A pyroptosis-related lncRNA-based prognostic index for hepatocellular carcinoma by relative expression orderings
Highlight box
Key findings
• Our study constructed a novel index based on pyroptosis and long non-coding RNA (lncRNA), which can effectively predict the prognosis of Hepatocellular carcinoma (HCC) patients.
What is known and what is new?
• Pyroptosis plays an important role in the progression of tumors, and lncRNAs are used as a reliable biomarker to predict tumors progression.
• A reliable pyroptosis-related index was constructed by relative expression orderings and robustness for clinical application. Additionally, the index showed the potential drugs for different stratification patients.
What is the implication, and what should change now?
• The index could offer a unique perspective for improving the clinical decision-making process for patients in different stratification and improve outcomes for patients with HCC.
Introduction
Hepatocellular carcinoma (HCC), as one of the most common cancers worldwide, is the third-leading cause of worldwide mortality of various cancers and its incidence rate per year remains high (1). Although liver transplantation and surgical resection are the backbones of curative therapies for HCC, the overall survival (OS) rate of patients with advanced HCC is still strongly unsatisfactory, with a postoperative 5-year recurrence rate of approximately 70% primarily due to tumor heterogeneity (2,3). Therefore, the urgent identification of reliable biomarkers and the establishment of a molecular prognostic signature for HCC are imperative. These advancements are not only important for aiding in the early detection of HCC but also critical for substantially improving the prognosis of patients through earlier diagnosis and precisely targeted therapeutic intervention.
Long non-coding RNAs (lncRNAs) are non-protein coding transcripts over 200 nucleotides in length, which are often dysregulated during tumorigenesis and might cause tumor development (4). As a result, they are used as valuable molecular biomarkers for the diagnosis and prediction of various types of cancers. For example, lncRNA H19 was reported to promote the cell pyroptosis by targeting miR-22-3p/NLRP3 axis in pneumonia, which could serve as an important curative marker (5). Chen et al. found that lncRNA SNHG7 expression led to NLRP3-dependent pyroptosis in HCC through the inhibition of NLR family domain and the upregulation of miR-34a, suggesting that SNHG7 may be regarded as a promising biomarker in HCC treatment outcomes (6). Liu et al. discovered that the overexpression of lncRNA HOTAIR may act as a marker of poor prognosis outcome and contribute to a better understanding of gastric carcinogenesis progression and metastasis (7).
Pyroptosis is a form of programmed cell death characterized by its inflammatory nature, typically initiated by the activation of caspase-1 or caspase-11 within inflammasomes in response to inflammatory signals. This process leads to the activation of gasdermin D and the disruption of the cell membrane, which facilitates the release of cellular contents and the generation of an inflammatory response, culminating in inflammatory cell death (8,9). Recent researches have highlighted that pyroptosis plays a crucial role in tumor suppression and cancer therapeutics (10-12). Based on these findings, researchers have proposed pyroptosis-based strategies to identify potential biomarkers and construct prognostic models associated with various types of cancers. For example, Yang et al. constructed a risk model based on 10 pyroptosis-related lncRNAs in breast cancer, which showed great prognostic prediction ability (13). Song et al. constructed a pyroptosis‐related prognostic model for colorectal cancer, which could provide help in the choice of immunotherapy strategies and chemotherapy drugs for patients (14). Wang et al. proposed a prognostic signature based on pyroptosis‐related genes, potentially offering the optimal therapeutic schedule for multiple myeloma patients (15). Zhou et al. built a pyroptosis-related prognostic signature which had the potential to offer novel directions for immunotherapy in lung squamous cell carcinoma (16), and so on.
The aforementioned pyroptosis-related studies have made contributions to the identification of biomarkers and the establishment of prognostic model for various cancers. However, most of them are based on the quantitative expression value of genes and thus lack robustness for clinical applications, mainly because these methods require the pre-collection of samples for data normalization and there is a need to eliminate the batch effect (17,18). These requirements may not be feasible for clinical practice as they add complexity to sample collection and processing. Fortunately, research has shown that the within-sample relative expression orderings (REOs) of gene pairs are robust against the influence of batch effects and data normalization, which is an individual qualitative transcriptional trait (19,20). Consequently, several individual qualitative signatures have been proposed based on the with-sample REOs of gene pairs for predicting the prognosis of several cancers, including that of HCC (21-23). However, it is important to note that the most recent REO-based prognostic model associated with pyroptosis for HCC still has limitations, including limited consideration of the number of pyroptosis datasets and a lack of independent external sets for validation, etc. To overcome these limitations, it is necessary to develop a more reliable prognostic model for HCC, which can be better applied in clinical practice, providing more accurate prognosis assessment and treatment guidance for HCC patients.
In this study, we developed a novel pyroptosis-related lncRNA-based prognostic index (PLPI) using a set of 10 pyroptosis-related lncRNA pairs. The results demonstrated that PLPI exhibited great performance in predicting the prognosis of HCC patients, indicating its potential to aid personalized therapy and management of HCC patients. The workflow of this study is shown in Figure 1. We present this article in accordance with the TRIPOD reporting checklist (available at https://tcr.amegroups.com/article/view/10.21037/tcr-23-1804/rc).
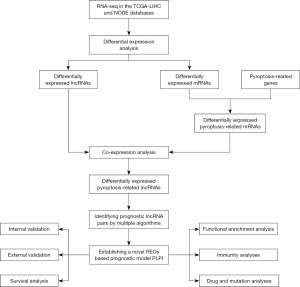
Methods
Data collection
In this study, we selected two independent databases from different high-throughput platforms, including 374 HCC samples and 50 normal samples from The Cancer Genome Atlas-Liver Hepatocellular Carcinoma (TCGA-LIHC) database (https://xena.ucsc.edu/public), and 159 HCC samples from the National Omics Data Encyclopedia (NODE) database (https://www.biosino.org/node/). Additionally, we extracted gene expression data and matching clinical information from these two databases. Patients with an OS time of less than 30 days and incomplete clinical information were excluded from the survival analysis. The study was conducted in accordance with the Declaration of Helsinki (as revised in 2013). There were 155 pyroptosis-related genes obtained from a previous study (24), which are shown in table available at https://cdn.amegroups.cn/static/public/tcr-23-1804-1.pdf.
Differentially expressed pyroptosis-related lncRNAs (DEPR-lncRNAs)
First, lncRNAs and messenger RNAs (mRNAs) were identified for further analyses using the annotation files (hg38.99) downloaded from the Ensemble database (https://ensemblgenomes.org/). The expression profile of mRNAs and lncRNAs extracted from RNA sequencing (RNA-seq) count data was normalized using the edgeR package (version 3.32.1). Then, differentially expressed mRNAs (DEmRNAs) and differentially expressed lncRNAs (DElncRNAs) shared by the TCGA-LIHC and NODE databases were identified by the Wilcoxon rank-sum test [|log2(fold change)| >1, false discovery rate (FDR) <0.05]. The intersections between the DEmRNAs and the 155 pyroptosis-related genes were considered as DEPR-mRNAs. To identify the DEPR-lncRNAs, Pearson correlation analysis was performed to construct the co-expression network between the DEPR-mRNAs and the DElncRNAs. Finally, the DElncRNAs with |R2|>0.4 and P<0.001 in the co-expression network remained as the DEPR-lncRNAs.
