Machine learning methods predict recurrence of pN3b gastric cancer after radical resection
Highlight box
Key findings
• Machine leaning method predicts postoperative recurrence of pN3b gastric cancer.
What is known and what is new?
• pN3b gastric cancer has a high mortality rate and a high recurrence rate.
• In view of the high recurrence rate of pN3b gastric cancer, different machine leaning models were used to predict it.
What is the implication, and what should change now?
• Different models are needed for prediction in patients with different characteristics. In addition, postoperative follow-up strategies may need to be adjusted for pN3b gastric cancer.
Introduction
According to the incomplete statistics of GLOBOCAN in 2020, gastric cancer (GC) is the fifth most common cancer worldwide and the fourth most common leading cause of cancer-related death, with approximately 760,000 deaths each year (1). Adequate surgery is the cornerstone of GC treatment and one of the most important GC treatment strategies (2). However, the 5-year survival rate in GC patients after surgery remains poor, at less than 40% (2,3). Recurrence is one of the main reasons for the poor prognosis of GC patients after surgery (4). Approximately 40–50% of GC patients are reported to have recurrence after surgery, including local recurrence, distant recurrence and peritoneal recurrence (2,5,6). However, there is no standardized treatment and follow-up strategy for recurrent GC (5,7). Therefore, assessing patients’ risk of recurrence may be relevant to clinical practice and help in selecting effective treatment modalities as well as enhancing follow-up strategies.
The pN3 staging includes at least seven positive metastatic lymph nodes, which were further divided into pN3a (7–15 positive lymph nodes) and pN3b (at least 16 positive lymph nodes). Some studies have shown that pN3b disease is associated with a worse prognosis than pN3a disease (8,9), with a 5-year survival rate of less than 20% (10). More importantly, the American Joint Committee on Cancer (AJCC) 8th edition states that the comprehensive staging of pN3b GC is ultimately classified as stage III regardless of the pT1–pT4 stage (11). This suggests that pN3b GC is characterized by extensive lymph node metastasis and an extremely heavy tumor burden. However, pN3b GC accounts for a relatively low percentage, only 4.7–14.8% of GC patients (12-15), and most prognostic studies on pN3b GC have focused on overall survival and less on recurrence rates (8-10,12-15). Nevertheless, some small sample studies have found that postoperative recurrence rates are higher than 60% for pN3b disease, but there are significant differences in pN3b GC recurrence patterns (16-19). These results suggest that pN3b GC may be highly heterogeneous and that the recurrence pattern of pN3b GC still needs to be analyzed. Furthermore, the prognosis of patients with pN3b disease may be improved if recurrence can be effectively predicted, and a series of follow-ups and treatments can be developed to address recurrence.
Identifying long-term patient survival and recurrence requires accurate and robust predictive models. Machine learning (ML) can be used to discover and identify relationships between variables and outcomes from complex datasets to efficiently predict clinical outcomes for cancer patients, including death, recurrence, and adverse effects of chemotherapy (20,21). ML can significantly (15–25%) improve the accuracy of predicting death and recurrence in cancer patients (22). In GC, ML prediction models constructed based on patients’ clinicopathological characteristics can effectively predict patient recurrence (23). However, GC is a highly heterogeneous gastrointestinal malignancy, the predictive performance of ML models in different studies remains variable (23,24), and there are fewer studies on pN3b GC recurrence. Therefore, the prediction of pN3b GC recurrence by ML still deserves further study.
This study retrospectively analyzed pN3b patients who underwent radical GC surgery in the Department of Gastrointestinal Surgery of Harbin Medical University Cancer Hospital from January 2011 to December 2016. The recurrence pattern was analyzed and an ML predictive model was built. Finally, the postoperative recurrence of pN3b patients was accurately predicted based on ML. We present this article in accordance with the STARD reporting checklist (available at https://tcr.amegroups.com/article/view/10.21037/tcr-23-1367/rc).
Methods
Patients
This study retrospectively and continuously analyzed pN3b GC patients who underwent radical surgery at the Department of Gastrointestinal Surgery of Harbin Medical University Cancer Hospital from January 2011 to December 2016. The diagnosis of GC was based on tissue samples obtained by preoperative gastroscopy and was further confirmed postoperatively by a specialized pathologist through examination of pathological tissue. After admission to the Department of Gastroenterology, the patient underwent routine preoperative investigations, which included abdominal computed tomography (CT), chest CT, cardiac ultrasound, ultrasound of both supraclavicular lymph nodes, electrocardiography, gastroscopy, and routine hematology and tumor marker assessments. The surgical methods and postoperative chemotherapy standards were in accordance with the Japanese GC Treatment Guidelines (Fifth Edition) (25). The study was conducted in accordance with the Declaration of Helsinki (as revised in 2013). This research was approved by the ethics committee of Harbin Medical University Cancer Hospital, Harbin, Heilongjiang Province, China (decision number: HMUCH2010051). All patients have signed an informed consent form before surgery.
