Gastric cancer prognosis: unveiling autophagy-related signatures and immune infiltrates
Highlight box
Key findings
• Cathepsin B (CTSB) expression was elevated in gastric cancer.
• CTSB may be a key gene related to survival and prognosis in gastric cancer.
What is known and what is new?
• CTSB was a gene related to autophagy.
• CTSB may be a key gene in the treatment of gastric cancer.
What is the implication, and what should change now?
• CTSB may be a new immunotherapy target for gastric cancer.
• Further experiments are needed to validate the conclusion.
Introduction
Gastric cancer was a widely known type of cancer that had become a global health concern. It had four subtypes: adenocarcinoma, squamous cell carcinoma, adenosquamous carcinoma, and signet-ring cell carcinoma. Adenocarcinoma was the most common type and the fifth most widespread type of cancer. It was also the third leading cause of cancer-related deaths worldwide (1). Studies had shown that several factors contributed to the risk of developing gastric cancer, such as Helicobacter pylori (H. pylori) infection, age, high salt intake, and inadequate consumption of fruits and vegetables. The major cause of infection was believed to be H. pylori (2,3). There had been progress in diagnosing and treating gastric cancer in recent years. However, the 5-year survival rate for patients with stage III tumors who underwent surgery remained low, ranging from 18% to 50% (4). The mortality rate of gastric cancer has not been significantly improved. Consequently, pinpointing prospective molecular indicators and therapeutic focal points becomes imperative in the early detection, prevention, and management of gastric cancer, which contributes to the improvement of clinical results.
Autophagy was a vital metabolic process that helped maintain cellular balance by clearing out damaged cellular components through lysosome fusion. It significantly affected many cellular processes, such as cancer, development, aging, and stress responses. Dysregulation of autophagy had been linked to several human diseases, including cancer (5,6). Recent studies had highlighted the critical role of abnormal autophagy in breast cancer, non-small cell lung cancer, and liver cancer (7-9). Similar evidence suggested that autophagy also played a crucial role in the development of gastric cancer, with Beclin-1 as a key regulatory factor in this context (10). Moreover, researchers had developed predictive models using genetic information to assess prognosis and diagnose gastric cancer. One model used six genes (DYNLL1, PGK2, HPR, PLOD2, PHYHIP, and CXCR4) to predict gastric cancer outcomes (11), while another identified a risk-scoring model for overall survival based on four genes (GRID2, ATG4D, GABARAPL2, and CXCR4) (12). These findings validated the association between autophagy and gastric cancer, highlighting the potential of autophagy-related genes (ARGs) as prognostic markers for the disease. Furthermore, ARGs might emerge as promising targets for therapeutic interventions in gastric cancer.
This research focused on uncovering genes linked to autophagy and delineating pathways potentially implicated in gastric cancer. We used the Gene Expression Omnibus (GEO) dataset and the Human Autophagy Database (HADb) to screen for relevant genes to accomplish this. We also verified their expression using the Human Protein Atlas (HPA) and GSE19826 databases. Furthermore, this study explored the relationship between the expression of cathepsin B (CTSB) in gastric cancer tissues and immune cell infiltration, utilizing the Tumor Immune Estimation Resource (TIMER) and Tumor Immune System Interactions Database (TISIDB) databases. These findings offer valuable and dependable targets for treating gastric cancer. We present this article in accordance with the STREGA reporting checklist (available at https://tcr.amegroups.com/article/view/10.21037/tcr-23-1755/rc).
Methods
Acquisition of gene expression profile data
We downloaded GSE19826, GSE79973, and GSE54129 gene expression profiles from the GEO database (https://www.ncbi.nlm.nih.gov/). The GSE19826 dataset comprised 12 gastric cancer and 15 normal tissue samples. The GSE79973 dataset included 10 gastric cancer and 10 normal tissue samples. The GSE54129 dataset comprised 111 gastric cancer and 21 normal tissue samples. GSE79973 and GSE54129 were used as training sets, while GSE19826 was used for validation. Subsequently, we collected 232 ARGs from the Human Autophagy Database (http://www.autophagy.lu/index HTML). The study was conducted in accordance with the Declaration of Helsinki (as revised in 2013).
