The emerging field of radiomics in esophageal cancer: current evidence and future potential
Introduction
Esophageal cancer continues to affect more than 450.000 people worldwide, making it the eighth most common malignancy and the sixth leading cause of cancer-related mortality (1). Despite recent improvements in staging, multimodality treatment, and peri-operative care, it remains a devastating disease with a 5-year overall survival rate of 15–25% (1-3). Best outcomes are achieved in patients with early carcinoma of the esophagus for which endoscopic mucosal (or submucosal) resection with or without local ablative techniques is now more extensively employed, associated with 5-year survival rates of 60–80% (4,5). Recently, prognosis of locally advanced esophageal cancer has been markedly improved from a 5-year overall survival rate of 23–34% with surgery alone, to 36–47% with the addition of neoadjuvant chemoradiotherapy (nCRT) or peri-operative chemotherapy (6-8). Due to the late onset of symptoms, the majority of patients present at an advanced stage with unresectable or metastatic disease, for which concurrent chemoradiotherapy (CRT) and combination chemotherapy are considered the best palliative options, respectively (9-11).
Current diagnostic work-up consists of endoscopy with biopsy for histopathologic confirmation of the diagnosis and endoscopic ultrasound (EUS) for determination of the local tumor extent (T-stage) and regional lymph node involvement (N-stage) (12,13). Integrated 18F-fluorodeoxyglucose (18F-FDG) positron emission tomography (PET)/computed tomography (CT) is also used for N-staging, and particularly important for the detection of distant metastasis (M-stage) (14-16). In addition, 18F-FDG PET/CT is increasingly applied for the detection of interval metastasis after (neoadjuvant) treatment as well as for follow-up after treatment with curative intent (17-20). Unfortunately, these modalities all have their limitations regarding three clinically relevant areas that are in need of improvement, including staging, prediction of response to treatment, and prediction of survival.
It is increasingly recognized that the amount of information currently extracted from available images may be substantially enhanced by quantitative imaging analysis (21). The emerging field of ‘radiomics’ focuses on these improvements of image analysis by extracting large amounts of quantitative image features from volumes of interest on medical images (21). Radiomics approaches can extract more information from medical images by post-processing techniques including quantification of the heterogeneity within a tumor, which is a well-recognized feature of malignancy associated with adverse tumor biology (22). Substantial spatial heterogeneity in metabolism, vasculature, oxygenation, and gene expression is often found in malignant tumors, which relates to chemoradiation-resistance and poor prognosis (23-26). As such, it has been hypothesized that image-based quantification of tumor heterogeneity—through its relation with biologic tumor characteristics may provide important information for staging, predicting response to treatment, and predicting prognosis in cancer patients (21,22,27,28). Indeed, growing evidence suggests that image analysis of tumor heterogeneity could be useful in several cancer types (29-34).
The innovative field of radiomics could provide opportunities in the management of patients with esophageal cancer for improvements in staging, predicting treatment response, and predicting survival. The aim of this review is to outline the current evidence and future potential for the application of radiomics in patients with esophageal cancer.
Texture feature analysis
Both CT and 18F-FDG PET images are of particular interest when considering quantitative analysis in esophageal tumors, since these modalities are routinely used in clinical practice. CT images are mostly used to extract morphologic information of esophageal tumors, but recent studies suggest that quantitative image features can provide additional information (22,35,36). Most 18F-FDG PET studies in esophageal cancer quantify metabolic tumor activity solely by using the maximum standardized uptake value (SUVmax) (37,38). However, extracted from a single voxel, SUVmax does not characterize the total activity nor heterogeneity of the 18F-FDG uptake for the entire tumor (39,40). Recent studies suggest that spatial image information, such as metabolic tumor volume (MTV), total lesion glycolysis (TLG), tumor shape, and texture features, provide more useful information than SUVmax (41-45).
Texture features
Among the studied CT and 18F-FDG PET quantitative image parameters, texture features are most informative on tumor heterogeneity, and thought to be most closely related to underlying physiologic processes such as vascularization, perfusion, cellular proliferation, and hypoxia (36,46). Texture is defined as a spatial arrangement of voxels allowing extraction of complex image properties (41,46). Different approaches can be used to quantify tumor texture, including model-based fractal analysis and statistic-based methods (47). Model-based fractal analysis methods describe the complexity of an object by identifying the property of self-similarity and roughness of a surface at different levels, and have so far only been described twice in esophageal tumors (48,49). Statistic-based approaches have been most widely used for texture analysis in (esophageal) oncology, and are based on the distribution and spatial relationship of voxel intensity values within an image (47).
Within the statistic-based approaches, first-order statistics represent texture on a global scale calculated from the original voxel intensity values without taking the spatial relationship between voxels into account (e.g., mean, median, percentiles, quartiles, range, interquartile range, standard deviation [SD], coefficient of variation [COV], skewness, kurtosis) (49). Second-order statistics represent texture on a local scale and measure co-occurrence of voxel pairs using grey-level co-occurrence matrices (GLCM; e.g., entropy, energy, homogeneity, contrast/inertia, correlation, dissimilarity) (28). Higher-order statistics capture properties of three or more voxels occurring at specific locations relative to each other, and represent regional texture extracted from grey-level run length matrices (GLRLM; e.g., high/low grey-level run emphasis, run percentage), grey-level size zone matrices (GLSZM; e.g., high/low intensity zone emphasis, zone percentage), or local texture extracted from neighborhood gray-tone difference matrices (NGTDM; e.g., coarseness, busyness, texture strength, complexity) (28,49,50).