Datasets
All study participants or their legal guardians provided written informed consent for personal and medical data collection prior to study enrollment. The clinicopathological data of the patients were saved in the GC information management system v1.2 of the Harbin Medical University Cancer Hospital (HMU, copyright number 2013SR087424, http://www.sgihmu.com), including sex, age, smoking history, drinking history, tumor markers, tumor location, tumor size, pathology (pTNM) stage, carcinoembryonic antigen (CEA), and carbohydrate antigen 19-9 (CA19-9). The exclusion criteria were as follows: (I) preoperative chemotherapy; (II) preoperative radiotherapy; (III) other systemic malignancies; and (IV) multiple recurrences (Figure 1).
Follow-up and recurrence
All patients were discharged from the hospital for regular follow-up examinations by telephone, e-mail or examination at the outpatient complex of the Harbin Medical University Cancer Hospital that included hematological analysis, tumor markers, gastroscopy, abdominal ultrasonography, abdominal CT, and positron emission tomography (PET) CT for some patients according to their conditions. Stage I patients were followed up every 12 months, stage II patients were followed up every 6 months, and stage III patients were followed up every 3–6 months.
Chest or abdominal CT was performed for suspected tumor recurrence or elevated tumor marker levels above pathological levels, and a bone scan was performed for suspected bone metastases. The diagnosis of recurrence was confirmed on the basis of imaging or resurgical pathology. Considering the initial site of recurrence, the types of recurrence were classified as locoregional recurrence, distant recurrence and peritoneal recurrence. Locoregional recurrence was defined as recurrence in the gastric bed, anastomosis, regional lymph nodes (para-aortic lymph nodes), or direct spread of adjacent structures. Distant recurrence was defined as the involvement of specific organs through systemic metastases, and lesions in extra-abdominal lymph nodes were considered distant metastases. Peritoneal recurrence was defined as positive ascites cells, peritoneal nodules, tumors invading the ovaries (Krukenberg’s tumor), and plasma membrane deposited in the abdominal organs (5,7,16). To ensure the accuracy of the study, we only analyzed the initial recurrence pattern of patients, and for survival and loss to follow-up, patients who could not provide a specific site of recurrence were grouped as non-recurrence or unknown. In the end, a total of 205 patients experienced recurrence during follow-up.
ML
In this study, logistic regression, random forest, extreme gradient boosting (XGBoost), support vector machine (SVM), multilayer perceptron (MLP) and Gaussian naive Bayes (GNB) were used as ML methods to predict pN3b GC recurrence. Logistic regression is a generalized linear regression analysis model commonly used to predict outcomes with only two values, and logistic regression can be used to obtain the weights of independent variables to understand the risk factors for outcomes and make accurate predictions (26). Random forest is a classifier that uses multiple trees to train and predict samples and is a classifier that contains multiple decision trees; the final classification result is determined by each decision tree vote. Since the data and variables are different for each tree, random forests can be used for large datasets to produce unbiased estimates of the error after internal generalization, allowing for a high success rate (27). XGBoost is an optimized distributed gradient enhancement library structured for classification and regression prediction modeling problems to solve data problems quickly and accurately (28). SVM is a class of generalized linear classifiers that perform binary classification of data in a supervised learning fashion, with a decision boundary that is a maximum-margin hyperplane solved for the learned samples (29). An MLP is a neural network consisting of fully connected layers containing at least one hidden layer, and the output of each hidden layer is transformed by an activation function (30). GNB is a classification algorithm that directly measures the probability between labels and features and is mostly used to deal with continuous values, which can substantially improve the accuracy of classifiers (31).
K-fold cross-validation
fold cross-validation is a technique used to evaluate the performance of ML models in a robust manner. In k-fold cross-validation, the original sample is systematically partitioned into k subsets of equal size, one subset is reserved as validation data for testing the model, and the remaining k − 1 subset is used as training data. The cross-validation process is then repeated k times, measuring performance as the average of all test machines. The advantage of this approach is that all observations are used for training and validation, and each observation is used only once for validation. In this study, we applied 3-fold cross-validation to all ML methods, dividing the total sample into training and testing sets in a 2:1 ratio to assess prediction error.
Statistical analysis
Principal component analysis (PCA) is a multivariate analysis technique used to identify correlated patterns within variables. During PCA calculations, an effort is made to minimize the loss of information while also preserving possible variations in a given dataset. The original dimension (input variable) is converted to a new coordinate system where the new axis (orthogonal principal components) contains the maximum variance.
In the PCA process, data scaling is first performed by a standardized covariance matrix so that the variable has a unit variance. The matrix is decomposed by eigenvalues to obtain its eigenvectors and corresponding eigenvalues. Eigenvectors refer to the contribution of the original dimension to the principal component, and eigenvalues refer to the amount of variance captured by the principal component (or correlation due to normalized input structure). Finally, the feature vector is used as the weighting coefficient to transform the original dataset to obtain the main component score.
The dataset considered in this study was the HMU dataset, which contained 11 independent variables, namely, sex, age, smoking history, alcohol history, CEA, CA19-9, tumor location, Borrmann type, tumor size, histological type, and pT stage. First, the Kaiser-Meyer-Olkin (KMO) test and the Bartlett sphericity test were used to determine the data suitable for PCA; if the KMO >0.6, the variables were related and in line with the PCA. The data were considered to be significant when P<0.05 was obtained by the Bartlett sphericity test; then PCA could be performed. After PCA, a linear combination of the characteristics of the new variable was constructed. To ensure a cumulative contribution of 90%, we ended up using PCA to construct 9 new variable features, with a final cumulative variance interpretation rate of 90.71% (Figure 2). After determining the linear combination of the new variables, we used a grid search to pick the optimal hyperparameters of the model, find the combination of the best hyperparameters, and improve the predictive performance of the model.
