Construction and validation of a prognostic model for stemness-related genes in lung adenocarcinoma
Highlight box
Key findings
• A prognostic model based on 12 stemness-related genes (ACTB, PDGFB, MAGEH1, CPS1, KCTD9, FOLR1, SLC29A1, ENY2, INTS7, FUT1, SNN, TLE1) was established. This model can be used to predict the prognosis of lung adenocarcinoma (LUAD) patients.
What is known and what is new?
• Cancer stem cells possess significant characteristics such as self-renewal and multi-lineage differentiation, which can lead to LUAD metastasis, drug resistance, and recurrence.
• We established a stemness-related genes prognostic model to promote the development of precision medicine in LUAD.
What is the implication, and what should change now?
• The prognostic model could be used as a useful tool for clinicians to judge the prognosis of patients and develop new therapeutic targets for LUAD.
• Future diagnosis and treatment will further focus on stemness perspectives.
Introduction
Background
As one of the leading causes of cancer-related deaths worldwide, lung cancer maintains high incidence and mortality rates globally. Approximately 2.2 million cases are diagnosed with lung cancer each year, and the number of deaths related to lung cancer reaches nearly 1.8 million (1). Non-small cell lung carcinoma (NSCLC) accounts for about 85% of all lung cancer cases, with lung adenocarcinoma (LUAD) being the most common histological subtype, representing approximately 50% of all NSCLC cases (2). Despite the possibility of surgical treatment for early-stage LUAD patients, there is still a risk of postoperative recurrence. Additionally, many patients are diagnosed at advanced stages, and thus missing the opportunity for surgery. The overall 5-year survival rate for LUAD patients is only 26.4% (3). Early identification of high-risk lung cancer patients and providing personalized treatment can significantly reduce adverse prognosis. Currently, Tumor Node Metastasis (TNM) staging is still used in clinical practice to predict the prognosis of LUAD patients. With the emergence of targeted therapies, immunotherapy, and the continuous development of bioinformatics, there is an urgent clinical need for multiple biomarkers to predict patient prognosis.
Rationale and knowledge gap
The theory of cancer stem cells (CSCs) provides new insights into the diagnosis and treatment of tumors. The study (4) has confirmed that CSCs possess significant characteristics such as self-renewal and multi-lineage differentiation, which can lead to tumor metastasis, drug resistance, and recurrence. Targeting stemness-related signaling pathways (such as Hedgehog, Notch, Wnt, and TGF-β inhibitors), stemness surface markers (such as CD44 and CD133 inhibitors), and stemness metabolism (such as Bcl2 inhibitors) have been shown in phase I–III clinical trials to effectively reverse tumor stemness, inhibit malignant progression, and increase treatment sensitivity (5). Identifying the degree of stemness in lung cancer can effectively distinguish patients with poor prognosis and provide timely individualized comprehensive treatment to improve patient outcomes. In order to better describe the characteristics of CSCs, the concept of “stemness indices” has been introduced. This index model was established by Malta et al. (6) using machine learning algorithms based on a dataset of progenitor cells. This method allows for the calculation of mRNA-based stemness index (mRNAsi) for samples in The Cancer Genome Atlas (TCGA) database through RNA-sequencing analysis, enabling the evaluation of their stem cell properties. Due to the substantial heterogeneity among individual tumors, a single biomarker often lacks sufficient sensitivity and specificity to accurately predict patient prognosis. Therefore, integrating multiple biomarkers into the same model significantly improves its predictive value for tumor prognosis.
Weighted gene co-expression network analysis (WGCNA) (7) is a commonly used bioinformatics analysis method that can be used to describe the correlation patterns between high-throughput sequencing samples and their expressed genes. It helps discover highly correlated gene clusters, identify characteristics of gene modules and key genes within those modules, and establish relationships between gene modules and external sample features. The least absolute shrinkage and selection operator (LASSO) (8) is a regression analysis method that allows for simultaneous variable selection and regularization, aiming to improve the predictive accuracy and interpretability of statistical models. This algorithm has been widely applied in Cox proportional hazards regression models for survival analysis of high-dimensional data.
Objective
Therefore, in this study, based on the calculation of the stemness index (mRNAsi) using TCGA LUAD dataset, WGCNA analysis was applied to identify stemness-related genes. Furthermore, LASSO regression analysis was performed based on the prognostic information of LUAD to construct a risk prognostic model consisting of 12 genes for predicting the prognosis of LUAD. To facilitate clinical application, a nomogram based on this risk prognostic model was established, which can accurately and effectively identify high-risk LUAD patients with poor prognosis at an early stage, bringing clinical benefits to the patients. Additionally, using this risk model, the differences in immune response between the high- and low-risk groups were further explored, providing guidance for clinical immunotherapy. We present this study in accordance with the TRIPOD reporting checklist (available at https://tcr.amegroups.com/article/view/10.21037/tcr-23-1847/rc).
Methods
Data download and preprocessing
LUAD RNA-sequencing data and clinical data were downloaded from the TCGA database (http://portal.gdc.cancer.gov/). Cases with missing overall survival (OS) values or OS <30 days were excluded to improve the accuracy of the prognostic model. Finally, information on 428 cases with gene expression values and survival time was obtained. The external validation dataset, GSE68465, was obtained from the Gene Expression Omnibus (GEO) database (http://www.ncbi.nlm.nih.gov/geo). GSE68465 consisted of 442 LUAD patient samples and complete OS and transcriptome data were retrieved from the Affymetrix GPL6947 platform (Illumina HumanHT-12 v.3.0 Expression BeadChips). Data for mRNAsi calculation specific to LUAD were downloaded and processed from https://bioinformaticsfmrp.github.io/PanCanStem Web. The study was conducted in accordance with the Declaration of Helsinki (as revised in 2013).