Reproducibility
An overview of studies reporting on the reproducibility and precision of image texture features in esophageal cancer is provided in Table 1. Two studies (in 18F-FDG PET) have demonstrated that only a limited number of texture features are reproducible with respect to physiological variability as assessed on double baseline scans (46,50). Tixier et al. acquired double baseline 18F-FDG PET scans within 2–7 days of each other on the same scanner in 16 esophageal cancer patients and reported that the most reproducible features were local entropy, local homogeneity, regional intensity variability, and regional size-zone variability (46). Similarly, in a study from the US including 7 patients who underwent double baseline 18F-FDG PET scanning within 11–42 days of each other on scanners from different institutions, van Rossum et al. found that the reproducibility was good for (local) second-order and regional higher-order features, but poor for local higher-order features (50). Although the two studies have small samples, these results suggest that only a certain amount of texture features may be used in further research, and other features with poor reproducibility should be abandoned as results will likely not be generalizable. Similar findings were observed by Galavis et al. that found good reproducibility of some texture features but poor reproducibility for others when acquisition modes and reconstruction parameters were varied in 18F-FDG PET scans of 20 solid tumors (54). To this regard, additional investigation of reproducibility should be encouraged to move this field forward.
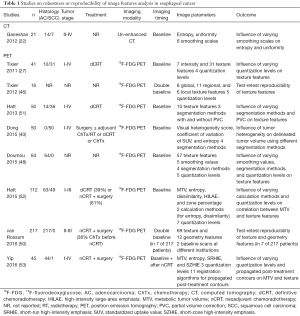
Full table
Influence of smoothing
Besides reproducibility, it is also important that similar measurements from the scan data are obtained when changing parameters such as smoothing, quantization, and segmentation. The ability of texture features to stay similar across variation of these parameters is often referred to as the ‘precision’ of texture features (48). Particularly in CT images, different scales of smoothing (i.e., image filtration) using Laplacian of Gaussian spatial band-pass filters are of importance to be able to reduce image noise and highlight different anatomical spatial scales from fine to medium and coarse texture within the tumor (22,35). Commonly used filter values for smoothing are 1.0 (highlighting fine textures, which may enhance tissue parenchymal features), 1.5–2.0 (highlighting medium textures), and 2.5 (highlighting coarse textures, which may enhance vascular features) (35). Ganeshan et al. showed that CT-based texture features (entropy and uniformity) were influenced by the level of smoothing and significantly associated with tumor stage and survival only after smoothing (22).
Influence of quantization
In both CT and 18F-FDG PET image post-processing, quantization (i.e., resampling) refers to the important process of resampling the Hounsfield (HU) or SUV levels in the image to a certain number of bins. Choosing the number of bins is a trade-off between gaining texture information accuracy with reduced noise effects (using less bins) and gaining amount of texture information (using more bins). Hence, quantization may influence texture features measurements (48,52). In a recent study with 35 lung cancer patients, Leijennar et al. indeed found that the manner of SUV quantization had a crucial effect on the resulting texture features and their interpretation, emphasizing the importance of standardized methodology in texture analysis (55). The most common quantization method includes the use of a fixed number of discrete bins (e.g., 8, 16, 32, 64, 128 bins) to divide the image SUV range into equally spaced intervals resulting in discretized images with varying bin sizes depending on the SUV range (55). However, this method appeared less appropriate for inter- and intra-patient comparison of texture features in a clinical setting than an alternative method that resamples the SUVs with a fixed bin size in units of SUV (e.g., 0.1, 0.5), maintaining a constant intensity resolution across all images (55).
Tixier et al. showed in 12 esophageal tumors that texture features describing local tumor heterogeneity were insensitive to 5 different quantization values using a fixed number of bins (i.e., 8, 16, 32, 64, or 128 bins), while several regional features were sensitive to the chosen quantization value (46). The same authors described a multi-center series of 555 patients with different types of cancer (including 112 esophageal cancer patients) in which they found that significant texture details are lost when using a quantization of less than 32 bins (52). Also, a higher potential for providing complementary information of texture features (i.e., a lower correlation) with respect to MTV was found using 64 rather than 32 bins (52). Yip et al. reported in a series of 45 patients that the value of texture features for predicting response to nCRT was highest when a quantization level of 128 was chosen (53). Doumou et al. found that 51 of 57 studied texture features showed poor agreement across varying quantization levels with a fixed number of bins (i.e., 8, 16, 32, 64, 128 bins), which stresses the need for further evaluation and standardization of quantization in future studies (48). So far, no studies in esophageal cancer patients reported on the influence of varying quantization using a fixed bin size rather than a fixed number of bins.
Influence of segmentation
Accurate segmentation (i.e., contouring) of the tumor volume is crucial for computing texture features (40,48,51). Many segmentation methods have been proposed including manual delineation, fixed or adaptive thresholding, and multiple (semi-)automatic algorithms, but no consensus seems to emerge (56,57). Most popular segmentation methods in esophageal cancer literature include manual contouring(35,36,40,53,58), thresholding methods capturing aligned voxels with SUV values of ≥2.5 (39,40,58-60) or with values ≥30–60% of the maximum intensity or grey-level (22,40,48,49,51), semi-automatic gradient-based contouring (50), and an automatic fuzzy locally adaptive Bayesian (FLAB) method (41,46,51,52).
Tumor heterogeneity is one of the most important factors influencing the results of different segmentation methods (40). Dong et al. recently demonstrated in 50 patients with esophageal squamous cell carcinoma that in tumors with a large size and high 18F-FDG uptake heterogeneity, large differences in delineated tumor volume across various manual and thresholding segmentation methods existed (40). More specifically, these authors suggest that in large or highly heterogeneous tumors one must be cautious to use frequently applied and relatively simple threshold-based segmentation methods (40). Similarly, Hatt et al. demonstrated that thresholding methods and automatic FLAB contouring led to substantially different functional volumes, significantly affecting some texture features (e.g., dissimilarity, size-zone variability), while not affecting others (e.g., entropy, homogeneity, zone percentage) (51). Doumou et al. found that varying the relative threshold (45%, 50%, 55%, or 60% of SUVmax) resulted in moderate agreement in second-order (regional) features, but in poor agreement in higher-order (regional and local) features (48). Besides the high sensitivity to tumor heterogeneity, thresholding techniques are also sensitive to motion artifacts, noise and contrast variations, leading to disappointing results for small and non-spherical tumors (56,57). Manual delineation is easy to apply, but time-consuming, susceptible to window-level settings, suffering from intra- and inter-observer variability, and results strongly depend on experience of the reader (56). In general, (semi-)automatic segmentation methods are able to provide superior accuracy, reproducibility, and robustness for tumor volume contouring compared with manual and thresholding methods, and should therefore be preferred (61,62).
