Identification of an immune-related signature as a prognostic classifier for patients with early-stage head and neck squamous cell carcinoma
Highlight box
Key findings
• Our novel immune-related gene signature (IRGS) is a reliable and robust classifier for accurate patient stratification and prognostic evaluation.
What is known and what is new?
• Despite advances in early diagnosis and treatment strategies—chemotherapy, surgical resection, and radiotherapy—5-year survival remains grim.
• The incidence of local recurrence is high even in early disease.
What is the implication, and what should change now?
• There is an urgent and unmet need for practical approaches to predict disease progression while considering patient-specific factors.
Introduction
Head and neck cancers are highly aggressive and heterogeneous tumors that originate in the upper aero-digestive tract, including the oral cavity, nasal cavity, larynx, and pharynx (1). Head and neck squamous cell carcinoma (HNSCC) is the most common type and accounts for 90% of all head and neck cancer cases. More than 350,000 new cases are diagnosed worldwide, and 170,000 deaths are reported annually (2). Unfortunately, despite advances in early diagnosis and treatment strategies—chemotherapy, surgical resection, and radiotherapy—5-year survival remains grim, at less than 50% (3). One problem is that many patients are diagnosed with advanced-stage disease. Even among those with early-stage disease, local recurrence may occur in up to 40% (4,5). Consequently, there is an urgent and unmet need for practical approaches to predict disease progression while considering patient-specific factors.
Conventional HNSCC prognostic stratification and clinical management depend on clinicopathological features. Meanwhile, our ability to predict disease course remains insufficient, especially in patients with early-stage HNSCC. The rapid advance and application of high-throughput sequencing technologies have revealed the molecular complexity of HNSCC and offered insight into disease-specific biomarkers (6,7). Numerous studies have affirmed the essential role of gene expression signatures in HNSCC diagnosis, prognostication, surveillance, and treatment (8,9). Portions of the multigene signature have already been incorporated into routine clinical practice; however, we require large, multi-center studies to validate the signature and confirm its predictive value. Information gleaned from such studies should allow us to improve clinical outcomes, particularly in patients with early-stage HNSCC.
The complex immune system plays a decisive role in tumor surveillance. Immune system dysfunction allows cancer cells to evade immune surveillance, facilitating tumor growth, invasion, and metastasis (10,11). Therefore, immunotherapy enhances a tumor-specific immune response and inhibits cancer cell proliferation through active and passive immune system activation (12). Numerous clinical trials of immune checkpoint inhibitors (ICIs) have produced durable clinical responses that are translated into survival benefits in patients with early-stage HNSCC (13-15). Additionally, immune cell infiltration of the tumor microenvironment (TME) could mediate immune responsiveness (10) and may convey a more-favorable prognosis in patients with HNSCC (16,17). Several studies found that the immune-related gene signature (IRGS), determined by RNA sequencing, has significant predictive value in patients with HNSCC. However, the immune-related molecular profiles of the TME and the signature’s predictive value require validation in patients with early-stage HNSCC.
To address this unmet need, we developed an IRGS for risk stratification and prognostication in patients with early-stage HNSCC. Internal and external sample clinical cohorts were applied to validate the IRGS. Finally, the relationship between the IRGS score and underlying mechanisms of immune cell infiltration, checkpoints, and tumor mutation burden (TMB) was analyzed using a bioinformatics approach. We present this article in accordance with the TRIPOD reporting checklist (available at https://tcr.amegroups.com/article/view/10.21037/tcr-23-1791/rc).
Methods
Public cohorts and immune genes sets
We examined 230 early-stage HNSCC samples with gene expression profiles and clinical information, including 100 cases from The Cancer Genome Atlas (TCGA) (https://portal.gdc.cancer.gov/), 49 cases from the Gene Expression Omnibus (GEO; GSE65858) (https://www.ncbi.nlm.nih.gov/geo/), and 81 cases from an independent clinical cohort. Transcriptome data of immune-related genes (IRGs) in which the fragments per kilo base of transcript per million mapped fragments (FPKM) were downloaded for further analysis. The remaining data were log2 transformed and quantile normalized for further analysis. An additional list of immune genes was acquired from the AmiGO 2 Web portal (http://amigo.geneontology.org/amigo/landing).