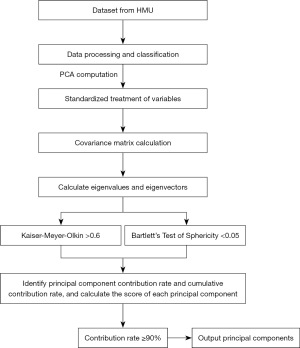
Data processing
Data processing involved SPSS 25.0. Chi-squared test was used to analyze the relationship between recurrence and patient characteristics, and P<0.05 was considered to be statistically significant. SPSS was used to standardize the data and analyze the PCA characteristics. Python 3.9.10 was used to analyze the ML algorithms and divide the dataset into training and testing sets according to a ratio of 2:1. In addition, after determining the PCA characteristics, we used a grid search to select the optimal hyperparameters of the model and find the optimal combination of hyperparameters to obtain the optimal model for this purpose. Finally, model performance was evaluated using accuracy versus receiver operating characteristic (ROC) curves. ROC was used to calculate the area under the curve (AUC).
Results
Patient recurrence
Finally, a total of 336 patients were included in this study, of which 205 (61.0%) had recurrence. The baseline characteristics of the patient are shown in Table 1. There were 69 (33.7%), 83 (40.5%), and 53 (25.9%) patients with locoregional recurrence, distant recurrence, and peritoneal recurrence. The proportion of tumors located in the upper third of the stomach (P=0.018), the proportion of pT4 stage (P<0.001), and the proportion of imprinted ring cell carcinoma (P<0.001) in recurrence patients were higher than that of non-recurrent patients. In addition, the proportion of patients with recurrence at less than 60 years, although higher than that of non-recurrence patients (60.5% vs. 50.4%, P=0.068), but not statistically significant.
Table 1
Variable | Total, n (%) | Recurrence, n (%) | P value | |
---|---|---|---|---|
Yes | No/unknown | |||
Number | 336 | 205 (61.0) | 131 (39.0) | – |
Sex | 0.054 | |||
Male | 250 (74.4) | 145 (70.7) | 105 (80.2) | |
Female | 86 (25.6) | 60 (29.3) | 26 (19.8) | |
Age (years) | 0.068 | |||
≤60 | 190 (56.5) | 124 (60.5) | 66 (50.4) | |
>60 | 146 (43.5) | 81 (39.5) | 65 (49.6) | |
Smoking history | 0.175 | |||
No | 164 (48.8) | 94 (45.9) | 70 (53.4) | |
Yes | 172 (51.2) | 111 (54.1) | 61 (46.6) | |
Drinking history | 0.871 | |||
No | 207 (61.6) | 127 (62.0) | 80 (61.1) | |
Yes | 129 (38.4) | 78 (38.0) | 51 (38.9) | |
CEA (ng/mL) | 0.348 | |||
≤5 | 247 (73.5) | 147 (71.7) | 100 (76.3) | |
>5 | 89 (26.5) | 58 (28.3) | 31 (23.7) | |
CA19-9 (U/mL) | 0.993 | |||
≤37 | 245 (72.9) | 149 (72.7) | 96 (73.3) | |
>37 | 91 (27.1) | 56 (27.3) | 35 (26.7) | |
Tumor location | 0.018 | |||
Upper third | 34 (10.1) | 27 (13.2) | 7 (5.3) | |
Middle third | 68 (20.2) | 40 (19.5) | 28 (21.4) | |
Lower third | 213 (63.4) | 121 (59.0) | 92 (70.2) | |
Total stomach | 21 (6.3) | 17 (8.3) | 4 (3.1) | |
Tumor size (mm) | 0.333 | |||
≤50 | 84 (25.0) | 55 (26.8) | 29 (22.1) | |
>50 | 252 (75.0) | 150 (73.2) | 102 (77.9) | |
Borrmann type | 0.064 | |||
0–II | 53 (15.8) | 39 (19.0) | 14 (10.7) | |
III | 195 (58.0) | 110 (53.7) | 85 (64.9) | |
IV | 88 (26.2) | 56 (27.3) | 32 (24.4) | |
pT stage | <0.001 | |||
pT1–pT2 | 7 (2.1) | 2 (1.0) | 5 (3.8) | |
pT3 | 132 (39.3) | 64 (31.2) | 68 (51.9) | |
pT4 | 197 (58.6) | 139 (67.8) | 58 (44.3) | |
Histological type | <0.001 | |||
Well-moderately differentiated | 77 (22.9) | 42 (20.5) | 35 (26.7) | |
Poorly-undifferentiated | 162 (48.2) | 97 (47.3) | 65 (49.6) | |
Signet ring cell carcinoma | 85 (25.3) | 64 (31.2) | 21 (16.0) | |
Others | 12 (3.6) | 2 (1.0) | 10 (7.6) | |
Categories of recurrences | ||||
Locoregional | – | 69 (33.7) | – | |
Distant | – | 83 (40.5) | – | |
Peritoneal | – | 53 (25.9) | – |
CEA, carcinoembryonic antigen; CA19-9, carbohydrate antigen 19-9; pT, pathology stage.