Influence of tumor volume
Several texture features have appeared highly correlated with esophageal tumor volume suggesting a certain level of dependency (50,52). When high correlations between parameters exist, an added contribution over each other is unlikely. As such, in the previously mentioned French series of 555 patients with different types of cancer (including 112 esophageal cancer patients), Hatt et al. found that the complementary information of tumor volume and heterogeneity increased substantially with larger tumors (52). In fact, added value of texture features over tumor volume alone for outcome prediction was found in tumors ≥10 cm3 only (52). However, instead of excluding tumors smaller than 10cm3 in future texture studies, they recommended that the correlation of texture features and tumor volume should always be reported to show whether texture and volume provide independent or redundant information (52).
Staging
Accurate tumor staging is crucial for determining prognosis and treatment decision-making in individual patients. EUS is the current modality of choice for primary tumor staging, with reported accuracies for distinguishing T-stages of 53–94% (median, 83%) and better performance in advanced compared to early disease (63,64). Disadvantages of EUS include the invasiveness of the technique, a failure rate of 14–25% due to stenotic tumors preventing passage of the endoscope, and the strong dependence of diagnostic performance on the experience of the endoscopist (65-67). CT is inferior to EUS in the evaluation of T-stage, but CT is useful for predicting surgical resectability by excluding tumors that show ingrowth into surrounding structures (66,68). Regional lymph node involvement is generally evaluated using EUS (sensitivity 80%, specificity 70%), CT (sensitivity 50%, specificity 83%), and 18F-FDG PET (sensitivity 51%, specificity 84%) (69,70). For the detection of distant metastasis, 18F-FDG PET provides additional diagnostic information over CT in 5–28% of patients at initial presentation (14). Clearly, current clinical staging is suboptimal and in need of improvement (71).
Radiomics
Three studies (1 using CT and 2 using 18F-FDG PET) reported on the potential value of texture features analysis for staging in esophageal cancer (Table 2) (22,59,60). Ganeshan et al. related two tumor heterogeneity features on unenhanced CT (entropy and uniformity) on 6 different smoothing scales (fine to coarse details) to the clinical American Joint Committee on Cancer (AJCC) stage based on 18F-FDG PET, CT, and EUS (22). They found that tumor heterogeneity was significantly greater in patients with clinical stage III-IV compared to stage II (22). However, the potential additional value of texture features beyond conventional staging could not be studied as conventional staging was considered the reference standard an no comparison with pathologic tumor stage was performed.
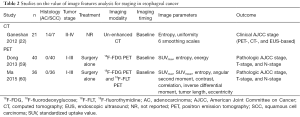
Full table
Dong et al. correlated 18F-FDG PET-based SUVmax, entropy, and energy before surgery with pathologic AJCC-stage, T-stage, and N-stage (59). Most of the studied correlations were weak to moderate only, with the exception of two strong correlations (AJCC-stage and entropy, Spearman’s r=0.63; T-stage and entropy, Spearman’s r=0.69) (59). Similar to the British study reported by Ganeshan et al. (22), higher clinical stage and node-positive tumors were associated with increased tumor heterogeneity (i.e., higher entropy) (59). In ROC curve analysis, an entropy value above 4.7 yielded a sensitivity of 78% and specificity of 73% for predicting pathologic AJCC-stage III as opposed to stage I-II (59). Unfortunately, a multivariable analysis to determine the potential incremental value of the entropy value to predict pathologic stage beyond conventional staging modalities (e.g., EUS, CT) was not performed (59).
Ma et al. included 36 patients who underwent both 18F-FDG and 18F-fluorothymidine (18F-FLT) PET, and compared the performance of 2 intensity, 2 geometry, and 5 texture features of both modalities for staging with pathologic AJCC-stage, T-stage, and N-stage as reference (60). They found that 18F-FDG PET features showed more significant associations with pathologic AJCC-stage and TN-stage than 18F-FLT PET features (60). Interestingly, SUVmax, tumor length, and eccentricity appeared more important than the studied texture features (e.g., entropy, correlation, contrast) for staging (60). Unfortunately, ROC curve analysis and multivariable analysis adjusting for conventional staging modalities were lacking, impairing proper interpretation of potential added value in clinical practice (60).
Prediction of treatment response
Esophageal tumors tend to respond differently to neoadjuvant chemo(radio)therapy or definitive CRT. Adenocarcinomas demonstrate a pathologic complete response (pCR; i.e., complete disappearance of viable tumor cells) to chemotherapy or CRT in 8–9% or 23–28% of patients, respectively (6,72,73), whereas squamous cell carcinomas have a pCR rate of 49% after CRT (6). A pCR is associated with favorable disease-free and overall survival rates, and it has been speculated that accurate identification of pCR prior to surgery could yield an organ-preserving approach avoiding unnecessary surgical morbidity (74-76). On the other hand, it is likely that non-responders to CRT (18–25%) or to chemotherapy (44–58%) are harmed by the toxicity of these therapies without prognostic benefit (6,8,77,78). Early identification of non-responders before or during treatment would be beneficial for this group as ineffective therapy could be modified or discontinued (advancing surgery without detrimental delay in the curative setting) (79).
Several diagnostic strategies have been proposed to predict response to treatment in esophageal cancer. The Response Evaluation Criteria in Solid Tumors (RECIST) method is often used for pre- and post-treatment CT scanning in the evaluation of response, but yields a poor sensitivity (33–55%) and moderate specificity (50–71%) for pathologic response (80). In fact, RECIST did not demonstrate any correlation with treatment response nor prognosis in a recent study in patients with esophageal cancer (81). Post-treatment endoscopic biopsy has a high specificity (91%), but poor sensitivity (35%) for detecting residual cancer, whereas EUS after treatment yields a high sensitivity (96%), but very low specificity (11%) (82). Sequential 18F-FDG PET-based SUV measurements are able to predict treatment response with a moderate sensitivity (67%) and specificity (68%) (37). In addition, some clinical parameters have been repeatedly found to yield minor—but independent—predictive ability for treatment response (i.e., gender, clinical T-stage, and histologic differentiation grade) (50,76,83). Unfortunately, so far even combinations of modalities and clinical parameters do not yield sufficient predictive ability for pathologic response to guide treatment decision-making in routine clinical practice, and a tool with improved accuracy is highly desired (37,50,84).