Identification of the prognostic pyroptosis-related lncRNA pairs
Initially, we conducted univariate Cox regression analysis in the TCGA-LIHC database to identify the significant prognostic DEPR-lncRNAs. These DEPR-lncRNAs were then used to construct the DEPR-lncRNA pairs. For a given lncRNA pair (lncRNA1, lncRNA2), the expression levels of lncRNA1 and lncRNA2 can be described as: , , respectively. In our study, the expression level of the given lncRNA pair (lncRNA1, lncRNA2) was defined as follows:
If the frequency of 1 or 0 in the expression level of the given lncRNA pair (lncRNA1, lncRNA2) was less than 85%, the lncRNA pair was remained. Then, the prognosis ability of these remained lncRNA pairs was further evaluated by the univariate Cox regression analysis, and the lncRNA pairs with FDR <0.2 were screened as the prognostic lncRNA pairs.
To improve the stability and generalization of PLPI, we employed Concordance index (C-index) to further select the optimal prognostic lncRNA pairs. The C-index, a commonly used evaluation metric, plays a crucial role in identifying the most relevant prognostic factors by helping assess the predictive ability of factors, which aids in improving the accuracy and reliability of prognostic models, and supporting clinical decision-making and patient management (25,26). The larger the C-index value for the prognostic factor, the better its predictive accuracy and discriminatory ability. In our study, we chose the prognostic lncRNA pair with the highest C-index value as the candidate seed lncRNA (CSlncRNA) pair, and then added additional prognostic lncRNA pairs in descending C-index order to the CSlncRNA pair one by one. Those prognostic lncRNA pairs that increased the C-index value were ultimately selected. Finally, to avoid overfitting, we further utilized least absolute shrinkage and selection operator (LASSO) regression analysis to select the prognostic lncRNA pairs, which were used for constructing the prognostic model PLPI.
Construction and validation of the prognostic model PLPI
In this study, the PLPI was constructed based on the prognostic pyroptosis-related lncRNA pairs to predict the prognosis of HCC patients. The risk score (RS) was calculated for each patient by the following formula:
where the is the gene expression level of , and the is the corresponding estimated regression coefficient for each lncRNA pairs in the LASSO regression analysis.
Firstly, we randomly divided the patients in the TCGA-LIHC database into a training set and a testing set at a ratio of 1:1 using the caret package. The training set was used to train and build the prognostic model PLPI, while the testing set and the external set (NODE database) were used to validate the predictive performance of the PLPI. All the patients in the training set, the testing set, and the external set were stratified into the high- or low-risk groups based on the median RS calculated from the training set. We employed receiver operating characteristic (ROC) analysis, utilizing the timeROC package, to assess the predictive performance of the PLPI and calculated the area under the curve (AUC) in the training set, the testing set, and the external set. Kaplan-Meier (KM) analysis was further used to evaluate the OS between the high- and low-risk groups. Additionally, we performed univariate and multivariate Cox regression analyses to investigate whether the RS could be regarded as an independent prognostic predictor after considering other clinical features, such as gender, age, tumor-node-metastasis (TNM) stage, and grade.
Functional gene set enrichment analysis (GSEA)
GSEA is a special type of computational method used to identify whether a predefined set of genes shows statistically significant differences in expression under different biological states, as determined by analyzing gene expression data. Therefore, we used GSEA to discover the potential pathways and explore the biological process between the high- and low-risk groups in the TCGA-LIHC database. Specifically, based on the Hallmarks gene set “h.all.v7.0.symbols.gmt” and the gene set “c2.cp.kegg.v7.5.1.symbols.gmt” from the MSigDB database, GSEA was carried out through GSEA software (27,28) and clusterProfiler package (version 4.8.2) (29). The gene sets could be downloaded on the website (https://www.gsea-msigdb.org.). Additionally, P<0.05, |normalized enrichment score (NES)| >1, and FDR <0.25 were set as the significance threshold for our statistical analyses.
Analyses of tumor-infiltrating immune cells
To validate the effectiveness of the PLPI model we proposed, we conducted an analysis of tumor-infiltrating immune cells using three tools: Cell-Type Identification by Estimating Relative Subsets of RNA Transcripts (CIBERSORT) (https://cibersort.stanford.edu/), the single-sample GSEA (ssGSEA), and the Estimation of Stromal and Immune Cells in Malignant Tumor Tissues using Expression Data (ESTIMATE). CIBERSORT can determine the proportions of different cell types within a mixed cell population by analyzing gene expression data of the cancer patients (30). We utilized it to analyze the distribution of 22 immune cell types in the high- and low-risk patient groups from the TCGA-LIHC database. The detailed list of 22 cell types is shown in Table S1. ssGSEA, a bioinformatics method, is used to calculate enrichment scores for gene sets within individual samples and has been employed to assess the infiltration of 28 immune cells in the high- and low-risk patient groups from the TCGA-LIHC database (31). The 28 immune gene sets are shown in table available at https://cdn.amegroups.cn/static/public/tcr-23-1804-2.pdf. ESTIMATE is a tool that estimate the compositions of stromal and immune cells within the tumor microenvironment by analyzing gene expression data from cancer patients (32), which was applied to estimate tumor purity, stromal, immune, and estimate scores.
Drug and mutation analyses
Following the acquisition of tumor mutation data of HCC patients, we utilized the TCGAbiolinks package and maftools package to explore somatic mutations in the high- and low-risk groups from the TCGA-LIHC database. In addition, the pRRophetic package was used to establish the relation between drug response and gene expression data to identify potential therapeutic drugs. Therefore, we used the pRRophetic package to calculate the half-maximal inhibitory concentration (IC50) values of each patient in the high- and low-risk groups of 29 drugs, which had previously been shown to have promising efficacy in relevant studies (33-35). In this study, the gene expression and drug sensitivity data from cell lines in the Cancer Genome Project (https://www.sanger.ac.uk/group/cancer-genome-project/) and gene expression data from the TCGA-LIHC database were used in the drug analysis.
Statistical analysis
Statistical analyses were performed using R 4.0.3 and GSEA software. Univariate and multivariate Cox proportional hazard regression analyses were utilized to evaluate the independent prognostic predictive power of the proposed PLPI. Besides, the Wilcoxon rank-sum test was used to analyze immune infiltration cell scores and the drug sensitivity between high- and low-risk groups. Statistical significance was defined as P<0.05, unless otherwise noted.
