J Clin Lab Anal 2022;36:e24507. [Crossref] [PubMed]
- Zhao F, Li Y, Dong Z, et al. Identification of A Risk Signature Based on Lactic Acid Metabolism-Related LncRNAs in Patients With Esophageal Squamous Cell Carcinoma. Front Cell Dev Biol 2022;10:845293. [Crossref] [PubMed]
- Sun K, Hong JJ, Chen DM, et al. Identification and validation of necroptosis-related prognostic gene signature and tumor immune microenvironment infiltration characterization in esophageal carcinoma. BMC Gastroenterol 2022;22:344. [Crossref] [PubMed]
- Liu Y, Hao H, Kang L, et al. Construction of a novel necroptosis-related lncRNA signature for prognosis prediction in esophageal cancer. BMC Gastroenterol 2022;22:345. [Crossref] [PubMed]
- Zhu J, Zhao Y, Wu G, et al. Ferroptosis-Related lncRNA Signature Correlates with the Prognosis, Tumor Microenvironment, and Therapeutic Sensitivity of Esophageal Squamous Cell Carcinoma. Oxid Med Cell Longev 2022;2022:7465880. [Crossref] [PubMed]
- Irizarry RA, Hobbs B, Collin F, et al. Exploration, normalization, and summaries of high density oligonucleotide array probe level data. Biostatistics 2003;4:249-64. [Crossref] [PubMed]
- Li HL, Wang CP, Zhang Y, et al. Long non-coding RNA PVT1 facilitates cell migration and invasion by regulating miR-148a-3p and ROCK1 in breast cancer. Clin Transl Oncol 2022;24:882-91. [Crossref] [PubMed]
- McCarthy DJ, Chen Y, Smyth GK. Differential expression analysis of multifactor RNA-Seq experiments with respect to biological variation. Nucleic Acids Res 2012;40:4288-97. [Crossref] [PubMed]
- Diboun I, Wernisch L, Orengo CA, et al. Microarray analysis after RNA amplification can detect pronounced differences in gene expression using limma. BMC Genomics 2006;7:252. [Crossref] [PubMed]
- Huang da W. Sherman BT, Lempicki RA. Systematic and integrative analysis of large gene lists using DAVID bioinformatics resources. Nat Protoc 2009;4:44-57. [Crossref] [PubMed]
- Xie C, Mao X, Huang J, et al. KOBAS 2.0: a web server for annotation and identification of enriched pathways and diseases. Nucleic Acids Res 2011;39:W316-22. [Crossref] [PubMed]
- Dubecz A, Gall I, Solymosi N, et al. Temporal trends in long-term survival and cure rates in esophageal cancer: a SEER database analysis. J Thorac Oncol 2012;7:443-7. [Crossref] [PubMed]
- Njei B, McCarty TR, Birk JW. Trends in esophageal cancer survival in United States adults from 1973 to 2009: A SEER database analysis. J Gastroenterol Hepatol 2016;31:1141-6. [Crossref] [PubMed]
- van Hagen P, Hulshof MC, van Lanschot JJ, et al. Preoperative chemoradiotherapy for esophageal or junctional cancer. N Engl J Med 2012;366:2074-84. [Crossref] [PubMed]
- Davies AR, Gossage JA, Zylstra J, et al. Tumor stage after neoadjuvant chemotherapy determines survival after surgery for adenocarcinoma of the esophagus and esophagogastric junction. J Clin Oncol 2014;32:2983-90. [Crossref] [PubMed]
- Gavin AT, Francisci S, Foschi R, et al. Oesophageal cancer survival in Europe: a EUROCARE-4 study. Cancer Epidemiol 2012;36:505-12. [Crossref] [PubMed]
- Bhan A, Soleimani M, Mandal SS. Long Noncoding RNA and Cancer: A New Paradigm. Cancer Res 2017;77:3965-81. [Crossref] [PubMed]
- Zhang X, Wang Y, Meng L. Comparative genomic analysis of esophageal squamous cell carcinoma and adenocarcinoma: New opportunities towards molecularly targeted therapy. Acta Pharm Sin B 2022;12:1054-67. [Crossref] [PubMed]
