Segmentation method of magnetic resonance imaging brain tumor images based on improved UNet network
Highlight box
Key findings
• The method based on the improved UNet network has obvious advantages in the magnetic resonance imaging (MRI) brain tumor image segmentation method.
What is known and what is new?
• It is known that the traditional segmentation methods of image copy processing and machine learning are not satisfactory in the segmentation of glioma.
• The method used in this study showed stronger feature extraction ability than the UNet model. In addition, our findings demonstrated that the use of generalized Dice loss and subjective cross entropy as loss functions in the training process effectively alleviated the class imbalance of glioma data and effectively segmented glioma.
What is the implication, and what should change now?
• This study explored the potential of MRI brain tumor images as an effective segmentation method of glioma.
Introduction
According to a 5-fold cross-validation evaluation results (1,2), glioma is accounting for 27% of primary central nervous system tumors (3-6) due to the influence of ionizing radiation and gene mutation. The incidence of glioma increases with age (1,2,6-8). With different-grade gliomas, there is different incidence rate. According to malignancy, glioma is pathologically classified into grade I to grade IV, among which grade II and below are low-grade gliomas (LGGs), and grade III and above are high-grade gliomas (HGGs) (9). For instance, the median survival time (MST) of HGG patients is generally less than 2 years, whereas the MST of HGG patients with HGGs is only 4–9 months. Furthermore, a molecular study has identified features that can enhance diagnosis and provide biomarkers (10). The presence of Isocitrate dehydrogenase 1 and 2 (IDH1/2) mutation along with X-encoded protein (ATRX) and TP53 mutations is indicative of diffuse astrocytomas, while IDH1/2 mutations combined with 1p19q loss is indicative of oligodendroglioma (10). Focal amplifications of receptor tyrosine kinase genes, telomerase reverse transcriptase (TERT) promoter mutation, and the loss of chromosomes 10 and 13 with trisomy of chromosome 7 are distinctive features of glioblastoma and can be utilized for diagnostic purposes (10). Additionally, the presence of B-Raf proto-oncogene (BRAF) gene fusions and mutations in LGGs and mutations in histone H3 in HGGs can also serve as diagnostic markers (11).
Magnetic resonance imaging (MRI) is the only magnetic imaging technology that can evaluate the biochemical and metabolic conditions of cells and tissues without trauma by evaluating the structure of tissue. It can initially assess the degree of tumor deterioration, identify the scope of invasion, and then analyze and judge the condition of brain tumors. In order to facilitate the accurate resection of glioma during surgery, the clinical treatment generally relies on a variety of advanced imaging modalities such as computed tomography (CT), MRI, positron emission tomography (PET), and diffusion tensor imaging (DTI) to delineate the boundaries of the glioma lesion. These imaging technologies assist surgeons in achieving maximal extent of resection. Among these modalities, MRI stands out for its multiplanar imaging capability and high spatial resolution, making it a widely utilized tool in the clinical imaging diagnosis of gliomas.
Currently, glioma has been confirmed to consist of tumors in a variety of tissue types (necrotic nucleus, active tumor margin, and edema tissue). MRI can scan different tumor tissues and generate correct glioma imaging reports, thus identifying and judging the types of gliomatosis (11), as shown in Figure 1. MRI astrocytoma imaging uses the following 4 main sequences: T1-weighted image (T1WI), T1WI contrast enhancement (T1WIce), T2-weighted image (T2WI), and fluid-attenuated inversion recovery (FLAIR), reflecting the different tissues (12). For example, FLAIR sequences are suitable for observing edematous tissues whereas T1ce sequences (MRI sequences obtained after injection of gadolinium-based contrast agent) are appropriate for the observation of the active components of the tumor nucleus. Thus, multiple MRI sequences allow physicians to visualize and analyze different tumor tissues and to treat them with the appropriate therapy.
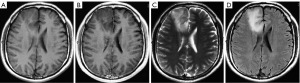
Glioma segmentation is believed to be one of the most important stages of treatment management (13,14). Recent developments in MRI protocols have led to a renewed interest in using automatic glioma segmentation with different MRI image weights. MRI can better distinguish any glioma and its surrounding abnormal tissues, capture the external morphology of individual tumor tissues of glioma patients, and grade the degree of glioma through image analysis to help further treatment. Glioma segmentation is considered an important step in MRI analysis in glioma patients. Typically, different degrees of gliomas are identified by MRI modality and multi-lateral 3-dimensional (3D) scanning of the area. Therefore, it is essential to grow an automatic glioma segmentation process with better precision in clinical practice to reduce the segmentation errors caused by self-related factors (13,14).
Although traditional image processing-based glioma segmentation algorithms have achieved certain segmentation results in small-scale and low-modality glioma datasets, reports on large-scale and multimodal glioma dataset using these techniques are rare. Additionally, these methods process two-dimensional (2D) images and are not effective in segmenting process particularly 3D glioma regions. These methods require manual correction or post-processing of the segmentation results and do not achieve fully automated segmentation of gliomas (15,16).
During the last two decades, computer-aided diagnosis (CAD) techniques, which are based on machine learning algorithms (MLA), have been widely used in the analysis of medical imagery (17,18). Various features of medical images are generated based on computer-aided technology to train the model’s parameters and provide rich features through the exercise model. These models have solved classification, regression, clustering, and association problems in medical images. For instance, deep learning techniques automatically obtain comprehensive image features directly from the data (19-21). Additionally, forward and backward adjustment algorithms have been used to adjust the parameters of the multi-modal MRI model to optimize its performance in glioma grading.
The classification of glioma plays a primary function in the formulation of a treatment plan and prognosis, and the machine segmentation mechanism of glioma is an ideal choice to solve the problem of traditional artificial pattern recognition and overcome the shortcomings of traditional image processing algorithm. The computer segmentation algorithm can realize automatic segmentation of glioma, greatly improve the accuracy of the algorithm, and execute large-scale multimodal segmentation of complex glioma. These algorithms have obtained remarkable results in the segmentation of large-scale multimodal complex glioma (22,23).