Results
Identification of DEPR-lncRNAs
Initially, we obtained 10,877 lncRNAs and 54,561 mRNAs shared in TCGA-LIHC and NODE databases according to the same gene symbol. We performed the Wilcoxon rank sum test and identified 764 DElncRNAs and 5,642 DEmRNAs [|log2(fold change)| >1, FDR <0.05], which are shown in tables available at https://cdn.amegroups.cn/static/public/tcr-23-1804-3.pdf and https://cdn.amegroups.cn/static/public/tcr-23-1804-4.pdf. The volcano plot of the DElncRNAs is displayed in Figure 2A. Next, we intersected the 5,642 DEmRNAs and 155 pyroptosis-related mRNAs, resulting in 110 DEPR-mRNAs. Finally, we identified 407 DEPR-lncRNAs from the co-expression network between 110 DEPR-mRNAs and 764 DElncRNAs (|R2|>0.04, P<0.001). Additionally, a data flow diagram of the data collection for DEPR-lncRNAs is shown in Figure S1.
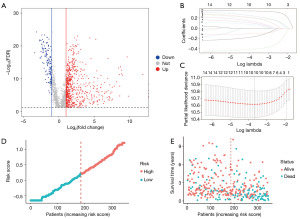
Construction of the prognostic pyroptosis-related lncRNA pairs
Based on the 407 DEPR-lncRNAs, we performed univariate Cox regression analysis and identified 35 DEPR-lncRNAs that were significantly associated with the OS of HCC patients (P<0.01). Following the methodology detailed in the Methods section, we obtained a set of 595 DEPR-lncRNA pairs that were sorted in descending order based on the C-index, which is shown in table available at https://cdn.amegroups.cn/static/public/tcr-23-1804-5.pdf. We identified the DEPR-lncRNA pair with the highest C-index as the candidate seed DEPR-lncRNA pair, and subsequently added other DEPR-lncRNA pairs to the candidate seed DEPR-lncRNA pair. As a result, a total of 15 DEPR-lncRNA pairs that exhibited improved the C-index were remained. To further refine the DEPR-lncRNA pairs and reduce the risk of overfitting, LASSO Cox regression analysis was employed. We displayed the LASSO coefficients of the 15 lncRNA pairs in Figure 2B and conducted 10-fold cross-validation to identify the optimal penalty parameter lambda. Our findings showed that the result achieved satisfactory performance with the fewest possible parameters when logλ =−3.60010 (Figure 2C). Finally, we identified 10 DEPR-lncRNA pairs by the above multiple algorithms for constructing the PLPI, which is shown in Table S2.
Construction and validation of PLPI based on the DEPR-lncRNA pairs
In this study, we excluded patients with an OS time of less than 30 days or incomplete clinical information, leaving a total of 346 HCC patients from the TCGA-LIHC database. These patients were randomly divided into the training and testing sets in a ratio of 1:1. In the training set, we constructed the prognostic model PLPI using the 10 DEPR-lncRNA pairs selected by the LASSO regression analysis. By the multivariate Cox regression analysis, the calculation formula of the RS was as follows:
Using the median RS in the training set (RS =0.2113288), we segregated the 346 HCC patients into high- and low-risk groups. The distribution of the RS values and survival status of the 346 patients were showed in Figure 2D,2E. Furthermore, we performed KM analysis and observed that patients in the low-risk group had significantly longer OS time than those in the high-risk group, both in the training set and the testing set (Figure 3A,3B; P<0.001). In the training set, the time-dependent ROC curves revealed that the AUCs for 1-, 3-, and 5-year OS predictions were 0.76, 0.73, and 0.73, respectively (Figure 3C). Similarly, in the testing set, the AUCs used for 1-, 3-, and 5-year OS predictions were 0.8, 0.75, and 0.81, respectively (Figure 3D).
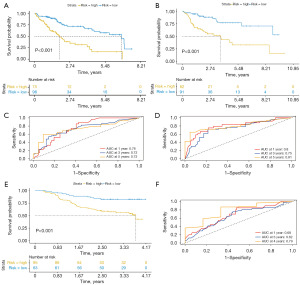
To validate the effectiveness of the PLPI, we analyzed 158 HCC patients whose OS time were more than 30 days from the external set (NODE database). These patients were divided into high- and low-risk groups based on the median RS cutoff value obtained from the training set. KM analysis was conducted on the external set, which showed similar results as those in the training set (Figure 3E; P<0.001). Since the patients’ survival time from the external set was less than 5 years, the AUCs for 1-, 3-, and 4-year OS of the time-dependent ROC curves were analyzed, which were 0.68, 0.62, and 0.79, respectively (Figure 3F).
The above results suggested that the prognostic model PLPI has good predictive accuracy for OS of HCC patients. To evaluate the independent prognostic ability of the PLPI, we also performed both univariate and multivariate Cox regression analyses on the RS and other clinical information in the TCGA-LIHC database. As shown in Table 1, in the training set, the multivariate analysis showed that RS was significantly independent with other clinical information [hazard ratio (HR): 4.8455; 95% confidence interval (CI): 2.3239−10.1036; P<0.001]. Similar findings were obtained in the testing set (HR: 4.6485; 95% CI: 2.2084−9.7847; P<0.001). Moreover, these results were further confirmed in the whole database (HR: 4.1956; 95% CI: 2.5865−6.806; P<0.001). Overall, these findings indicated that the proposed model PLPI has an independent prognostic predictive ability for OS in patients with HCC.
Table 1
Variables | Univariate analysis | Multivariate analysis | |||||
---|---|---|---|---|---|---|---|
HR | 95% CI | P value | HR | 95% CI | P value | ||
Training set (n=112) | |||||||
RS† | 4.2617 | 2.2129−8.2077 | <0.001 | 4.8455 | 2.3239−10.1036 | <0.001 | |
Age (<70 vs. ≥70 years) | 0.9993 | 0.9706−1.0288 | 0.96 | 0.9917 | 0.9601−1.0244 | 0.61 | |
Gender (male vs. female) | 0.9162 | 1.8976−1.4859 | 0.81 | 1.5029 | 0.6548−3.4500 | 0.33 | |
T stage (T1 + T2 vs. T3 + T4) | 3.9694 | 1.9898−7.9183 | <0.001 | 4.0839 | 1.7948−9.2928 | <0.001 | |
M stage (M0 vs. M1) | 3.7882 | 0.8964−16.0100 | 0.07 | 2.1067 | 0.3925−11.3070 | 0.38 | |
N stage (N0 vs. N1) | 1.4585 | 0.1975−10.7686 | 0.71 | 1.8048 | 0.2095−15.5495 | 0.59 | |
Grade (G1 + G2 vs. G3 + G4) | 0.7885 | 0.3945−1.5761 | 0.50 | 1.1744 | 0.5233−2.6356 | 0.69 | |
Testing set (n=111) | |||||||
RS† | 4.8504 | 2.5743−9.1390 | <0.001 | 4.6485 | 2.2084−9.7847 | <0.001 | |
Age (<70 vs. ≥70 years) | 0.9968 | 0.9740−1.0201 | 0.78 | 1.0067 | 0.9776−1.0366 | 0.65 | |
Gender (male vs. female) | 0.6816 | 0.3502−1.3268 | 0.25 | 1.2176 | 0.5469−2.7106 | 0.62 | |
T stage (T1 + T2 vs. T3 + T4) | 4.0337 | 1.9926−8.1659 | <0.001 | 2.5369 | 1.1138−5.7784 | 0.02 | |
M stage (M0 vs. M1) | 11.5824 | 1.4612−91.4354 | 0.02 | 22.3088 | 2.1846−22.7814 | 0.08 | |
N stage (N0 vs. N1) | 6.3612 | 0.8428−48.0121 | 0.07 | 1.1614 | 0.1071−12.5593 | 0.90 | |
Grade (G1 + G2 vs. G3 + G4) | 1.4851 | 0.7644−2.8856 | 0.24 | 0.6583 | 0.3062−1.4151 | 0.28 | |
The whole database (n=223) | |||||||
RS† | 4.5425 | 2.8975−7.1215 | <0.001 | 4.1956 | 2.5865−6.806 | <0.001 | |
Age (<70 vs. ≥70 years) | 0.9982 | 0.9803−1.0164 | 0.84 | 1.0001 | 0.9808−1.020 | >0.99 | |
Gender (male vs. female) | 0.7677 | 0.4723−1.2481 | 0.28 | 1.2836 | 0.7479−2.203 | 0.36 | |
T stage (T1 + T2 vs. T3 + T4) | 3.7191 | 2.3173−5.9688 | <0.001 | 2.7875 | 1.6746−4.640 | <0.001 | |
M stage (M0 vs. M1) | 4.3522 | 1.3607−13.9208 | 0.01 | 2.5552 | 0.7342−8.892 | 0.14 | |
N stage (N0 vs. N1) | 2.2997 | 0.5608−9.4306 | 0.24 | 1.1807 | 0.2698−5.167 | 0.82 | |
Grade (G1 + G2 vs. G3 + G4) | 1.0496 | 0.6547−1.6828 | 0.85 | 0.8515 | 0.5104−1.420 | 0.53 |
†, derived from the RS model proposed in our study. HR, hazard ratio; CI, confidence interval; RS, risk score.