Identification of differentially expressed genes (DEGs)
In analyzing the GEO dataset using R software (version 4.3.0), we utilized the limma, tidyverse, and ggpubr packages. We selected DEGs based on the |log2FC| >0.5 criteria and adj.P.Val <0.05. These DEGs were further employed to create a volcano plot using the ggpubr package. To obtain the ARG-DEGs, we utilized the ggvenn package to generate a Venn diagram depicting the overlap between the DEGs and ARGs. This enabled the identification of genes that were both differentially expressed and associated with autophagy.
Functional enrichment analysis of autophagy related DEGs
We conducted Gene Ontology (GO) and Kyoto Encyclopedia of Genes and Genomes (KEGG) enrichment analysis on autophagy related DEGs using the clusterProfiler, org.Hs.eg.db, and tidyverse packages in R software (version 4.3.0). The value of P<0.05 was considered a significant enrichment.
Construction of protein-protein interaction (PPI) network and selection of hub genes
The STRING database (https://cn.string-db.org) was used to analyze and construct a PPI network of autophagy-related DEGs. The PPI network consisted of 15 nodes and 38 edges. Further analysis was performed using Cytoscape software to rank the autophagy related DEGs based on their degree. The top five DEGs with the highest degree were selected for further validation in subsequent studies.
The HPA
HPA, an open-access resource, permitted both academic and industrial researchers to examine the human proteome freely (13). In our research, the HPA database (http://www.proteinatlas.org/) served as a tool for verifying the protein expression of five pivotal genes, selected from both normal and tumor tissues, using immunohistochemistry.
Validation of CTSB expression
The Genomic Data Commons/The Cancer Genome Atlas-Stomach Adenocarcinoma (GDC/TCGA-STAD) transcriptome data was downloaded from the Xena website (https://xena.ucsc.edu). This dataset included 373 gastric cancer tissues and 32 normal gastric tissues samples. Then, the data analyzed and processed using R software (version 4.3.0) with the tidyverse and limma packages. The FPKM expression levels of CTSB in both gastric cancer and normal gastric tissues were extracted. The data was then imported into GraphPad Prism 9.0 for visualization. Additionally, the expression of CTSB was validated using the GSE19826 dataset, and a P value of <0.05 indicated statistical significance.
Survival analysis of CTSB in gastric cancer
In this study, the survival analysis of CTSB in gastric cancer was conducted utilizing the Kaplan-Meier plotter (http://kmplot.com/analysis).
Gene set enrichment analysis (GSEA)
Based on the RNA-seq data from the TCGA database, gene enrichment analysis was performed on CTSB using GSEA. GO terms were utilized in the GSEA analysis to explore the potential biological functions of CTSB in gastric cancer. The value of P<0.05 was considered statistically significant.
Tumor immune estimation database
TISIDB (http://cis.hku.hk/TISIDB/index.php) integrated diverse data, acting as a platform for investigating the correlation between CTSB and Spearman immune regulator’s expression, thus yielding insights into interactions within the tumor-immune system (14). TIMER (http://cistrome.shinyapps.io/timer/) reliably assessed immune infiltration levels and helped uncover the associations between immune infiltration, gene expression, mutations, and survival features in TCGA cohorts. In summary, the TIMER web server provided comprehensive analysis and visualization of tumor-infiltrating immune cells (15).
Statistical analyses
All statistical analyses were performed using R software and GraphPad Prism 9.0. The differential expression levels of CTSB in tumor versus normal samples were evaluated through a non-paired t-test.
Results
Identification of relevant DEGs in the GEO database
The filtering criteria were as follows: |log2FC| >0.5 and P<0.05. In 111 gastric cancer and 21 non-cancer tissues from the GSE54129 dataset, 2,761 downregulated DEGs and 2,724 upregulated DEGs were identified (Figure 1A). In addition, from the GSE79973 dataset, 1,689 downregulated DEGs and 2,130 upregulated DEGs were identified in 10 normal gastric and 10 gastric cancer samples (Figure 1B). Next, to illustrate the overlap of autophagy-related DEGs across both datasets, a Venn diagram was constructed using the ggvenn package in R software (version 4.3.0). As depicted in Figure 1C,1D and Table 1, 15 autophagy-related DEGs were identified.
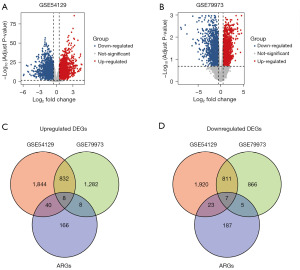
Table 1
Category | DEGs |
---|---|
Upregulated | FKBP1A, IL24, PEA15, HSP90AB1, CTSB, ITGB1, SPHK1, HIF1A |
Downregulated | DAPK2, EIF2AK3, FKBP1B, PTK6, NKX2-3, NFE2L2, PRKCD |
DEGs, differentially expressed genes.