Correlation between mRNAsi and survival prognosis and clinical features
mRNAsi based on mRNA expression is a quantitative representation of the stem cell characteristics of a sample, ranging from 0 to 1. The tumor samples were divided into high mRNAsi and low mRNAsi groups based on the median mRNAsi value. Survival analysis comparing the differences between the groups was conducted using the “survival” and “survminer” packages in R (9). The visualization analysis of clinical feature correlations was performed using the “beeswarm” package in R, and the differences in mRNAsi values among the groups were analyzed using the Kruskal-Wallis test.
WGCNA network construction for screening stemness-related genes
The gene co-expression network was constructed using the “WGCNA” R package to analyze and identify gene modules closely related to mRNAsi. The expression data in the samples were subjected to sample clustering analysis using the “hclust” function, and outlier samples were removed. Then, the “pickSoftThreshold” function was used to select the soft threshold. Further analysis of the modules was performed to calculate the differences between modules and construct a module dendrogram. The minimum number of genes in each module was set to 50, and a threshold of 0.25 for the cutting height was chosen to merge modules with high similarity. Correlation analysis was conducted between various enriched gene modules and mRNAsi. Stemness-related genes were further selected from the gene module with the highest correlation coefficient with mRNAsi.
Enrichment analysis of stemness-related genes using Gene Ontology (GO) and Kyoto Encyclopedia of Genes and Genomes (KEGG)
To explore the potential biological roles of differentially expressed stemness-related genes, the “clusterProfiler” package was used to perform GO and KEGG pathway enrichment analysis (10). Pathways and functions with q-value <0.05 were considered as enriched pathways and functions associated with stemness-related genes. Additionally, a circular plot was generated to visually display the stemness-related genes involved in significantly enriched pathways and GO functions.
Establishment of multi-genes LASSO regression model
The above-mentioned stemness-related genes were used to construct a prognostic model. The “survival” package was used to perform univariate Cox regression analysis, and genes with a significance level of P<0.05 were considered as prognostic-related genes. LASSO regression analysis, as a common machine learning method, can effectively handle multicollinearity issues and is often used for variable selection and prognostic model construction. The R package “glmnet” was utilized for LASSO Cox regression analysis (11), further screening prognostic genes, and constructing the prognostic model in the discovery cohort. The computational formula used in this analysis is as follows: risk score = ∑ coef(nk=1 gene k) * expr(gene k).
Here, coef(gene k) represents the abbreviated form of the gene coefficient associated with survival, and expr(gene k) denotes the expression level of the gene. Patients were classified into high-risk and low-risk groups based on the median risk score.
Validation of prognostic models
Using the external validation cohort GSE68465, the “survival” R package was utilized to compare the survival differences between high-risk and low-risk groups through Kaplan-Meier survival curves. The receiver operating characteristic (ROC) curve provides the magnitude of sensitivity and specificity, with specificity and sensitivity plotted on the x-axis and y-axis, respectively. The area under the curve (AUC) represents the accuracy of the prediction, with a larger AUC indicating higher predictive accuracy. The “survivalROC” package was used to generate ROC curves to evaluate the accuracy of the model in predicting survival.
In the TCGA LUAD discovery cohort, the cohort was randomly divided into an experimental group and a validation group. ROC curves were plotted on the entire discovery cohort to evaluate the accuracy of the model. Furthermore, Kaplan-Meier survival curves, as well as gene expression heatmaps and risk curves between high-risk and low-risk groups, were separately plotted on the whole discovery cohort, experimental group, and validation group. This evaluation aimed to assess the expression of prognostic-associated genes in the high-risk and low-risk groups within the model and further validate the differences in patient survival between the high-risk and low-risk groups.
The TCGA LUAD discovery cohort was subjected to univariable Cox regression analysis and multivariable Cox regression analysis using the “survival” R package. This analysis aimed to investigate whether the risk score and clinical features are independent factors in the analysis.
Construction of the nomogram
Using the “regplot” and “rms” R packages, a nomogram was constructed to predict the 1-, 3-, and 5-year survival rates of LUAD patients. The risk score and tumor stage were included in constructing the nomogram. Additionally, a calibration curve was built based on the Hosmer-Lemeshow test to evaluate the effectiveness of this nomogram.
Evaluation of immune phenotypes in high-risk and low-risk groups
Immune cell content, immune cell infiltration, and expression of immune checkpoint genes are all relevant indicators of tumor immunity and play an important role in the effectiveness of immunotherapy. We compared the differences in these immune-related indicators between high-risk and low-risk groups to assess the relationship between the prognostic model and tumor immunity. Single Sample Gene Set Enrichment Analysis (ssGSEA) (12) was used to evaluate the level of immune cell infiltration. We examined the expression of 22 immune checkpoint genes in different risk groups and identified significantly differentially expressed checkpoints. Data from Tumor Immune Dysfunction and Exclusion (TIDE) were used to predict patients’ potential response to immunotherapy (13). Tumor mutational burden (TMB) represents the total number of detected errors in somatic gene coding, including base substitutions, gene insertions or deletions, per million bases. TMB is used as an indicator to assess the frequency of gene mutations (14).