Radiomics
An overview of studies reporting on the value of radiomics for the prediction of treatment response in esophageal cancer is presented in Table 3. Studies that performed imaging before and after treatment reported that tumor heterogeneity generally decreased following treatment (36,50). It has been hypothesized that tumors could be rendered more homogeneous following treatment due to a reduction in cellular density and interstitial pressure, and normalization of the vasculature with improved intra-tumor perfusion and oxygenation (36).
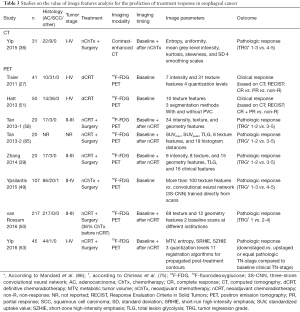
Full table
Yip et al. studied the value of contrast-enhanced CT image features analysis before and after neoadjuvant chemotherapy in 31 patients for the prediction of good versus poor pathologic response [tumor regression grade (TRG) 1-3 vs. 4-5] (36). Statistical significance was not reached for any of the univariable associations between image features and pathologic response with the exception of pre- and post-treatment SD, which however disappeared after correction for multiple testing (36). They also studied the value of >100 baseline 18F-FDG PET texture features versus a three-slices convolutional neural network (3S-CNN; which is trained directly from scans rather than ‘manually’ calculated) for the prediction of good versus poor pathologic response (n=217) (49). They found that 3S-CNN outperformed texture features analysis resulting in a sensitivity of 81% and specificity of 82% (49), but this finding has not yet been validated in other studies.
In two studies, a French group determined the associations of tumor texture features on baseline 18F-FDG PET scans with clinical response to definitive CRT (41,51). In their first study (n=41), the authors reported superior univariable discriminatory ability (area-under-the-curves [AUCs] 0.82–0.89) of several texture features (i.e., homogeneity, entropy, intensity variability, and size-zone variability) over SUVmax and SUVmean (AUCs 0.59–0.70) for the prediction of clinical complete response or non-response (41). Similarly, in their second study with a partly overlapping study population (n=50), good univariable discriminatory ability (AUCs 0.80–0.90) was achieved with several image features (i.e., MTV, entropy, homogeneity, dissimilarity, intensity variability, and zone percentage) for the prediction of clinical non-response (51). Important limitations of these studies (41,51) include the suboptimal reference standard defined by the CT-based RECIST method—which is known to correlate poorly to true (pathologic) response and survival (80,81)—and the lack of multivariable prediction modeling (adjusted for clinical parameters and other predictive modalities) impairing proper interpretation of potential incremental value in clinical practice.
Based on baseline and post-treatment 18F-FDG PET scans, investigators from the US aimed to predict pathologic response (TRG 1-2 vs. 3-5) to nCRT in the same 20 patients with esophageal cancer in three separate articles (39,58,85). By extracting 34 intensity, texture, and geometry features at both time points, they found that changes of features over treatment (∆features) appeared more predictive of response than pre- or post-treatment assessment alone (58). Baseline skewness, ∆SUVmean, post-treatment inertia (contrast), correlation, and cluster prominence were found to be significant predictors of pathologic response in univariable analysis (AUCs 0.76–0.85) (58). In the second study, cross-bin histogram distance features were studied (capturing both 18F-FDG uptake distribution and longitudinal information), resulting in slightly higher prediction accuracies than texture features (85). This finding requires validation as to date no other studies have reported on cross-bin histogram distance features in esophageal cancer imaging. In the third study, multivariable support vector machine (SVM) and logistic regression models were constructed including 33 18F-FDG PET image features as well as 16 clinical parameters (39). SVM models achieved higher accuracy than logistic regression models, particularly in models combining many variables (maximum AUC 1.00 vs. 0.90) (39), but it is important to acknowledge that substantial model overfitting has likely occurred given the small sample size and large amount of predictors included in the modeling, resulting in overoptimistic results.
van Rossum et al. studied the value of clinical parameters along with subjective and quantitative parameters from baseline and post-treatment 18F-FDG PET scans in 217 patients with esophageal adenocarcinoma for the prediction of pCR as opposed to residual cancer after nCRT (50). Similar to other studies, lower baseline heterogeneity and a greater change towards more homogeneous 18F-FDG uptake after treatment were associated with better response (50). In multivariable analysis and after internal validation using bootstrapping techniques, both 18F-FDG PET-based subjective assessment of response and texture features analysis provided incremental value beyond clinical predictors, but this discriminatory improvement did not translate into a clinically relevant benefit as determined by decision-curve analysis (50).
Yip et al. addressed the time-consuming issue of contouring longitudinal scans and investigated the usefulness of 11 different registration algorithms for post-treatment contour propagation in relation to their ability to predict pathologic response (53). They showed that propagated contours could be constructed fast (<30 seconds) and that 3 texture features (e.g., entropy) resulting from most algorithms significantly predicted pathologic responders (AUCs 0.72–0.78), with the exception of fast-demons and fast-free-form deformable algorithms, and rigidly propagated contours, which should therefore not be used (53). An uncommon reference standard was used consisting of pathologic TN-downstaging (responders) versus TN-upstaging or no change in stage (non-responders) as compared to the baseline clinical TN-stage (53). This endpoint was likely suboptimal since it has not been validated as surrogate marker for long-term outcomes and clinical TN-staging is inaccurate in many cases.
