The prognostic biomarker TPGS2 is correlated with immune infiltrates in pan-cancer: a bioinformatics analysis
Highlight box
Key findings
• Tubulin polyglutamylase complex subunit 2 (TPGS2) is a potential prognostic and immunotherapeutic biomarker in many types of cancers, especially colon adenocarcinoma and stomach adenocarcinoma.
What is known and what is new?
• TPGS2 has been found to be associated with some tumors.
• TPGS2 plays a crucial role in tumor immunity.
What is the implication, and what should change now?
• TPGS2 is a promising tumor immune target, and more research on TPGS2 and tumor immunity should be conducted.
Introduction
At present, cancer is a leading cause of disease morbidity and mortality worldwide. Unfortunately, the number of newly diagnosed cases continues to grow (1,2). Current mainstream treatment modalities, including surgery, chemotherapy, radiotherapy, targeted therapy, and immunotherapy, still do not provide a satisfactory prognosis for cancer patients (3). This pan-cancer study sought to apply diagnostic and therapeutic applications in gastric cancer to a broad range of tumors with characteristics of similarity. The identification of common key genes between different types of cancers can help in cancer diagnosis and treatment (4,5).
The application of immunotherapy has opened up a new era in tumor treatment, and it has achieved encouraging results in the treatment of several tumors (including lung cancer, melanoma, and liver cancer), greatly improving the prognosis of patients (6,7). Many immune checkpoint inhibitors (ICIs), such as programmed cell death protein 1 (PD-1), programmed cell death ligand 1 (PD-L1), and cytotoxic T lymphocyte-associated antigen 4 (CTLA-4) inhibitors, have been established as routine treatments for many types malignancies; however, their clinical efficacy is limited (8,9). Given the complexities in the efficacy of immunotherapy, it is of great significance to explore new and more effective immune biomarkers.
The tumor microenvironment (TME) refers to the pericellular environment including immune cells, blood vessels, extracellular matrices, fibroblasts, mast cells, and various signaling molecules around the tumor (10,11). In recent years, the important role of the TME in tumorigenesis and development has been recognized. Tumor proliferation, infiltration, and metastasis depend not only on the tumor cells but also on the regulation of various cellular and signaling molecules in the TME (12). T cells, which are crucial in the anti-tumor immune response, have been relatively well studied among adaptive immune cells (13,14). Conversely, the second adaptive immune cell population (i.e., B cells) in the TME has not been well characterized.
However, in the last 5 years, several studies have reported an association between the presence of B cells in the TME and improved clinical outcomes (15-20). B cells can resist tumors by producing tumor-specific antibodies under certain conditions, but specific B cell subsets and antibody specificity can also suppress anti-tumor immunity and promote tumor growth (21). Meanwhile, infiltrating B cells are an important component of tertiary lymphoid structures (TLSs) in tumor tissues (15). Many studies have shown that the number and proportion of stromal cells and immune cells in tumor tissues are closely related to clinical features and prognosis (22-28). A thorough understanding of the TME is essential for accurate evaluation and treatment. To improve prognosis, more targeted molecules need to be identified for cancer diagnosis and treatment and to assess patient prognosis.
Few relevant studies have been conducted on tubulin polyglutamylase complex subunit 2 (TPGS2). We obtained partial information on TPGS2 from the Entrez Molecular Sequence Database Entrez (https://www.ncbi.nlm.nih.gov/search/), which showed that TPGS2 encodes a protein that is an element of the neuronal polyglutamylase complex, which plays a role in the post-translational addition of glutamate residues to C-terminal tubulin tails, and alternatively spliced transcript variants encoding multiple isoforms have been observed for this gene. TPGS2 also appears to be associated with tumors and the TME (29,30).
In our preliminary analysis, we confirmed that TPGS2 has a special role in cancer immunity. Based on this finding, we then performed the pan-cancer analysis to explore the expression, prognostic function, and immune role of TPGS2 in various cancers. We comprehensively analyzed the relationship between TPGS2 expression and patient prognosis in 33 types of cancer. Additionally, we further evaluated the association between TPGS2 and tumor-infiltrating immune cells. Our findings revealed that TPGS2 has a potential role in the development and progression of cancers; thus, TPGS2 may serve as a potential prognostic and immunotherapeutic biomarker. We present this article in accordance with the REMARK reporting checklist (available at https://tcr.amegroups.com/article/view/10.21037/tcr-23-113/rc).
Methods
The research data were downloaded from The Cancer Genome Atlas (TCGA), Genotype-Tissue Expression (GTEx), Cancer Cell Line Encyclopedia (CCLE), and Human Protein Atlas (HPA). databases. Data were downloaded for the following tumors: adrenocortical carcinoma (ACC), bladder urothelial carcinoma (BLCA), breast carcinoma (BRCA), cervical squamous cell carcinoma and endocervical adenocarcinoma (CESC), cholangiocarcinoma (CHOL), colon adenocarcinoma (COAD), lymphoid neoplasm diffuse large B-cell lymphoma (DLBC), esophageal carcinoma (ESCA), glioblastoma multiforme (GBM), head and neck squamous cell carcinoma (HNSC), kidney chromophobe (KICH), kidney renal clear cell carcinoma (KIRC), kidney renal papillary cell carcinoma (KIRP), acute myeloid leukemia (LAML), brain low-grade glioma (LGG), liver hepatocellular carcinoma (LIHC), lung adenocarcinoma (LUAD), lung squamous cell carcinoma (LUSC), mesothelioma (MESO), ovarian serous cystadenocarcinoma (OV), pancreatic adenocarcinoma (PAAD), pheochromocytoma and paraganglioma (PCPG), prostate adenocarcinoma (PRAD), rectum adenocarcinoma (READ), sarcoma (SARC), skin cutaneous melanoma (SKCM), stomach adenocarcinoma (STAD), testicular germ cell tumor (TGCT), thyroid carcinoma (THCA), thymoma (THYM), uterine corpus endometrial carcinoma (UCEC), uterine carcinosarcoma (UCS), and uveal melanoma (UM or UVM). The study was conducted in accordance with the Declaration of Helsinki (as revised in 2013).