- Ren DY, Yuan XR, Tu CX, et al. Long Noncoding RNA 00472: A Novel Biomarker in Human Diseases. Front Pharmacol 2021;12:726908. [Crossref] [PubMed]
- Chatterjee D, Rahman MM, Saha AK, et al. Transcriptomic analysis of esophageal cancer reveals hub genes and networks involved in cancer progression. Comput Biol Med 2023;159:106944. [Crossref] [PubMed]
- Rajaraman P, Schwartz BS, Rothman N, et al. Delta-aminolevulinic acid dehydratase polymorphism and risk of brain tumors in adults. Environ Health Perspect 2005;113:1209-11. [Crossref] [PubMed]
- Ye Q, Yang X, Zheng S, et al. Low expression of moonlight gene ALAD is correlated with poor prognosis in hepatocellular carcinoma. Gene 2022;825:146437. [Crossref] [PubMed]
- Ge J, Yu Y, Xin F, et al. Downregulation of delta-aminolevulinate dehydratase is associated with poor prognosis in patients with breast cancer. Cancer Sci 2017;108:604-11. [Crossref] [PubMed]
- Wu Y, Wei X, Feng H, et al. An eleven metabolic gene signature-based prognostic model for clear cell renal cell carcinoma. Aging (Albany NY) 2020;12:23165-86. [Crossref] [PubMed]
- Kwong LN, Chin L. Chromosome 10, frequently lost in human melanoma, encodes multiple tumor-suppressive functions. Cancer Res 2014;74:1814-21. [Crossref] [PubMed]
- Ren L, Xu Y, Liu C, et al. IL-17RB enhances thyroid cancer cell invasion and metastasis via ERK1/2 pathway-mediated MMP-9 expression. Mol Immunol 2017;90:126-35. [Crossref] [PubMed]
- Bie Q, Sun C, Gong A, et al. Non-tumor tissue derived interleukin-17B activates IL-17RB/AKT/β-catenin pathway to enhance the stemness of gastric cancer. Sci Rep 2016;6:25447. [Crossref] [PubMed]
- Wu HH, Hwang-Verslues WW, Lee WH, et al. Targeting IL-17B-IL-17RB signaling with an anti-IL-17RB antibody blocks pancreatic cancer metastasis by silencing multiple chemokines. J Exp Med 2015;212:333-49. [Crossref] [PubMed]
- Zhang X, Yang L, Kong M, et al. Development of a prognostic signature of patients with esophagus adenocarcinoma by using immune-related genes. BMC Bioinformatics 2021;22:536. [Crossref] [PubMed]
- Tang K, Cheng Y, Li Q. Construction and Verification of a Hypoxia-Stemness-Based Gene Signature for Risk Stratification in Esophageal Cancer. Med Sci Monit 2021;27:e934359. [Crossref] [PubMed]
- Tahara E Jr, Tahara H, Kanno M, et al. G1P3, an interferon inducible gene 6-16, is expressed in gastric cancers and inhibits mitochondrial-mediated apoptosis in gastric cancer cell line TMK-1 cell. Cancer Immunol Immunother 2005;54:729-40. [Crossref] [PubMed]
- Gao Y, Dai J, Xu XP, et al. Role of interferon alpha-inducible protein 6 in modulating the proliferation, apoptosis and senescence of oesophageal squamous cell carcinoma cells. J Physiol Pharmacol 2023; [PubMed]
- Liu Z, Gu S, Lu T, et al. IFI6 depletion inhibits esophageal squamous cell carcinoma progression through reactive oxygen species accumulation via mitochondrial dysfunction and endoplasmic reticulum stress. J Exp Clin Cancer Res 2020;39:144. [Crossref] [PubMed]
- Zitvogel L, Galluzzi L, Kepp O, et al. Type I interferons in anticancer immunity. Nat Rev Immunol 2015;15:405-14. [Crossref] [PubMed]
- Legrier ME, Bièche I, Gaston J, et al. Activation of IFN/STAT1 signalling predicts response to chemotherapy in oestrogen receptor-negative breast cancer. Br J Cancer 2016;114:177-87. [Crossref] [PubMed]