In the evaluation outcomes of the Brain Tumor Image Segmentation Benchmark (BraTS) validation set, Zikic et al. (24) used a single-branch 2D Conv neural network based on pixel-by-pixel segmentation, yet Pereira et al. (25) used a dual-branch pixel-by-pixel segmentation network. Meanwhile, Ben et al. (26) used 3 full convolutional networks for segmentation of gliomas, including a 2D full convolutional network and a 3D full convolutional network and fused the results by integrated learning techniques. Li et al. (27) conducted quantitative and qualitative experiments on the BraTS 2020 dataset to evaluate the performance of the Evidential Deep Learning model for segmentation and uncertainty estimation. The results show that this method has excellent performance in quantitative segmentation of uncertainty and robust segmentation of tumors (27). The publicly available training dataset provided for the 2021 RSNA-ASNR-MICCAI Brain Tumor Segmentation (BraTS) Challenge was used in Boehringer’s study, consisting of 1,251 multi-institutional, multi-parametric MR images (28). Their result showed that the active learning approach, when applied to model training, can drastically reduce the time and labor spent on preparation of ground truth training data (28).
We developed a 2D residual block UNet in order to improve the incorporation of glioma segmentation into the clinical process. The efficiency is seamlessly interrelated with using something called the BraTS. Twenty of the most advanced tumor segmentation algorithms were used on sixty-five multi-contrast MR scans of LGG and HGG patients. As many as four raters had personally annotated these scans, and they were compared to sixty-five scans that were created using tumor image simulation software. It was specified that the Dice scores for WT, TC, and ET would be provided, with expert segmentation serving as the benchmark. Hospital of Hubei University of Arts and Science provided a distinct clinical dataset that was utilized in the subsequent evaluation of the approach. We present this article in accordance with the MDAR reporting checklist (available at https://tcr.amegroups.com/article/view/10.21037/tcr-23-1858/rc).
Methods
Network structure of the proposed model
In this area, either a thorough explanation of the possible analysis system, which is utilized in prior investigation, or the proposed mechanism is presented, including UNet model improvement procedures. Inclusion criteria were followed: All patients with MRI images were glioma patients. Therefore, the 200 MRI images have been taken from Hospital of Hubei University of Arts and Science. Training is a modeling process, and the process of verifying the model is verification. The study was conducted in accordance with the Declaration of Helsinki (as revised in 2013). The study was approved by the ethics committee of Hospital of Hubei University of Arts and Science (No. XYS20220518) and individual consent for this retrospective analysis was waived.
Improvement of the UNet model
To address various problems associated with the traditional full convolutional networks such as shallow model depth (Single hidden layer neural network and single layer neural network (that is, logistic regression), usually a neural network with few hidden layers, such as only one or two layers, is called shallow model) and inadequate acquisition of image feature information, a full convolutional network model (the proposed network is a simple design that employs different heads involving graph convolutions focused on edges and nodes, capturing representations from the input data thoroughly) using the residual block (ResBlock, the residual block transfers information along the depth of the network by introducing skip connections that add the network output of the previous layer directly to the network input of the current layer) mechanism is proposed, which is based on the UNet model, for glioma segmentation. This model is known as the 2D residual block UNet (2DResUNet model; UNet with residual block helps to solve the gradient vanishing and gradient exploding problems and train deeper networks while ensuring good performance, Figure 2).
The proposed 2DResUNet model makes the following improvements in the original UNet partitioning model:
- Based on the numerous varieties in the label, the picture is segmented according to many sequences in the input model and multiple gliomas. The original UNet single-channel input layer and single-channel output layer are modified to 4-channel input layer and 4-channel output layer (29). Additionally, a 2D Input Module is set to add a Gaussian noise layer to input data for data enhancement during the model training process.
- The encoder ResBlock structure is used to replace two convolutional layers in the down sample portion of the prototype to encode the picture information. This structure improves the model structure by using the residual mechanism to prevent the gradient disappearance and gradient explosive such that the features are extracted more effectively than simply overlaying the convolutional layers. Meanwhile, the pooling layer is replaced by a convolutional layer with a step size of two, which reduces the loss of information in the down sampling process (30,31).
- In the up-sampling part of the model for solving the encoded element maps, the Decoder ResBlock’ structure is used to replace the two convolutional layers, which effectively reduces the information loss during the up-sampling process due to this model incorporating a modified atrous spatial pyramid pooling module to learn the location information and to extract multi-level contextual information. Meanwhile, the feature map is bilinearly interpolated using the UpSampling layer, thus, gradually recovering the image size.
- The total of generalized die loss and weighted cross entropy (WCE) is used as the loss function (Loss function is a function that maps the value of a random event or its related random variables to a non-negative real number to represent the “risk” or “loss” of that random event) of model training for glioma class imbalance. Thus, the pixel weights are adjusted according to the distribution of positive and negative samples in the glioma labels. Additionally, loss value is continuously reduced towards the direction with the largest segmentation cross-comparison ratio.
Bra TS2018 local 5-fold cross-validated dataset was used as benchmark for models. The evaluation results in the BraTS2018 native 5-fold cross-validation are shown in Tables 1,2. Hence, to assess the division outcome of the suggested models, the intermediate networks designed during the model building process were experimentally evaluated and these intermediate networks mainly included the 2DDenseUNet, DM-ResUNet, DM-DenseUNet, and other networks.
Table 1
Model name | Dice score | Specificity | Sensitivity | ||||||||
---|---|---|---|---|---|---|---|---|---|---|---|
WT | TC | ET | WT | TC | ET | WT | TC | ET | |||
2DUNet | 0.8447±0.111 | 0.7687±0.218 | 0.6929±0.259 | 0.9966±0.002 | 0.9979±0.001 | 0.9981±0.001 | 0.8585±0.111 | 0.7740±0.220 | 0.7927±0.164 | ||
3DUNet | 0.8588±0.111 | 0.7687±0.218 | 0.6926±0.259 | 0.9966±0.002 | 0.9979±0.001 | 0.9981±0.001 | 0.8628±0.056 | 0.8316±0.166 | 0.7927±0.164 | ||
SegAN | 0.8951±0.064 | 0.7619±0.216 | 0.7397±0.269 | 0.9974±0.001 | 0.9955±0.001 | 0.9972±0.002 | 0.9955±0.001 | 0.9972±0.002 | 0.8674±0.178 | ||
Isensee 3DresUNet | 0.8952±0.056 | 0.7902±0.215 | 0.7087±0.257 | 0.9969±0.002 | 0.9982±0.002 | 0.9989±0.001 | 0.8804±0.068 | 0.8012±0.144 | 0.8018±0.146 | ||
2DResUNet | 0.8551±0.131 | 0.7430±0.220 | 0.7149±0.290 | 0.9970±0.001 | 0.9990±0.001 | 0.9996±0.003 | 0.9011±0.178 | 0.7982±0.252 | 0.7997±0.244 | ||
3DResUNet | 0.8612±0.148 | 0.7561±0.131 | 0.7150±0.143 | 0.9939±0.001 | 0.9950±0.001 | 0.9931±0.001 | 0.9022±0.112 | 0.8052±0.200 | 0.8003±0.244 | ||
DM-ResUNet | 0.8951±0.002 | 0.7902±0.131 | 0.7087±0.210 | 0.9969±0.002 | 0.9987±0.001 | 0.9989±0.003 | 0.8804±0.123 | 0.8012±0.198 | 0.8018±0.201 | ||
DM-DenseUNet | 0.9013±0.059 | 0.7893±0.224 | 0.7132±0.267 | 0.9950±0.002 | 0.9983±0.003 | 0.9990±0.001 | 0.8936±0.068 | 0.7916±0.008 | 0.7931±0.188 | ||
DM-DA-UNet | 0.9060±0.060 | 0.8911±0.205 | 0.7209±0.270 | 0.9967±0.002 | 0.9983±0.002 | 0.9990±0.001 | 0.9015±0.067 | 0.8189±0.146 | 0.7963±0.190 |
2DResUNet, 2-dimensional residual block UNet; WT, whole tumor; TC, tumor core; ET, enhancing tumor.