Potential pathways associated with the PLPI
GSEA was performed to explore the differences of the underlying biological functions between high- and low-risk groups in the TCGA-LIHC database. The results showed that several metabolism-related pathways, (xenobiotic metabolism, fatty acid metabolism, bile acid metabolism, drug metabolism-cytochrome p450, propanoate metabolism, and tryptophan metabolism), some HCC-related pathways [peroxisome, peroxisome proliferators-activated receptor (PPAR) signaling pathway and cytokine-cytokine receptor interaction], were significantly enriched in the low-risk group (Figure 4A,4B). On the other hand, several disease-related pathways (Huntington disease, Alzheimer disease, non-alcoholic fatty liver disease, Parkinson disease, and prion disease), some metastatic pathways (DNA repair, Myc targets V1, Myc targets V2) were enriched in the high-risk group (Figure 4C,4D).
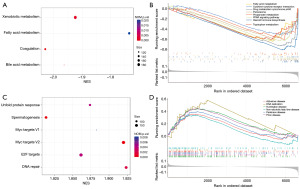
Association between the PLPI and the tumor-infiltrating immune cells
We also investigate the relationship between the PLPI and the tumor-infiltrating immune cells using CIBERSORT analysis. Our results showed that the patients in the high-risk group had higher fractions of macrophages_M0, and T cell regulatory, while lower fractions of monocytes, macrophages_M1, and T cell gamma delta compared to the low-risk group (Figure 5A). Furthermore, we utilized the ssGSEA algorithm to estimate the infiltration of 28 types of immune cells in HCC patients and found significant differences in 18 types of immune cells (Figure 5B). Additionally, ESTIMATE analysis revealed that the stromal score, immune score, and estimate score were higher in the low-risk group than those in the high-risk group (Figure 5C; P<0.001, P<0.01, P<0.001). However, the high-risk group showed higher tumor purity compared to the low-risk group (Figure 5D), where tumor purity represents the proportion of tumor cells compared to normal cells.
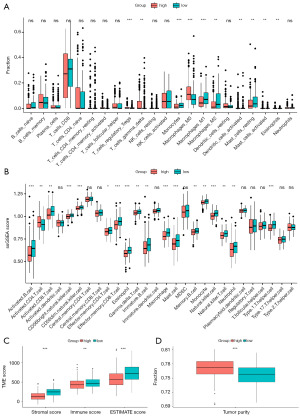
Potential drugs for the patients and somatic mutation analyses
To assess the potential effectiveness of immune-based therapy for HCC patients, it is important to analyze the somatic mutational landscape of the tumor. We compared the somatic mutations of 335 patients with complete mutation data from the TCGA-LIHC database who were divided into high- and low-risk groups based on the median RS of the training set. Then, it was calculated that 71.82% of low-risk group patients (130/181) and 85.06% of high-risk group patients (131/154) altered somatic mutation. Additionally, missense mutations were the highest frequency of all the variants, single nucleotide polymorphism (SNP) was the most common variant type, and C>T was the most frequent single nucleotide variation (SNV) in both low- and high-risk groups. Besides, detailed information on the frequency of variant classification, variant type, SNV class, variant classification summary and the number of variants per sample for both the low- and high-risk groups were displayed (Figure 6A,6B). Next, the top ten genes exhibiting the highest mutation frequency were analyzed, with TP53, TTN, and CTNNB1 being found to be the major driver genes in HCC, consistent with previous studies (36-38). Interestingly, we noted significantly lower mutation frequencies of TP53, TTN, and CTNNB1 in the low-risk group compared to the high-risk group. Our findings could have significant implications in identifying potential therapeutic targets for patients in the high-risk groups and devising personalized treatment strategies for HCC.
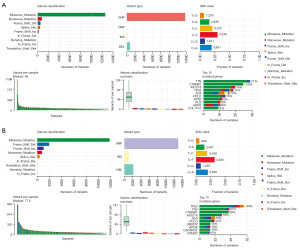
Meanwhile, several metabolism-related pathways were significantly enriched in the low-risk group, suggesting the potential for personalized drug susceptibility analysis. The IC50, which evaluates drug sensitivity, is vital in determining appropriate drug options for patients. The results displayed that erlotinib (P<0.001) and lapatinib (P=0.04) showed a lower IC50 in the low-risk group compared to the high-risk group (Figure 7A,7B), indicating that they may be effective options for this patient population. In addition, AKT inhibitor VIII (P=0.02), cytarabine (P=0.009), docetaxel (P<0.001), etoposide (P<0.001), gemcitabine (P<0.001), imatinib (P=0.004), methotrexate (P<0.001), paclitaxel (P<0.001), sorafenib (P=0.04), vorinostat (P<0.001), tipifarnib (P<0.001), and vinblastine (P<0.001) showed lower IC50 in the patients in the high-risk group compared to the low-risk group (Figure 7C-7N).