Clinical specimens
We collected 81 surgically resected, formalin-fixed paraffin-embedded (FFPE) HNSCC tissues from a surgical specimen database in The First Affiliated Hospital of Zhengzhou University. All enrolled patients were pathologically diagnosed as early-stage (I and II) HNSCC, had no other cancers, and no history of pre-surgical treatment. Pathological staging was as per the 8th Edition American Joint Committee on Cancer Staging (18). The study was conducted in accordance with the Declaration of Helsinki (as revised in 2013). The study was approved by the ethics board of The First Affiliated Hospital of Zhengzhou University (No. KY-2021-0403) and informed consent was waived due to retrospective nature of this study. Demographic and clinical data are summarized in Table S1.
Quantitative real-time polymerase chain reaction (qRT-PCR) analysis
We used qRT-PCR to determine the nine immune gene signatures from FFPE surgical specimens. We extracted the total RNA from 81 samples using the RNAiso Plus reagent (Takara, Beijing, China, #9109). RNA concentration was quantified using a NanoDrop 2000C spectrophotometer (Thermo Scientific, Waltham, MA, USA), and an A260/A280 ratio of 1.8:2.1 was considered highly purified RNA. Then, cDNA was synthesized, and qRT-PCR was performed using SYBR Premix Ex Taq II (Takara, #RR820A), further analyzed by Agilent Mx3005P (Los Angeles, USA). We used the glyceraldehyde-3-phosphate dehydrogenase (GAPDH) expression as a referent for quantification assays, and the ΔCt values were considered sufficiently stable for further analysis. The primer sequence of the selected immune genes is presented in Table S2.
Functional enrichment analyses
Gene Ontology (GO) analyses were used to explore IRGS biological processes and pathway enrichment using DAVID 6.8 (http://david.abcc.ncifcrf.gov/).
Estimation of immune cell infiltration
Immune cell infiltration was estimated using the Microenvironment Cell Populations-counter (MCP-counter). This method quantifies immune and stromal cells from RNA-sequencing data (19).
Gene set variation analysis (GSVA)
Inflammatory metagenes clusters acted as surrogate markers for the diverse immune cells, including seven types of LCK, IgG, HCK, STAT1, interferon, MHC-I, and MHC-II. GSVA was used to transform gene expression values into immune-related metagene set scores, followed by correlograms to investigate the relationship between IRGS and metagenes (20).
Signature construction and statistical analysis
The prognostic IRGS was identified based on training cohorts (TCGA), followed by validation of the predictive value in the test set (GSE65858) and an external validation cohort (clinical samples obtained from The First Affiliated Hospital of Zhengzhou University). The Kaplan-Meier method estimated relationships between immune genes and prognosis in the TCGA and GSE65858 cohorts. Prognostic-related immune genes with P<0.05 were selected. After the intersection of prognostic immune genes was identified in the two datasets, ten immune genes were selected. Then, the optimal signature was further constructed through least absolute shrinkage and selection operator (LASSO) regression analysis. The IRGS score was calculated based on the expression values of the nine selected immune genes with corresponding coefficients. Patients were separated into high- or low-risk groups based on the optimal cutoff point. A Kaplan-Meier analysis was used to determine the prognostic value of the identified signature in the training and validation cohorts, and between-group differences were compared using the log-rank test. The signature’s ability was assessed using time-dependent receiver operating characteristic (ROC) curves. Pearson’s correlation analysis was performed to measure the statistical correlation. All statistical analyses were conducted using R software version 3.5.3 (https://www.r-project.org) with two-tail P values <0.05 considered significant.
Results
Identification of differentially expressed genes (DEGs) in patients with early-stage HNSCC
To characterize the immune profiles associated with early-stage HNSCC, we initially included 707 immune genes. Through Kaplan-Meier analysis, we identified 83 genes with a significant relationship to prognosis from the TCGA dataset and 693 genes from GSE65858 dataset. After cross-referencing all prognosis related genes from the two databases, we retained ten immune genes capable of estimating prognosis for HNSCCs, including three with HR >1 and seven with HR <1 (Figure 1A,1B). Then, we used a LASSO COX regression analysis to screen out the most important prognostically related genes in early-stage HNSCC. The nine-immune-gene signature was constructed based on the optimum λ value (Figure 1C,1D). The corresponding coefficients of nine IRGs are shown in Figure 1E and six protective and three risk-enhancing genes were included. The IRGS was constructed based on the following formula: Risk score = (−0.5284 × METTL3 expression) + (−0.4777 × PRKCH expression) + (−0.4107 × NLRC5 expression) + (−0.1916 × IRAK4 expression) + (−0.0986 × PLD1 expression) + (−0.0273 × IFNE expression) + (0.1128 × NLRP2 expression) + (0.1602 × HLA-DQA2 expression) + (0.1734 × TNFSF9 expression). The correlation matrix also showed a close relationship between the nine selected genes and corresponding risk scores. This suggested a close functional relationship among the genes (Figure 1F).