Data source and processing
The gene expression and clinical data of normal human tissues and cancer tissues were downloaded from the GTEx database and TCGA by UCSC Xena (https://xenabrowser.net/) (31). For a multidimensional demonstration, the expression of TPGS2 was analyzed in various cancer cell lines with data from the CCLE. The transcripts per million (TPM) format and the log2(TPM+1) format were used for the expression profiles and subsequent analyses. Statistical significance was defined as follows: P<0.05, P<0.01, and P<0.001.
IHC of TPGS2
The protein expression of the tumor tissues and normal tissues from the HPA (http://www.proteinatlas.org/) database was applied to verify the protein expression levels of TPGS2 (32). Data from the HPA database were also used to confirm the intensity of TPGS2 in immunohistochemical staining in six normal and cancer tissues, including LIHC, LUSC, COAD, STAD, PRAD, and TGCT.
Genomic alterations analysis of TPGS2
The cBioPortal (http://www.cbioportal.org) is a multipurpose cancer genomics database that can recognize the molecular information of cancer tissues and comprehend the associated genetics, epigenetics, gene expression, and proteome information (33,34). The cBioPortal was used to display the alteration frequency (including mutation, structural variation, amplification, deep deletion, and multiple alterations) across cancers, and the results were visualized in bar plots. We also obtained a landscape map of the gene mutation sites, a correlation diagram of the copy number alterations (CNAs) and TPGS2 expression, and Kaplan-Meier curves of the pan-cancer data using the cBioPortal webtool.
Prognostic analysis
The UCSC Xena database was used to download the related prognostic data, including overall survival (OS), progression-free survival (PFS), and disease-specific survival (DSS) data. Next, we plotted the Kaplan-Meier model and univariate Cox regression results to assess the prognosis of various cancers. The TPGS2 expression median of each cancer was used to divide patients into high- and low-expression subgroups. Next, the Kaplan-Meier method was used to compute the log-rank P value and hazard ratio (HR) with a 95% confidence interval (CI). The “survival” package (3.2-10) was used for the statistical analysis of the survival data, and the “survminer” package (0.4.9) was used for the visualization.
Single-cell analysis of TPGS2
The Cancer Single-cell State Atlas (CancerSEA), a specialized single-cell sequencing database, provides various functional data on cancer cells at the single-cell level (35). The average correlations between the TPGS2 expression and functional states in different cancers were summarized and presented in a heatmap. The correlations between TPGS2 expression and several tumor functions were investigated using single-cell sequencing data. The TPGS2 expression profiles of single cells are shown in the t-distributed stochastic neighbor embedding (t-SNE) diagrams.
Gene set enrichment analysis (GSEA)
A GSEA was conducted using the “clusterProfiler” package (3.14.3), and “ggplot2” (3.3.3) was used to graph the results (36,37). The reference gene set was c5.bp.v7.2.symbols.gmt (Gene Ontology), which was derived from the website of the Molecular Signatures Database (MSigDB, https://www.gsea-msigdb.org/gsea/index.jsp). It is generally accepted that the threshold of significant enrichment is a false discovery rate (FDR) <0.25 and a P adjusted value <0.05.
Immune cell infiltration analysis and TME
The immune cell infiltration analysis was largely performed using the Tumor Immune Estimation Resource (TIMER) (38). The TPGS2-associated immune cell infiltration correlations were downloaded from the TIMER 2.0 database (http://timer.cistrome.org/). Finally, we visualized the statistical Spearman correlations between TPGS2 messenger RNA (mRNA) expression and 20 immune cell subsets.
Correlation analysis of the TMB, MSI, and immune regulators
A Spearman correlation analysis of immune regulators and TPGS2 expression was performed to investigate the correlation between TPGS2 and the reported biomarkers of cancer immunotherapy, including immunostimulators, immunoinhibitors, the major histocompatibility complex (MHC) genes, chemokines, and chemokine receptors, for various cancer types. A Spearman correlation analysis was also conducted to analyze the relationship between the tumor mutational burden (TMB) (39), microsatellite instability (MSI), and TPGS2 expression (40) across various cancers.
Statistical analysis
The Wilcoxon rank-sum test was used to evaluate the statistical significance of and compare the TPGS2 expression levels between tumor and normal tissues. The survival analysis was performed using the Kaplan-Meier method (log-rank test) and a univariate Cox regression analysis was also conducted. A Spearman correlation analysis was conducted to evaluate the correlations between TPGS2 and other factors, such as immune cell infiltration, the TMB, and MSI. A P value <0.05 was considered statistically significant.
Results
Expression of TPGS2 in cancer tissues
First, we integrated the mRNA expression levels of normal tissues in the GTEx database. The results showed that TPGS2 was highly expressed in the normal tissues of TGCT, BLCA, and OV, and most lowly expressed in LIHC (Figure 1A). According to the tumor cell data from the CCLE, compared to the other tumor cells, TPGS2 was the most highly expressed in small cell lung cancer and CESC, and the most lowly expressed in chronic lymphocytic leukemia (Figure 1B). We then combined the data from TCGA and GTEx databases to reflect the expression levels of TPGS2 mRNA in various malignancies (Figure 1C). The results showed that TPGS2 mRNA was more highly expressed in 22 kinds of tumors (BRCA, CESC, CHOL, COAD, DLBC, ESCA, GBM, HNSC, LGG, LIHC, LUAD, LUSC, OV, PAAD, PCPG, READ, SKCM, STAD, THCA, THYM, UCEC, and UCS) than their respective normal tissues. Conversely, TPGS2 was more lowly expressed in five tumors (ACC, KIRC, LAML, PRAD, and TGCT) than their normal tissues. Further, TPGS2 mRNA was significantly more highly expressed in BLCA, CHOL, COAD, ESCA, HNSC, LIHC, LUSC, STAD, and UCEC cancer tissues than matched normal tissues, and more highly expressed in KICH and PRAD tumor tissues (Figure 1D).