Enrichment analysis of autophagy related DEGs
In this study, we conducted a functional enrichment analysis of these ARG-DEGs to understand the potential functions and pathways of autophagy-related DEGs in gastric cancer development. As shown in Figure 2A, we presented the top five P values for GO analysis in biological process (BP), molecular function (MF), and cellular component (CC). The BP analysis revealed the involvement of these ARGs in the regulation of apoptotic signaling pathways, response to reactive oxygen species (ROS), regulation of protein dephosphorylation, intrinsic apoptotic signaling pathway, and regulation of dephosphorylation. KEGG analysis showed that these genes were primarily associated with autophagy, protein processing in the endoplasmic reticulum (ER), nucleotide-binding oligomerization domain (NOD)-like receptor (NLR) signaling pathway, lipid and atherosclerosis, chemical carcinogenesis—ROS, mitophagy, and antigen processing and presentation (Figure 2B).
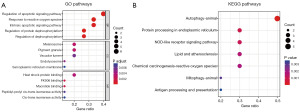
Construction of the PPI network and identification of hub genes
We constructed a PPI network of autophagy-related DEGs using protein interaction analysis based on the STRING database. As demonstrated in Figure 3A, the PPI network encompassed 15 nodes and 38 edges. Utilizing Cytoscape software for in-depth analysis, the autophagy-related DEGs were ordered by their degree values. This led to the identification of the foremost five DEGs with the highest degree values: HIF1A, CTSB, PRKCD, HSP90AB1, and EIF2AK3, classified as hub genes (Figure 3B). Among them, CTSB was selected as a potential biomarker for gastric cancer, and further validation will be conducted in subsequent studies.
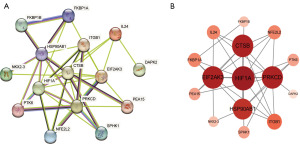
Validation of CTSB expression levels in multiple databases
The expression of CTSB mRNA in gastric cancer was detected using the TCGA database. As depicted in Figure 4A, CTSB was significantly upregulated in tumor samples compared to normal samples (P<0.0001). We also validated the mRNA expression of CTSB in gastric cancer tissues and normal tissues using the GSE19826 dataset. As shown in Figure 4B, CTSB expression was significantly upregulated in tumor samples compared to normal samples (P<0.05). Additionally, data from immunohistochemical staining in the HPA database revealed elevated protein expression of CTSB in tumor samples (Figure 4C,4D). Overall, these findings suggest the upregulation of CTSB in gastric cancer patients.
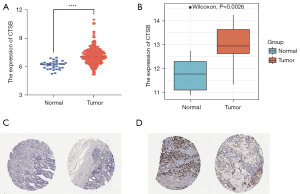
The survival analysis of CTSB and its value in the differential diagnosis of gastric cancer
In this study, we used the Kaplan-Meier plotter to analyze the overall survival of CTSB and further investigate its impact on the overall survival of gastric cancer patients. According to the results shown in Figure 5A, we found a significant correlation between high expression of CTSB and poorer overall survival in gastric cancer patients. This suggested a close association between CTSB and gastric cancer progression, making it a potential tumor biomarker for gastric cancer patients. We also conducted a receiver operating characteristic (ROC) curve analysis to evaluate the value of CTSB in the clinical diagnosis of gastric cancer. As shown in Figure 5B, the area under the curve (AUC) was 0.866, indicating that CTSB had a high value in distinguishing gastric cancer.
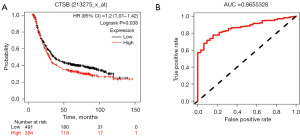
Identification of relevant pathways of CTSB in gastric cancer via GSEA
Furthermore, we utilized the TCGA database to conduct a GSEA to assess the potential functions of CTSB in the progression of gastric cancer. The reference gene set consisted of GO pathways, and significant enrichment was determined by |Normalized Enrichment Score|(|NES|) >1, P.adjust <0.05, and FDR value (q-value) <0.25. The GSEA findings demonstrated that genes associated with CTSB were significantly enriched in pathways related to immune functions. These pathways encompassed the regulation of leukocyte degranulation, the regulation of myeloid leukocyte-mediated immunity, the regulation of macrophage activation, positive regulation of cytokine production involved in immune response, antigen binding, and regulatory T cell differentiation (Figure 6A-6F). These findings strongly indicate the involvement of immune-related pathways in the development of gastric cancer. Consequently, We then delved into the relationship between CTSB expression and immune infiltration within gastric cancer.