Table 2
Model name | Hausdorff distance | ||
---|---|---|---|
WT | TC | ET | |
2DUNet | 9.15±3.571 | 8.14±4.701 | 3.82±2.120 |
3DUNet | 11.24±4.445 | 8.40±4.715 | 3.20±1.714 |
GAN | 8.14±3.089 | 8.48±3.631 | 4.44±2.404 |
Isensee 3DResUNet | 10.69±3.783 | 7.55±4.199 | 4.22±1.883 |
2DResUNet | 8.50±3.255 | 7.17±3.909 | 4.02±1.876 |
3DResUNet | 9.20±3.332 | 6.11±4.018 | 3.12±1.913 |
DM-ResUNet | 10.32±4.001 | 7.05±3.821 | 3.56±2.301 |
DM-DenseUNet | 12.62±4.225 | 8.45±4.215 | 3.97±1.903 |
DM-DA-UNet | 12.20±3.907 | 8.53±4.721 | 4.15±2.018 |
2DResUNet, 2-dimensional residual block UNet; GAN, Generative adversarial network; WT, whole tumor; TC, core tumor; ET, enhancing tumor.
Splitting approach
The 2DResUNet-based glioma segmentation method consists of 6 steps: data pre-processing, data enhancement, model training, performance evaluation, prediction data, data post-processing. The overall flow of segmentation is shown in Figure 3.
- Step 1 of the algorithm is to perform preprocessing operations on the data, which is performed on the raw MRI of glioma to eliminate the factors affecting the activity of the neural network in the basic image acquisition and transform the image into the input form required by the model. Its main operations include image bias correction, image cropping, image normalization, random sampling, and other operations.
- Step 2 is to enhance the data of the pre-processed image, increase the amount and type of data in the procedure of model activity, and improve the generalization ability and segmentation effect of the model. For a relatively small data set of gliomas, this step has effectively improved the segmentation effect of the model, mainly including the image up and down, left and right rotation, random cropping, translation transformation, random scaling, random rotation, and other data enhancement operations.
- Step 3 is model training, thus training the model parameters. For model training, the environment of the exercise parameters and the initialization of the model weight parameters have a direct impact on the model training.
- Step 4 is to estimate the enactment of the activity standard using the Dice score, specificity, sensitivity, and Hausdorff distance, as described in section Training of the splitting model.
- Step 5 is the process of predicting new cases, namely, the process of predicting test data without labels, and the trained model can be used to predict the test data after the model training is completed.
- Step 6 is the process of data post-processing, as the model may have mis-scored multiple score, so the data post-processing operation is needed for the model-predicted glioma mask.
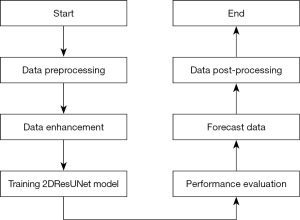
Additionally, the model uses a 2D convolutional full convolutional network for feature extraction and sampling of the complete input image, which has a good performance in distinguishing the types of diffuse glioma and makes up for the problem of a small amount of data in model training. 2DDenseUNet is the 2D full convolutional network in the first stage of DM-DA-UNet network, which is extracted separately for experiments in local cross-validation in order to confirm the efficacy of DenseBlock. Compared with the 3D convolutional full convolutional network, feature extraction has higher sensitivity, and is suitable for segmentation of glioma regions with fewer middle layers and larger thickness in brain MRI, which has better clinical applicability (32,33).
Structure of the proposed 2DResUNet
In the proposed 2DResUNet structure, the Input Module is used as shown in Figure 4 and the Gaussiannoise layer is added to the Input Module structure to add a Gaussian noise with normal variation of 0.01 to the input data to alleviate the overfitting phenomenon during the model training. Features are extracted from input images using a 2×2 kernel size (64-kernel) 1-step 2D convolution (Conv) layer.
Meanwhile, for a traditional L-layer convolutional neural network, if there is an input image, then the nonlinear transformation of the ith layer of the gannet is denoted as H(.) where H(.) is the accumulation of various function processes such as batch normalization (BN), rectified linear unit (ReLU), pooling DX* Conv, and so on. Moreover, if we let X denote output of the ith network layer in the conventional neural network, then it is represented in Eq. [1].
For ResNet, a SkipConnection is added to the traditional convolutional network such that the ith layer of the ResNet network is expressed as shown in Eq. [2].
This structure is generally called ResBlock structure, which has effectively replaced the simple convolutional layer overlay in the convolutional network, thus improving the model performance.
Based on the idea of ResBlock, 3 Encoder ResBlocks for feature encoding and 3 2D Convs with step size 2 are used in the down sampling part of 2DResUNet. The Encoder ResBlock structure is the basic structure of the model for encoding the feature map during down sampling, as shown in Figure 5, and consists of 2 Conv nuclei of size 3×3 (step 1), a BN layer and parametric ReLU (PReLU) activation function. The ADD-tool fuses the input features with the output features to construct the ResBlock, so Encoder ResBlock is a structure based on the ResBlock structure for feature extraction.