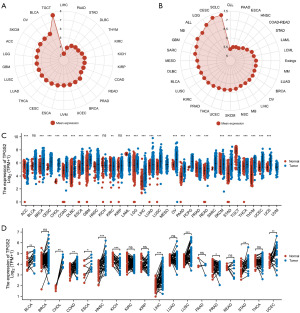
Moreover, we evaluated the protein expression of TPGS2 between normal and tumor tissues using the HPA database. As Figure 2 shows, compared to the weak staining of TPGS2 in the normal liver, lung, colon, and stomach tissues, stronger staining was observed in the LIHC, LUSC, COAD, and STAD tissues (Figure 2A-2D). Normal prostate and testes tissues had medium TPGS2 staining, while their tumor tissues had weaker staining (Figure 2E,2F). Thus, the immunohistochemistry (IHC) results re-confirmed our previous analyses. These results indicated that TPGS2 is aberrantly expressed across human cancers, and we speculated that TPGS2 may be able to inform the prognosis and treatment of various cancers.
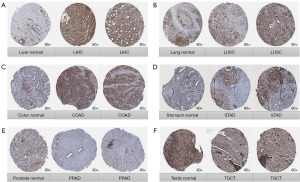
Association with molecular and immune subtypes
To explore the associations between TPGS2 expression and molecular and immune subtypes across human cancers, we performed a further analysis by the tumor-immune system interaction database (TISDB). As Figure 3A-3F show, TPGS2 expression was significantly associated with the molecular stages of many cancers, such as BRCA, COAD, HNSC, LGG, LUSC, and PCPG. To explore the relationship between TPGS2 and cancer immunity, we analyzed the correlation between the immune subtypes and TPGS2 expression, and found that the expression of TPGS2 was significantly related to immune subtypes in many cancers, including BRCA, KIRC, LIHC, STAD, OV, and SARC (Figure 3G-3L). These results indicated that TPGS2 has potential prediction and treatment functions in pan-cancer.
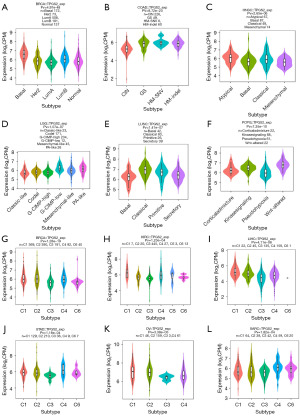
Genetic alteration of TPGS2
Given the abnormal expression of TPGS2 observed in cancer, we sought to examine whether genetic alterations in TPGS2 caused this change. Therefore, we conducted a genetic alteration analysis of TPGS2 using the cBioPortal database with data from TCGA, and PanCancer Atlas. As Figure 4A shows, PAAD had the highest alteration rate (6%) with “amplification” and “deep deletion” as the primary types. Conversely, the “deep deletion” type of the CNAs was the primary altered type in the ESAD cases, which had an alteration frequency of ~4%. Notably, the main genetic alteration in UCEC and SKCM was “mutation” (Figure 4A). As Figure 4B shows, after examining putative CNAs from the significant targets in cancer (GISTIC) module, the CNAs were closely related to the mRNA expression of TPGS2.
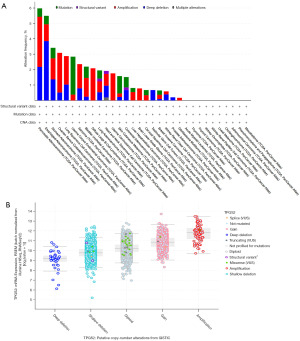
Clinical prognostic significance of TPGS2
To examine the prognostic role of TPGS2 across human cancers, prognostic indicators in 33 cancers were investigated using the Kaplan-Meier method and a univariate Cox regression analysis. The results showed that TPGS2 was highly correlated with the prognosis of most cancers. As Figure 5A shows, the univariate Cox regression analysis of OS suggested that the high expression of TPGS2 was a risk factor for the poor prognosis of ACC, BLCA, LGG, LIHC, MESO, SARC, STAD, and UCS, while it was a protective factor for OV and THYM. The results of the Kaplan-Meier OS analysis, which were basically consistent with the results of the univariate Cox regression analysis, showed that the high expression of TPGS2 was significantly correlated with a poor prognosis in SARC, MESO, LIHC, LGG, BLCA, and ACC, but was significantly correlated with a better prognosis in OV and THYM (Figure 5B-5J). The results of the Cox regression analysis of PFS revealed that TPGS2 was a risk factor in ACC, HNSC, MESO, and UCS (Figure 6A). The results of the Kaplan-Meier analysis showed that patients with high TPGS2 expression had poorer PFS than those with low TPGS2 expression in ACC, CHOL, HNSC, LIHC, SARC, and UCS, but had better PFS in PCPG, LUCA (Figure 6B-6I). Additionally, the results of the Cox regression analysis of DSS revealed that TPGS2 acts as a risk factor for ACC, LGG, LIHC, MESO, PAAD, and SARC, but acts as a protective factor for OV (Figure 7A). Further, the results of the Kaplan-Meier analysis of DSS showed that a high expression of TPGS2 was associated with a worse prognosis in ACC, LGG, LIHC, MESO, PAAD, and SARC (Figure 7B-7H). Overall, TPGS2 expression was significantly associated with prognostic parameters in many cancers.
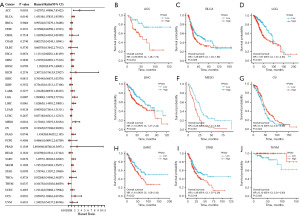
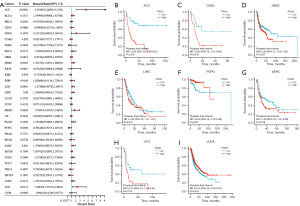
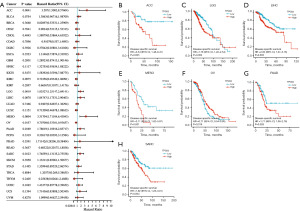
TPGS2 expression patterns in single-cell analyses and related-functional status
Due to the complexity of tumor cells, the new technique of single-cell transcriptome sequencing is increasingly being used to analyze a variety of cancer cells, immune cells, endothelial cells, and stromal cells. To explore the expression of TPGS2 in single-cell analyses in pan-cancer and its relationship with tumor functional status, we obtained tumor single-cell data on TPGS2 from the CancerSEA.