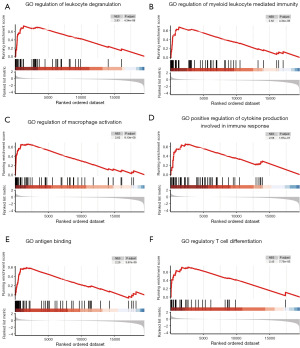
CTSB expression associated with immune-infiltrating cells in gastric cancer
Previous studies had established a close relationship between the immune system and tumor development. Therefore, in this study, we aimed to further investigate the impact of CTSB on immune factors. Utilizing the TISIDB database, we identified significant positive correlations between CTSB and immune factors, including CCL4, CSF1R, HAVCR2, IL10, PDCD1LG2, CD86, TNSRFS18, Macrophage, myeloid-derived suppressor cell (MDSC), natural killer (NK) cells, and Treg cells (Figure 7). Additionally, Within the TIMER database, the association between CTSB expression and levels of immune infiltration was examined, as shown in Figure 8A. Remarkably, CTSB expression displayed a significant positive correlation with macrophage infiltration level (P=9.71×10−7), neutrophil level (P=7.32×10−13), dendritic cells (P=1.18×10−19), and CD8+ T cell infiltration level (P=1.53×10−7). Conversely, a significant negative correlation was observed between CTSB expression and B cell infiltration level (P=2.84×10−12). Moreover, we conducted further analysis to explore the association between CTSB copy number variation and immune infiltration levels in gastric cancer. The copy number variation of CTSB exhibited significant correlations with infiltration levels of dendritic cells, macrophages, neutrophils, CD4+ T cells, CD8+ T cells, and B cells (Figure 8B). These findings collectively highlight the crucial role of CTSB in immune infiltration within gastric cancer.
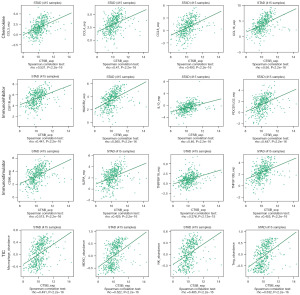
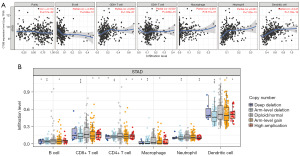
Discussion
The severity of gastric cancer was characterized by its low survival rate and high probability of mortality. In areas lacking in early detection initiatives, patients were often diagnosed in later stages due to the non-specific nature of the initial symptoms. As a result, timely detection played a critical role in improving patient outcomes. Research had indicated that early detection could reduce the mortality rate of gastric cancer by 30–65% (16,17). The most efficient way to detect and evaluate gastric cancer in its early stages was through endoscopic biopsy. However, this method had some drawbacks. It could be invasive and uncomfortable for patients, and it also required expensive equipment. Moreover, it relied on highly skilled endoscopists and patient cooperation, which could be challenging (18,19). Screening for gastric cancer on a large scale could be quite formidable using the current method. To ensure it was more attainable in the future, we must identify a cost-effective and universally applicable solution. A promising avenue to explore was uncovering an optimal biomarker.
Autophagy, a vital process for maintaining cellular homeostasis, was regulated by ARGs. There was growing evidence linking autophagy to the development of gastric cancer (20). Numerous autophagy-related proteins, including P62, Beclin 1, and LC3, were closely associated with the prognosis of gastric cancer patients (21). For example, studies had shown that the long non-coding RNA (lncRNA) CCAT1 weakened the inhibitory effect of miR-140-3p on ATG5 expression, leading to enhanced autophagy in gastric cancer cells (22). Therefore, the primary objective of this study was to identify genes associated with autophagy in gastric cancer and evaluate their influence on immune cell infiltration employing bioinformatics techniques.