Prediction of survival
Accurate stratification of patients according to their expected prognosis is crucial at the time of diagnosis as well as throughout treatment and follow-up. The most important prognostic factor before treatment is the clinical TNM-stage, and -to a lesser extent- the initial SUVmax value (4,87). Endoscopic biopsy, subjective 18F-FDG PET-based response, and SUVmax after chemo (radio)therapy are other parameters with some prognostic value (38,87,88). After surgery, pathologic TNM-staging, lymph node ratio, extracapsular lymph node involvement, radicality of resection, and pathologic response to neoadjuvant therapy have prognostic impact (75,76,89-92). Despite the availability of these prognostic factors, we are still failing our patients in terms of accurate individualized prediction of survival probability resulting in inaccurate patient selection for different treatment approaches, as for example can be seen from the high number of patients with very early progression (24–41% within 1 year) after treatment with curative intent (93).
Radiomics
Studies on the value of radiomics for the prediction of survival in esophageal cancer are outlined in Table 4. Three studies from the UK have described predictive value for survival using texture features based on baseline unenhanced CT (22) or pre- and post-treatment contrast-enhanced CT (35,36). Ganeshan et al. included 21 patients with esophageal cancer for which the important prognostic factor of treatment was not reported- and studied tumor entropy and uniformity for 6 smoothing scales (22). It was demonstrated that the CT-based coarse uniformity feature was superiorly predictive for overall survival, even resulting in redundancy of clinical TNM-stage and SUVmax in a stepwise forward Cox regression analysis, suggesting substantial overlap in information (22). In the two other studies, tumor entropy, uniformity, mean grey-level intensity, kurtosis, skewness, and SD for 4 quantization levels on baseline and post-treatment contrast-enhanced CT was related to overall survival (35,36). After adjusting for tumor stage and age, post-treatment entropy and uniformity features on a medium to coarse scale remained significant prognostic factors in 36 patients who underwent definitive CRT (35). Yip et al. reported that a relative change in skewness on a fine smoothing scale was associated with survival in 31 patients who underwent neoadjuvant chemotherapy followed by surgery (36).
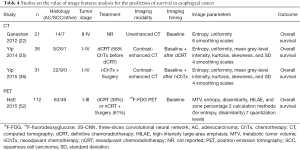
Full table
In a French study that included baseline 18F-FDG PET scans of 555 cancer patients (of whom 112 had esophageal cancer and underwent definitive CRT or nCRT followed by surgery), Hatt et al. assessed the value of MTV, entropy, dissimilarity, high-intensity large-area emphasis, and zone percentage for the prediction of overall survival (52). Although MTV and heterogeneity (along with tumor stage) were independent prognostic factors in non-small cell lung cancer, these parameters had less complementary value in esophageal cancer which was attributed to smaller overall volumes (52). The local dissimilarity parameter appeared most predictive for overall survival in the patients with esophageal cancer (52).
Conclusions
Since the first publication on image texture feature analysis in esophageal cancer in the year 2011 (41), the body of evidence on radiomics in this setting has been growing steadily suggesting potential incremental value for staging, prediction of response to chemo(radio)therapy, and predicting survival. As such, radiomics approaches may contribute to the ongoing movement towards more individualized treatment strategies for these patients. An advantage of this emerging field is that it can fit in within existing practice without imposing additional burden to patients, as it involves post-processing techniques on standard CT or 18F-FDG PET images which are performed as part of routine clinical practice. However, current evidence is still exploratory in nature and further validation in larger studies is required before implementation in clinical practice could be considered.
Acknowledgement and further evaluation of limitations with respect to reproducibility of image features and the substantial influence of varying smoothing scales, quantization levels, and contouring methods is of crucial importance to move this field forward. To this regard, parameters such as local entropy derived from GLCMs (and to a lesser extent uniformity, dissimilarity, or zone percentage) for tumor heterogeneity characterization should be preferred, as these appear most reproducible and robust, and have repeatedly shown high predictive ability for staging, prediction of response, and prediction of survival. Standardization of imaging and radiomics approaches, multivariable prediction modeling focusing on incremental value of radiomics beyond conventional diagnostics and predictors, and validation of findings are key to successful future introduction of radiomics in the clinical management of esophageal cancer.
Acknowledgments
Funding: Funding was provided in part by The University of Texas MD Anderson Cancer Center and by the National Cancer Institute Cancer Center Support Grant CA016672.
Footnote
Provenance and Peer Review: This article was commissioned by the editorial office, Translational Cancer Research for the series “Radiomics in Radiation Oncology”. The article has undergone external peer review.
Conflicts of Interest: Steven H. Lin has research grant support from Elekta, STCube Pharmaceuticals, Roche/Genentech, and Peregrine Pharmaceuticals, and has received honorarium from AstraZeneca and US Oncology. The other authors have no conflicts of interest to declare.
Ethical Statement: The authors are accountable for all aspects of the work in ensuring that questions related to the accuracy or integrity of any part of the work are appropriately investigated and resolved.
Open Access Statement: This is an Open Access article distributed in accordance with the Creative Commons Attribution-NonCommercial-NoDerivs 4.0 International License (CC BY-NC-ND 4.0), which permits the non-commercial replication and distribution of the article with the strict proviso that no changes or edits are made and the original work is properly cited (including links to both the formal publication through the relevant DOI and the license). See: https://creativecommons.org/licenses/by-nc-nd/4.0/.
References
- Pennathur A, Gibson MK, Jobe BA, et al. Oesophageal carcinoma. Lancet 2013;381:400-12. [Crossref] [PubMed]
- Enzinger PC, Mayer RJ. Esophageal cancer. N Engl J Med 2003;349:2241-52. [Crossref] [PubMed]
- Omloo JM, Lagarde SM, Hulscher JB, et al. Extended transthoracic resection compared with limited transhiatal resection for adenocarcinoma of the mid/distal esophagus: five-year survival of a randomized clinical trial. Ann Surg 2007;246:992-1000; discussion 1000-1. [Crossref] [PubMed]
- Rice TW, Rusch VW, Ishwaran H, et al. Cancer of the esophagus and esophagogastric junction: data-driven staging for the seventh edition of the American Joint Committee on Cancer/International Union Against Cancer Cancer Staging Manuals. Cancer 2010;116:3763-73.