Statistical analysis
All statistical tests were performed using R4.1.3, including the two-sample Mann-Whitney test for continuous data, Fisher’s exact test or Chi-square test for categorical data, log-rank test for Kaplan-Meier curves, Hosmer-Lemeshow test for nomogram and Cox proportional hazards regression for estimating hazard ratios (HRs) and 95% confidence intervals (CIs). Correlation coefficients between different genes were estimated via Pearson correlation analysis. All statistical P values were two-sided, and P<0.05 was considered statistically significant.
Results
mRNAsi is significantly associated with LUAD
The Kaplan-Meier survival curve results showed that although not statistically significant (P=0.23), the overall mortality rate was higher in the high mRNAsi group compared to the low mRNAsi group (Figure 1A). This study also demonstrated a statistically significant difference of mRNAsi among genders (P=0.00016), with males showing higher mRNAsi levels (Figure 1B). Clinically, a later tumor stage indicates a poorer prognosis. This study revealed that late-stage patients had higher mRNAsi levels compared to early-stage patients, and the difference was statistically significant (P=0.041, P=0.034) (Figure 1C).
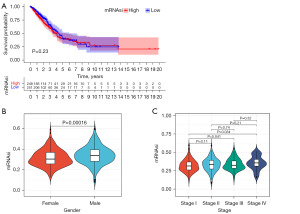
Selection of stemness-related genes
Sample clustering analysis using WGCNA was performed, and outlier samples with a height greater than 50,000 were removed. Further analysis of the modules resulted in the construction of a co-expression gene module dendrogram (Figure 2A). Based on the obtained mRNAsi index for TCGA LUAD, the correlation between each gene module and mRNAsi was analyzed. The results showed that the turquoise gene module had the highest positive correlation (correlation coefficient =0.65, P<0.001), and the green gene module had the highest negative correlation (correlation coefficient =−0.57, P<0.001) (Figure 2B).
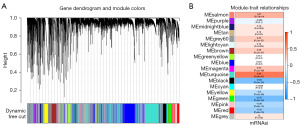
Enrichment analysis of stemness-related genes using GO and KEGG
The stemness-related genes with the highest positive and negative correlation coefficients obtained from WGCNA analysis were subjected to GO and KEGG enrichment analysis to identify relevant biological functions and signaling pathways. The results of the GO analysis showed that positively correlated stemness-related genes were mainly enriched in mRNA processing, chromosomal regions, and RNA catalytic activity, while negatively correlated stemness-related genes were primarily enriched in extracellular matrix organization and extracellular matrix structural constituent (Figure 3A,3B). The results of the KEGG analysis revealed that positively correlated stemness-related genes were mainly enriched in the cell cycle signaling pathway, while negatively correlated stemness-related genes were primarily enriched in the PI3K-Akt signaling pathway (Figure 3C,3D).
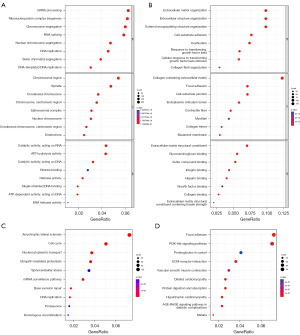
Construction of LASSO model for predicting the prognosis of LUAD stemness-related genes
Univariable Cox regression analysis was performed on the aforementioned stemness-related genes, and those with P<0.05 were further analyzed and included in the construction of the LASSO regression model. The R package “glmnet” returned a series of LASSO risk models, where each curve represents a gene, and the genes with non-zero coefficients at different log λ values form a LASSO risk model at that specific log λ value. Further analysis was conducted to select the optimal risk model (Figure 4). Based on the genes and regression coefficients obtained from LASSO Cox regression analysis, our prognostic model was constructed.
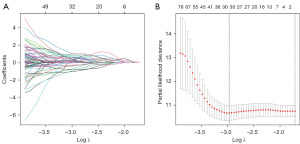
The calculation formula for the risk prognostic model is as follows: risk score =11.30019 × Exp (ACTB) + 2.160222 × Exp (PDGFB) + (−1.77683) × Exp (MAGEH1) + 1.02370 × Exp (CPS1) + 1.54319 × Exp (KCTD9) + (−1.14144) × Exp (FOLR1) + (−3.64535) × Exp (SLC29A1) + 4.11178 × Exp (ENY2) + 1.74603 × Exp (INTS7) + (−1.39269) × Exp (FUT1) + (−3.52131) × Exp (SNN)+ 1.54206 × Exp (TLE1). Each LUAD patient can calculate the risk score, which represents the prognostic score related to mRNAsi, based on the sum of the expression levels of each gene in the model multiplied by their respective regression coefficients.
Validation of the LASSO risk model
A series of analyses were conducted in the entire discovery cohort and external validation cohort GSE68465 in LUAD. Kaplan-Meier survival curves revealed that patients with a lower risk score based on stemness-related genes had longer survival times in the discovery cohort (P<0.001) (Figure 5A), the randomly divided experimental group within the discovery cohort (P<0.001) (Figure 5B), the randomly divided validation group within the discovery cohort (P<0.001) (Figure 5C), and the external validation cohort (P=0.009) (Figure 5D). In the discovery cohort, the results of univariable Cox regression analysis (Figure 5E) demonstrated that the risk score (HR =1.029, 95% CI: 1.020–1.038, P<0.001) was a significant risk factor for survival. The results of multivariable Cox regression analysis (Figure 5F) showed that the risk score (HR =1.031, 95% CI: 1.022–1.040, P<0.001) remained a significant risk factor for survival, consistent with the results of the univariate Cox regression analysis. Furthermore, the impact of the risk score of stemness-related genes on survival was independent of age, gender, and tumor stage, making it an independent predictor of survival.