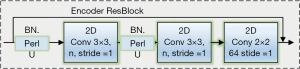
Up-sampling part of 2DResUNet uses 4 Decoder ResBlocks for decoding, 3 2D UpSampling for up-sampling the feature map to restore the size, and 1 Output Module for output masks.
In the up-sampling part of 2DResUNet, the Decoder ResBlock structure is the basic structure for decoding the feature map in the up-sampling process, as shown in Figure 5, which mainly consists of two convolutional kernels with a size of 3×3 (step 1). The corresponding BN layer and PReLU activate these functions of these two convolutional layers and a convolutional block for extracting the features on the input side. The kernel size is 1×1 with fixed bias and the ADD mechanism is used to fuse the input and output features to construct the ResBlock (34,35).
Loss function
In the glioma segmentation task, the glioma region to be segmented often accounts for a small percentage of the multi-layered 3D brain MRI and there is an imbalance between the foreground and background pixel categories. This category imbalance can cause the model to fall into a local minimum of the loss function during training and lead to poor training results. Therefore, this category imbalance can be mitigated by adjusting the loss function to increase the weight of the foreground region.
A commonly used UNet loss function is Dice loss, which was proposed by Kshirsagar et al. (35). This loss function makes the model reduce the loss in the direction of the largest intersection ratio between the predicted result and the true mask during the training process, thus alleviating the category imbalance. The original Dice loss can be expressed as shown in Eq. [3].
Where N, pn, rn, and ε denote the number of pixels, pixel value of the predicted result, pixel value of the true mask, and constant value, respectively.
Although Dice loss can alleviate the category imbalance problem in binary classification problems to some extent, it often performs poorly on multi-region segmentation tasks. This is because during the training process, as soon as a prediction result of a region in the multi-region is wrong, a large change in Dice loss will occur, which leads to drastic gradient changes and unstable training.
In response to this phenomenon, a loss function called generalized Dice loss (GDL) has been proposed, which integrates Dice loss for multiple regions of the lesion based on Dice loss and quantifies the results of multi-region segmentation using a single metric. The GDL calculation is expressed as shown in Eq. [4].
L, N, rln, and pln denote title classifications, the numeral of pixels, the pixel value of the true mask, and the pixel value of the predicted outcome, respectively.
wl denotes the weight of each category.
In the process of 2DResUNet model design, to better solve the problem of unbalanced categories in glioma segmentation and the need to segment multiple regions, the proposed approach adds the WCE on top of the GDL as the loss function. The WCE is based on the Cross Entropy loss procedure to weight the target region to be segmented, so as to enhance the learning of the target region of the model.
Therefore, the loss function utilized by the 2DResUNet is the total of WCE and GDL, which can be expressed as Eq. [7].
In order to verify that the loss function has effectively mitigate the category imbalance, a comparison experiment of the loss function was designed.
Data pre-processing methods and statistical analysis
The preprocessing mechanism used in the 2DResUNet-based glioma segmentation method is described as follows:
- Initially, 4 input sequences correspond to T1WI, T1ceWI, T2WI, and FLAIR of size 240×240×155 are bias corrected, respectively.
- Brain region of the 3D image is determined, and the image cropping frame is obtained.
- The bias corrected image is cropped in 3D using the cropping frame to remove unnecessary background information.
- The cropped image is then normalized to make it easier for the neural network to learn.
- Finally, the normalized image is randomly sampled to obtain the input sequence for training the 2DResUNet model with a size of 4×128×128.
In the process of MRI generation by MRI machines, due to the performance limitations of the machines and the surrounding environment, the generated MRIs often show bias field effects with inhomogeneous intensity and motion artifacts (36,37). This bias field effect causes image blurring and noise, which makes image segmentation more difficult, so bias correction of the original MRI is needed to eliminate the effect of bias field effect on model training and prediction.
Image standardization
Generally, MRI is obtained by different scanners and the intensity value distribution of the original MRI is often uneven. This uneven intensity distribution is not conducive to the training of neural network models. Therefore, the cropped images need to be intensity normalized using axial series of a normal brain from the same MR machine so that the intensity distribution of the images is limited to a certain range, which is more conducive to the convergence of the model training process and reduces the influence of discrete points.
As for the glioma image, the image contains a large number of background regions with zero-pixel intensity. The mean and standard deviation of all pixel points cannot be used as normalization as in the natural image when performing pixel normalization. The standard and friction of the pixel intenseness of non-zero areas need to be used to normalize each pixel point in the image such that the pixel intensity in the image is uniformly distributed around 0, as shown in Eq. [8].
The intensity histograms of the original T1 sequence images of patients in the BraTS2018 (38-40) dataset and the intensity histograms of the normalized images are shown in Figure 6. The ratio of test set, validation set, and training set 1:1:8 was divided by the BraTS2018 data set. Dice scores of different loss functions evaluated by validation set were obtained after 10 generations of training on the local data set.
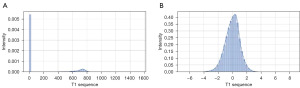
Training of the splitting model
For the pre-processed glioma images, online data enhancement can be performed in batch, which allows the generation of enhanced data and their insertion into the model for training. The standard with the promising metrics is established and saved.
In the process of model activity, in order to protect computer recollection and acquire the full picture data of the patient, image data should be input as much as possible. 2DResUNet uses random sampling to obtain 128×128 patches for training and continuously adjusts the parameters of relevant convolutional layers by forward and backward propagation during the training process. First, it is established to 8 and then the numeral of activity iterations (epoch) is set to 13. The optimizer employed is Stochastic Gradient Descent (SGD) procedure where the learning rate (lr) is 0.08, the momentum parameter (momentum) is set to 0.9, and the decay value of the lr after each parameter update is set to 0.00005.
Results
The Dice score of different loss functions evaluated by the validation set after 10 generations of training in a local dataset divided by the BraTS2018 dataset with the ratio of test set, validation set, and training set, in a ratio of 1:1:8 is shown in Table 3. As mentioned above, using the loss function of the sum of GDL and WCE can significantly improve the segmented glioma region.
Table 3
Loss function | Dice score | ||
---|---|---|---|
WT | TC | ET | |
WCE | 0.8209 | 0.7096 | 0.6823 |
GDL | 0.8393 | 0.7280 | 0.7058 |
GDL+ WCE | 0.8579 | 0.7463 | 0.7100 |
WT, TC, ET are the target of three different types of BraTS segmentation. 2DResUNet, 2D residual block UNet; WT, whole tumor; TC, tumor core; ET, enhancing tumor; WCE, weighted cross entropy; GDL, generalized Dice loss.