- Bhatt A, Abe S, Kumaravel A, et al. Indications and Techniques for Endoscopic Submucosal Dissection. Am J Gastroenterol 2015;110:784-91. [Crossref] [PubMed]
- van Hagen P, Hulshof MC, van Lanschot JJ, et al. Preoperative chemoradiotherapy for esophageal or junctional cancer. N Engl J Med 2012;366:2074-84. [Crossref] [PubMed]
- Cunningham D, Allum WH, Stenning SP, et al. Perioperative chemotherapy versus surgery alone for resectable gastroesophageal cancer. N Engl J Med 2006;355:11-20. [Crossref] [PubMed]
- Sjoquist KM, Burmeister BH, Smithers BM, et al. Survival after neoadjuvant chemotherapy or chemoradiotherapy for resectable oesophageal carcinoma: an updated meta-analysis. Lancet Oncol 2011;12:681-92. [Crossref] [PubMed]
- Wong R, Malthaner R. Combined chemotherapy and radiotherapy (without surgery) compared with radiotherapy alone in localized carcinoma of the esophagus. Cochrane Database Syst Rev 2006;1:CD002092 [PubMed]
- Homs MY, van der Gaast A, Siersema PD, et al. Chemotherapy for metastatic carcinoma of the esophagus and gastro-esophageal junction. Cochrane Database Syst Rev 2006;4:CD004063 [PubMed]
- Cunningham D, Starling N, Rao S, et al. Capecitabine and oxaliplatin for advanced esophagogastric cancer. N Engl J Med 2008;358:36-46. [Crossref] [PubMed]
- Diederich S. Staging of oesophageal cancer. Cancer Imaging 2007;7:S63-6. [Crossref] [PubMed]
- Jamil LH, Gill KR, Wallace MB. Staging and restaging of advanced esophageal cancer. Curr Opin Gastroenterol 2008;24:530-4. [Crossref] [PubMed]
- van Rossum PS, van Lier AL, Lips IM, et al. Imaging of oesophageal cancer with FDG-PET/CT and MRI. Clin Radiol 2015;70:81-95. [Crossref] [PubMed]
- Räsänen JV, Sihvo EI, Knuuti MJ, et al. Prospective analysis of accuracy of positron emission tomography, computed tomography, and endoscopic ultrasonography in staging of adenocarcinoma of the esophagus and the esophagogastric junction. Ann Surg Oncol 2003;10:954-60. [Crossref] [PubMed]
- Munden RF, Macapinlac HA, Erasmus JJ. Esophageal cancer: the role of integrated CT-PET in initial staging and response assessment after preoperative therapy. J Thorac Imaging 2006;21:137-45. [Crossref] [PubMed]
- Bruzzi JF, Swisher SG, Truong MT, et al. Detection of interval distant metastases: clinical utility of integrated CT-PET imaging in patients with esophageal carcinoma after neoadjuvant therapy. Cancer 2007;109:125-34. [Crossref] [PubMed]
- Monjazeb AM, Riedlinger G, Aklilu M, et al. Outcomes of patients with esophageal cancer staged with 18F-fluorodeoxyglucose positron emission tomography (FDG-PET): can postchemoradiotherapy FDG-PET predict the utility of resection? J Clin Oncol 2010;28:4714-21. [Crossref] [PubMed]
- Blom RL, Schreurs WM, Belgers HJ, et al. The value of post-neoadjuvant therapy PET-CT in the detection of interval metastases in esophageal carcinoma. Eur J Surg Oncol 2011;37:774-8. [Crossref] [PubMed]
- Goense L, van Rossum PS, Reitsma JB, et al. Diagnostic Performance of 18F-FDG PET and PET/CT for the Detection of Recurrent Esophageal Cancer After Treatment with Curative Intent: A Systematic Review and Meta-Analysis. J Nucl Med 2015;56:995-1002. [Crossref] [PubMed]
- Lambin P, Rios-Velazquez E, Leijenaar R, et al. Radiomics: extracting more information from medical images using advanced feature analysis. Eur J Cancer 2012;48:441-6. [Crossref] [PubMed]
- Ganeshan B, Skogen K, Pressney I, et al. Tumour heterogeneity in oesophageal cancer assessed by CT texture analysis: preliminary evidence of an association with tumour metabolism, stage, and survival. Clin Radiol 2012;67:157-64. [Crossref] [PubMed]
- Maley CC, Galipeau PC, Finley JC, et al. Genetic clonal diversity predicts progression to esophageal adenocarcinoma. Nat Genet 2006;38:468-73. [Crossref] [PubMed]
- Gerlinger M, Rowan AJ, Horswell S, et al. Intratumor heterogeneity and branched evolution revealed by multiregion sequencing. N Engl J Med 2012;366:883-92. [Crossref] [PubMed]
- Marusyk A, Almendro V, Polyak K. Intra-tumour heterogeneity: a looking glass for cancer? Nat Rev Cancer 2012;12:323-34. [Crossref] [PubMed]
- Fisher R, Pusztai L, Swanton C. Cancer heterogeneity: implications for targeted therapeutics. Br J Cancer 2013;108:479-85. [Crossref] [PubMed]
- Tixier F, Le Rest CC, Hatt M, et al. Intratumor heterogeneity characterized by textural features on baseline 18F-FDG PET images predicts response to concomitant radiochemotherapy in esophageal cancer. J Nucl Med 2011;52:369-78. [Crossref] [PubMed]
- Chicklore S, Goh V, Siddique M, et al. Quantifying tumour heterogeneity in 18F-FDG PET/CT imaging by texture analysis. Eur J Nucl Med Mol Imaging 2013;40:133-40. [Crossref] [PubMed]
- Goh V, Sanghera B, Wellsted DM, et al. Assessment of the spatial pattern of colorectal tumour perfusion estimated at perfusion CT using two-dimensional fractal analysis. Eur Radiol 2009;19:1358-65. [Crossref] [PubMed]
- Goh V, Ganeshan B, Nathan P, et al. Assessment of response to tyrosine kinase inhibitors in metastatic renal cell cancer: CT texture as a predictive biomarker. Radiology 2011;261:165-71. [Crossref] [PubMed]