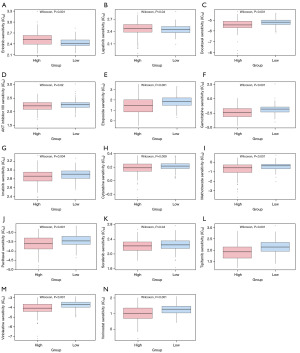
Discussion
HCC is a major cause of mortality in Asia and America, mainly due to the poor performance of conventional treatment and the heterogeneity (39,40). LncRNA has been extensively studied as oncogenes or tumor suppressor genes that play a crucial role in the physiological and pathological processes of various cancers, including gastric cancer, HCC, and glioma (41). Recent studies have shown that pyroptosis, a type of programmed cell death, has an important role in anti-cancer defense, activation of the immune system, and enhancing the efficacy of cancer treatment combined with chemotherapy (42,43). Additionally, the REOs of gene pairs have been considered as a reliable method that reduces statistical errors from data normalization and batch effect among different samples, and is widely applicable to clinical treatment (44,45).
In this study, we developed a novel REOs based-prognostic model PLPI using 10 pyroptosis-related lncRNA pairs for predicting the prognosis of HCC patients. The effectiveness of the proposed PLPI was successfully validated on the TCGA-LIHC and NODE databases, which demonstrated that the prognostic model PLPI achieved a high prediction accuracy by separating the patients into high- and low-risk groups. Specifically, the 5-year AUC for the TCGA-LIHC database was 0.81, while the 4-year AUC for the NODE database was 0.79. Compared to previous signatures which were constructed by pyroptosis-related lncRNAs in HCC, the AUCs of our prognostic model are greater than others and show better predictive ability (46-48). These results indicated that our PLPI has a favorable performance for survival prediction.
The prognostic model constructed in this study was based on 10 prognostic lncRNA pairs, consisting of 12 lncRNAs (CTC-297N7.9, CTD-2510F5.4, RP11-432J22.2, LINC00942, RP11-92C4.6, RP11-150O12.3, RP11-187E13.1, RP11-443B20.1, RP11-519G16.5, RP11-536K7.3, RP11-540A21.2, and RP3-439F8.1). Notably, several lncRNAs included in our model have been previously shown to be association with various cancers, indicating their potential as powerful prognostic biomarkers. For example, Zhu et al. uncovered that the expression of CTC-297N7.9 is associated with tumor stage, tumor differentiation, and serum alpha-fetal protein, which suggested its clinical significance as a prognostic biomarker (49). Similarly, CTD-2510F5.4 had been identified as a crucial lncRNA possibly involved in the molecular pathogenesis of gastric cancer by regulating cell proliferation, cell cycle, and apoptosis (50). Moreover, Wang et al. have discovered the importance of LINC00942 in the initiation and progression of lung adenocarcinoma, as it promotes apoptosis of A549 and H1299 cells (51). Previous studies have also highlighted that RP11-150O12.3 might exert an indispensable role in the development and prognosis of different types of tumors, including HCC, colorectal adenocarcinoma, and gastric cancer (52-54). Furthermore, Qi et al. demonstrated that the lncRNA RP3-439F8.1 would motivate the proliferation and development of glioblastoma multiform tumor cells through vitro experiment (55). These findings, combined with our study, further confirmed the significance of these lncRNAs identified by multiple algorithms. Moreover, the identification of 10 prognostic lncRNA pairs holds promising implications for personalized effective treatment strategies for patients with HCC.
To better investigate the underlying biological functions of the PLPI, GSEA was performed on the high- and low-risk groups. The results of GSEA revealed that several pathways were significantly enriched in the low-risk group, which have been proved to play a key role in HCC. For instance, Che et al. reported that fatty acid metabolism is involved in the molecular pathogenesis of HCC and is greatly harmful to the growth of HCC cells, showing the potential to be a new treatment method (56). Meanwhile, Sato et al. had explored the association between HCC prognosis and coagulation, which showed future prospect for HCC treatment (57). Additionally, cytokine-cytokine receptor interaction is essential for understanding the immunological and inflammatory responses in disease and may be a key pathway during HCC development (58,59). Moreover, the function of peroxisome has been shown to be highly correlated with metabolic stress, mammalian target of rapamycin (mTOR) inhibition, and lethality in liver cancer cells, as well as serving as a key regulator of immune function and inflammation during development and infection (60,61). Kimura et al. found that the PPAR signaling is essential for the mechanism of carcinogenesis in fatty liver, which also is involved in several other mechanisms such as apoptosis and anti-inflammatory responses (62). In contrast, the GSEA results indicated that three essential signal pathways were notably enriched in the high-risk group, including DNA repair, Myc targets V1, Myc targets V2, which were primarily associated with initiation, invasion, and metastasis of tumor cells (63). Patel et al. found that the enrichment of Unfold protein response is associated with poorer OS in HCC patients (64). Simultaneously, the high-risk group was also enriched in some disease pathways such as Parkinson disease, Alzheimer disease, and non-alcoholic fatty liver disease, while the low-risk group was also enriched in metabolism-related pathways and immune-related pathways, such as drug metabolism cytochrome p450 and PPAR signaling pathway. Therefore, these findings suggest that the prognostic model PLPI is highly correlated with the development of HCC, and the pathways highlighted above may help uncover the potential mechanisms of HCC carcinogenesis and development.
Pyroptosis is a type of programmed cell death that has been implicated in inflammation and tumor immunity (65). In this study, we utilized CIBERSORT and ssGSEA analyses to evaluate the composition of tumor-infiltrating immune cells in HCC. Our finding showed that the high-risk group had higher proportions of M0 macrophages and T regulatory (Treg) cells, whereas the low-risk group had more infiltrated of gamma delta T cell, monocytes, and B cells. Notably, Farha et al. discovered that high enrichment of M0 macrophages may contribute to a poor prognostic outcome in HCC (66). Treg cells may not only promote the invasion and immune escape of HCC but also suppress the immune response (67,68). However, gamma delta T-cells are not only essential for a favorable prognosis of HCC by assessing the patients’ survival time and tumor size, but also play a crucial role in tumor immunosurveillance, cancer immunotherapy, and anti-tumor cytokine production (69,70). Activated monocytes in the peritumoral stroma of HCC turn the inflammatory response into tissue remodeling and proangiogenic pathway and accelerate expansion of memory T helper 17 cells, which might help the development of anticancer therapy (71). Garnelo et al. found that the interaction between tumor-infiltrating B cells and T cells is beneficial to enhance the local immune activation and lead to the better survival outcomes (72). Moreover, the results of ESTIMATE analysis displayed that the stromal score, immune score, and estimate score were higher in the low-risk group than those in the high-risk group. In the meanwhile, it also demonstrated that the PLPI could partially reflect the immune status of patients with HCC and is useful for assessing the abundance of immune cell infiltration in different risk groups. In conclusion, the results of immune analyses demonstrated that immune therapy may be beneficial for patients in the low-risk group, which implied the crucial role of PLPI in the clinical treatment of HCC.