As Figure 8A shows, we found that many types of cancers, including UM, non-small cell lung cancer (NSCLC), and high-grade glioma (HGG), were associated with most tumor functional states. We also analyzed expression distribution with t-SNE plots, and the correlation between TPGS2 expression and functional states in different cancers based on the CancerSEA database, and found that TPGS2 expression in UM was significantly associated with DNA repair, DNA damage, apoptosis, metastasis, invasion, and quiescence (Figure 8B); acute myelocytic leukemia (AML) was closely associated with invasion and differentiation (Figure 8C); retinoblastoma (RB) was correlated with differentiation and angiogenesis (Figure 8D); chronic myeloid leukemia (CML) was closely associated with proliferation (Figure 8E); and NSCLC was associated with epithelial-to-mesenchymal transition (Figure 8F). The t-SNE plots showed the expression distribution of TPGS2 in UM, AML, RB, CML and NSCLC cells (Figure 8G-8K). In the plots, every point represents a single cell, and the color of the point represents the expression level of TPGS2 in the cell. The results suggest TPGS2 might play an important role in some functional states, and most of the functional states have been linked to the occurrence and progression of cancers.
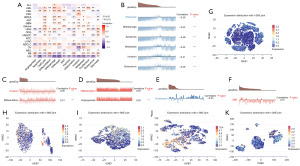
GSEA
To explore the biological processes related to TPGS2 expression across human cancers, we performed a differential expression analysis between the top 50% TPGS2 expression subgroup and the bottom TPGS2 expression subgroup for each type of cancer. We conducted a GSEA to evaluate the biological processes of 33 types of cancers from TCGA. The results showed that TPGS2 is likely involved in a great deal of immune regulation-related biological processes, especially immunoglobulin (Ig) production, the humoral immune response mediated by circulating Ig, B cell-mediated immunity, the regulation of the humoral immune response, and the B cell receptor signaling pathway (Figure 9A-9F). This provides evidence that TPGS2 may be involved in the immune response and cancers, which prompted us to explore the role of TPGS2 in the cancer-immune process and immune microenvironment.
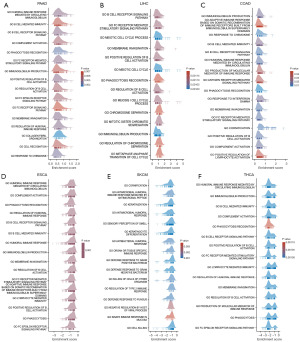
Immune cell infiltration analysis
To further explore the role of TPGS2 in tumor immunity, we explored the correlations between TPGS2 expression and immune cell infiltration levels across cancers. TIMER 2.0 was used to generate a heatmap associated with a variety of immune cell infiltration, which was performed on a variety of quantitative immuno-infiltration platforms. The results were then categorized based on different immune score categories (Figures S1-S6). These analyses showed the infiltration levels of cluster of differentiation (CD)8+ T cells, CD4+ T cells, T regulatory cells (Tregs), B cells, neutrophils, macrophages, progenitors, dendritic cells (DCs), mast cells, cancer-associated fibroblasts (CAFs), endothelial cell (endos), natural killer (NK) cells, T cell follicular helper (Tfh) cells, T cell gamma delta (γδT) cells, NK T cells, monocytes, myeloid-derived suppressor cells (MDSCs), and eosinophils (Eos). As Figure 10 shows, TPGS2 was positively related to the level of many cells, such as macrophages, CD4+ T cells, CD8+ T cells, B cells, neutrophils, and CAFs. We also found that TPGS2 had a higher correlation with immune cell infiltration in THYM and HNSC than other tumors, especially in CD4+ T cells, CD8+ T cells, macrophages, and B cells. However, their trend of correlation was slightly different, which might be related to the immune specificity of these cancers.
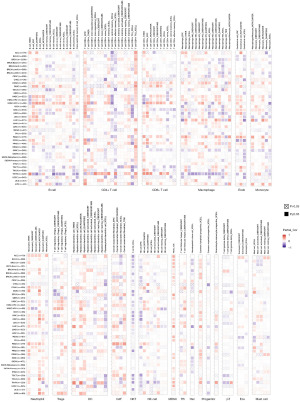
Co-expression of TPGS2 with immune-associated genes
To further explore the role of TPGS2 in cancer immunity, we performed a Spearman correlation analysis to reveal the correlation between TPGS2 expression and immune-related genes (Figure 11A-11E). The heatmaps illustrated that the gene encoding immunoinhibitor, immunostimulator, MHC, chemokine, and chemokine receptor proteins were significantly correlated with the expression of TPGS2 in most cancers. The correlation analyses revealed strong connections between TPGS2 and specific cancer types, such as HNSC, LIHC, PAAD, PRAD, SARC, THYM, and UM. Additionally, TPGS2 was positively correlated with most of the immunomodulatory factors in COAD, HNSC, KIRP, LIHC, PAAD, PRAD, READ, and UVM, but negatively correlated with ESCA and SARC.
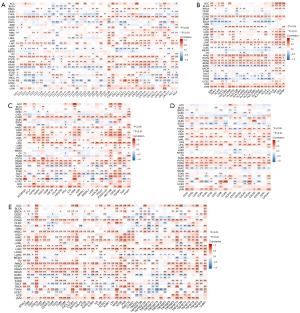
TMB and MSI analysis
The TMB and MSI are two well-known biomarkers that predict immune therapy responses across different cancers (41,42). Most scholars believe that patients with a high TMB and MSI have increased response rates to immunotherapy and display better outcomes to immunotherapy treatments. Therefore, we assessed the correlation with TMB and MSI to evaluate the efficacy of TPGS2 in predicting ICIs therapy outcomes in pan-cancer. As Figure 12 shows, TPGS2 expression was positively correlated with the TMB in BRCA, BLCA, ACC, SKCM, STAD, and COAD, and TPGS2 expression was negatively correlated with the TMB in ESCA, KIRC, THCA, and THYM (Figure 12A). Additionally, TPGS2 expression was positively correlated with MSI in BRCA, COAD, and STAD, and TPGS2 expression was negatively correlated with MSI in DLBC and PCPG (Figure 12B). Thus, our analyses indicate that TPGS2 could have a potential role in predicting the effectiveness of ICIs in a number of cancers.