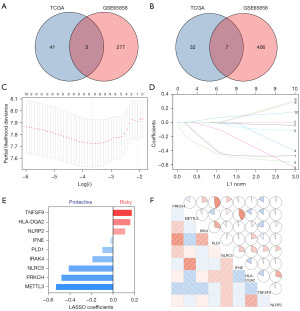
Construction of the IRGS in patients with early-stage HNSCC
Each patient’s IRGS score was determined based on the model formula. We used these scores to stratify patients into high- and low-risk groups according to the optimal cutoff point (−13.523) in TCGA dataset. Figure 2A depicts the relationships among gene expression, IRGS score, and survival status. Then, a principal component analysis (PCA) showed significant heterogeneity between the high- and low-risk IRGS groups (Figure 2B). We further explored the prognostic significance of the IRGS score in the training cohort [hazard ratio (HR) =13.795, 95% confidence interval (CI): 3.275–58.109, P<0.001]. Here, patients with scores indicating high-risk demonstrated significantly worse overall survival (OS) (Figure 2C). This was true for patients with either stage I or II HNSCC (Figure 2D,2E). The Kaplan-Meier curve also showed significant between-group differences in relapse-free survival (RFS) (optimal cutoff point =−12.471, HR =10.520, 95% CI: 3.094–35.771, P<0.001) (Figure 2F).
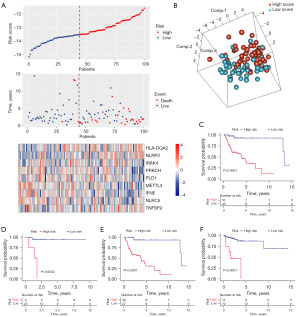
Validation of IRGS score in multiple early-stage HNSCC cohorts
To further validate the reliability, robustness, and predictive value of the IRGS score in patients with early-stage HNSCC, 49 cases from the GEO dataset (GSE65858) and 81 cases from an independent clinical cohort with qRT-PCR data were integrated into our study. In the GSE65858 dataset, patients with early-stage HNSCC were divided into high- and low-risk score groups based on the optimal cutoff point (Figure 3A). The IRGS score showed excellent predictive stability, and patients with high-risk scores exhibited shorter OS than those with low scores for stage I and II HNSCC (OS, HR 19.940, 95% CI: 2.280–174.299; RFS, HR 3.588, 95% CI: 1.284–10.026, P<0.001) (Figure 3B,3C). The clinical application of IRGS was also validated in an independent clinical FFPE cohort with quantitative polymerase chain reaction (qPCR) data from The First Affiliated Hospital of Zhengzhou University. We calculated IRGS scores based on the same formula, and patients were classified into high-and low-score groups using the optimal cutoff point (Figure 3D). As expected, the IRGS score accurately estimated OS in patients with early-stage HNSCC (Figure 3E). Once again, we observed significant between-group differences in RFS (HR =9.995, 95% CI: 3.027–33.002, P<0.001) (Figure 3F).
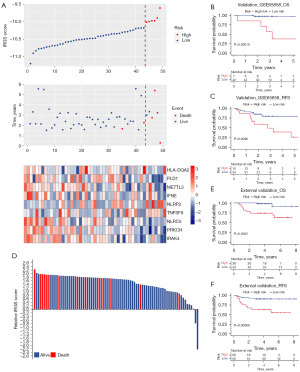
We additionally explored the prognostic value of the IRGS score across different clinical subgroups based on age, sex, smoking status, alcohol consumption, tumor stage, and IRGS score. Our signature demonstrated strong and stable predictive value for estimating OS and RFS in patients with early-stage HNSCC, with remarkably consistent results noted across cohorts. Patients with low IRGS scores experienced better clinical outcomes across different clinical subtypes, including age (old/young), sex (male/female), smoking status (smoker/non-smoker), alcohol (drinker/non-drinker), and tumor stage (stage I/II HNSCC). These results are presented in Figures S1-S3.
Evaluation of the predictive performance of the IRGS score in patients with early-stage HNSCC
We further evaluated the predictive performance of IRGS on early-stage HNSCCs using time-dependent ROC curves and C-indices. The area under the curves (AUCs) for predicting 1-, 3-, and 5- year OS were 0.738, 0.661, and 0.715 in the training cohort, and outperformed than other clinical recognized risk factors (Figure 4A-4C). Thus, the IRGS score could accurately predict survival in patients with early-stage HNSCC. Subsequently, we also determined the predictive performance of the IRGS score in the validation and clinical cohorts. The IRGS scores achieved AUCs for estimating 3-, 5-year OS of 0.766 and 0.822 in the validation cohort and 0.755 and 0.714 in the independent clinical cohort (Figure 4D-4G). The C-index further confirmed the predictive value of the IRGS score compared with other clinical factors (Figure 4H,4I). The IRGS score C-index was higher than other clinicopathological parameters (Figure 4H). C-index values of IRGS and clinical parameters for OS is in the training and validation cohort, respectively (Figure 4I).