The development of cancer is known to be influenced by the accumulation of genetic mutations (73). In our study, we performed mutation analysis and found that the levels of TP53, CTHNB1, and TTN were significantly higher in the high-risk group compared to the low-risk group, which indicated that the low-risk group had a better prognosis for HCC patients. Hussain et al. have previously demonstrated that TP53 mutations are relevant to viruses as well as chemicals during the HCC pathogenesis period (74). Tornesello et al. found that CTNNB1 mutations activate the development of HCC and cause the aberrant activation of Wnt signaling and nuclear accumulation of β-catenin, which play an important role in HCC (75). Kong et al. found that TTN mutations, with the second highest mutation frequency in HCC, causes familial hypertrophic cardiomyopathy and the detection of TTN mutation, and could help patients make better treatment decisions (76). These findings indicated that the prognostic model PLPI constructed in our study may not only cast a new light on the HCC development, but also contribute to individualized treatment for HCC patients.
Drug sensitivity analysis suggested that HCC patients in the low-risk group were more sensitivity to erlotinib and lapatinib, which showed consistency to previous studies (77,78). On the other hand, HCC patients in the high-risk group showed more sensitivity to drugs, such as AKT inhibitor VIII, Cytarabine, and Docetaxel. These results suggest that the prognostic model PLPI not only aids in selection of chemotherapy regimens, but also serves as a clinical treatment guide for HCC.
In conclusion, PLPI is a novel prognostic model for predicting the OS of the patients with HCC. It not only incorporates the significance of pyroptosis but also effectively mitigates the impact of the experimental batch effects through the application of REO method. The robustness and reliability of PLPI has been evaluated and validated using both the testing set (TCGA-LIHC dataset) and an independent external set (NODE-OEP000321 dataset), which significantly enhancing its reliability and potential for clinical application. However, this study has several limitations. For example, the clinical information available from the TCGA-LIHC and NODE databases is inadequate and limited, and additional studies are necessary to confirm our findings through in vitro or in vivo experiments. Additionally, to enhance our understanding of the underlying mechanism of PLPI, further investigations should focus on the specific lncRNAs involved in its construction, which can provide valuable insights into the biological processes underlying HCC prognosis and potentially identify new therapeutic targets.
Conclusions
In summary, we developed a novel prognostic model PLPI using 10 pyroptosis-related lncRNA pairs, which offers a unique perspective for improving the clinical decision-making process for patients with HCC. Moreover, this study could provide a valuable contribution towards the development of more effective treatments plans and improve outcomes for patients with HCC.
Acknowledgments
Funding: This study was supported by
Footnote
Reporting Checklist: The authors have completed the TRIPOD reporting checklist. Available at https://tcr.amegroups.com/article/view/10.21037/tcr-23-1804/rc
Peer Review File: Available at https://tcr.amegroups.com/article/view/10.21037/tcr-23-1804/prf
Conflicts of Interest: Both authors have completed the ICMJE uniform disclosure form (available at https://tcr.amegroups.com/article/view/10.21037/tcr-23-1804/coif). The authors have no conflicts of interest to declare.
Ethical Statement: The authors are accountable for all aspects of the work in ensuring that questions related to the accuracy or integrity of any part of the work are appropriately investigated and resolved. The study was conducted in accordance with the Declaration of Helsinki (as revised in 2013).
Open Access Statement: This is an Open Access article distributed in accordance with the Creative Commons Attribution-NonCommercial-NoDerivs 4.0 International License (CC BY-NC-ND 4.0), which permits the non-commercial replication and distribution of the article with the strict proviso that no changes or edits are made and the original work is properly cited (including links to both the formal publication through the relevant DOI and the license). See: https://creativecommons.org/licenses/by-nc-nd/4.0/.
References
- Venook AP, Papandreou C, Furuse J, et al. The incidence and epidemiology of hepatocellular carcinoma: a global and regional perspective. Oncologist 2010;15:5-13. [Crossref] [PubMed]
- Llovet JM, Kelley RK, Villanueva A, et al. Hepatocellular carcinoma. Nat Rev Dis Primers 2021;7:6. [Crossref] [PubMed]
- Yang C, Qin S. Apatinib targets both tumor and endothelial cells in hepatocellular carcinoma. Cancer Med 2018;7:4570-83. [Crossref] [PubMed]
- Yang L, Si H, Ma M, et al. LINC00221 silencing prevents the progression of hepatocellular carcinoma through let-7a-5p-targeted inhibition of MMP11. Cancer Cell Int 2021;21:202. [Crossref] [PubMed]
- Sun J, Mao S, Ji W. LncRNA H19 activates cell pyroptosis via the miR-22-3p/NLRP3 axis in pneumonia. Am J Transl Res 2021;13:11384-98. [PubMed]
- Chen Z, He M, Chen J, et al. Long non-coding RNA SNHG7 inhibits NLRP3-dependent pyroptosis by targeting the miR-34a/SIRT1 axis in liver cancer. Oncol Lett 2020;20:893-901. [Crossref] [PubMed]
- Liu XH, Sun M, Nie FQ, et al. Lnc RNA HOTAIR functions as a competing endogenous RNA to regulate HER2 expression by sponging miR-331-3p in gastric cancer. Mol Cancer 2014;13:92. [Crossref] [PubMed]
- Frank D, Vince JE. Pyroptosis versus necroptosis: similarities, differences, and crosstalk. Cell Death Differ 2019;26:99-114. [Crossref] [PubMed]
- Zhang Y, Chen X, Gueydan C, et al. Plasma membrane changes during programmed cell deaths. Cell Res 2018;28:9-21. [Crossref] [PubMed]
- Lou J, Zhou Y, Feng Z, et al. Caspase-Independent Regulated Necrosis Pathways as Potential Targets in Cancer Management. Front Oncol 2021;10:616952. [Crossref] [PubMed]
- Broz P, Pelegrín P, Shao F. The gasdermins, a protein family executing cell death and inflammation. Nat Rev Immunol 2020;20:143-57. [Crossref] [PubMed]
- Hou J, Hsu JM, Hung MC. Molecular mechanisms and functions of pyroptosis in inflammation and antitumor immunity. Mol Cell 2021;81:4579-90. [Crossref] [PubMed]