In our research, we first gathered all genes from the GEO database. Subsequently, we identified 15 DEGs associated with autophagy in tumor samples: eight upregulated and seven downregulated genes. Following this, GO and KEGG enrichment analyses were conducted to explore the potential molecular mechanisms of these autophagy-related DEGs. The results of our study indicated that these genes were predominantly involved in the regulation of apoptotic signaling pathways, response to ROS, protein dephosphorylation regulation, intrinsic apoptotic signaling pathways, autophagy, protein processing in the ER, NLR signaling pathway, chemical carcinogenesis involving ROS, mitophagy, and antigen processing and presentation. These pathways may have played crucial roles in autophagy and gastric cancer pathogenesis. In previous studies, the significant role of the apoptotic mechanism in the development of gastric cancer was emphasized (23). For instance, FoxP3 had been shown to inhibit gastric cancer cell proliferation and induce apoptosis by regulating apoptotic signaling transduction, offering a potential novel therapeutic strategy for gastric cancer (24). Moreover, ER stress could trigger an unfolded protein response (UPR), leading to either the restoration of cellular homeostasis or cell death, which had been observed in certain invasive gastric cancers (25). In normal physiological processes, the gastrointestinal mucosa produced a significant amount of ROS, and disruption of the microbial community and mucosal oxidative balance was closely associated with most digestive tract diseases, including gastritis, gastric cancer, inflammatory bowel disease, colitis, and cancer. The crucial role of ROS was implicated in these conditions (26). Additionally, SAP-1 had been identified as a negative regulator of the integrin-stimulated signaling pathway by dephosphorylating p130cas and other components, inhibiting gastric cancer cell proliferation and metastasis (27). Tumor immunogenicity involved tumor antigenicity and antigen presentation, which played integral roles in the response to immune checkpoint inhibitors (ICIs) across various cancer types (28,29). A recent study proposed a novel feature based on antigen processing and presentation-related genes, which could aid in identifying immune therapy responses, high-risk patient stratification, and prognostication of gastric cancer patients (30). Furthermore, evidence suggested a synergistic interaction between NLR and Helicobacter pylori in the pathogenesis of gastric cancer, promoting its development (31). Therefore, both GO and KEGG enrichment analyses had unveiled the association of these autophagy-related DEGs with the progression of gastric cancer.
Subsequently, we constructed a PPI network and identified five hub genes, including HIF1A, CTSB, PRKCD, HSP90AB1, and EIF2AK3. We identified overexpression of HIF1A and EIF2AK3 (PERK) in gastric cancer tissues. Surprisingly, the analysis of KM plots demonstrated that their overexpression correlated with improved patient survival, as illustrated in Figure S1A,S1B, suggesting a potential protective role. In contrast, the diagnostic model’s predictive ability for the PRKCD gene was inferior to CTSB (Figure S1C). Despite the favorable characteristics of the HSP90AB1 gene, its extensive prior investigation (32), including Wang H et al.’s elucidation of Hsp90ab1’s role and mechanism in gastric cancer invasion and metastasis, led us to opt out of further exploration of this gene. Lastly, we designated CTSB as the candidate gene. Results from immunohistochemistry and mRNA analysis substantiated the elevated presence of CTSB in gastric cancer tissues relative to non-cancerous tissues. CTSB, a unique multifunctional protein in the cysteine protease family, had an additional pH-sensitive occluding loop that acted as an endo- and exopeptidase depending on the pH value (33). The entire process of CTSB, from biosynthesis to lysosome targeting, had been tightly regulated at the transcriptional, post-transcriptional, and post-translational levels through mechanisms such as increased transcription levels, alterations in transcription start sites, splicing variants adjustment, and post-translational modifications through proteolytic processing, glycosylation, inhibition, and trafficking (34,35). CTSB played a crucial role in various physiological and pathological processes, such as cell proliferation, migration, autophagy, antigen presentation, cell apoptosis, hippocampus-dependent memory function, cellular