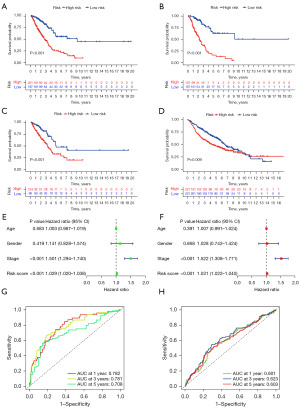
Subsequently, we plotted ROC curves for 1-, 3-, and 5-year predictions to assess the accuracy of the model. In the discovery cohort, the AUC values for the ROC curves at 1-, 3-, and 5-year were 0.782, 0.781, and 0.708, respectively (Figure 5G). In the external validation cohort, the AUC values for the ROC curves at 1-, 3-, and 5-year were 0.601, 0.623, and 0.603, respectively (Figure 5H).
Furthermore, we conducted a series of risk analyses for the prognostic model in the entire TCGA LUAD discovery cohort, as well as the randomly divided experimental and validation groups. We observed that the high-risk group had higher expression levels of ACTB, PDGFB, CPS1, CTD9, ENY2, INTS7, and TLE1, while the low-risk group had higher expression levels of MAGEH1, FOLR1, SLC29A1, FUT1, and SNN (Figure 6A). Patients were ranked according to their risk scores based on stemness-related genes risk (Figure 6B), and it was observed that the high-risk group had fewer surviving patients compared to the low-risk group (Figure 6C).
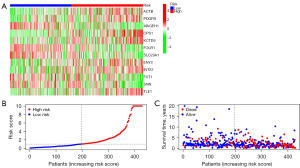
The risk analysis results conducted on the two subsets randomly divided within the discovery cohort were consistent with the results of the entire TCGA LUAD discovery cohort. The risk analysis also indicated that the high-risk group in both subsets had higher expression levels of ACTB, PDGFB, CPS1, CTD9, ENY2, INTS7, and TLE1, while the low-risk group had higher expression levels of MAGEH1, FOLR1, SLC29A1, FUT1, and SNN (Figure 7A,7B). Patients were ranked according to their risk scores based on mRNAsi-related gene risk (Figure 7C,7D), and it was observed that the high-risk group had fewer surviving patients compared to the low-risk group (Figure 7E,7F).
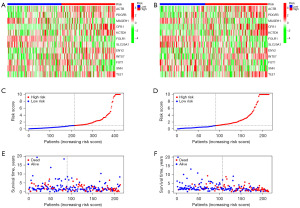
Establishment and evaluation of nomogram
For the convenience of clinical application, based on the LASSO risk model, various clinical pathological features (gender, T stage, N stage) were integrated to construct a nomogram (Figure 8A). The total score was obtained by summing up each score in the model, and it was used to predict the 1-, 3-, and 5-year survival probabilities of patients. The calibration curves for 1, 3, and 5 years demonstrated high concordance between predicted survival and actual survival in the nomogram (Figure 8B). The nomogram constructed based on the prognostic model accurately predicts the OS of LUAD patients, further indicating that the prognostic model exhibits good predictive performance.
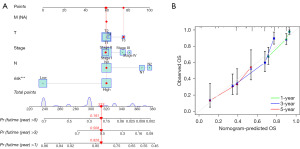
Tumor microenvironment characteristics and treatment response in high-risk and low-risk groups
Tumor stemness is associated with tumor invasion and metastasis. Invasion and metastasis are closely related to the extracellular matrix, which plays a crucial role in determining the anti-tumor immune response in solid tumors. Therefore, we further investigated the differences in immune aspects between the high-risk and low-risk groups. The results of ssGSEA showed significant differences in the infiltration of 15 immune cell subtypes between the high-risk and low-risk groups. In the low-risk group, the tumor infiltration of activated B cells, eosinophils, immature B cells, immature dendritic cells, mast cells, monocytes, plasma cell-like dendritic cells, T follicular helper cells, and Th17 cells was higher compared to the high-risk group. On the other hand, the high-risk group had a higher proportion of activated CD4 T cells, CD56 natural killer cells, γδ T cells, natural killer T cells, neutrophils, and Th2 cells than the low-risk group (Figure 9A). Furthermore, we analyzed the relationship between the high-risk and low-risk groups and the expression of immune checkpoint genes. The expression of TNFRSF9, CD200, NRP1, CD276, TNFSF4, CD274, TNFSF9, and PDCD1LG2 was higher in the high-risk group, while the expression of CD40LG, HHLA2, CD48, TNFSF18, ADORA2A, ATCN1, CD27, TNFRSF14, CD200R1, LGALS9, CD28, TNFSF15, BTLA, and IDO2 was higher in the low-risk group (Figure 9B). TIDE uses a set of gene expression markers to evaluate the functional impairment of tumor-infiltrating cytotoxic T lymphocytes (CTLs) and the inhibitory factors of the immune response to CTLs. Higher TIDE scores indicate poorer response to immune checkpoint blockade (ICB). The high-risk group had higher TIDE scores compared to the low-risk group (Figure 9C). TMB serves as a biomarker for predicting the response to immunotherapy (15). Higher TMB indicates a higher frequency of gene mutations. The high-risk group had a higher TMB level than the low-risk group (Figure 9D).