The training procedure of the 2DResUNet model is demonstrated in Figure 7. The N4 bias correction algorithm (41), an improved bias correction algorithm based on the N3 bias correction algorithm, is an effective and stable bias correction algorithm based on hierarchical processing methods and B spline interpolation. For a glioma patient data in the BraTS2018 dataset, the intensity histogram of the original T1 sequence is shown in Figure 8A,8B, whereas the intensity histogram of the image with non-zero pixel intensity is shown in Figure 8C,8D, which show that in the original glioma MRI, the intensity distribution of the image is uneven and the overall intensity value is low, yet the pixels with weak intensity occupy a large ratio of the pixels with intensity 0. The overall intensity histogram and the non-zero power histogram of the T1 series after the N4 bias correction algorithm are shown in Figure 8B and Figure 6C, respectively, which show that after the N4 bias correction algorithm, the number of low-intensity pixels in the glioma image is significantly reduced, the proportion of pixels with intensity 0 is effectively reduced, and the overall image intensity distribution is more uniform. The intensity imbalance was eliminated.
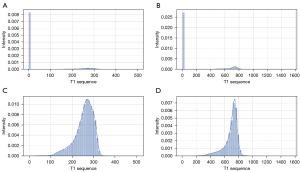
In order to obtain the optimal model structure for segmentation, the loss function significance (Loss) of the verification set is utilized as the standard for model conservation during the activity method. The loss curves of the validation set of the original UNet model and the 2DResUNet model during training which are shown in Figure 9 were used to verify the validity of the network structure, with the full of GDL and WCE as the loss procedure and the models trained for 13 generations. From the decreasing trend of the curves, we can see that the loss curve of 2DResUNet model decreases more rapidly than that of the original UNet model during the training process, and the loss value in each iteration is lower than that of the original UNet model. The 2DResUNet model is superior to the original UNet model in terms of network structure. However, since 2DResUNet is an improved network based on UNet, the number of iterations in which the loss does not decrease anymore is relatively similar, and both of them stop decreasing after about 8 iterations.
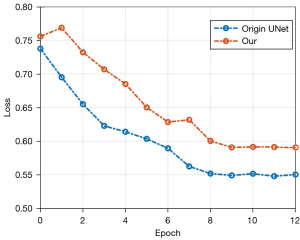
The change curve of validation set loss during training for each model is shown in Figure 10. Among these curves, the 2DDenseUNet model had a better loss reduction trend than the 2DResUNet model and the traditional UNet model. Additionally, it was structurally better than the other two models.
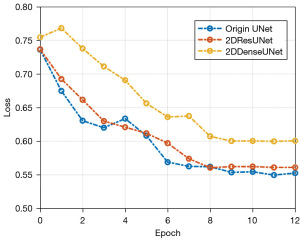
During the training process, the loss curve of validation set allows a comparative analysis of models using the same loss function but with different structures. For our study, to confirm efficiency of the suggested 2DResUNet and 2DDenseUNet hybrid approach, Bra TS2018 local 5-fold cross-validated dataset was used as benchmark for models. Additionally, it was used under the condition that the lot of training repetitions is set to 12 and the sum of GDL and WCE is used as the loss function, the variation curves of the validation set loss for each prototypical throughout the exercise procedure. The change curve of validation set loss during training for each model is shown in Figure 10. Among these curves, the 2DDenseUNet model has a better loss reduction trend than the 2DResUNet model and the traditional UNet model. Additionally, it is structurally better than the other two models.
We conducted experiments to verify the cogency of the 3D-DA UNet density-block. These trials were approved out under the state that the BraTS2018 local 5-fold cross-validation divided dataset was used as a model and both models were trained for 100 generations. Additionally, wavelength dependent loss (WDL) was used as the loss function and validation set loss of each model during the training process, as shown in Figure 11. The trend of each model during training is shown in Figure 11.
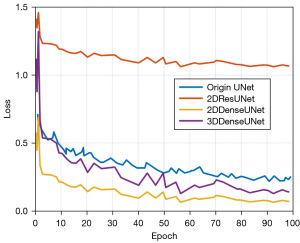
As soon as model training was completed, the model was mature enough to be used as a prediction instrument, examination set, to acquire the model-predicted glioma segmentation outcomes, namely, the glioma segmentation mask. In the examination set split up by local 5-fold cross-validation, the proposed glioma prediction outcomes of per model are demonstrated in Figure 12, where Figure 12A is the original T1 sequence of a patient in BraTS2018 dataset; Figure 12B is real labeled glioma division mask of the patient and the green area indicates the edema region, the yellow province demonstrates the enhancement province, and the red area demonstrates the essence province; Figure 12C is the prediction mask of the proposed 2DResUNet model and we have observed that the segmentation result has a good segmentation sensitivity and can segment the real glioma region, but there are cases of over-segmentation and mis-segmentation; Figure 12D is the prediction mask of the enhanced 2DDenseUNet on the 2DResUNet, which reduces the cases of over-segmentation or mis-segmentation in the 2DResUNet while ensuring the segmentation sensitivity and improves the segmentation accuracy; Figure 12E is the prediction mask of DM-DenseUNet model, the attention mechanism is removed from DM-DenseUNet model such that the effectiveness of the engagement tool is verified, and the segmentation outcomes display that the attention mechanism can effectively improve the segmentation accuracy; Figure 12F is the prediction mask of DM-DA-UNet, which has a better segmentation effect on the three regions of glioma compared with other models, and effectively reduces the mis-segmentation and multi-segmentation in each region, which is basically consistent with the mask distribution of real glioma.
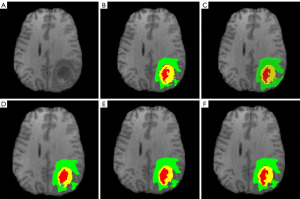
The evaluation results in the BraTS2018 local 5-fold cross-validation are shown in Tables 1,2, respectively. To assess the division effect of the suggested models, the intermediate networks designed during the model building process are experimentally evaluated and these intermediate networks mainly include the 2DDenseUNet, DM-ResUNet, DM-DenseUNet, and other networks.