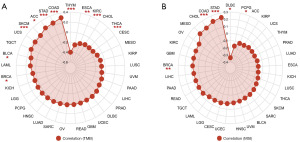
Discussion
Currently, many immune checkpoint molecules have been applied to pharmacotherapy, such as CTLA-4, PD-1, PD-L1, T cell immunoreceptors with Ig and immunoreceptor tyrosine-based inhibitory motif domains (TIGIT), and lymphocyte activating 3 (43-47). CTLA-4 and PD-L1/PD-1 have received the greatest attention; thus, anti-PD-1/PD-L1 and anti-CTLA-4 techniques have been applied to the immunotherapy of a number of cancers (46). However, at present, the most serious challenges for such treatments are related to their inapparent efficacy and side effects (48). As a result, there is a pressing need to investigate novel immunological checkpoints and methods to estimate the effects of cancer immunotherapy (48,49). Our results showed that TPGS2 is a promising biomarker of cancer, which provides a vital clue for further research on the potential role of TPGS2 in prognosis and tumor immunity.
The analysis of TPGS2 expression based on the GTEx and TCGA databases revealed that TPGS2 was abnormally upregulated in 22 cancers and downregulated in five cancers (Figure 1A). Moreover, the expression of TPGS2 was notably downregulated in TGCT tissue compared with normal testicular tissue. Data about RNA was used to cluster genes based on their expression in single-cell types. Based on the single-cell type expression cluster from HPA, we found that TPGS2 was mainly expressed in spermatids. Thus, we speculate that the specific expression in normal testicular tissue is connected with the lower expression of TPGS2 in TGCT. However, it is not yet known why TPGS2 is unevenly expressed across cancers.
Next, the IHC results from the HPA were consistent with our preliminary conclusions (Figure 2). According to the analysis of the association between TPGS2 and various subtypes, TPGS2 may be associated with molecular and immune subtypes across human cancers (Figure 3). This suggests that TPGS2 can be used to differentiate among molecular and immune types of tumors. Since most genetic alteration proportions of TPGS2 in cancers are less than 5%, there appears to be no significant correlation between TPGS2 expression and genomic alterations (Figure 4A).
In addition, we evaluated the clinical prognosis of patients who were grouped according to TPGS2 expression levels. There were differences among the various survival measures (i.e., OS, PFI, and DSS); however, the expression of TPGS2 was still significantly associated with survival (Figures 5-7). According to the Kaplan-Meier and univariate Cox regression analyses, the upregulated expression of TPGS2 was associated with a poor prognosis in patients with SARC, LIHC, and LGG, while the high expression of TPGS2 was associated with a better OS prognosis in patients with OV and THYM. Thus, TPGS2 is likely to be an important biomarker for predicting the prognosis of cancer patients.
The single-cell analysis showed that TPGS2 expression was associated with a number of functional states, including the cell cycle, metastasis, invasion, inflammation, DNA damage, and stemness, in various cancers (Figure 8). These results suggest that TPGS2 is associated with multiple cancer functional states in many human cancers.
According to the GSEA, TPGS2 was closely related to some immune response processes, such as Ig production, B cell-mediated immunity, the humoral immune response mediated by circulating Ig, the regulation of the humoral immune response, and the B cell receptor signaling pathway (Figure 9). Therefore, it is very likely that TPGS2 is involved in the functions related to B cells.
Recently, the importance of B cells has been found in tumor immunity. Since 2020, three research teams from the United States, France, and Sweden have analyzed a large sample of clinical cohort studies, and reported a positive correlation between B-cell infiltration and the formation of TLSs and the response to immunotherapy in a variety of cancer types (50-52). B cells also express a number of checkpoint molecules, including PD-1, PD-L1/2, and CTLA-4B (15). Patients who responded to ICIs therapy were reported that more memory B cells, C-X-C motif chemokine receptor 3+ cells, and germinal center-like B cells were found in their TMEs than patients who did not response to ICIs therapy (52).
In addition, studies (21,53) have shown that the Igs in many antibody-secreting B cells, which mainly secrete IgG and IgA, are tumor-dependent. These Igs are correlated with the tumorigenesis site, and higher proportions of IgG have been observed in thyroid, testicular, and skin tumors, while higher proportions of IgA been observed in kidney, ovarian, pancreatic, and colorectal cancers. This is consistent with our GSEA finding that a higher number of Ig-related biological states were enriched.
Another important finding of our research is that TPGS2 plays a pivotal role in cancer immunity. In recent years, many studies have shown that the immune status of cancers is closely correlated to the cell composition of and infiltration concentration around the tumor (54-56). TPGS2 was found to be positively correlated with the infiltrating levels of multiple immune cells, such as macrophages, CD4+ T cells, CD8+ T cells, B cells, and neutrophils (Figure 10), which suggests that TPGS2 is likely to influence development and prognosis of various cancers by affecting the TME.
Pro-inflammatory mediators, including chemokines, cytokines, and prostaglandins, have been found in the TME, which affects tumor initiation, progression, and metastasis (57-59). Our analyses of TPGS2 expression and pan-cancer immunomodulatory factors suggested that TPGS2 is co-expressed with genes that encode the immunoinhibitor, immunostimulator, MHC, chemokine, and chemokine receptor proteins, especially in THYM and HNSC (Figure 11). These results suggest that TPGS2 is likely to be involved in the progression and prognosis of cancers by interacting with the TME.
Further, the TMB is a promising prognostic and predictive biomarker for immunotherapy in human cancers (60-63). Research has demonstrated that patients, suffering from melanoma (64,65) or urothelial carcinoma (66,67) with a high TMB achieve better clinical outcomes from ICIs. Similarly, MSI also plays a vital role as a predictive biomarker for tumor immunotherapy (68). The Food and Drug Administration has authorized MSI-high status or deficient mismatch repair as prognostic biomarkers for directing the therapeutic application of ICIs in certain malignancies (69). According to our analyses, the TMB in 10 types of cancers and MSI in five types of cancers were significantly correlated with the expression of TPGS2. Thus, TPGS2 is likely to act as a predictor of the efficacy of immunotherapy in many cancers.