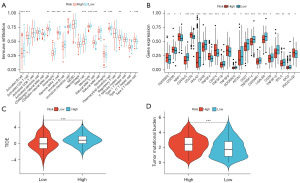
Discussion
Key findings and explanations of findings
CSCs are the source of malignant tumor proliferation and recurrence and have become a hot research topic in the field of oncology in recent years. CSCs are closely associated with various malignant phenotypes such as tumor cell proliferation, invasion, metastasis, and drug resistance (16,17). In order to effectively assess the degree of tumor stemness, Malta et al. (6) proposed the mRNAsi in 2018. Therefore, biomarkers related to stemness identified through mRNAsi analysis hold great prognostic potential. In this study, we first calculated the mRNAsi data for LUAD provided by Malta et al. and validated the differential expression of mRNAsi in LUAD samples from the TCGA database. We found that mRNAsi values were positively correlated with distant metastasis and tumor histological grade. Survival curve analysis showed a significant decrease in OS rate in the high mRNAsi group compared to the low mRNAsi group. These results are consistent with the characteristics of CSCs and in line with expected outcomes. We then constructed a WGCNA network based on the relationship between genes and mRNAsi in LUAD samples from TCGA. From the WGCNA analysis, we identified the turquoise and green gene modules with the highest correlation with mRNAsi. Subsequently, we performed univariate Cox regression analysis on the differentially expressed genes identified through the WGCNA analysis to obtain prognostic-related stemness-associated genes. Using LASSO regression analysis, we constructed a prognostic model based on the expression of these stemness-associated genes. The model included 12 stemness-associated genes (ACTB, PDGFB, MAGEH1, CPS1, KCTD9, FLOR1, SLC29A1, ENY2, INTS7, FUT1, SNN, TLE1). Among these 12 genes, ACTB, PDGFB, CPS1, KCTD9, ENY2, INTS7, and TLE1 were positively correlated with stemness and acted as oncogenes, with their high expression being a risk factor for patient survival. The remaining five genes showed a negative correlation with stemness and their high expression was associated with longer OS in LUAD patients, indicating their potential tumor suppressor role in LUAD.
Strengths and limitations
This study identified 12 stemness-related genes that are associated with prognosis and used them to construct a prognostic model. This prognostic model effectively predicts the prognosis of LUAD patients in a clinical setting and may serve as a valuable supplement to the current clinical TNM staging system. Undeniably, our study has some limitations. Firstly, our LUAD samples were retrospectively collected from public databases. Secondly, certain important clinical pathological indicators such as pleural invasion, intravascular tumor emboli, and imaging features were unavailable in the TCGA dataset. Additionally, this study did not consider the systemic therapies (adjuvant or first-line treatment) received by patients. These may reduce the prognostic predictive value of the integrated risk model. Lastly, we have not conducted mechanistic studies on the role of prognostically related stemness-associated genes in LUAD.
Comparison with similar researches
The ACTB gene encodes β-actin, which is considered an endogenous housekeeping gene and widely used as a reference gene for quantifying expression levels in tumors (18). However, increasing evidence suggests that ACTB is upregulated in melanoma (19), renal cancer (20), lung cancer (21), and other types of tumors. Abnormal expression and polymerization of ACTB, along with the resulting changes in cellular cytoskeleton, are associated with cancer invasion and metastasis. The dysregulation of ACTB may be involved in the development and malignancy of lung cancer, and its upregulation could serve as a marker for tumor occurrence in lung cancer cells (22,23). PDGFB is a platelet-derived growth factor located on chromosome 22 and is normally expressed at relatively low levels. PDGFB is highly expressed in NSCLC tissue (24). Its expression is correlated with tumor cell growth, metastasis, and invasion. High expression of PDGFB and platelet-derived growth factor receptor (PDGFR) in tumor cells is an independent prognostic risk indicator for disease-specific survival in NSCLC patients (25). In tumor angiogenesis, there are complex interactions between endothelial cells, stromal cells, and tumor cells. Platelet-derived growth factors (PDGFs) and their receptors (PDGFRs) play crucial roles in these interactions and are important targets for novel anti-angiogenic therapy. The CPS1 gene encodes carbamoyl-phosphate synthetase 1, which is not only a key catalyst in the urea cycle but also plays a role in cancer progression. The study has shown that CPS1 is downregulated in hepatocellular carcinoma (HCC), and its low expression predicts poor prognosis for patients (26). CPS1 has also been identified as a biomarker for colorectal cancer progression (27). Upregulation of CPS1 expression in LUAD is generally associated with poor prognosis and lower OS rates. Research (28) has shown that knocking out CPS1 in LADC cells depletes metabolites in the nucleotide synthesis pathway, inhibiting cell proliferation and showing synergistic effects with drugs that block DNA synthesis pathways. Furthermore, LUAD is a common type of lung cancer associated with overexpression and activating mutations of EGFR, which has been targeted for the treatment of LUAD patients. The experimental study (29) has shown that when EGFR is inhibited, LUAD cells become more dependent on the urea cycle, particularly CPS1. Inhibition of both CPS1 and EGFR suppresses cell cycle progression and cell proliferation. CPS1 is likely to be a promising therapeutic target for LUAD in the future. The gene KCTD9 encodes a protein containing a potassium channel tetramerization domain, sharing a conserved BTB domain at the N-terminal (30). Most KCTD proteins interact