Discussion
Alternatively, existing techniques have used multiple full convolutional networks such as DeepMedic, FCN, and UNet for integrated learning to segment the gliomas and obtained the best segmentation results on the BraTS2017 (38) dataset so far. Therefore, it is observed that the present full convolutional network-based glioma division method is mightily better than the pixel-by-pixel convolutional neural network segmentation method in terms of segmentation precision and segmentation sensitivity. Additionally, the simultaneous usage of considerable different dimensional models to segment gliomas has effectively enhanced the segmentation outcomes as demonstrated in Table 4. This is because the proposed model adds (I) ResBlock mechanism to the UNet model, (II) a Gaussian noise layer to the input layer for data enhancement, and (III) replaces the pooling layer with a 2D convolutional layer. Thus, it has a stronger feature extraction ability than the original UNet model.
Table 4
Model structure | Dice score | Specificity | Sensitivity | ||||||||
---|---|---|---|---|---|---|---|---|---|---|---|
WT | TC | ET | WT | TC | ET | WT | TC | ET | |||
2DResUNet | 0.85 | 0.74 | 0.70 | 0.99 | 0.99 | 0.99 | 0.87 | 0.76 | 0.72 | ||
DM-DA-UNet | 0.90 | 0.80 | 0.73 | 0.99 | 0.99 | 0.99 | 0.89 | 0.77 | 0.75 | ||
Naceur | 0.89 | 0.76 | 0.81 | – | – | – | 0.82 | 0.82 | 0.69 | ||
Wang | 0.88 | 0.82 | 0.74 | – | – | – | – | – | – | ||
Havaei | 0.88 | 0.79 | 0.63 | – | – | – | 0.87 | 0.79 | 0.80 | ||
Kamnitsas | 0.90 | 0.79 | 0.73 | – | – | – | 0.90 | 0.78 | 0.76 | ||
Zikic | 0.84 | 0.74 | 0.69 | – | – | – | – | – | – | ||
Isensee | 0.89 | 0.79 | 0.73 | 0.99 | 0.99 | 9.99 | 0.89 | 0.82 | 0.80 | ||
Pereira | 0.87 | 0.73 | 0.68 | – | – | – | 0.86 | 0.77 | 0.70 |
2DResUNet, 2-dimensional residual block UNet; WT, whole tumor; TC, core tumor; ET, enhancing tumor.
In our work, to assess the division outcome of the suggested models, the intermediate networks designed during the model building process were experimentally evaluated and these intermediate networks mainly included the 2DDenseUNet, DM-ResUNet, DM-DenseUNet, and other networks. Among these networks, 2DDenseUNet is the 2D full convolutional network in the first stage of DM-DA-UNet network, which is extracted separately for experiments in local cross-validation in order to verify the effectiveness of DenseBlock. DM-ResUNet is a network structure that uses only the ResBlock mechanism without the Attention mechanism in the design of DM-DA-UNet. DM-DenseUNet structure is a network structure that uses only the DenseBlock mechanism without the attention mechanism in the design of DM-DA-UNet. In addition, 2DUNet in relevant papers (42-44) is copied (the original UNet configuration is utilized as the intake picture of the convolutional network neural in the 2D Conv model).
Meanwhile, the results of the 5-fold cross-validation evaluation display that the proposed 2DResUNet structure has a high sensitivity despite the slightly lower evaluation result on the Dice score. At the same time, compared with other models used in the experiment, the DM-DA-UNet model suggested in this paper was significantly improved in various indicators (dice score, specificity, sensitivity, Hausdorff distance), increasing the reliability of the model and providing a reference and basis for the accurate formulation of clinical treatment strategies.
Although the models have evaluated quantitatively through local partitioning of datasets and cross-validation, the validation by local partitioning of datasets alone may suffer from training overfitting due to the small amount of data compared to real-world scenarios. The suggested 2DResUNet and DM-DA-UNet models were used to perform predictions and validations on the BraTS2017 dataset, and the results of those analyses were then uploaded to the official website for users to review and provide input on. Comparing the glioma segmentation model to the glioma segmentation model in relevant research (38-40) provided an objective verification of the model’s validity through comprehensive examination of the validation data set. This allowed for a more accurate diagnosis of gliomas. In the model evaluation using the BraTS2017 verification set, the 2DResUNet structure demonstrated higher sensitivity but a lower Dice score compared with the glioma segmentation model in relevant papers (40-42). The proposed DM-DA-Unet structure has a significant segmentation effect on specificity, sensitivity, and the Dice score are all terms that are discussed. The cutting-edge model also has a Dice score that is incredibly near to the Dice score accuracy of the promising segmentation model on the BraTS2017 forum at the moment.
However, existing glioma segmentation methods, which rely on traditional image processing and computer parameter acquisition, show shortcomings in glioma diagnosis and evaluation. Therefore, deep learning in brain glioma segmentation and grading is of great significance for the establishment of surgical plan and the improvement of prognosis. Among the deep learning-based glioma segmentation methods, the full convolutional network model has been shown to be very effective in glioma segmentation. In this paper, we have suggested the 2DResUNet model to address problems of small perceptual field, shallow model depth, and large information loss in the coding and decoding process of the current full convolutional network model. The proposed model adds (I) ResBlock mechanism to the UNet model, (II) a Gaussian noise layer to the input layer for data enhancement, and (III) replaces the pooling layer with a 2D convolutional layer. Thus, it has a stronger feature extraction ability than the original UNet model. In addition, the category imbalance in the glioma data is alleviated and gliomas are efficiently segmented thanks to the profession of the total of GDL and WCE as the failure process in the exercise method. Various experiments were performed to verify the presentation of the proposed structure, confirming its effectiveness in glioma segmentation. Of course, it is obvious that the method based on the improved UNet network has obvious advantages in MRI brain tumor image segmentation and has stronger feature extraction ability than the earlier UNet model. In addition, it can effectively alleviate the classification imbalance of glioma data and effectively segment glioma. We have found that using GDL and subjective cross entropy as loss functions in the training process can effectively alleviate the class imbalance of glioma data and effectively segment glioma. Despite the novel findings, our study has several limitations that should be considered: the 2D networks still have the problem of losing some of the spatial information of 3D images. For instance, the batch size was limited by graphics processing unit (GPU) memory during training and evaluation. We will continue to ameliorate the web based on the difficulties of the 2D network and the issue of too many 3D network parameters to improve the segmentation effect.