Wang’s study demonstrated that the cir-TPGS2 (derived from TPGS2)–related axis promoted breast cancer cell motility by the TME (30). Another study demonstrated that TPGS2 could be a potential gene in renal cell carcinoma (29). Together with our findings, such results suggest that TPGS2 could serve as a potential biomarker in anti-tumor immunity treatments.
However, our research had a number of limitations. First, while we showed that TPGS2 is a promising predictor of prognosis and immunotherapy in many cancers, the mechanism by which this occurs remains unknown, and we have no evidence of any direct interaction. Second, our data were mainly obtained from open databases, and no clinical cohort was used for verification, which inevitably led to various biases and decreased the credibility of the results. Third, TPGS2 has rarely been studied in human tumors, and in-depth studies need to be conducted to verify its role in cancer prognosis prediction and immunotherapy. Fourth, our research revealed a promising direction for tumor research in TPGS2; however, this study was a descriptive study based on bioinformatics, and the mechanism related to both TPGS2 and the development and therapy of specific tumors need to be further explored, and experimental verification in vitro and in vivo is required. In the future, we intend to conduct further experiments to examine the mechanism of TPGS2 across human cancers.
Conclusions
In summary, we performed a comprehensive pan-cancer analysis that demonstrated that the aberrant expression of TPGS2 was associated with the prognosis, immune cell infiltration, TME, some immune response processes, and various function states across human cancers. Thus, TPGS2 could serve not only as a biomarker for predicting clinical outcomes, but also as a promising biomarker for evaluating and developing new approaches to immunotherapy in many types of cancers, especially COAD and STAD.
Acknowledgments
We greatly appreciate the TCGA database for providing useful data, as well as bioinformatics tools for data analyses.
Funding: This work was supported by
Footnote
Reporting Checklist: The authors have completed the REMARK reporting checklist. Available at https://tcr.amegroups.com/article/view/10.21037/tcr-23-113/rc
Peer Review File: Available at https://tcr.amegroups.com/article/view/10.21037/tcr-23-113/prf
Conflicts of Interest: All authors have completed the ICMJE uniform disclosure form (available at https://tcr.amegroups.com/article/view/10.21037/tcr-23-113/coif). The authors have no conflicts of interest to declare.
Ethical Statement: The authors are accountable for all aspects of the work in ensuring that questions related to the accuracy or integrity of any part of the work are appropriately investigated and resolved. The study was conducted in accordance with the Declaration of Helsinki (as revised in 2013).
Open Access Statement: This is an Open Access article distributed in accordance with the Creative Commons Attribution-NonCommercial-NoDerivs 4.0 International License (CC BY-NC-ND 4.0), which permits the non-commercial replication and distribution of the article with the strict proviso that no changes or edits are made and the original work is properly cited (including links to both the formal publication through the relevant DOI and the license). See: https://creativecommons.org/licenses/by-nc-nd/4.0/.
References
- Miller KD, Ortiz AP, Pinheiro PS, et al. Cancer statistics for the US Hispanic/Latino population, 2021. CA Cancer J Clin 2021;71:466-87. [Crossref] [PubMed]
- Sung H, Ferlay J, Siegel RL, et al. Global Cancer Statistics 2020: GLOBOCAN Estimates of Incidence and Mortality Worldwide for 36 Cancers in 185 Countries. CA Cancer J Clin 2021;71:209-49. [Crossref] [PubMed]
- Siegel RL, Miller KD, Jemal A. Cancer statistics, 2019. CA Cancer J Clin 2019;69:7-34. [Crossref] [PubMed]
- Urbanek-Trzeciak MO, Galka-Marciniak P, Nawrocka PM, et al. Pan-cancer analysis of somatic mutations in miRNA genes. EBioMedicine 2020;61:103051. [Crossref] [PubMed]
- O'Donnell JS, Teng MWL, Smyth MJ. Cancer immunoediting and resistance to T cell-based immunotherapy. Nat Rev Clin Oncol 2019;16:151-67. [Crossref] [PubMed]
- Schoepp M, Ströse AJ, Haier J. Dysregulation of miRNA Expression in Cancer Associated Fibroblasts (CAFs) and Its Consequences on the Tumor Microenvironment. Cancers (Basel) 2017;9:54. [Crossref] [PubMed]
- Abbott M, Ustoyev Y. Cancer and the Immune System: The History and Background of Immunotherapy. Semin Oncol Nurs 2019;35:150923. [Crossref] [PubMed]
- Kennedy LB, Salama AKS. A review of cancer immunotherapy toxicity. CA Cancer J Clin 2020;70:86-104. [Crossref] [PubMed]
- Herbst RS, Giaccone G, de Marinis F, et al. Atezolizumab for First-Line Treatment of PD-L1-Selected Patients with NSCLC. N Engl J Med 2020;383:1328-39. [Crossref] [PubMed]
- Del Prete A, Schioppa T, Tiberio L, et al. Leukocyte trafficking in tumor microenvironment. Curr Opin Pharmacol 2017;35:40-7. [Crossref] [PubMed]
- Arneth B. Tumor Microenvironment. Medicina (Kaunas) 2019;56:15. [Crossref] [PubMed]