with Cullin3-dependent E3 ubiquitin ligase through the BTB domain and are closely associated with protein ubiquitination (31). The study suggests that KCTD family genes are involved in the regulation of tumor development (32). KCTD9 is one of the members of the KCTD protein family (33). Zhang et al.’s research (34) confirms that natural killer cells containing silenced KCTD9 exhibit weakened tumor cytotoxicity in vitro. KCTD9 influences human innate immune cells, leading to tumor progression. Studies have indicated a significant correlation between high expression of KCTD9 and advanced lung cancer, lymph node metastasis, TP53 mutation, and poor prognosis. ENY2, along with USP22 and ATXN7L3, is a component of the deubiquitinating module in SAGA complexes. These three molecules are essential co-factors for transcriptional activity, and an imbalance in their activity will promote tumor growth (35). Xie’s research data indicates that upregulation of ENY2 expression can promote invasion and lung metastasis of triple-negative breast cancer cells both in vitro and in vivo (36). INT is one of the major components of ribonucleic acid (RNA) polymerase II mediated transcription machinery, and is involved in regulating most dependent genes (37). Studies have shown that certain INT subunits may be associated with human cancer. INTS7 has been demonstrated to be significantly overexpressed in various human cancers, including breast cancer, cholangiocarcinoma, and HCC (38). Li’s team found that the expression of INTS7 in LUAD tissues was significantly higher than in adjacent normal tissues. Additionally, Kaplan-Meier survival analysis indicated that LUAD patients with high levels of INTS7 expression had a poorer prognosis. It was also discovered that INTS7 could enhance the migration and invasion of LUAD cells, induce cell proliferation, and weaken apoptotic ability (39). TLE1 is a member of the Groucho/TLE family of transcriptional co-repressors that regulate the transcriptional activity of a wide range of genes (40). Yao’s team discovered that TLE1 is significantly upregulated in A549 LUAD cells, and it promotes epithelial-mesenchymal transition (EMT) by inhibiting E-cadherin, leading to tumor migration and invasion (41).
MAGEH1 belongs to the non-cancer/testis subgroup of the melanoma-associated antigen (MAGE) superfamily (42). A study on MAGH1 in HCC revealed that the expression of MAGEH1 is negatively correlated with HCC cell migration, proliferation, and invasion. Furthermore, patients with high expression of MAGEH1 have higher survival rates and lower recurrence rates after radical resection (43). The SLC29A1 gene encodes the human equilibrative nucleoside transporter 1 (hENT1). The nucleoside transporters play an important role in modulating the physiological activity of nucleosides and in the transport of many therapeutic nucleoside drugs used as cancer treatment. Research has confirmed that NSCLC patients with low expression of hENT1 are unresponsive to chemotherapy drugs containing gemcitabine, which leads to a higher likelihood of disease progression (44). The FUT1 gene encodes fucosyltransferase 1. Laminin N-glycosylation plays a crucial role in the process of cellular adhesion and migration, and abnormal laminin glycosylation has been observed in various types of cancers, which is associated with tumor development and metastatic ability (45). Previous study (46) has shown that patients with high expression of FUT1 have a poorer prognosis in colon adenocarcinoma. However, the situation is opposite in lung cancer. The study has shown that FUT1 is downregulated in NSCLC patients and is associated with poor prognosis. These results suggest that targeting laminin glycosylation may be a promising strategy for developing novel NSCLC treatments (47). However, there have been relatively few studies on the FLOR1 and SNN genes in cancer, and their mechanisms and functions are still being investigated. Nevertheless, they may be involved in regulating and modulating various biological activities.
Implications and actions needed
The prognostic model developed in this study can serve as a supplement to the TNM (48) staging system. Physicians can use this prognostic risk model to provide personalized predictions for LUAD patients and develop appropriate treatment plans for maximizing clinical benefits. On this basis, prospective cohort studies are needed to validate the prognostic genes and prognostic model we obtained. Further cellular and animal experiments are required to elucidate the mechanisms of action of these stemness-related genes in tumorigenesis.
Conclusions
In conclusion, this study identified 12 stemness-related genes that are associated with prognosis and used them to construct a prognostic model. This prognostic model effectively predicts the prognosis of LUAD patients in a clinical setting and may serve as a valuable supplement to the current clinical TNM staging system. Physicians can use this prognostic risk model to provide personalized predictions for LUAD patients and develop appropriate treatment plans for maximizing clinical benefits.
Acknowledgments
We would like to acknowledge the contributions from the TCGA and GEO networks.
Funding: None.
Footnote
Reporting Checklist: The authors have completed the TRIPOD reporting checklist. Available at https://tcr.amegroups.com/article/view/10.21037/tcr-23-1847/rc
Peer Review File: Available at https://tcr.amegroups.com/article/view/10.21037/tcr-23-1847/prf
Conflicts of Interest: All authors have completed the ICMJE uniform disclosure form (available at https://tcr.amegroups.com/article/view/10.21037/tcr-23-1847/coif). The authors have no conflicts of interest to declare.
Ethical Statement: The authors are accountable for all aspects of the work in ensuring that questions related to the accuracy or integrity of any part of the work are appropriately investigated and resolved.