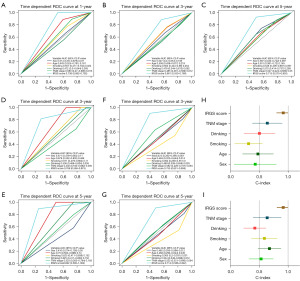
To determine if the IRGS score independently predicted prognosis in patients with early-stage HNSCC, we carried out univariate and multivariable analyses. These revealed that disease stage and IRGS score demonstrated predictive value in patients with early-stage HNSCC included within the training cohort. IRGS score and drinking were predictive within the validation cohort. Finally, IRGS score and stage were predictive within the clinical cohort. After integrating the important clinical parameters in multivariable Cox regression analysis, IRGS score was the only stable predictor of prognosis in patients with early-stage HNSCC (Tables S3,S4).
IRGS score-related biological pathways and inflammation
The mechanisms contributing to IRGS score were examined using Pearson correlation analysis to identify 707 strongly associated genes (|R|≥0.3), and heatmap is presented in Figure 5A. Then we found the selected genes were enriched in immune response pathways (Figure 5B). We also enrolled seven metagenes to investigate the relationship between IRGS and inflammation (Figure 5C). Heatmaps and correlation matrix indicated a close relationship with IRGS score; IRGS score was positively correlated with HCK, IgG, and MHC-II and negatively correlated with interferon, MHC-I, and STAT1 (Figure 5D).
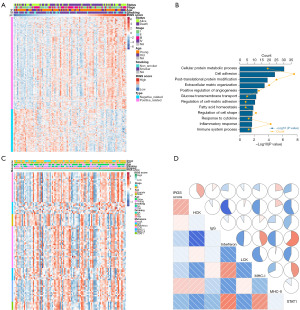
Exploration of IRGS score related immune landscape and immune checkpoints
The MCP-counter method was used to examine immune cell and stromal cell infiltration relative to IRGS score (Figure 6A); there was a positive correlation between IRGS score and immune cell infiltration—especially fibroblasts and myeloid dendritic cells (Figure 6B,6C). Considering the importance of immune checkpoints for maintaining immune homeostasis, we investigated the relationship between the IRGS score and typical immune checkpoints from the B7-CD28 and other families. We found a positive relationship between the IRGS score and TNFSF4, TNFRSF4, CD70, TNFSF9, TNFRSF9, SIGLEC15, CD276, ICOSLG, VTCN1, and HAVCR2 (Figure 6D,6E).
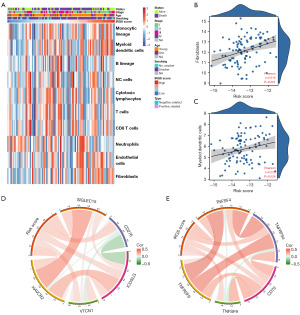
Discussion
Patients with early-stage HNSCC are at high risk of recurrence, metastasis, and death, even after radical surgery. There is increasing interest in finding reliable prognostic biomarkers capable of identifying patients at increased survival risk. Many studies have found the TME influences tumor progression, highlighting its potential clinical application. In contrast, little is known about the predictive role of IRGs in early-stage HNSCC. Therefore, there is a pressing need for exploring and developing an IRGS score for prognostication in patients with early-stage HNSCC.
This is the first integrative analysis of IRGs among 194 patients with early-stage HNSCC using three large-scale cohorts. This analysis identified a nine-gene IRGS that accurately stratified patients into high- and low-risk groups. The IRGS score accurately estimated OS and RFS in the training cohort, and its predictive performance further validated using internal and external cohorts. Furthermore, the IRGS score could independently predict prognosis in patients with early-stage HNSCC and even performed better—across cohorts—than multiple clinical parameters. Lastly, we investigated inflammatory profiles related to the IRGS score.