According to the random forest and XGBoost (Figure 3), for overall recurrence, the first four important features were pT stage, histological type, Borrmann type, tumor location. For locoregional recurrence, the first four important features were smoking history, pT stage, histological type, Borrmann type. For distant recurrence, the first four important features were smoking history, histologic type, Borrmann type, tumor location. For peritoneal recurrence, the first four important features were pT stage, histological type, Borrmann type, age.
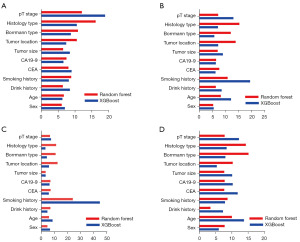
PCA
In order to perform PCA processing, first, determine whether the data was suitable for PCA. The KMO test value was 0.615, and the P value obtained by the Bartlett sphericity test showed that the HMU dataset was very suitable for PCA. After that, the cumulative variance interpretation rate was used to determine the number of PCAs, and when the number of PCAs was 9, the cumulative variance interpretation rate was 90.71%. Therefore, nine PCA features were finally used for model analysis.
ML models
After training the model, we tested the model. The test results showed (Figures 4,5) that for the overall recurrence, the accuracy rates for SVM, XGBoost, random forest, MLP, logistic, and GNB were 81.7%, 84.1%, 80.5%, 79.3%, 68.3%, and 63.4%; the AUCs were 0.879, 0.924, 0.927, 0.894, 0.782 and 0.781, respectively. For locoregional recurrence, the accuracy rates for SVM, XGBoost, random forest, MLP, logistic, and GNB were 80.4%, 84.1%, 89.7%, 71.0%, 64.5%, and 54.2%; the AUCs were 0.836, 0.913, 0.940, 0.783, 0.716 and 0.734, respectively. For distant recurrence, the accuracy rates for SVM, XGBoost, random forest, MLP, logistic, and GNB were 81.4%, 81.4%, 84.3%, 70.6%, 62.7%, and 66.7%; the AUCs were 0.867, 0.866, 0.896, 0.755, 0.704 and 0.716, respectively. For peritoneal recurrence, the accuracy rates for SVM, XGBoost, random forest, MLP, logistic, and GNB were 82.5%, 86.0%, 83.3%, 78.9%, 67.5%, and 64.4%; The AUCs were 0.907, 0.920, 0.923, 0.857, 0.703 and 0.707, respectively.
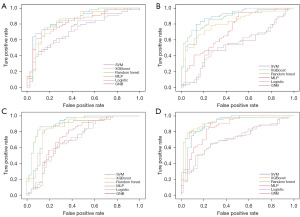
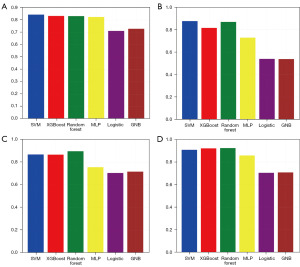
Discussion
This study attempts to systematically identify, summarize, and distinguish the substantial heterogeneity in clinical manifestations for contribute to the understanding of the disease process of pN3b GC and provide specific phenotypic insights related to long-term disease processes. Preoperative prediction of pN3b GC recurrence is important for clinical practice and can help screen patients with high-risk recurrence, develop intensive follow-up strategies, and improve the prognosis of pN3b GC patients. In this study, the postoperative recurrence of pN3b GC was predicted for the first time based on ML algorithms. In addition, we found that the importance of different patient characteristics differed significantly for different recurrence patterns after surgery in pN3b GC patients, further revealing the disease process and heterogeneity of pN3b GC.
In clinical setting, gastrointestinal surgeons assess the risk of postoperative recurrence primarily based on pTNM staging. However, GC is a highly heterogeneous gastrointestinal malignancy, and patient-related features such as age, lifestyle habits, tumor location, histological type, and other factors also play an important role in tumor recurrence. While traditional statistical predictive models developed based on multivariate risk factors can assess the probability of occurrence of outcome events, they are limited by the need for priori hypotheses about potential associations despite the considerable efforts that have been made. However, it is often necessary to exclude potentially useful variables when building models, and the same model cannot contain highly collinear covariates; there are often complex nonlinear relationships among different factors, which make the linear relationship of finite variables in statistical models often insufficient for the prediction tasks. Therefore, we predict recurrence in pN3b GC patients based on the powerful prediction ability of ML and multidimensional analysis.
Since pN3b GC has already exhibited a large number of lymph node metastases, there is a heavy lymphatic burden, which makes it easier for tumors to spread through the lymphatic system. We found significant differences in the importance of different features of different recurrence patterns, suggesting that pN3b GC may be highly heterogeneous. Obviously, in the context of lymph node metastasis, the influence of important features on recurrence patterns indirectly contributes to the heterogeneity of clinical outcomes in patients with pN3b GC and makes it possible for clinicians to predict recurrence through important features. Therefore, focusing on important features may have additional importance in pN3b GC; it may help improve clinicians’ postoperative decision-making and develop additional follow-up plans and targeted treatments based on possible recurrence patterns. However, we may not be able to explain all the huge heterogeneity on relapse through current clinical studies. Therefore, our research focus is not on the root cause of recurrence heterogeneity but on distinguishing some patients at high risk of postoperative recurrence through existing clinical information, thereby ultimately meeting clinical utility.