Open Access Statement: This is an Open Access article distributed in accordance with the Creative Commons Attribution-NonCommercial-NoDerivs 4.0 International License (CC BY-NC-ND 4.0), which permits the non-commercial replication and distribution of the article with the strict proviso that no changes or edits are made and the original work is properly cited (including links to both the formal publication through the relevant DOI and the license). See: https://creativecommons.org/licenses/by-nc-nd/4.0/.
References
- Sung H, Ferlay J, Siegel RL, et al. Global Cancer Statistics 2020: GLOBOCAN Estimates of Incidence and Mortality Worldwide for 36 Cancers in 185 Countries. CA Cancer J Clin 2021;71:209-49. [Crossref] [PubMed]
- Thai AA, Solomon BJ, Sequist LV, et al. Lung cancer. Lancet 2021;398:535-54. [Crossref] [PubMed]
- Ganti AK, Klein AB, Cotarla I, et al. Update of Incidence, Prevalence, Survival, and Initial Treatment in Patients With Non-Small Cell Lung Cancer in the US. JAMA Oncol 2021;7:1824-32. [Crossref] [PubMed]
- Peitzsch C, Tyutyunnykova A, Pantel K, et al. Cancer stem cells: The root of tumor recurrence and metastases. Semin Cancer Biol 2017;44:10-24. [Crossref] [PubMed]
- Saygin C, Matei D, Majeti R, et al. Targeting Cancer Stemness in the Clinic: From Hype to Hope. Cell Stem Cell 2019;24:25-40. [Crossref] [PubMed]
- Malta TM, Sokolov A, Gentles AJ, et al. Machine Learning Identifies Stemness Features Associated with Oncogenic Dedifferentiation. Cell 2018;173:338-354.e15. [Crossref] [PubMed]
- Langfelder P, Horvath S. WGCNA: an R package for weighted correlation network analysis. BMC Bioinformatics 2008;9:559. [Crossref] [PubMed]
- Ternès N, Rotolo F, Michiels S. Empirical extensions of the lasso penalty to reduce the false discovery rate in high-dimensional Cox regression models. Stat Med 2016;35:2561-73. [Crossref] [PubMed]
- Li JCA. Modeling survival data: Extending the Cox model. Sociological Methods & Research. 2003;32:117-20. [Crossref]
- Wu T, Hu E, Xu S, et al. clusterProfiler 4.0: A universal enrichment tool for interpreting omics data. Innovation (Camb) 2021;2:100141. [Crossref] [PubMed]
- Friedman J, Hastie T, Tibshirani R. Regularization Paths for Generalized Linear Models via Coordinate Descent. J Stat Softw 2010;33:1-22. [Crossref] [PubMed]
- Hänzelmann S, Castelo R, Guinney J. GSVA: gene set variation analysis for microarray and RNA-seq data. BMC Bioinformatics 2013;14:7. [Crossref] [PubMed]
- Jiang P, Gu S, Pan D, et al. Signatures of T cell dysfunction and exclusion predict cancer immunotherapy response. Nat Med 2018;24:1550-8. [Crossref] [PubMed]
- Chan TA, Yarchoan M, Jaffee E, et al. Development of tumor mutation burden as an immunotherapy biomarker: utility for the oncology clinic. Ann Oncol 2019;30:44-56. [Crossref] [PubMed]
- Ock CY, Hwang JE, Keam B, et al. Genomic landscape associated with potential response to anti-CTLA-4 treatment in cancers. Nat Commun 2017;8:1050. [Crossref] [PubMed]
- Chang JC. Cancer stem cells: Role in tumor growth, recurrence, metastasis, and treatment resistance. Medicine (Baltimore) 2016;95:S20-5. [Crossref] [PubMed]
- Olmeda F, Ben Amar M. Clonal pattern dynamics in tumor: the concept of cancer stem cells. Sci Rep 2019;9:15607. [Crossref] [PubMed]
- Guo C, Liu S, Wang J, et al. ACTB in cancer. Clin Chim Acta 2013;417:39-44. [Crossref] [PubMed]
- Suzuki A, Iizuka A, Komiyama M, et al. Identification of melanoma antigens using a Serological Proteome Approach (SERPA). Cancer Genomics Proteomics 2010;7:17-23. [PubMed]
- Jung M, Ramankulov A, Roigas J, et al. In search of suitable reference genes for gene expression studies of human renal cell carcinoma by real-time PCR. BMC Mol Biol 2007;8:47. [Crossref] [PubMed]
- Nguewa PA, Agorreta J, Blanco D, et al. Identification of importin 8 (IPO8) as the most accurate reference gene for the clinicopathological analysis of lung specimens. BMC Mol Biol 2008;9:103. [Crossref] [PubMed]
- Saviozzi S, Cordero F, Lo Iacono M, et al. Selection of suitable reference genes for accurate normalization of gene expression profile studies in non-small cell lung cancer. BMC Cancer 2006;6:200. [Crossref] [PubMed]
- Gámez-Pozo A, Sánchez-Navarro I, Nistal M, et al. MALDI profiling of human lung cancer subtypes. PLoS One 2009;4:e7731. [Crossref] [PubMed]
- Donnem T, Al-Saad S, Al-Shibli K, et al. Co-expression of PDGF-B and VEGFR-3 strongly correlates with lymph node metastasis and poor survival in non-small-cell lung cancer. Ann Oncol 2010;2Rf1:223-31.