differentiation, and tumor development (36-38). CTSB was an important biomarker and potential therapeutic target in many cancers (34). Recently, several studies had reported the importance of CTSB gene polymorphisms in gastric cancer incidence and the predictive value of CTSB gene polymorphisms for the risk and prognosis of gastric cancer (39). Furthermore, high expression of CTSB had been positively correlated with immune cell infiltration in gliomas and COVID-19-related lung adenocarcinoma (40,41). In this study, GSEA revealed that genes linked with CTSB predominantly concentrated in immune-related pathways. These pathways include the regulation of leukocyte degranulation, myeloid cell-mediated immunity, macrophage activation, augmented cytokine production in immune response, antigen binding, and the modulation of T cell differentiation. Consequently, it was postulated that increased CTSB expression could impact the tumor immune microenvironment in gastric cancer. Earlier studies had established a significant link between immunity and autophagy (42,43). Contemporary research had indicated that autophagy might regulate immune responses by altering cytokine secretion and immune cell function (44,45). CD4+ T cells were known to exert a potent immunosuppressive effect, furthering tumor progression by impeding robust anti-tumor immunity (46). Tumor-associated macrophages promoted tumor progression by subduing protective adaptive immunity, nurturing cancer stem cells, and facilitating genetic instability (47). This research utilized the TISIDB and TIMER databases to investigate the association between CTSB expression and immune infiltration in gastric cancer. In TISIDB, CTSB had shown significant positive correlations with immune factors such as CCL4, CSF1R, HAVCR2, IL10, PDCD1LG2, CD86, TNSRFS18, macrophage, MDSC, NK cells, and Treg cells. In the TIMER database, CTSB expression had been significantly positively correlated with the infiltration levels of macrophages, neutrophils, dendritic cells, and CD8+ T cells. Copy number variation of CTSB had been significantly correlated with the infiltration levels of dendritic cells, macrophages, neutrophils, CD4+ T cells, CD8+ T cells, and B cells. These results suggested an important role of CTSB in the recruitment and regulation of immune cell infiltration in gastric cancer.
This study had limitations as the information used was retrospective and needed verification through further studies. We were also unable to analyze specific clinical features, including the correlation between autophagy genes and certain subtypes of gastric cancer.
Conclusions
Our research has yielded significant results indicating the association between the rise in CTSB expression and the pessimistic outlook among gastric cancer patients. Additionally, our findings revealed a correlation between CTSB expression and various immune cells, highlighting its potential as a diagnostic tool for gastric cancer patients. Nonetheless, further clinical and translational investigations were required to ascertain whether CTSB served as a valuable therapeutic target.
Acknowledgments
We acknowledge the TCGA and GEO databases for providing researchable patient data.
Funding: This work was supported by grants from
Footnote
Reporting Checklist: The authors have completed the STREGA reporting checklist. Available at https://tcr.amegroups.com/article/view/10.21037/tcr-23-1755/rc
Peer Review File: Available at https://tcr.amegroups.com/article/view/10.21037/tcr-23-1755/prf
Conflicts of Interest: All authors have completed the ICMJE uniform disclosure form (available at https://tcr.amegroups.com/article/view/10.21037/tcr-23-1755/coif). The authors have no conflicts of interest to declare.
Ethical Statement: The authors are accountable for all aspects of the work in ensuring that questions related to the accuracy or integrity of any part of the work are appropriately investigated and resolved. The study was conducted in accordance with the Declaration of Helsinki (as revised in 2013).
Open Access Statement: This is an Open Access article distributed in accordance with the Creative Commons Attribution-NonCommercial-NoDerivs 4.0 International License (CC BY-NC-ND 4.0), which permits the non-commercial replication and distribution of the article with the strict proviso that no changes or edits are made and the original work is properly cited (including links to both the formal publication through the relevant DOI and the license). See: https://creativecommons.org/licenses/by-nc-nd/4.0/.