- Erin N, Grahovac J, Brozovic A, et al. Tumor microenvironment and epithelial mesenchymal transition as targets to overcome tumor multidrug resistance. Drug Resist Updat 2020;53:100715. [Crossref] [PubMed]
- de Miguel M, Calvo E. Clinical Challenges of Immune Checkpoint Inhibitors. Cancer Cell 2020;38:326-33. [Crossref] [PubMed]
- Ye W, Olsson-Brown A, Watson RA, et al. Checkpoint-blocker-induced autoimmunity is associated with favourable outcome in metastatic melanoma and distinct T-cell expression profiles. Br J Cancer 2021;124:1661-9. [Crossref] [PubMed]
- Downs-Canner SM, Meier J, Vincent BG, et al. B Cell Function in the Tumor Microenvironment. Annu Rev Immunol 2022;40:169-93. [Crossref] [PubMed]
- Hu Q, Hong Y, Qi P, et al. Atlas of breast cancer infiltrated B-lymphocytes revealed by paired single-cell RNA-sequencing and antigen receptor profiling. Nat Commun 2021;12:2186. [Crossref] [PubMed]
- Biswas S, Mandal G, Payne KK, et al. IgA transcytosis and antigen recognition govern ovarian cancer immunity. Nature 2021;591:464-70. [Crossref] [PubMed]
- Griss J, Bauer W, Wagner C, et al. B cells sustain inflammation and predict response to immune checkpoint blockade in human melanoma. Nat Commun 2019;10:4186. [Crossref] [PubMed]
- Selitsky SR, Mose LE, Smith CC, et al. Prognostic value of B cells in cutaneous melanoma. Genome Med 2019;11:36. [Crossref] [PubMed]
- Wieland A, Patel MR, Cardenas MA, et al. Defining HPV-specific B cell responses in patients with head and neck cancer. Nature 2021;597:274-8. [Crossref] [PubMed]
- Sharonov GV, Serebrovskaya EO, Yuzhakova DV, et al. B cells, plasma cells and antibody repertoires in the tumour microenvironment. Nat Rev Immunol 2020;20:294-307. [Crossref] [PubMed]
- Cao J, Yu C. Identification of Immune Infiltration and Prognostic Biomarkers in Small Cell Lung Cancer Based on Bioinformatic Methods from 3 Studies. Comb Chem High Throughput Screen 2023;26:507-16. [Crossref] [PubMed]
- Chen Y, He F, Wang R, et al. NCF1/2/4 Are Prognostic Biomarkers Related to the Immune Infiltration of Kidney Renal Clear Cell Carcinoma. Biomed Res Int 2021;2021:5954036. [Crossref] [PubMed]
- Li M, Chen P, Zhao Y, et al. Immune Infiltration Represents Potential Diagnostic and Prognostic Biomarkers for Esophageal Squamous Cell Carcinoma. Biomed Res Int 2022;2022:9009269. [Crossref] [PubMed]
- Li M, Ren H, Zhang Y, et al. MECOM/PRDM3 and PRDM16 Serve as Prognostic-Related Biomarkers and Are Correlated With Immune Cell Infiltration in Lung Adenocarcinoma. Front Oncol 2022;12:772686. [Crossref] [PubMed]
- Tao D, Wang Y, Zhang X, et al. Identification of Angiogenesis-Related Prognostic Biomarkers Associated With Immune Cell Infiltration in Breast Cancer. Front Cell Dev Biol 2022;10:853324. [Crossref] [PubMed]
- Wang YY, Shi LY, Xu MH, et al. A pan-cancer analysis of the expression of gasdermin genes in tumors and their relationship with the immune microenvironment. Transl Cancer Res 2021;10:4125-47. [Crossref] [PubMed]
- Xu F, Cui WQ, Liu C, et al. Prognostic biomarkers correlated with immune infiltration in non-small cell lung cancer. FEBS Open Bio 2023;13:72-88. [Crossref] [PubMed]
- Khouja HI, Ashankyty IM, Bajrai LH, et al. Multi-staged gene expression profiling reveals potential genes and the critical pathways in kidney cancer. Sci Rep 2022;12:7240. [Crossref] [PubMed]
- Wang S, Feng X, Wang Y, et al. Dysregulation of tumour microenvironment driven by circ-TPGS2/miR-7/TRAF6/NF-κB axis facilitates breast cancer cell motility. Autoimmunity 2021;54:284-93. [Crossref] [PubMed]
- Goldman MJ, Craft B, Hastie M, et al. Visualizing and interpreting cancer genomics data via the Xena platform. Nat Biotechnol 2020;38:675-8. [Crossref] [PubMed]
- Colwill K. Gräslund S. A roadmap to generate renewable protein binders to the human proteome. Nat Methods 2011;8:551-8. [Crossref] [PubMed]
- Haeussler M, Zweig AS, Tyner C, et al. The UCSC Genome Browser database: 2019 update. Nucleic Acids Res 2019;47:D853-8. [Crossref] [PubMed]
- Gao J, Aksoy BA, Dogrusoz U, et al. Integrative analysis of complex cancer genomics and clinical profiles using the cBioPortal. Sci Signal 2013;6:pl1. [Crossref] [PubMed]
- Yuan H, Yan M, Zhang G, et al. CancerSEA: a cancer single-cell state atlas. Nucleic Acids Res 2019;47:D900-8. [Crossref] [PubMed]
- Yu G, Wang LG, Han Y, et al. clusterProfiler: an R package for comparing biological themes among gene clusters. OMICS 2012;16:284-7. [Crossref] [PubMed]
- Subramanian A, Tamayo P, Mootha VK, et al. Gene set enrichment analysis: a knowledge-based approach for interpreting genome-wide expression profiles. Proc Natl Acad Sci U S A 2005;102:15545-50. [Crossref] [PubMed]
- Li T, Fu J, Zeng Z, et al. TIMER2.0 for analysis of tumor-infiltrating immune cells. Nucleic Acids Res 2020;48:W509-14. [Crossref] [PubMed]
- Thorsson V, Gibbs DL, Brown SD, et al. The Immune Landscape of Cancer. Immunity 2018;48:812-830.e14. [Crossref] [PubMed]
- Bonneville R, Krook MA, Kautto EA, et al. Landscape of Microsatellite Instability Across 39 Cancer Types. JCO Precis Oncol 2017;2017:PO.17.00073.