- Donnem T, Al-Saad S, Al-Shibli K, et al. Prognostic impact of platelet-derived growth factors in non-small cell lung cancer tumor and stromal cells. J Thorac Oncol 2008;3:963-70. [Crossref] [PubMed]
- Ridder DA, Schindeldecker M, Weinmann A, et al. Key Enzymes in Pyrimidine Synthesis, CAD and CPS1, Predict Prognosis in Hepatocellular Carcinoma. Cancers (Basel) 2021;13:744. [Crossref] [PubMed]
- Palaniappan A, Ramar K, Ramalingam S. Computational Identification of Novel Stage-Specific Biomarkers in Colorectal Cancer Progression. PLoS One 2016;11:e0156665. [Crossref] [PubMed]
- Çeliktas M, Tanaka I, Tripathi SC, et al. Role of CPS1 in Cell Growth, Metabolism and Prognosis in LKB1-Inactivated Lung Adenocarcinoma. J Natl Cancer Inst 2017;109:1-9. [Crossref] [PubMed]
- Pham-Danis C, Gehrke S, Danis E, et al. Urea Cycle Sustains Cellular Energetics upon EGFR Inhibition in EGFR-Mutant NSCLC. Mol Cancer Res 2019;17:1351-64. [Crossref] [PubMed]
- Liu Z, Xiang Y, Sun G. The KCTD family of proteins: structure, function, disease relevance. Cell Biosci 2013;3:45. [Crossref] [PubMed]
- Pinkas DM, Sanvitale CE, Bufton JC, et al. Structural complexity in the KCTD family of Cullin3-dependent E3 ubiquitin ligases. Biochem J 2017;474:3747-61. [Crossref] [PubMed]
- Angrisani A, Di Fiore A, De Smaele E, et al. The emerging role of the KCTD proteins in cancer. Cell Commun Signal 2021;19:56. [Crossref] [PubMed]
- Shi YX, Zhang WD, Dai PH, et al. Comprehensive analysis of KCTD family genes associated with hypoxic microenvironment and immune infiltration in lung adenocarcinoma. Sci Rep 2022;12:9938. [Crossref] [PubMed]
- Zhang X, Wang P, Chen T, et al. Kctd9 Deficiency Impairs Natural Killer Cell Development and Effector Function. Front Immunol 2019;10:744. [Crossref] [PubMed]
- Zhao Y, Lang G, Ito S, et al. A TFTC/STAGA module mediates histone H2A and H2B deubiquitination, coactivates nuclear receptors, and counteracts heterochromatin silencing. Mol Cell 2008;29:92-101. [Crossref] [PubMed]
- Xie G, Yang H, Ma D, et al. Integration of whole-genome sequencing and functional screening identifies a prognostic signature for lung metastasis in triple-negative breast cancer. Int J Cancer 2019;145:2850-60. [Crossref] [PubMed]
- Baillat D, Wagner EJ. Integrator: surprisingly diverse functions in gene expression. Trends Biochem Sci 2015;40:257-64. [Crossref] [PubMed]
- Federico A, Rienzo M, Abbondanza C, et al. Pan-Cancer Mutational and Transcriptional Analysis of the Integrator Complex. Int J Mol Sci 2017;18:936. [Crossref] [PubMed]
- Li Z, Zhu P, Wang M, et al. Correlation between oncogene integrator complex subunit 7 and a poor prognosis in lung adenocarcinoma. J Thorac Dis 2022;14:4815-27. [Crossref] [PubMed]
- Chen G, Courey AJ. Groucho/TLE family proteins and transcriptional repression. Gene 2000;249:1-16. [Crossref] [PubMed]
- Yao X, Ireland SK, Pham T, et al. TLE1 promotes EMT in A549 lung cancer cells through suppression of E-cadherin. Biochem Biophys Res Commun 2014;455:277-84. [Crossref] [PubMed]
- Chomez P, De Backer O, Bertrand M, et al. An overview of the MAGE gene family with the identification of all human members of the family. Cancer Res 2001;61:5544-51. [PubMed]
- Wang PC, Hu ZQ, Zhou SL, et al. Downregulation of MAGE family member H1 enhances hepatocellular carcinoma progression and serves as a biomarker for patient prognosis. Future Oncol 2018;14:1177-86. [Crossref] [PubMed]
- Oguri T, Achiwa H, Muramatsu H, et al. The absence of human equilibrative nucleoside transporter 1 expression predicts nonresponse to gemcitabine-containing chemotherapy in non-small cell lung cancer. Cancer Lett 2007;256:112-9. [Crossref] [PubMed]
- Tuccillo FM, de Laurentiis A, Palmieri C, et al. Aberrant glycosylation as biomarker for cancer: focus on CD43. Biomed Res Int 2014;2014:742831. [Crossref] [PubMed]
- Wang P, Liu X, Yu J, et al. Fucosyltransferases Regulated by Fusobacterium Nucleatum and Act as Novel Biomarkers in Colon Adenocarcinoma. J Inflamm Res 2023;16:747-68. [Crossref] [PubMed]
- Park S, Lim JM, Chun JN, et al. Altered expression of fucosylation pathway genes is associated with poor prognosis and tumor metastasis in non‑small cell lung cancer. Int J Oncol 2020;56:559-67. [PubMed]
- Asamura H, Nishimura KK, Giroux DJ, et al. IASLC Lung Cancer Staging Project: The New Database to Inform Revisions in the Ninth Edition of the TNM Classification of Lung Cancer. J Thorac Oncol 2023;18:564-75.