We first screened out ten genes correlated with tumor initiation and progression that could act as biomarkers for diagnosis or prognostication. Then, using LASSO cox regression, the prognostic signature of nine-immune genes was constructed, including three risk-enhancing genes (NLRP2, TNFSF9, and HLA-DQA2) and six protective genes (METTL3, PRKCH, NLRC5, IRAK4, PLD1, and IFNE). TNFSF9—also known as 4-1BBL or CD137L—belongs to the tumor necrosis factor (TNF) family and is expressed on the surface of antigen-presenting cells and various tumor cells. The interaction between TNFRSF9 and TNFSF9 could create bidirectional signals between activated and antigen-presenting cells. This process may mediate complex immune responses in cancer, autoimmune, infectious, and inflammatory diseases (21). TNFSF9 is identified as a prognostic risk factor for HNSCC due to immune cell regulation or cytokine release within the TME (22). NLRP2 belongs to the nucleotide-binding and leucine-rich repeat receptor (NLR) family. High NLRP2 expression has been associated with poor prognosis in HNSCC; however, its biological mechanisms require further exploration (23). HLA-DQA2, belonging to the HLA class II alpha chain family, is associated with worse prognosis (24). PRKCH belongs to the protein kinase C family and helps regulate apoptosis and anti-apoptosis (25,26). PRKCH is associated with better survival in patients with head and neck cancer, which is consistent with our findings (27). NLRC5 is an essential transcriptional regulator for MHC class I genes (28) and its overexpression has been found to link to CD8+ T cell activation, enhanced tumor immunogenicity, and the immune escape response (29). NLRC5 is a prognostic marker for various types of cancer (30,31). IRAK4 is a serine/threonine kinase and aids scaffolding and phosphorylation in Toll-like receptor (TLR) and interleukin (IL)-1R pathways (32). IRAK4 is associated with less-favorable cancer types, including pancreatic ductal adenocarcinoma (33) and glioma (34). In contrast, IRAK4 has a protective function in patients with early-stage HNSCC, influenced mainly by tumor heterogeneity. PLD1 hydrolyzes phosphatidylcholine and is involved in thrombosis and cancer (35). PLD1 has a protective function in patients with early-stage HNSCC (although the relevant mechanisms require further elucidation). METTL3 is the main catalytic enzyme in the N6-adenosine-methyltransferase system (36,37) and is upregulated in several cancers. Its presence has therefore been correlated with worse prognosis (38,39). METTL3 may suppress some tumor types (40) and corresponds with a better prognosis in patients with early-stage HNSCC. IFNE regulates apoptosis and may inhibit colorectal cancer cell proliferation (41). It participates in immune system and cytokine signaling pathways (42). IFNE is associated with a better prognosis in patients with early-stage HNSCC; however, its mechanisms require further investigation.
In terms of clinical relevance, the IRGS score along with clinical parameters was significantly correlated to OS and RFS in patients with HNSCC. In tumor stage subgroups (including stage I and II), the IRGS score could predict OS in the training, internal, and external validation cohorts. Alcohol and tobacco intake are established risk factors for HNSCC and influence nutrient status and immune system (43). Thus, we explored the application of the IRGS in those subgroups. As excepted, the IRGS score was better at estimating prognosis among drinking and non-drinking and smoking and non-smoking groups. Next, considering lymph node metastasis is a crucial risk factor for prognostication, we explored the association of the IRGS score and clinical outcome (OS and RFS) in positive (LN+) and negative (LN−) lymph node metastasis groups. Due to the limitation of sample size after subgrouping, we found that the IRGS score still demonstrated stable prognostic ability in the external validation cohort. We also tested its predictive value in sex- and age-based subgroups where it performed well across males and females and old and young patients. Collectively, these results confirmed the predictive value of the IRGS score in patients with early-stage HNSCC.
Our biological pathway analysis indicated that the IRGS score was closely associated with the inflammatory response and immune system process pathways, both of which contribute to the immune response. The IRGS score was consistently and positively related to HCK, IgG, and MHC-II. Considering the importance of immune cell infiltration of the TME for tumor growth, MCP was used to examine the proportions of 12 types of immune cells in HNSCC based on gene expression. The abundance of immune cells reflects a patient’s immune status. High-risk patients had more immune cell infiltration, including fibroblasts and myeloid dendritic cells. Previous studies reported fibroblasts are associated with increased recurrence risk and poor prognosis in certain solid tumors (44-46). As an essential component of the TME, fibroblasts play critical roles in tumor transformation, progression, and drug resistance, consistent with our results.