Conclusions
Since the traditional image replication processing and machine learning segmentation methods are not ideal in the segmentation of glioma, this paper explores the potential of MRI brain tumor images like the previously published papers as an effective segmentation method for gliomas.
In conclusion, MRI scanning can provide clinical information on the signal characteristics of glioma. It offers important reference value for the degree grading of glioma, provides scientific basis for the accuracy and sensitivity of tumor diagnosis, and is worthy of clinical popularization and application. In addition, the method based on the improved UNet network has obvious advantages in MRI brain tumor image segmentation and has stronger feature extraction ability than the earlier UNet model. Moreover, it can effectively alleviate the class imbalance of glioma data and effectively segment glioma. Our findings demonstrated that the use of GDL and subjective cross entropy as loss functions in the training process effectively alleviated the class imbalance of glioma data and effectively segmented glioma.
Acknowledgments
We thank Hospital of Hubei University of Arts and Science for supporting our research.
Funding: None.
Footnote
Reporting Checklist: The authors have completed the MDAR reporting checklist. Available at https://tcr.amegroups.com/article/view/10.21037/tcr-23-1858/rc
Data Sharing Statement: Available at https://tcr.amegroups.com/article/view/10.21037/tcr-23-1858/dss
Peer Review File: Available at https://tcr.amegroups.com/article/view/10.21037/tcr-23-1858/prf
Conflicts of Interest: All authors have completed the ICMJE uniform disclosure form (available at https://tcr.amegroups.com/article/view/10.21037/tcr-23-1858/coif). The authors have no conflicts of interest to declare.
Ethical Statement: The authors are accountable for all aspects of the work in ensuring that questions related to the accuracy or integrity of any part of the work are appropriately investigated and resolved. The study was conducted in accordance with the Declaration of Helsinki (as revised in 2013). The study was approved by the ethics committee of Hospital of Hubei University of Arts and Science (No. XYS20220518) and individual consent for this retrospective analysis was waived.
Open Access Statement: This is an Open Access article distributed in accordance with the Creative Commons Attribution-NonCommercial-NoDerivs 4.0 International License (CC BY-NC-ND 4.0), which permits the non-commercial replication and distribution of the article with the strict proviso that no changes or edits are made and the original work is properly cited (including links to both the formal publication through the relevant DOI and the license). See: https://creativecommons.org/licenses/by-nc-nd/4.0/.
References
- Paul Y, Mondal B, Patil V, et al. DNA methylation signatures for 2016 WHO classification subtypes of diffuse gliomas. Clin Epigenetics 2017;9:32. [Crossref] [PubMed]
- Macaulay RJ. Impending Impact of Molecular Pathology on Classifying Adult Diffuse Gliomas. Cancer Control 2015;22:200-5. [Crossref] [PubMed]
- Ferris SP, Hofmann JW, Solomon DA, et al. Characterization of gliomas: from morphology to molecules. Virchows Arch 2017;471:257-69. [Crossref] [PubMed]
- Pachocki CJ, Hol EM. Current perspectives on diffuse midline glioma and a different role for the immune microenvironment compared to glioblastoma. J Neuroinflammation 2022;19:276. [Crossref] [PubMed]
- Wu W, Li D, Du J, et al. An Intelligent Diagnosis Method of Brain MRI Tumor Segmentation Using Deep Convolutional Neural Network and SVM Algorithm. Comput Math Methods Med 2020;2020:6789306. [Crossref] [PubMed]
- Chen LZ, Yao Y. Consensus update, promote the standardization of glioma immunity and targeted therapy in China. China Medical News 2020;11.
- Glioma Professional Committee of Neurosurgeon Branch of Chinese Medical Doctor Association. Multidisciplinary Diagnosis and Treatment of Glioma (MDT) Consensus of Chinese Experts. Chinese Journal of Neurosurgery 2018;34:113-8.
- Compilation Group of Guidelines for the Diagnosis and Treatment of Gliomas of the Central Nervous System in China. Guidelines for the diagnosis and treatment of central nervous system gliomas in China (2015). National Medical Journal of China 2016;96:485-509.
- Wang Y, Zhang Y, Navarro L, et al. Multilevel segmentation of intracranial aneurysms in CT angiography images. Med Phys 2016;43:1777. [Crossref] [PubMed]
- Bale TA, Rosenblum MK. The 2021 WHO Classification of Tumors of the Central Nervous System: An update on pediatric low-grade gliomas and glioneuronal tumors. Brain Pathol 2022;32:e13060. [Crossref] [PubMed]
- Chen Z, Li N, Liu C, et al. Deep Convolutional Neural Network-Based Brain Magnetic Resonance Imaging Applied in Glioma Diagnosis and Tumor Region Identification. Contrast Media Mol Imaging 2022;2022:4938587. [Crossref] [PubMed]
- Shattuck D, Sutherling W, Leahy R. Improved cortical MRI segmentation of brains with intracranial grid electrodes. NeuroImage 2001;13:244. [Crossref]
- Ma JY. Postoperative irradiation of intracranial glioma. Zhonghua Shen Jing Jing Shen Ke Za Zhi 1984;17:304-7. [PubMed]
- Jiang J, Hu YC, Liu CJ, et al. Multiple Resolution Residually Connected Feature Streams for Automatic Lung Tumor Segmentation From CT Images. IEEE Trans Med Imaging 2019;38:134-44. [Crossref] [PubMed]
- Hata N, Muragaki Y, Inomata T, et al. Intraoperative tumor segmentation and volume measurement in MRI-guided glioma surgery for tumor resection rate control. Acad Radiol 2005;12:116-22. [Crossref] [PubMed]
- Altameem A, Mallikarjuna B, Saudagar AKJ, et al. Improvement of Automatic Glioma Brain Tumor Detection Using Deep Convolutional Neural Networks. J Comput Biol 2022;29:530-44. [Crossref] [PubMed]
- Stanescu T, Wachowicz K, Fallone B. WE-C-BRB-04: MR Image Susceptibility Dis-tortions: Quantification of Impact On the Radiation Treatment Planning of Cancer Sites. Med Phys 2009;36:2758. [Crossref]
- Kadhim YA, Khan MU, Mishra A. Deep Learning-Based Computer-Aided Diagnosis (CAD): Applications for Medical Image Datasets. Sensors (Basel) 2022;22:8999. [Crossref] [PubMed]
- Botta F, Raimondi S, Rinaldi L, et al. Association of a CT-Based Clinical and Radiomics Score of Non-Small Cell Lung Cancer (NSCLC) with Lymph Node Status and Overall Survival. Cancers (Basel) 2020;12:1432. [Crossref] [PubMed]
- Ferrante M, Rinaldi L, Botta F, et al. Application of nnU-Net for Automatic Segmentation of Lung Lesions on CT Images and Its Implication for Radiomic Models. J Clin Med 2022;11:7334. [Crossref] [PubMed]
- Celli V, Guerreri M, Pernazza A, et al. MRI- and Histologic-Molecular-Based Radio-Genomics Nomogram for Preoperative Assessment of Risk Classes in Endometrial Cancer. Cancers (Basel) 2022;14:5881. [Crossref] [PubMed]
- Yang T, Zhou Y, Li L, et al. DCU-Net: Multi-scale U-Net for brain tumor segmentation. J Xray Sci Technol 2020;28:709-26. [Crossref] [PubMed]
- Manoharan H, Teekaraman Y, Kuppusamy R, et al. An Intellectual Energy Device for Household Appliances Using Artificial Neural Network. Math Probl Eng 2021;2021:7929672. [Crossref]
- Zikic D, Ioannou Y, Brown M, Criminisi A. Segmentation of brain tumor tissues with convolutional neural networks. Proceedings MICCAI-BRATS 2014;36:36-9.