Given gastric anatomical factors including the presence of abundant lymphatic vessels in the stomach wall and perigastric area, GC cells can metastasize through the lymphatic system, so the recurrence of GC is closely related to lymphatic generation (32). We found that smoking history is one of the important factors influencing regional recurrence and distant recurrence and that nicotine components in tobacco can significantly induce the expression of vascular endothelial growth factor (VEGF) and cyclooxygenase 2 (COX-2), thereby promoting angiogenesis (33). In addition, smoking promotes DNA methylation in the cadherin-1 (CDH 1) promoter region of the E-cadherin gene and decreases E-cadherin levels, and the deletion of E-cadherin is also one of the typical manifestations of epithelial mesenchymal transformation (EMT), which enhances the migration ability of GC cells (34). Ultimately, the deletion of E-cadherin promotes GC liver metastasis, lung metastasis, bone metastasis, etc. (35-37). In addition, histological type, tumor location, and Borrmann type are the more important influencing factors affecting regional recurrence and distant recurrence, and one of the common features of these factors is the impact on lymph node metastasis. For example, in terms of lymph node metastasis frequency, poorly differentiated adenocarcinoma ranks higher than high/medium differentiated adenocarcinoma; the tumor located in the upper third of the stomach ranks higher than that in the lower third of the stomach; and Borrmann type III is higher than Borrmann type I/II (38-40). Therefore, with current information, we speculate that tumor-related factors that increase the burden on lymph nodes cause recurrence by increasing metastasis pathways or giving cancer cells the ability to migrate. Clearly, the status of the lymph nodes is critical for pN3b GC recurrence. Even with R0 D2/D2+ dissection, additional postoperative treatment and intensive follow-up are particularly important. We recommend smoking, poorly differentiated adenocarcinoma, and pN3b GC patients with tumors located in the upper third of the stomach, postoperatively, extra attention should be given to locoregional recurrence and distant recurrence. In addition to abdominal contrast-enhanced CT examination, some patients may need chest X-ray, chest CT, PET/CT, bone marrow aspiration biopsy, etc., to detect lung metastases, bone metastases, etc., early. This facilitates early intervention as well as the selection of specific treatment options, ultimately meeting clinical needs.
For peritoneal recurrence, the importance analysis showed that histological type, Borrmann type, and age were the most important factors. Previous study has shown that Borrmann type IV accounts for 8% to 13% of GC cases (41) and is strongly associated with peritoneal recurrence, whereas 24.3% of patients in our study were diagnosed with Borrmann type IV, possibly related to our inclusion of pN3b GC only. Borrmann type IV is highly aggressive and closely related to lymph node metastasis; Chen et al. found that pN3b disease is an independent prognostic factor for Borrmann type IV peritoneal metastasis (41), which fully indicates that Borrmann type IV pN3b GC patients are a high-risk group for peritoneal recurrence. With regard to histologic types, in addition to considering the frequency of persistent metastasis, the specific biological behavior of some histologic types would further differentiate people with peritoneal recurrence. Signet ring cell carcinoma (SRCC) is a specific GC subtype characterized by a mucus filling of cells that pushes the nucleus to one side to have a ring-like appearance, and SRCC is highly aggressive and has a high risk of lymph node metastasis. Furthermore, SRCC may be more likely to spread through neurospatial areas with low resistance and further into the extragastric nerve, which may be a source of peritoneal recurrence (42,43). We found that age is one of the important factors influencing peritoneal recurrence and is inversely correlated with peritoneal recurrence; this means that younger patients are more likely to develop peritoneal recurrence after surgery, consistent with previous study (44). Clearly, peritoneal recurrence requires age-related follow-up planning. At the same time, the studies found that peritoneal recurrence was more aggressive than distant recurrence (45,46), further suggesting that the highly aggressive nature of young, Borrmann IV, SRCC may increase the risk of peritoneal recurrence in patients. Therefore, we suggest that in young, Borrmann IV, SRCC pN3b patients, close attention must be given to peritoneal recurrence after surgery, focusing on abdominal CT with contrast and performing laparoscopic peritoneal biopsy if necessary to facilitate early detection of peritoneal recurrence. Meanwhile, neoadjuvant chemotherapy and prophylactic intraperitoneal chemotherapy have become important treatment measures to improve the prognosis of patients with peritoneal recurrence.
Unlike previous study, multiple independent factors for patient outcomes statistically represent individual patient phenotypes (16). However, we found notable differences in the importance of features, and given that different clinical variables contribute differently to the main components, we emphasized the interaction among variables, which is often overlooked in traditional statistical models and is critical for understanding patient phenotypes. PCA uses dimensionality reduction analysis as the analysis method, can map high-dimensional data into low-dimensional space through a linear projection, and is designed for the data in the projected dimension to have the largest amount of information (maximum variance) while using fewer data dimensions and retaining more characteristics of the original data. Similar study often yields insights through the application of PCA to whole-genome sequencing to classify tumor outcomes according to the different contributions of different combinations of genetic abnormalities to pathological phenotypes (47). We performed a PCA test on the data, and the results showed that the KMO test value was 0.615, and P<0.05 was obtained by the Bartlett sphericity test, so the study data were very suitable for PCA. After constructing new features, we used six ML models to predict recurrence. The results showed that there were significant differences in the prediction accuracy and AUC of different models, which further suggested the high heterogeneity of pN3b GC. Therefore, an important challenge in supplemental anatomical pTNM staging prediction is the integration of clinical parameters, and our study shows that different ML algorithms exhibit strong efficacy in predicting postoperative recurrence of pN3b GC, indicating good clinical application prospects in future treatment and follow-up management.