We also explored potential links between the IRGS score and immune checkpoints—including members from the B7-CD28, TNF, and other families. Here, IRGS score was positively correlated with molecules from the B7-CD28 (Siglec-15, CD276, ICOSLG, VTCN1, and HAVCR2) and TNF families (TNFSF4, TNFRSF4, CD70, TNFSF9, and TNFRSF9). These molecules are involved in the transmission of negative or positive signals to T-cells and may determine the nature of the immune response when an antigen is required for T-cell activation (47,48). Our results alert us that the high-risk patients were more likely to benefit from ICI-based therapies.
There are several limitations in our study. First, the signature was established based on retrospective data, and large, well-designed, prospective studies are necessary to further optimize the IRGS score. Additionally, the signature was identified using public datasets and constructed using bioinformatics analysis. Finally, the results may have also been influenced by noise.
To summarize, the IRGS score identified by our team may provide a legitimate approach for clinical management in patients with early-stage HNSCC. The signature can accurately and effectively predict survival. Furthermore, exploring the signature-related immune landscape suggests a positive correlation between immune cells and the inflammatory response; this link is relevant to future development of personalized treatments in the era of immunotherapy.
Conclusions
Our novel IRGS is a reliable and robust classifier for accurate patient stratification and prognostic evaluation. Future studies will attempt to affirm the signature’s clinical application to early-stage HNSCC.
Acknowledgments
All authors would like to thank the specimen donors and research groups for the TCGA and GSE65858 cohort. All authors would also like to thank CapitalBio Technology for the technical support.
Funding: This work was supported by
Footnote
Reporting Checklist: We present this article in accordance with the TRIPOD reporting checklist. Available at https://tcr.amegroups.com/article/view/10.21037/tcr-23-1791/rc
Data Sharing Statement: Available at https://tcr.amegroups.com/article/view/10.21037/tcr-23-1791/dss
Peer Review File: Available at https://tcr.amegroups.com/article/view/10.21037/tcr-23-1791/prf
Conflicts of Interest: All authors have completed the ICMJE uniform disclosure form (available at https://tcr.amegroups.com/article/view/10.21037/tcr-23-1791/coif). The authors have no conflicts of interest to declare.
Ethical Statement: The authors are accountable for all aspects of the work in ensuring that questions related to the accuracy or integrity of any part of the work are appropriately investigated and resolved. The study was conducted in accordance with the Declaration of Helsinki (as revised in 2013). The study was approved by the ethics board of The First Affiliated Hospital of Zhengzhou University (No. KY-2021-0403) and informed consent was waived due to retrospective nature of this study.
Open Access Statement: This is an Open Access article distributed in accordance with the Creative Commons Attribution-NonCommercial-NoDerivs 4.0 International License (CC BY-NC-ND 4.0), which permits the non-commercial replication and distribution of the article with the strict proviso that no changes or edits are made and the original work is properly cited (including links to both the formal publication through the relevant DOI and the license). See: https://creativecommons.org/licenses/by-nc-nd/4.0/.
References
- Leemans CR, Braakhuis BJ, Brakenhoff RH. The molecular biology of head and neck cancer. Nat Rev Cancer 2011;11:9-22. [Crossref] [PubMed]
- Sung H, Ferlay J, Siegel RL, et al. Global Cancer Statistics 2020: GLOBOCAN Estimates of Incidence and Mortality Worldwide for 36 Cancers in 185 Countries. CA Cancer J Clin 2021;71:209-49. [Crossref] [PubMed]
- Marur S, Forastiere AA. Head and Neck Squamous Cell Carcinoma: Update on Epidemiology, Diagnosis, and Treatment. Mayo Clin Proc 2016;91:386-96. [Crossref] [PubMed]