- Mu W, Chen Z, Liang Y, et al. Staging of cervical cancer based on tumor heterogeneity characterized by texture features on 18F-FDG PET images. Phys Med Biol 2015;60:5123-39. [Crossref] [PubMed]
- Fried DV, Tucker SL, Zhou S, et al. Prognostic value and reproducibility of pretreatment CT texture features in stage III non-small cell lung cancer. Int J Radiat Oncol Biol Phys 2014;90:834-42. [Crossref] [PubMed]
- Fried DV, Mawlawi O, Zhang L, et al. Stage III Non-Small Cell Lung Cancer: Prognostic Value of FDG PET Quantitative Imaging Features Combined with Clinical Prognostic Factors. Radiology 2016;278:214-22. [Crossref] [PubMed]
- Knogler T, El-Rabadi K, Weber M, et al. Three-dimensional texture analysis of contrast enhanced CT images for treatment response assessment in Hodgkin lymphoma: comparison with F-18-FDG PET. Med Phys 2014;41:121904 [Crossref] [PubMed]
- Yip C, Landau D, Kozarski R, et al. Primary esophageal cancer: heterogeneity as potential prognostic biomarker in patients treated with definitive chemotherapy and radiation therapy. Radiology 2014;270:141-8. [Crossref] [PubMed]
- Yip C, Davnall F, Kozarski R, et al. Assessment of changes in tumor heterogeneity following neoadjuvant chemotherapy in primary esophageal cancer. Dis Esophagus 2015;28:172-9. [Crossref] [PubMed]
- Kwee RM. Prediction of tumor response to neoadjuvant therapy in patients with esophageal cancer with use of 18F FDG PET: a systematic review. Radiology 2010;254:707-17. [Crossref] [PubMed]
- Zhu W, Xing L, Yue J, et al. Prognostic significance of SUV on PET/CT in patients with localised oesophagogastric junction cancer receiving neoadjuvant chemotherapy/chemoradiation:a systematic review and meta-analysis. Br J Radiol 2012;85:e694-701. [Crossref] [PubMed]
- Zhang H, Tan S, Chen W, et al. Modeling pathologic response of esophageal cancer to chemoradiation therapy using spatial-temporal 18F-FDG PET features, clinical parameters, and demographics. Int J Radiat Oncol Biol Phys 2014;88:195-203. [Crossref] [PubMed]
- Dong X, Wu P, Sun X, et al. Intra-tumour 18F-FDG uptake heterogeneity decreases the reliability on target volume definition with positron emission tomography/computed tomography imaging. J Med Imaging Radiat Oncol 2015;59:338-45. [Crossref] [PubMed]
- Tixier F, Hatt M, Pradier O, et al. Intra-tumor heterogeneity on baseline 18F-FDG PET images characterized by textural features predicts response to concomitant radio-chemotherapy in esophageal cancer. Med Nucl 2011;35:265.
- El Naqa I, Grigsby P, Apte A, et al. Exploring feature-based approaches in PET images for predicting cancer treatment outcomes. Pattern Recognit 2009;42:1162-71. [Crossref] [PubMed]
- Roedl JB, Colen RR, Holalkere NS, et al. Adenocarcinomas of the esophagus: response to chemoradiotherapy is associated with decrease of metabolic tumor volume as measured on PET-CT. Comparison to histopathologic and clinical response evaluation. Radiother Oncol 2008;89:278-86. [Crossref] [PubMed]
- Blom RL, Steenbakkers IR, Lammering G, et al. PET/CT-based metabolic tumour volume for response prediction of neoadjuvant chemoradiotherapy in oesophageal carcinoma. Eur J Nucl Med Mol Imaging 2013;40:1500-6. [Crossref] [PubMed]
- Hatt M, Visvikis D, Pradier O, et al. Baseline 18F-FDG PET image-derived parameters for therapy response prediction in oesophageal cancer. Eur J Nucl Med Mol Imaging. 2011;38:1595-606. [Crossref] [PubMed]
- Tixier F, Hatt M, Le Rest CC, et al. Reproducibility of tumor uptake heterogeneity characterization through textural feature analysis in 18F-FDG PET. J Nucl Med 2012;53:693-700. [Crossref] [PubMed]
- Castellano G, Bonilha L, Li LM, et al. Texture analysis of medical images. Clin Radiol 2004;59:1061-9. [Crossref] [PubMed]
- Doumou G, Siddique M, Tsoumpas C, et al. The precision of textural analysis in 18F-FDG-PET scans of oesophageal cancer. Eur Radiol 2015;25:2805-12. [Crossref] [PubMed]
- Ypsilantis PP, Siddique M, Sohn HM, et al. Predicting Response to Neoadjuvant Chemotherapy with PET Imaging Using Convolutional Neural Networks. PLoS One 2015;10:e0137036 [Crossref] [PubMed]
- van Rossum PS, Fried DV, Zhang L, et al. The Incremental Value of Subjective and Quantitative Assessment of 18F-FDG PET for the Prediction of Pathologic Complete Response to Preoperative Chemoradiotherapy in Esophageal Cancer. J Nucl Med 2016;57:691-700. [Crossref] [PubMed]
- Hatt M, Tixier F, Cheze Le Rest C, et al. Robustness of intratumour 18F-FDG PET uptake heterogeneity quantification for therapy response prediction in oesophageal carcinoma. Eur J Nucl Med Mol Imaging 2013;40:1662-71. [Crossref] [PubMed]
- Hatt M, Majdoub M, Vallieres M, et al. 18F-FDG PET uptake characterization through texture analysis: investigating the complementary nature of heterogeneity and functional tumor volume in a multi-cancer site patient cohort. J Nucl Med 2015;56:38-44. [Crossref] [PubMed]
- Yip SS, Coroller TP, Sanford NN, et al. Use of registration-based contour propagation in texture analysis for esophageal cancer pathologic response prediction. Phys Med Biol 2016;61:906-22. [Crossref] [PubMed]