At the same time, there are some limitations in this study that must be acknowledged. First, as a retrospective single-center study, it yields results that need to be verified. Second, not all patients completed the follow-up plan developed by the surgeon, and some patients were lost to follow-up and could not provide detailed recurrence information; this is one of the disadvantages of retrospective recurrence studies. Therefore, we will be committed to conducting forward-looking, multicenter and detailed research in the future. In addition, we excluded cases of multi-site recurrence, primarily due to the high heterogeneity of such tumors. Additionally, given the retrospective nature of the study, we were unable to determine the chronological sequence of occurrences in multifocal tumors. Therefore, prospective studies are needed in the future to further investigate this matter. Finally, 11 factors may be quite small for an ML model. Some molecular characteristics of patients, such as gene mutations, transcriptome sequencing, DNA methylation, etc., were not included. Therefore, given that feature selection in ML is an ongoing process, it is necessary to incorporate more features in the future to improve the model. It is clear that the prediction of recurrence by integrating molecular features through PCA is still worth further exploration.
Despite these limitations, the current study has important implications for clinical practice and policy-making. Surgeons, as decision-makers in interventions, need to look for clinical cues and intervene for relapse outcomes. We found a high degree of heterogeneity in pN3b GC, and more importantly, the comprehensive stage of all pN3b GC patients was stage III. Therefore, it is not sufficient to predict pN3b GC recurrence by pTNM staging alone. The study also provides surgeons with clear evidence that some common patient characteristics, such as age, histological type, Borrmann type, and smoking history, play a key role in predicting pN3b GC recurrence. In general, patients are advised to have check-ups every 6 months for 2 years after surgery (48). However, personalized predictions through ML can help diagnose recurrence early and provide a chance for a cure. We also recommend that patients at high risk of pN3b GC recurrence would undergo checking every 3 months after surgery, which can facilitate further development of relevant treatment strategies. In conclusion, clinical factors may be as effective as TNM staging systems when deciding on cancer treatment, and subgroup analyses with the same defining characteristics may play an equally important role in clinical research on cancer.
Conclusions
pN3b GC is highly heterogeneous, and ML can personalize prediction of recurrence patterns and reduce prediction bias due to heterogeneity. This facilitates accurate and personalized treatment and follow-up strategies for GC patients in a clinical setting.
Acknowledgments
We would like to thank AJE for their help in polishing our paper.
Funding: This work was supported by
Footnote
Reporting Checklist: The authors have completed the STARD reporting checklist. Available at https://tcr.amegroups.com/article/view/10.21037/tcr-23-1367/rc
Data Sharing Statement: Available at https://tcr.amegroups.com/article/view/10.21037/tcr-23-1367/dss
Peer Review File: Available at https://tcr.amegroups.com/article/view/10.21037/tcr-23-1367/prf
Conflicts of Interest: All authors have completed the ICMJE uniform disclosure form (available at https://tcr.amegroups.com/article/view/10.21037/tcr-23-1367/coif). The authors have no conflicts of interest to declare.
Ethical Statement: The authors are accountable for all aspects of the work in ensuring that questions related to the accuracy or integrity of any part of the work are appropriately investigated and resolved. The study was conducted in accordance with the Declaration of Helsinki (as revised in 2013). This research was approved by the ethics committee of Harbin Medical University Cancer Hospital, Harbin, Heilongjiang Province, China (decision number: HMUCH2010051). All patients have signed an informed consent form before surgery.
Open Access Statement: This is an Open Access article distributed in accordance with the Creative Commons Attribution-NonCommercial-NoDerivs 4.0 International License (CC BY-NC-ND 4.0), which permits the non-commercial replication and distribution of the article with the strict proviso that no changes or edits are made and the original work is properly cited (including links to both the formal publication through the relevant DOI and the license). See: https://creativecommons.org/licenses/by-nc-nd/4.0/.