- Chan JYK, Zhen G, Agrawal N. The role of tumor DNA as a diagnostic tool for head and neck squamous cell carcinoma. Semin Cancer Biol 2019;55:1-7. [Crossref] [PubMed]
- Chauhan SS, Kaur J, Kumar M, et al. Prediction of recurrence-free survival using a protein expression-based risk classifier for head and neck cancer. Oncogenesis 2015;4:e147. [Crossref] [PubMed]
- Hong M, Tao S, Zhang L, et al. RNA sequencing: new technologies and applications in cancer research. J Hematol Oncol 2020;13:166. [Crossref] [PubMed]
- Magnes T, Wagner S, Kiem D, et al. Prognostic and Predictive Factors in Advanced Head and Neck Squamous Cell Carcinoma. Int J Mol Sci 2021;22:4981. [Crossref] [PubMed]
- Alsahafi E, Begg K, Amelio I, et al. Clinical update on head and neck cancer: molecular biology and ongoing challenges. Cell Death Dis 2019;10:540. [Crossref] [PubMed]
- Oliva M, Spreafico A, Taberna M, et al. Immune biomarkers of response to immune-checkpoint inhibitors in head and neck squamous cell carcinoma. Ann Oncol 2019;30:57-67. [Crossref] [PubMed]
- Yoshihara K, Shahmoradgoli M, Martínez E, et al. Inferring tumour purity and stromal and immune cell admixture from expression data. Nat Commun 2013;4:2612. [Crossref] [PubMed]
- Beatty GL, Gladney WL. Immune escape mechanisms as a guide for cancer immunotherapy. Clin Cancer Res 2015;21:687-92. [Crossref] [PubMed]
- Baxter D. Active and passive immunization for cancer. Hum Vaccin Immunother 2014;10:2123-9. [Crossref] [PubMed]
- Ferris RL, Blumenschein G Jr, Fayette J, et al. Nivolumab for Recurrent Squamous-Cell Carcinoma of the Head and Neck. N Engl J Med 2016;375:1856-67. [Crossref] [PubMed]
- Cohen EEW, Soulières D, Le Tourneau C, et al. Pembrolizumab versus methotrexate, docetaxel, or cetuximab for recurrent or metastatic head-and-neck squamous cell carcinoma (KEYNOTE-040): a randomised, open-label, phase 3 study. Lancet 2019;393:156-67. [Crossref] [PubMed]
- Burtness B, Harrington KJ, Greil R, et al. Pembrolizumab alone or with chemotherapy versus cetuximab with chemotherapy for recurrent or metastatic squamous cell carcinoma of the head and neck (KEYNOTE-048): a randomised, open-label, phase 3 study. Lancet 2019;394:1915-28. [Crossref] [PubMed]
- Peltanova B, Raudenska M, Masarik M. Effect of tumor microenvironment on pathogenesis of the head and neck squamous cell carcinoma: a systematic review. Mol Cancer 2019;18:63. [Crossref] [PubMed]
- Elmusrati A, Wang J, Wang CY. Tumor microenvironment and immune evasion in head and neck squamous cell carcinoma. Int J Oral Sci 2021;13:24. [Crossref] [PubMed]
- Zanoni DK, Patel SG, Shah JP. Changes in the 8th Edition of the American Joint Committee on Cancer (AJCC) Staging of Head and Neck Cancer: Rationale and Implications. Curr Oncol Rep 2019;21:52.
- Becht E, Giraldo NA, Lacroix L, et al. Estimating the population abundance of tissue-infiltrating immune and stromal cell populations using gene expression. Genome Biol 2016;17:218. [Crossref] [PubMed]
- Rody A, Holtrich U, Pusztai L, et al. T-cell metagene predicts a favorable prognosis in estrogen receptor-negative and HER2-positive breast cancers. Breast Cancer Res 2009;11:R15. [Crossref] [PubMed]
- Shuh M, Bohorquez H, Loss GE Jr, et al. Tumor Necrosis Factor-α: Life and Death of Hepatocytes During Liver Ischemia/Reperfusion Injury. Ochsner J 2013;13:119-30. [PubMed]
- Wu J, Wang Y, Yang Y, et al. TNFSF9 promotes metastasis of pancreatic cancer through Wnt/Snail signaling and M2 polarization of macrophages. Aging (Albany NY) 2021;13:21571-86. [Crossref] [PubMed]
- Wang J, Chen X, Tian Y, et al. Six-gene signature for predicting survival in patients with head and neck squamous cell carcinoma. Aging (Albany NY) 2020;12:767-83. [Crossref] [PubMed]
- Lenormand C, Bausinger H, Gross F, et al. HLA-DQA2 and HLA-DQB2 genes are specifically expressed in human Langerhans cells and encode a new HLA class II molecule. J Immunol 2012;188:3903-11. [Crossref] [PubMed]
- Porter SN, Magee JA. PRKCH regulates hematopoietic stem cell function and predicts poor prognosis in acute myeloid leukemia. Exp Hematol 2017;53:43-7. [Crossref] [PubMed]