- Pereira S, Pinto A, Alves V, et al. Brain Tumor Segmentation Using Convolutional Neural Networks in MRI Images. IEEE Trans Med Imaging 2016;35:1240-51. [Crossref] [PubMed]
- Neceur MB, Akil M, Saouli R, et al. Deep Convolutional Neural Networks for Brain tumor segmentation: boosting performance using deep transfer learning: preliminary results. In: Crimi A, Bakas S. editors. Brainlesion: Glioma, Multiple Sclerosis, Stroke and Traumatic Brain Injuries. 5th International Workshop, BrainLes 2019, Held in Conjunction with MICCAI 2019, Shenzhen, China, October 17, 2019, Revised Selected Papers, Part II 5. Switzerland: Springer Cham, 2020:303-15.
- Li H, Nan Y, Del Ser J, et al. Region-based evidential deep learning to quantify uncertainty and improve robustness of brain tumor segmentation. Neural Comput Appl 2023;35:22071-85. [Crossref] [PubMed]
- Boehringer AS, Sanaat A, Arabi H, et al. An active learning approach to train a deep learning algorithm for tumor segmentation from brain MR images. Insights Imaging 2023;14:141. [Crossref] [PubMed]
- Zhao C, Tang H, McGonigle D, et al. Development of an approach to extracting coronary arteries and detecting stenosis in invasive coronary angiograms. J Med Imaging (Bellingham) 2022;9:044002. [Crossref] [PubMed]
- Chapiro J, Wood LD, Lin M, et al. Radiologic-pathologic analysis of contrast-enhanced and diffusion-weighted MR imaging in patients with HCC after TACE: diagnostic accuracy of 3D quantitative image analysis. Radiology 2014;273:746-58. [Crossref] [PubMed]
- Zhao Y, Haroun RR, Sahu S, et al. Three-Dimensional Quantitative Tumor Response and Survival Analysis of Hepatocellular Carcinoma Patients Who Failed Initial Transarterial Chemoembolization: Repeat or Switch Treatment? Cancers (Basel) 2022;14:3615. [Crossref] [PubMed]
- Milletari F, Navab N, Ahmadi SA. V-Net: fully convolutional neural networks for volumetric medical image segmentation Proc. Fourth International Conference on 3D Vision (3DV), IEEE 2016, 565-571.
- Cao J, Lai H, Zhang J, et al. 2D-3D cascade network for glioma segmentation in multisequence MRI images using multiscale information. Comput Methods Programs Biomed 2022;221:106894. [Crossref] [PubMed]
- Li X, Arlinghaus LR, Chakravarthy AB, et al. Abstract P4-01-03: quantitative DCE-MRI to predict the response of primary breast cancer to neoadjuvant therapy. Cancer Res 2012;72:P4-01-03-P4-01-03.
- Kshirsagar PR, Manoharan H, Meshram P, et al. Recognition of Diabetic Retinopathy with Ground Truth Segmentation Using Fundus Images and Neural Network Algorithm. Comput Intell Neurosci 2022;2022:8356081. [Crossref] [PubMed]
- Meier R, Porz N, Knecht U, et al. Automatic estimation of extent of resection and residual tumor volume of patients with glioblastoma. J Neurosurg 2017;127:798-806. [Crossref] [PubMed]
- Wykes V, Zisakis A, Irimia M, et al. Importance and Evidence of Extent of Resection in Glioblastoma. J Neurol Surg A Cent Eur Neurosurg 2021;82:75-86. [Crossref] [PubMed]
- Menze BH, Jakab A, Bauer S, et al. The Multimodal Brain Tumor Image Segmentation Benchmark (BRATS). IEEE Trans Med Imaging 2015;34:1993-2024. [Crossref] [PubMed]
- Bakas S, Akbari H, Sotiras A, et al. Advancing The Cancer Genome Atlas glioma MRI collections with expert segmentation labels and radiomic features. Sci Data 2017;4:170117. [Crossref] [PubMed]
- Bakas S, Reyes M, Jakab A, et al. Identifying the Best Machine Learning Algorithms for Brain Tumor Segmentation, Progression Assessment, and Overall Survival Prediction in the BRATS Challenge. arXiv preprint arXiv, 2018;1811:02629. arXiv: 1811.02629.
- Zhu X, Zhu Y, Li L, et al. IoHT-enabled gliomas disease management using fog Computing computing for sustainable societies. Sustainable Cities and Society 2021;74:103215. [Crossref]
- Zhang G, Yang Z, Jiang S. Automatic lung tumor segmentation from CT images using improved 3D densely connected UNet. Med Biol Eng Comput 2022;60:3311-23. [Crossref] [PubMed]
- Zhang TC, Zhang J, Chen SC, et al. A Novel Prediction Model for Brain Glioma Image Segmentation Based on the Theory of Bose-Einstein Condensate. Front Med (Lausanne) 2022;9:794125. [Crossref] [PubMed]
- Lu M, Zhang X, Zhang M, et al. Non-model segmentation of brain glioma tissues with the combination of DWI and fMRI signals. Biomed Mater Eng 2015;26:S1315-24. [Crossref] [PubMed]