References
- Sung H, Ferlay J, Siegel RL, et al. Global Cancer Statistics 2020: GLOBOCAN Estimates of Incidence and Mortality Worldwide for 36 Cancers in 185 Countries. CA Cancer J Clin 2021;71:209-49. [Crossref] [PubMed]
- Songun I, Putter H, Kranenbarg EM, et al. Surgical treatment of gastric cancer: 15-year follow-up results of the randomised nationwide Dutch D1D2 trial. Lancet Oncol 2010;11:439-49. [Crossref] [PubMed]
- Maharjan U, Kauppila JH. Survival trends in gastric cancer patients between 1987 and 2016: a population-based cohort study in Finland. Gastric Cancer 2022;25:989-1001. [Crossref] [PubMed]
- Koemans WJ, Lurvink RJ, Grootscholten C, et al. Synchronous peritoneal metastases of gastric cancer origin: incidence, treatment and survival of a nationwide Dutch cohort. Gastric Cancer 2021;24:800-9. [Crossref] [PubMed]
- Marrelli D, De Stefano A, de Manzoni G, et al. Prediction of recurrence after radical surgery for gastric cancer: a scoring system obtained from a prospective multicenter study. Ann Surg 2005;241:247-55. [Crossref] [PubMed]
- D'Angelica M, Gonen M, Brennan MF, et al. Patterns of initial recurrence in completely resected gastric adenocarcinoma. Ann Surg 2004;240:808-16. [Crossref] [PubMed]
- Yago A, Haruta S, Ueno M, et al. Adequate period of surveillance in each stage for curatively resected gastric cancer: analyzing the time and rates of recurrence. Gastric Cancer 2021;24:752-61. [Crossref] [PubMed]
- Chae S, Lee A, Lee JH. The effectiveness of the new (7th) UICC N classification in the prognosis evaluation of gastric cancer patients: a comparative study between the 5th/6th and 7th UICC N classification. Gastric Cancer 2011;14:166-71. [Crossref] [PubMed]
- Sun Z, Wang ZN, Zhu Z, et al. Evaluation of the seventh edition of American Joint Committee on Cancer TNM staging system for gastric cancer: results from a Chinese monoinstitutional study. Ann Surg Oncol 2012;19:1918-27.
- Wang P, Deng J, Sun Z, et al. Proposal of a novel subclassification of pN3b for improvement the prognostic discrimination ability of gastric cancer patients. Eur J Surg Oncol 2020;46:e20-6. [Crossref] [PubMed]
- Amin MB, Greene FL, Edge SB, et al. The Eighth Edition AJCC Cancer Staging Manual: Continuing to build a bridge from a population-based to a more "personalized" approach to cancer staging. CA Cancer J Clin 2017;67:93-9.
- Pan S, Wang P, Xing Y, et al. Retrieved lymph nodes from different anatomic groups in gastric cancer: a proposed optimal number, comparison with other nodal classification strategies and its impact on prognosis. Cancer Commun (Lond) 2019;39:49. [Crossref] [PubMed]
- Xie J, Pang Y, Li X, et al. The log odds of negative lymph nodes/T stage: a new prognostic and predictive tool for resected gastric cancer patients. J Cancer Res Clin Oncol 2021;147:2259-69. [Crossref] [PubMed]
- Li S, Desiderio J, Li Z, et al. The development and external validation of a nomogram predicting overall survival of gastric cancer patients with inadequate lymph nodes based on an international database. Int J Clin Oncol 2021;26:867-74. [Crossref] [PubMed]
- Zheng G, Feng F, Guo M, et al. Harvest of at Least 23 Lymph Nodes is Indispensable for Stage N3 Gastric Cancer Patients. Ann Surg Oncol 2017;24:998-1002. [Crossref] [PubMed]
- Marrelli D, Morgagni P, de Manzoni G, et al. External Validation of a Score Predictive of Recurrence after Radical Surgery for Non-Cardia Gastric Cancer: Results of a Follow-Up Study. J Am Coll Surg 2015;221:280-90. [Crossref] [PubMed]
- Pachaury A, Chaudhari V, Batra S, et al. Pathological N3 Stage (pN3/ypN3) Gastric Cancer: Outcomes, Prognostic Factors and Pattern of Recurrences After Curative Treatment. Ann Surg Oncol 2022;29:229-39. [Crossref] [PubMed]
- Komatsu S, Ichikawa D, Miyamae M, et al. Positive Lymph Node Ratio as an Indicator of Prognosis and Local Tumor Clearance in N3 Gastric Cancer. J Gastrointest Surg 2016;20:1565-71. [Crossref] [PubMed]
- Kano K, Aoyama T, Maezawa Y, et al. The survival and prognosticators of peritoneal cytology-positive gastric cancer patients who received upfront gastrectomy and subsequent S-1 chemotherapy. Int J Clin Oncol 2017;22:887-96. [Crossref] [PubMed]
- Hindocha S, Charlton TG, Linton-Reid K, et al. A comparison of machine learning methods for predicting recurrence and death after curative-intent radiotherapy for non-small cell lung cancer: Development and validation of multivariable clinical prediction models. EBioMedicine 2022;77:103911. [Crossref] [PubMed]
- Fan K, Cheng L, Li L. Artificial intelligence and machine learning methods in predicting anti-cancer drug combination effects. Brief Bioinform 2021;22:bbab271. [Crossref] [PubMed]
- Cruz JA, Wishart DS. Applications of machine learning in cancer prediction and prognosis. Cancer Inform 2007;2:59-77. [PubMed]
- Zhou C, Wang Y, Ji MH, et al. Predicting Peritoneal Metastasis of Gastric Cancer Patients Based on Machine Learning. Cancer Control 2020;27:1073274820968900. [Crossref] [PubMed]
- Zhou C, Hu J, Wang Y, et al. A machine learning-based predictor for the identification of the recurrence of patients with gastric cancer after operation. Sci Rep 2021;11:1571. [Crossref] [PubMed]
- Japanese gastric cancer treatment guidelines 2018 (5th edition). Gastric Cancer 2021;24:1-21.