- Zhao P, Li L, Jiang X, et al. Mismatch repair deficiency/microsatellite instability-high as a predictor for anti-PD-1/PD-L1 immunotherapy efficacy. J Hematol Oncol 2019;12:54. [Crossref] [PubMed]
- Chan TA, Yarchoan M, Jaffee E, et al. Development of tumor mutation burden as an immunotherapy biomarker: utility for the oncology clinic. Ann Oncol 2019;30:44-56. [Crossref] [PubMed]
- Lee J, Choi Y, Jung HA, et al. Outstanding clinical efficacy of PD-1/PD-L1 inhibitors for pulmonary pleomorphic carcinoma. Eur J Cancer 2020;132:150-8. [Crossref] [PubMed]
- Okazaki T, Chikuma S, Iwai Y, et al. A rheostat for immune responses: the unique properties of PD-1 and their advantages for clinical application. Nat Immunol 2013;14:1212-8. [Crossref] [PubMed]
- Mayes PA, Hance KW, Hoos A. The promise and challenges of immune agonist antibody development in cancer. Nat Rev Drug Discov 2018;17:509-27. [Crossref] [PubMed]
- Qin S, Xu L, Yi M, et al. Novel immune checkpoint targets: moving beyond PD-1 and CTLA-4. Mol Cancer 2019;18:155. [Crossref] [PubMed]
- Rotte A, Jin JY, Lemaire V. Mechanistic overview of immune checkpoints to support the rational design of their combinations in cancer immunotherapy. Ann Oncol 2018;29:71-83. [Crossref] [PubMed]
- Naidoo J, Page DB, Li BT, et al. Toxicities of the anti-PD-1 and anti-PD-L1 immune checkpoint antibodies. Ann Oncol 2015;26:2375-91. [Crossref] [PubMed]
- Ramos-Casals M, Brahmer JR, Callahan MK, et al. Immune-related adverse events of checkpoint inhibitors. Nat Rev Dis Primers 2020;6:38. [Crossref] [PubMed]
- Petitprez F, de Reyniès A, Keung EZ, et al. B cells are associated with survival and immunotherapy response in sarcoma. Nature 2020;577:556-60. [Crossref] [PubMed]
- Cabrita R, Lauss M, Sanna A, et al. Tertiary lymphoid structures improve immunotherapy and survival in melanoma. Nature 2020;577:561-5. [Crossref] [PubMed]
- Helmink BA, Reddy SM, Gao J, et al. B cells and tertiary lymphoid structures promote immunotherapy response. Nature 2020;577:549-55. [Crossref] [PubMed]
- Pavoni E, Monteriù G, Santapaola D, et al. Tumor-infiltrating B lymphocytes as an efficient source of highly specific immunoglobulins recognizing tumor cells. BMC Biotechnol 2007;7:70. [Crossref] [PubMed]
- Gong Z, Zhang J, Guo W. Tumor purity as a prognosis and immunotherapy relevant feature in gastric cancer. Cancer Med 2020;9:9052-63. [Crossref] [PubMed]
- Chen M, Ren R, Lin W, et al. Exploring the oncostatin M (OSM) feed-forward signaling of glioblastoma via STAT3 in pan-cancer analysis. Cancer Cell Int 2021;21:565. [Crossref] [PubMed]
- Shiao SL, Chu GC, Chung LW. Regulation of prostate cancer progression by the tumor microenvironment. Cancer Lett 2016;380:340-8. [Crossref] [PubMed]
- Crusz SM, Balkwill FR. Inflammation and cancer: advances and new agents. Nat Rev Clin Oncol 2015;12:584-96. [Crossref] [PubMed]
- Mantovani A, Allavena P, Sica A, et al. Cancer-related inflammation. Nature 2008;454:436-44. [Crossref] [PubMed]
- Galdiero MR, Marone G, Mantovani A. Cancer Inflammation and Cytokines. Cold Spring Harb Perspect Biol 2018;10:a028662. [Crossref] [PubMed]
- Powles T, Durán I, van der Heijden MS, et al. Atezolizumab versus chemotherapy in patients with platinum-treated locally advanced or metastatic urothelial carcinoma (IMvigor211): a multicentre, open-label, phase 3 randomised controlled trial. Lancet 2018;391:748-57. [Crossref] [PubMed]
- Marabelle A, Fakih M, Lopez J, et al. Association of tumour mutational burden with outcomes in patients with advanced solid tumours treated with pembrolizumab: prospective biomarker analysis of the multicohort, open-label, phase 2 KEYNOTE-158 study. Lancet Oncol 2020;21:1353-65. [Crossref] [PubMed]
- Hellmann MD, Ciuleanu TE, Pluzanski A, et al. Nivolumab plus Ipilimumab in Lung Cancer with a High Tumor Mutational Burden. N Engl J Med 2018;378:2093-104. [Crossref] [PubMed]
- Rizvi NA, Hellmann MD, Snyder A, et al. Cancer immunology. Mutational landscape determines sensitivity to PD-1 blockade in non-small cell lung cancer. Science 2015;348:124-8. [Crossref] [PubMed]
- Chan TA, Wolchok JD, Snyder A. Genetic Basis for Clinical Response to CTLA-4 Blockade in Melanoma. N Engl J Med 2015;373:1984. [Crossref] [PubMed]
- Riaz N, Havel JJ, Makarov V, et al. Tumor and Microenvironment Evolution during Immunotherapy with Nivolumab. Cell 2017;171:934-949.e16. [Crossref] [PubMed]
- Snyder A, Nathanson T, Funt SA, et al. Contribution of systemic and somatic factors to clinical response and resistance to PD-L1 blockade in urothelial cancer: An exploratory multi-omic analysis. PLoS Med 2017;14:e1002309. [Crossref] [PubMed]
- Balar AV, Galsky MD, Rosenberg JE, et al. Atezolizumab as first-line treatment in cisplatin-ineligible patients with locally advanced and metastatic urothelial carcinoma: a single-arm, multicentre, phase 2 trial. Lancet 2017;389:67-76. [Crossref] [PubMed]
- Wang QX, Qu CH, Gao YH, et al. The degree of microsatellite instability predicts response to PD-1 blockade immunotherapy in mismatch repair-deficient/microsatellite instability-high colorectal cancers. Exp Hematol Oncol 2021;10:2. [Crossref] [PubMed]
- Li R, Han D, Shi J, et al. Choosing tumor mutational burden wisely for immunotherapy: A hard road to explore. Biochim Biophys Acta Rev Cancer 2020;1874:188420. [Crossref] [PubMed]