References
- Sung H, Ferlay J, Siegel RL, et al. Global Cancer Statistics 2020: GLOBOCAN Estimates of Incidence and Mortality Worldwide for 36 Cancers in 185 Countries. CA Cancer J Clin 2021;71:209-49. [Crossref] [PubMed]
- Hooi JKY, Lai WY, Ng WK, et al. Global Prevalence of Helicobacter pylori Infection: Systematic Review and Meta-Analysis. Gastroenterology 2017;153:420-9. [Crossref] [PubMed]
- Smyth EC, Nilsson M, Grabsch HI, et al. Gastric cancer. Lancet 2020;396:635-48. [Crossref] [PubMed]
- Sexton RE, Al Hallak MN, Diab M, et al. Gastric cancer: a comprehensive review of current and future treatment strategies. Cancer Metastasis Rev 2020;39:1179-203. [Crossref] [PubMed]
- Klionsky DJ. Autophagy: from phenomenology to molecular understanding in less than a decade. Nat Rev Mol Cell Biol 2007;8:931-7. [Crossref] [PubMed]
- Boya P, Reggiori F, Codogno P. Emerging regulation and functions of autophagy. Nat Cell Biol 2013;15:713-20. [Crossref] [PubMed]
- Wu Q, Sharma D. Autophagy and Breast Cancer: Connected in Growth, Progression, and Therapy. Cells 2023;12:1156. [Crossref] [PubMed]
- Liu G, Pei F, Yang F, et al. Role of Autophagy and Apoptosis in Non-Small-Cell Lung Cancer. Int J Mol Sci 2017;18:367. [Crossref] [PubMed]
- Chao X, Qian H, Wang S, et al. Autophagy and liver cancer. Clin Mol Hepatol 2020;26:606-17. [Crossref] [PubMed]
- Ahn CH, Jeong EG, Lee JW, et al. Expression of beclin-1, an autophagy-related protein, in gastric and colorectal cancers. APMIS 2007;115:1344-9. [Crossref] [PubMed]
- Li J, Pu K, Li C, et al. A Novel Six-Gene-Based Prognostic Model Predicts Survival and Clinical Risk Score for Gastric Cancer. Front Genet 2021;12:615834. [Crossref] [PubMed]
- Qiu J, Sun M, Wang Y, et al. Identification and validation of an individualized autophagy-clinical prognostic index in gastric cancer patients. Cancer Cell Int 2020;20:178. [Crossref] [PubMed]
- Uhlén M, Fagerberg L, Hallström BM, et al. Proteomics. Tissue-based map of the human proteome. Science 2015;347:1260419. [Crossref] [PubMed]
- Ru B, Wong CN, Tong Y, et al. TISIDB: an integrated repository portal for tumor-immune system interactions. Bioinformatics 2019;35:4200-2. [Crossref] [PubMed]
- Li T, Fan J, Wang B, et al. TIMER: A Web Server for Comprehensive Analysis of Tumor-Infiltrating Immune Cells. Cancer Res 2017;77:e108-10. [Crossref] [PubMed]
- Correa P. Gastric cancer: overview. Gastroenterol Clin North Am 2013;42:211-7. [Crossref] [PubMed]
- Digklia A, Wagner AD. Advanced gastric cancer: Current treatment landscape and future perspectives. World J Gastroenterol 2016;22:2403-14. [Crossref] [PubMed]
- Hamashima C, Ogoshi K, Okamoto M, et al. A community-based, case-control study evaluating mortality reduction from gastric cancer by endoscopic screening in Japan. PLoS One 2013;8:e79088. [Crossref] [PubMed]
- Hosokawa O, Miyanaga T, Kaizaki Y, et al. Decreased death from gastric cancer by endoscopic screening: association with a population-based cancer registry. Scand J Gastroenterol 2008;43:1112-5. [Crossref] [PubMed]
- Cao Y, Luo Y, Zou J, et al. Autophagy and its role in gastric cancer. Clin Chim Acta 2019;489:10-20. [Crossref] [PubMed]
- Masuda GO, Yashiro M, Kitayama K, et al. Clinicopathological Correlations of Autophagy-related Proteins LC3, Beclin 1 and p62 in Gastric Cancer. Anticancer Res 2016;36:129-36. [PubMed]
- Yang F, Peng ZX, Ji WD, et al. LncRNA CCAT1 Upregulates ATG5 to Enhance Autophagy and Promote Gastric Cancer Development by Absorbing miR-140-3p. Dig Dis Sci 2022;67:3725-41. [Crossref] [PubMed]
- Bir F, Calli-Demirkan N, Tufan AC, et al. Apoptotic cell death and its relationship to gastric carcinogenesis. World J Gastroenterol 2007;13:3183-8. [Crossref] [PubMed]
- Ma GF, Chen SY, Sun ZR, et al. FoxP3 inhibits proliferation and induces apoptosis of gastric cancer cells by activating the apoptotic signaling pathway. Biochem Biophys Res Commun 2013;430:804-9. [Crossref] [PubMed]