- Khandezamin Z, Naderan M, Rashti MJ. Detection and classification of breast cancer using logistic regression feature selection and GMDH classifier. J Biomed Inform 2020;111:103591. [Crossref] [PubMed]
- Quist J, Taylor L, Staaf J, et al. Random Forest Modelling of High-Dimensional Mixed-Type Data for Breast Cancer Classification. Cancers (Basel) 2021;13:991. [Crossref] [PubMed]
- Hsiao YW, Tao CL, Chuang EY, et al. A risk prediction model of gene signatures in ovarian cancer through bagging of GA-XGBoost models. J Adv Res 2020;30:113-22. [Crossref] [PubMed]
- Zhu ZH, Sun BY, Ma Y, et al. Three immunomarker support vector machines-based prognostic classifiers for stage IB non-small-cell lung cancer. J Clin Oncol 2009;27:1091-9. [Crossref] [PubMed]
- Lou Z, Cheng Z, Li H, et al. Predicting miRNA-disease associations via learning multimodal networks and fusing mixed neighborhood information. Brief Bioinform 2022;23:bbac159. [Crossref] [PubMed]
- Ontivero-Ortega M, Lage-Castellanos A, Valente G, et al. Fast Gaussian Naïve Bayes for searchlight classification analysis. Neuroimage 2017;163:471-9. [Crossref] [PubMed]
- Maehara Y, Hasuda S, Koga T, et al. Postoperative outcome and sites of recurrence in patients following curative resection of gastric cancer. Br J Surg 2000;87:353-7. [Crossref] [PubMed]
- Shin VY, Wu WK, Ye YN, et al. Nicotine promotes gastric tumor growth and neovascularization by activating extracellular signal-regulated kinase and cyclooxygenase-2. Carcinogenesis 2004;25:2487-95. [Crossref] [PubMed]
- Lien YC, Wang W, Kuo LJ, et al. Nicotine promotes cell migration through alpha7 nicotinic acetylcholine receptor in gastric cancer cells. Ann Surg Oncol 2011;18:2671-9. [Crossref] [PubMed]
- Tian L, Chen X, Cao L, et al. Effects of plant-based medicinal food on postoperative recurrence and lung metastasis of gastric cancer regulated by Wnt/β-catenin-EMT signaling pathway and VEGF-C/D-VEGFR-3 cascade in a mouse model. BMC Complement Med Ther 2022;22:233. [Crossref] [PubMed]
- Nomura T, Kamio Y, Takasu N, et al. Intrahepatic micrometastases around liver metastases from gastric cancer. J Hepatobiliary Pancreat Surg 2009;16:493-501. [Crossref] [PubMed]
- Iwatsuki M, Mimori K, Fukagawa T, et al. The clinical significance of vimentin-expressing gastric cancer cells in bone marrow. Ann Surg Oncol 2010;17:2526-33. [Crossref] [PubMed]
- Song XH, Zhang WH. Prognostic impact of Borrmann classification on advanced gastric cancer: a retrospective cohort from a single institution in western China. World J Surg Oncol 2020;18:204. [Crossref] [PubMed]
- Lee JH, Nam BH, Ryu KW, et al. Tumor differentiation is not a risk factor for lymph node metastasis in elderly patients with early gastric cancer. Eur J Surg Oncol 2014;40:1771-6. [Crossref] [PubMed]
- Deans C, Yeo MS, Soe MY, et al. Cancer of the gastric cardia is rising in incidence in an Asian population and is associated with adverse outcome. World J Surg 2011;35:617-24. [Crossref] [PubMed]
- Chen Y, Chen Y, Wen L, et al. PN3b as an independent risk factor for poor prognosis and peritoneal recurrence in Borrmann type IV gastric cancer: A retrospective cohort study. Front Surg 2022;9:986696. [Crossref] [PubMed]
- Piessen G, Messager M, Leteurtre E, et al. Signet ring cell histology is an independent predictor of poor prognosis in gastric adenocarcinoma regardless of tumoral clinical presentation. Ann Surg 2009;250:878-87. [Crossref] [PubMed]
- Lee D, Son SY, Kim YB, et al. Neural Invasion is a Significant Contributor to Peritoneal Recurrence in Signet Ring Cell Gastric Carcinoma. Ann Surg Oncol 2018;25:1167-75. [Crossref] [PubMed]
- Cheng L, Chen S, Wu W, et al. Gastric cancer in young patients: a separate entity with aggressive features and poor prognosis. J Cancer Res Clin Oncol 2020;146:2937-47. [Crossref] [PubMed]
- Liu D, Lu M, Li J, et al. The patterns and timing of recurrence after curative resection for gastric cancer in China. World J Surg Oncol 2016;14:305. [Crossref] [PubMed]
- Ikoma N, Chen HC, Wang X, et al. Patterns of Initial Recurrence in Gastric Adenocarcinoma in the Era of Preoperative Therapy. Ann Surg Oncol 2017;24:2679-87. [Crossref] [PubMed]
- Guan H, Lu J, Qu M, et al. DNA methylation patterns of SOCS1 gene in peripheral blood identifies risk loci associated with bladder cancer based on principal component analysis. Neoplasma 2021;68:482-9. [Crossref] [PubMed]
- Qiu WW, Chen QY, Zheng WZ, et al. Postoperative follow-up for gastric cancer needs to be individualized according to age, tumour recurrence pattern, and recurrence time. Eur J Surg Oncol 2022;48:1790-8. [Crossref] [PubMed]