- Abu-Ghanem S, Oberkovitz G, Benharroch D, et al. PKCeta expression contributes to the resistance of Hodgkin's lymphoma cell lines to apoptosis. Cancer Biol Ther 2007;6:1375-80. [Crossref] [PubMed]
- Schaafsma E, Fugle CM, Wang X, et al. Pan-cancer association of HLA gene expression with cancer prognosis and immunotherapy efficacy. Br J Cancer 2021;125:422-32. [Crossref] [PubMed]
- Meissner TB, Li A, Biswas A, et al. NLR family member NLRC5 is a transcriptional regulator of MHC class I genes. Proc Natl Acad Sci U S A 2010;107:13794-9. [Crossref] [PubMed]
- Chelbi ST, Guarda G. NLRC5, a promising new entry in tumor immunology. J Immunother Cancer 2016;4:39. [Crossref] [PubMed]
- Shukla A, Cloutier M, Appiya Santharam M, et al. The MHC Class-I Transactivator NLRC5: Implications to Cancer Immunology and Potential Applications to Cancer Immunotherapy. Int J Mol Sci 2021;22:1964. [Crossref] [PubMed]
- Cho SX, Vijayan S, Yoo JS, et al. MHC class I transactivator NLRC5 in host immunity, cancer and beyond. Immunology 2021;162:252-61. [Crossref] [PubMed]
- Kargbo RB. PROTAC Degradation of IRAK4 for the Treatment of Cancer. ACS Med Chem Lett 2019;10:1370-1. [Crossref] [PubMed]
- Zhang D, Li L, Jiang H, et al. Constitutive IRAK4 Activation Underlies Poor Prognosis and Chemoresistance in Pancreatic Ductal Adenocarcinoma. Clin Cancer Res 2017;23:1748-59. [Crossref] [PubMed]
- Li J, Sun Y, Ma Y, et al. Comprehensive Pan-Cancer Analysis of IRAK Family Genes Identifies IRAK1 as a Novel Oncogene in Low-Grade Glioma. J Oncol 2022;2022:6497241. [Crossref] [PubMed]
- Bowling FZ, Salazar CM, Bell JA, et al. Crystal structure of human PLD1 provides insight into activation by PI(4,5)P(2) and RhoA. Nat Chem Biol 2020;16:400-7. [Crossref] [PubMed]
- Zheng W, Dong X, Zhao Y, et al. Multiple Functions and Mechanisms Underlying the Role of METTL3 in Human Cancers. Front Oncol 2019;9:1403. [Crossref] [PubMed]
- Yankova E, Blackaby W, Albertella M, et al. Small-molecule inhibition of METTL3 as a strategy against myeloid leukaemia. Nature 2021;593:597-601. [Crossref] [PubMed]
- Xia TL, Yan SM, Yuan L, et al. Upregulation of METTL3 Expression Predicts Poor Prognosis in Patients with Esophageal Squamous Cell Carcinoma. Cancer Manag Res 2020;12:5729-37. [Crossref] [PubMed]
- Liu K, Gao Y, Gan K, et al. Prognostic Roles of N6-Methyladenosine METTL3 in Different Cancers: A System Review and Meta-Analysis. Cancer Control 2021;28:1073274821997455. [Crossref] [PubMed]
- Cai Y, Feng R, Lu T, et al. Novel insights into the m(6)A-RNA methyltransferase METTL3 in cancer. Biomark Res 2021;9:27. [Crossref] [PubMed]
- Paschall AV, Liu K. Epigenetic Regulation of Apoptosis and Cell Cycle Regulatory Genes in Human Colon Carcinoma Cells. Genom Data 2015;5:189-91. [Crossref] [PubMed]
- Fang S, Lu J, Zhou X, et al. Functional annotation of melanoma risk loci identifies novel susceptibility genes. Carcinogenesis 2020;41:452-7. [Crossref] [PubMed]
- Sawabe M, Ito H, Oze I, et al. Heterogeneous impact of alcohol consumption according to treatment method on survival in head and neck cancer: A prospective study. Cancer Sci 2017;108:91-100. [Crossref] [PubMed]
- Nishina T, Deguchi Y, Ohshima D, et al. Interleukin-11-expressing fibroblasts have a unique gene signature correlated with poor prognosis of colorectal cancer. Nat Commun 2021;12:2281. [Crossref] [PubMed]
- Li X, Sun Z, Peng G, et al. Single-cell RNA sequencing reveals a pro-invasive cancer-associated fibroblast subgroup associated with poor clinical outcomes in patients with gastric cancer. Theranostics 2022;12:620-38. [Crossref] [PubMed]
- Heichler C, Scheibe K, Schmied A, et al. STAT3 activation through IL-6/IL-11 in cancer-associated fibroblasts promotes colorectal tumour development and correlates with poor prognosis. Gut 2020;69:1269-82. [Crossref] [PubMed]
- Chen L, Flies DB. Molecular mechanisms of T cell co-stimulation and co-inhibition. Nat Rev Immunol 2013;13:227-42. [Crossref] [PubMed]
- Chen L. Co-inhibitory molecules of the B7-CD28 family in the control of T-cell immunity. Nat Rev Immunol 2004;4:336-47. [Crossref] [PubMed]