- Yang X, Weng X, Yang Y, et al. Pyroptosis-Related lncRNAs Predict the Prognosis and Immune Response in Patients With Breast Cancer. Front Genet 2022;12:792106. [Crossref] [PubMed]
- Song W, Ren J, Xiang R, et al. Identification of pyroptosis-related subtypes, the development of a prognosis model, and characterization of tumor microenvironment infiltration in colorectal cancer. Oncoimmunology 2021;10:1987636. [Crossref] [PubMed]
- Wang H, Shao R, Lu S, et al. Integrative Analysis of a Pyroptosis-Related Signature of Clinical and Biological Value in Multiple Myeloma. Front Oncol 2022;12:845074. [Crossref] [PubMed]
- Zhou W, Zhang W. A novel pyroptosis-related lncRNA prognostic signature associated with the immune microenvironment in lung squamous cell carcinoma. BMC Cancer 2022;22:694. [Crossref] [PubMed]
- Zheng H, Song K, Fu Y, et al. An absolute human stemness index associated with oncogenic dedifferentiation. Brief Bioinform 2021;22:2151-60. [Crossref] [PubMed]
- Ao L, Zhang Z, Guan Q, et al. A qualitative signature for early diagnosis of hepatocellular carcinoma based on relative expression orderings. Liver Int 2018;38:1812-9. [Crossref] [PubMed]
- Nygaard V, Rødland EA, Hovig E. Methods that remove batch effects while retaining group differences may lead to exaggerated confidence in downstream analyses. Biostatistics 2016;17:29-39. [Crossref] [PubMed]
- Guan Q, Chen R, Yan H, et al. Differential expression analysis for individual cancer samples based on robust within-sample relative gene expression orderings across multiple profiling platforms. Oncotarget 2016;7:68909-20. [Crossref] [PubMed]
- Deng M, Lin JB, Zhao RC, et al. Construction of a novel immune-related lncRNA signature and its potential to predict the immune status of patients with hepatocellular carcinoma. BMC Cancer 2021;21:1347. [Crossref] [PubMed]
- Hong W, Liang L, Gu Y, et al. Immune-Related lncRNA to Construct Novel Signature and Predict the Immune Landscape of Human Hepatocellular Carcinoma. Mol Ther Nucleic Acids 2020;22:937-47. [Crossref] [PubMed]
- Wu T, Li N, Luo F, et al. Screening prognostic markers for hepatocellular carcinoma based on pyroptosis-related lncRNA pairs. BMC Bioinformatics 2023;24:176. [Crossref] [PubMed]
- Zeng S, Li W, Ouyang H, et al. A Novel Prognostic Pyroptosis-Related Gene Signature Correlates to Oxidative Stress and Immune-Related Features in Gliomas. Oxid Med Cell Longev 2023;2023:4256116. [Crossref] [PubMed]
- Zhang Y, Hong YK, Zhuang DW, et al. Bladder cancer survival nomogram: Development and validation of a prediction tool, using the SEER and TCGA databases. Medicine (Baltimore) 2019;98:e17725. [Crossref] [PubMed]
- Tong D, Tian Y, Zhou T, et al. Improving prediction performance of colon cancer prognosis based on the integration of clinical and multi-omics data. BMC Med Inform Decis Mak 2020;20:22. [Crossref] [PubMed]
- Mootha VK, Lindgren CM, Eriksson KF, et al. PGC-1alpha-responsive genes involved in oxidative phosphorylation are coordinately downregulated in human diabetes. Nat Genet 2003;34:267-73. [Crossref] [PubMed]
- Subramanian A, Tamayo P, Mootha VK, et al. Gene set enrichment analysis: a knowledge-based approach for interpreting genome-wide expression profiles. Proc Natl Acad Sci U S A 2005;102:15545-50. [Crossref] [PubMed]
- Yu G, Wang LG, Han Y, et al. clusterProfiler: an R package for comparing biological themes among gene clusters. OMICS 2012;16:284-7. [Crossref] [PubMed]
- Newman AM, Liu CL, Green MR, et al. Robust enumeration of cell subsets from tissue expression profiles. Nat Methods 2015;12:453-7. [Crossref] [PubMed]
- Barbie DA, Tamayo P, Boehm JS, et al. Systematic RNA interference reveals that oncogenic KRAS-driven cancers require TBK1. Nature 2009;462:108-12. [Crossref] [PubMed]
- Yoshihara K, Shahmoradgoli M, Martínez E, et al. Inferring tumour purity and stromal and immune cell admixture from expression data. Nat Commun 2013;4:2612. [Crossref] [PubMed]
- Geeleher P, Cox N, Huang RS. pRRophetic: an R package for prediction of clinical chemotherapeutic response from tumor gene expression levels. PLoS One 2014;9:e107468. [Crossref] [PubMed]
- Zhang B, Zhao J, Liu B, et al. Development and Validation of a Novel Ferroptosis-Related Gene Signature for Prognosis and Immunotherapy in Hepatocellular Carcinoma. Front Mol Biosci 2022;9:940575. [Crossref] [PubMed]
- Wang T, Yang Y, Sun T, et al. The Pyroptosis-Related Long Noncoding RNA Signature Predicts Prognosis and Indicates Immunotherapeutic Efficiency in Hepatocellular Carcinoma. Front Cell Dev Biol 2022;10:779269. [Crossref] [PubMed]
- Villanueva A, Hoshida Y. Depicting the role of TP53 in hepatocellular carcinoma progression. J Hepatol 2011;55:724-5. [Crossref] [PubMed]
- Yin L, Zhou L, Xu R. Identification of Tumor Mutation Burden and Immune Infiltrates in Hepatocellular Carcinoma Based on Multi-Omics Analysis. Front Mol Biosci 2021;7:599142. [Crossref] [PubMed]
- Pinyol R, Sia D, Llovet JM. Immune Exclusion-Wnt/CTNNB1 Class Predicts Resistance to Immunotherapies in HCC. Clin Cancer Res 2019;25:2021-3. [Crossref] [PubMed]
- Yan TH, Qiu C, Sun J, et al. MiR-877-5p suppresses cell growth, migration and invasion by targeting cyclin dependent kinase 14 and predicts prognosis in hepatocellular carcinoma. Eur Rev Med Pharmacol Sci 2018;22:3038-46. [PubMed]
- Chen YF, Qi HY, Wu FL. Euxanthone exhibits anti-proliferative and anti-invasive activities in hepatocellular carcinoma by inducing pyroptosis: preliminary results. Eur Rev Med Pharmacol Sci 2018;22:8186-96. [PubMed]
- He A, He S, Li X, et al. ZFAS1: A novel vital oncogenic lncRNA in multiple human cancers. Cell Prolif 2019;52:e12513. [Crossref] [PubMed]
- Fang Y, Tian S, Pan Y, et al. Pyroptosis: A new frontier in cancer. Biomed Pharmacother 2020;121:109595. [Crossref] [PubMed]
- Wang L, Qin X, Liang J, et al. Induction of Pyroptosis: A Promising Strategy for Cancer Treatment. Front Oncol 2021;11:635774. [Crossref] [PubMed]
- Qian L, Ni T, Fei B, et al. An immune-related lncRNA pairs signature to identify the prognosis and predict the immune landscape of laryngeal squamous cell carcinoma. BMC Cancer 2022;22:545. [Crossref] [PubMed]
- Xie J, Xu Y, Chen H, et al. Identification of population-level differentially expressed genes in one-phenotype data. Bioinformatics 2020;36:4283-90. [Crossref] [PubMed]