- Maiuri MC, Zalckvar E, Kimchi A, et al. Self-eating and self-killing: crosstalk between autophagy and apoptosis. Nat Rev Mol Cell Biol 2007;8:741-52. [Crossref] [PubMed]
- Wang Y, Wang K, Jin Y, et al. Endoplasmic reticulum proteostasis control and gastric cancer. Cancer Lett 2019;449:263-71. [Crossref] [PubMed]
- Bhattacharyya A, Chattopadhyay R, Mitra S, et al. Oxidative stress: an essential factor in the pathogenesis of gastrointestinal mucosal diseases. Physiol Rev 2014;94:329-54. [Crossref] [PubMed]
- Noguchi T, Tsuda M, Takeda H, et al. Inhibition of cell growth and spreading by stomach cancer-associated protein-tyrosine phosphatase-1 (SAP-1) through dephosphorylation of p130cas. J Biol Chem 2001;276:15216-24. [Crossref] [PubMed]
- Wang S, He Z, Wang X, et al. Antigen presentation and tumor immunogenicity in cancer immunotherapy response prediction. Elife 2019;8:e49020. [Crossref] [PubMed]
- Jhunjhunwala S, Hammer C, Delamarre L. Antigen presentation in cancer: insights into tumour immunogenicity and immune evasion. Nat Rev Cancer 2021;21:298-312. [Crossref] [PubMed]
- Wang KW, Wang MD, Li ZX, et al. An antigen processing and presentation signature for prognostic evaluation and immunotherapy selection in advanced gastric cancer. Front Immunol 2022;13:992060. [Crossref] [PubMed]
- Wang H, Deng G, Ai M, et al. Hsp90ab1 stabilizes LRP5 to promote epithelial-mesenchymal transition via activating of AKT and Wnt/β-catenin signaling pathways in gastric cancer progression. Oncogene 2019;38:1489-507. [Crossref] [PubMed]
- Castaño-Rodríguez N, Kaakoush NO, Goh KL, et al. The NOD-like receptor signalling pathway in Helicobacter pylori infection and related gastric cancer: a case-control study and gene expression analyses. PLoS One 2014;9:e98899. [Crossref] [PubMed]
- Jakoš T, Pišlar A, Jewett A, et al. Cysteine Cathepsins in Tumor-Associated Immune Cells. Front Immunol 2019;10:2037. [Crossref] [PubMed]
- Pišlar A, Jewett A, Kos J. Cysteine cathepsins: Their biological and molecular significance in cancer stem cells. Semin Cancer Biol 2018;53:168-77. [Crossref] [PubMed]
- Schleyer KA, Cui L. Molecular probes for selective detection of cysteine cathepsins. Org Biomol Chem 2021;19:6182-205. [Crossref] [PubMed]
- Aggarwal N, Sloane BF. Cathepsin B: multiple roles in cancer. Proteomics Clin Appl 2014;8:427-37. [Crossref] [PubMed]
- Niemeyer C, Matosin N, Kaul D, et al. The Role of Cathepsins in Memory Functions and the Pathophysiology of Psychiatric Disorders. Front Psychiatry 2020;11:718. [Crossref] [PubMed]
- Ma X, Wang Y, Fan H, et al. Genetic polymorphisms of Cathepsin B are associated with gastric cancer risk and prognosis in a Chinese population. Cancer Biomark 2021;32:189-98. [Crossref] [PubMed]
- Ma K, Chen X, Liu W, et al. CTSB is a negative prognostic biomarker and therapeutic target associated with immune cells infiltration and immunosuppression in gliomas. Sci Rep 2022;12:4295. [Crossref] [PubMed]
- Ding X, Ye N, Qiu M, et al. Cathepsin B is a potential therapeutic target for coronavirus disease 2019 patients with lung adenocarcinoma. Chem Biol Interact 2022;353:109796. [Crossref] [PubMed]
- Levine B, Mizushima N, Virgin HW. Autophagy in immunity and inflammation. Nature 2011;469:323-35. [Crossref] [PubMed]
- Deretic V, Saitoh T, Akira S. Autophagy in infection, inflammation and immunity. Nat Rev Immunol 2013;13:722-37. [Crossref] [PubMed]
- Jiang GM, Tan Y, Wang H, et al. The relationship between autophagy and the immune system and its applications for tumor immunotherapy. Mol Cancer 2019;18:17. [Crossref] [PubMed]
- Zhong Z, Sanchez-Lopez E, Karin M. Autophagy, Inflammation, and Immunity: A Troika Governing Cancer and Its Treatment. Cell 2016;166:288-98. [Crossref] [PubMed]
- Takeuchi Y, Nishikawa H. Roles of regulatory T cells in cancer immunity. Int Immunol 2016;28:401-9. [Crossref] [PubMed]
- Mantovani A, Marchesi F, Malesci A, et al. Tumour-associated macrophages as treatment targets in oncology. Nat Rev Clin Oncol 2017;14:399-416. [Crossref] [PubMed]