- Qu G, Wang D, Xu W, et al. Comprehensive Analysis of the Correlation Between Pyroptosis-Related LncRNAs and Tumor Microenvironment, Prognosis, and Immune Infiltration in Hepatocellular Carcinoma. Front Genet 2022;13:867627. [Crossref] [PubMed]
- Zhang Z, Shang J, Hu B, et al. Prognosis and Tumour Immune Microenvironment of Patients With Hepatocellular Carcinoma by a Novel Pyroptosis-Related lncRNA Signature. Front Immunol 2022;13:836576. [Crossref] [PubMed]
- Liu ZK, Wu KF, Zhang RY, et al. Pyroptosis-Related LncRNA Signature Predicts Prognosis and Is Associated With Immune Infiltration in Hepatocellular Carcinoma. Front Oncol 2022;12:794034. [Crossref] [PubMed]
- Zhu S, Huang X, Zhang K, et al. Low expression of long noncoding RNA CTC-297N7.9 predicts poor prognosis in patients with hepatocellular carcinoma. Cancer Med 2019;8:7679-92. [Crossref] [PubMed]
- Wang Z, Qin B. Prognostic and clinicopathological significance of long noncoding RNA CTD-2510F5.4 in gastric cancer. Gastric Cancer 2019;22:692-704. [Crossref] [PubMed]
- Wang R, Wang X, Zhang J, et al. LINC00942 Promotes Tumor Proliferation and Metastasis in Lung Adenocarcinoma via FZD1 Upregulation. Technol Cancer Res Treat 2021;20:1533033820977526. [Crossref] [PubMed]
- Liu Q, Liu G, Lin Z, et al. The association of lncRNA SNPs and SNPs-environment interactions based on GWAS with HBV-related HCC risk and progression. Mol Genet Genomic Med 2021;9:e1585. [Crossref] [PubMed]
- Ren W, Zhang J, Li W, et al. A Tumor-Specific Prognostic Long Non-Coding RNA Signature in Gastric Cancer. Med Sci Monit 2016;22:3647-57. [Crossref] [PubMed]
- Zeng JH, Liang L, He RQ, et al. Comprehensive investigation of a novel differentially expressed lncRNA expression profile signature to assess the survival of patients with colorectal adenocarcinoma. Oncotarget 2017;8:16811-28. [Crossref] [PubMed]
- Qi J, Pan L, Yu Z, et al. The lncRNA RP3-439F8.1 promotes GBM cell proliferation and progression by sponging miR-139-5p to upregulate NR5A2. Pathol Res Pract 2021;223:153319. [Crossref] [PubMed]
- Che L, Paliogiannis P, Cigliano A, et al. Pathogenetic, Prognostic, and Therapeutic Role of Fatty Acid Synthase in Human Hepatocellular Carcinoma. Front Oncol 2019;9:1412. [Crossref] [PubMed]
- Sato M, Watanabe Y, Ueda S, et al. Microwave coagulation therapy for hepatocellular carcinoma. Gastroenterology 1996;110:1507-14. [Crossref] [PubMed]
- Turrin NP, Plata-Salamán CR. Cytokine-cytokine interactions and the brain. Brain Res Bull 2000;51:3-9. [Crossref] [PubMed]
- Jiang X, Hao Y. Analysis of expression profile data identifies key genes and pathways in hepatocellular carcinoma. Oncol Lett 2018;15:2625-30. [PubMed]
- Di Cara F, Andreoletti P, Trompier D, et al. Peroxisomes in Immune Response and Inflammation. Int J Mol Sci 2019;20:3877. [Crossref] [PubMed]
- Cai M, Sun X, Wang W, et al. Disruption of peroxisome function leads to metabolic stress, mTOR inhibition, and lethality in liver cancer cells. Cancer Lett 2018;421:82-93. [Crossref] [PubMed]
- Kimura O, Kondo Y, Shimosegawa T. PPAR Could Contribute to the Pathogenesis of Hepatocellular Carcinoma. PPAR Res 2012;2012:574180. [Crossref] [PubMed]
- Dai X, Jiang W, Ma L, et al. A metabolism-related gene signature for predicting the prognosis and therapeutic responses in patients with hepatocellular carcinoma. Ann Transl Med 2021;9:500. [Crossref] [PubMed]
- Patel A, Oshi M, Yan L, et al. The Unfolded Protein Response Is Associated with Cancer Proliferation and Worse Survival in Hepatocellular Carcinoma. Cancers (Basel) 2021;13:4443. [Crossref] [PubMed]
- Tan Y, Sun R, Liu L, et al. Tumor suppressor DRD2 facilitates M1 macrophages and restricts NF-κB signaling to trigger pyroptosis in breast cancer. Theranostics 2021;11:5214-31. [Crossref] [PubMed]
- Farha M, Jairath NK, Lawrence TS, et al. Characterization of the Tumor Immune Microenvironment Identifies M0 Macrophage-Enriched Cluster as a Poor Prognostic Factor in Hepatocellular Carcinoma. JCO Clin Cancer Inform 2020;4:1002-13. [Crossref] [PubMed]
- Lan YT, Fan XP, Fan YC, et al. Change in the Treg/Th17 cell imbalance in hepatocellular carcinoma patients and its clinical value. Medicine (Baltimore) 2017;96:e7704. [Crossref] [PubMed]
- Choi MR, Sosman JA, Zhang B. The Janus Face of IL-33 Signaling in Tumor Development and Immune Escape. Cancers (Basel) 2021;13:3281. [Crossref] [PubMed]
- Zakeri N, Hall A, Swadling L, et al. Characterisation and induction of tissue-resident gamma delta T-cells to target hepatocellular carcinoma. Nat Commun 2022;13:1372. [Crossref] [PubMed]
- Amajala KC, Gudivada IP, Malla RR. Gamma Delta T Cells: Role in Immunotherapy of Hepatocellular Carcinoma. Crit Rev Oncog 2023;28:41-50. [Crossref] [PubMed]
- Kuang DM, Peng C, Zhao Q, et al. Activated monocytes in peritumoral stroma of hepatocellular carcinoma promote expansion of memory T helper 17 cells. Hepatology 2010;51:154-64. [Crossref] [PubMed]
- Garnelo M, Tan A, Her Z, et al. Interaction between tumour-infiltrating B cells and T cells controls the progression of hepatocellular carcinoma. Gut 2017;66:342-51. [Crossref] [PubMed]
- Vassilev A, DePamphilis ML. Links between DNA Replication, Stem Cells and Cancer. Genes (Basel) 2017;8:45. [Crossref] [PubMed]
- Hussain SP, Schwank J, Staib F, et al. TP53 mutations and hepatocellular carcinoma: insights into the etiology and pathogenesis of liver cancer. Oncogene 2007;26:2166-76. [Crossref] [PubMed]
- Tornesello ML, Buonaguro L, Tatangelo F, et al. Mutations in TP53, CTNNB1 and PIK3CA genes in hepatocellular carcinoma associated with hepatitis B and hepatitis C virus infections. Genomics 2013;102:74-83. [Crossref] [PubMed]
- Kong F, Kong D, Yang X, et al. Integrative analysis of highly mutated genes in hepatitis B virus-related hepatic carcinoma. Cancer Med 2020;9:2462-79. [Crossref] [PubMed]
- Zhu AX, Rosmorduc O, Evans TR, et al. SEARCH: a phase III, randomized, double-blind, placebo-controlled trial of sorafenib plus erlotinib in patients with advanced hepatocellular carcinoma. J Clin Oncol 2015;33:559-66. [Crossref] [PubMed]
- Ramanathan RK, Belani CP, Singh DA, et al. A phase II study of lapatinib in patients with advanced biliary tree and hepatocellular cancer. Cancer Chemother Pharmacol 2009;64:777-83. [Crossref] [PubMed]