- Galavis PE, Hollensen C, Jallow N, et al. Variability of textural features in FDG PET images due to different acquisition modes and reconstruction parameters. Acta Oncol 2010;49:1012-6. [Crossref] [PubMed]
- Leijenaar RT, Nalbantov G, Carvalho S, et al. The effect of SUV discretization in quantitative FDG-PET Radiomics: the need for standardized methodology in tumor texture analysis. Sci Rep 2015;5:11075. [Crossref] [PubMed]
- Zaidi H, El Naqa I. PET-guided delineation of radiation therapy treatment volumes: a survey of image segmentation techniques. Eur J Nucl Med Mol Imaging 2010;37:2165-87. [Crossref] [PubMed]
- Lee JA. Segmentation of positron emission tomography images: some recommendations for target delineation in radiation oncology. Radiother Oncol 2010;96:302-7. [Crossref] [PubMed]
- Tan S, Kligerman S, Chen W, et al. Spatial-temporal [18F]FDG-PET features for predicting pathologic response of esophageal cancer to neoadjuvant chemoradiation therapy. Int J Radiat Oncol Biol Phys 2013;85:1375-82. [Crossref] [PubMed]
- Dong X, Xing L, Wu P, et al. Three-dimensional positron emission tomography image texture analysis of esophageal squamous cell carcinoma: relationship between tumor 18F-fluorodeoxyglucose uptake heterogeneity, maximum standardized uptake value, and tumor stage. Nucl Med Commun 2013;34:40-6. [Crossref] [PubMed]
- Ma C, Li D, Yin Y, et al. Comparison of characteristics of 18F-fluorodeoxyglucose and 18F-fluorothymidine PET during staging of esophageal squamous cell carcinoma. Nucl Med Commun 2015;36:1181-6. [Crossref] [PubMed]
- Werner-Wasik M, Nelson AD, Choi W, et al. What is the best way to contour lung tumors on PET scans? Multiobserver validation of a gradient-based method using a NSCLC digital PET phantom. Int J Radiat Oncol Biol Phys 2012;82:1164-71. [Crossref] [PubMed]
- Hatt M, Cheze le Rest C, Turzo A, et al. A fuzzy locally adaptive Bayesian segmentation approach for volume determination in PET. IEEE Trans Med Imaging 2009;28:881-93. [Crossref] [PubMed]
- Puli SR, Reddy JB, Bechtold ML, et al. Staging accuracy of esophageal cancer by endoscopic ultrasound: a meta-analysis and systematic review. World J Gastroenterol 2008;14:1479-90. [Crossref] [PubMed]
- van Vliet EP, Eijkemans MJ, Kuipers EJ, et al. Publication bias does not play a role in the reporting of the results of endoscopic ultrasound staging of upper gastrointestinal cancers. Endoscopy 2007;39:325-32. [Crossref] [PubMed]
- Preston SR, Clark GW, Martin IG, et al. Effect of endoscopic ultrasonography on the management of 100 consecutive patients with oesophageal and junctional carcinoma. Br J Surg 2003;90:1220-4. [Crossref] [PubMed]
- Wakelin SJ, Deans C, Crofts TJ, et al. A comparison of computerised tomography, laparoscopic ultrasound and endoscopic ultrasound in the preoperative staging of oesophago-gastric carcinoma. Eur J Radiol 2002;41:161-7. [Crossref] [PubMed]
- van Vliet EP, Eijkemans MJ, Poley JW, et al. Staging of esophageal carcinoma in a low-volume EUS center compared with reported results from high-volume centers. Gastrointest Endosc 2006;63:938-47. [Crossref] [PubMed]
- van Zoonen M, van Oijen MG, van Leeuwen MS, et al. Low impact of staging EUS for determining surgical resectability in esophageal cancer. Surg Endosc 2012;26:2828-34. [Crossref] [PubMed]
- van Vliet EP, Heijenbrok-Kal MH, Hunink MG, et al. Staging investigations for oesophageal cancer: a meta-analysis. Br J Cancer 2008;98:547-57. [Crossref] [PubMed]
- van Westreenen HL, Westerterp M, Bossuyt PM, et al. Systematic review of the staging performance of 18F-fluorodeoxyglucose positron emission tomography in esophageal cancer. J Clin Oncol 2004;22:3805-12. [Crossref] [PubMed]
- Hofstetter WL. Preoperative Chemoradiation in an Era of Suboptimal Clinical Staging. JAMA Surg 2016;151:245-6. [Crossref] [PubMed]
- van der Sluis PC, Ubink I, van der Horst S, et al. Safety, efficacy, and long-term follow-up evaluation of perioperative epirubicin, cisplatin, and capecitabine chemotherapy in esophageal resection for adenocarcinoma. Ann Surg Oncol 2015;22:1555-63. [Crossref] [PubMed]
- Klevebro F, Alexandersson von Dobeln G, Wang N, et al. A randomized clinical trial of neoadjuvant chemotherapy versus neoadjuvant chemoradiotherapy for cancer of the oesophagus or gastro-oesophageal junction. Ann Oncol 2016;27:660-7. [Crossref] [PubMed]
- Donahue JM, Nichols FC, Li Z, et al. Complete pathologic response after neoadjuvant chemoradiotherapy for esophageal cancer is associated with enhanced survival. Ann Thorac Surg 2009;87:392-8; discussion 398-9. [Crossref] [PubMed]
- Chirieac LR, Swisher SG, Ajani JA, et al. Posttherapy pathologic stage predicts survival in patients with esophageal carcinoma receiving preoperative chemoradiation. Cancer 2005;103:1347-55. [Crossref] [PubMed]
- Ajani JA, Correa AM, Hofstetter WL, et al. Clinical parameters model for predicting pathologic complete response following preoperative chemoradiation in patients with esophageal cancer. Ann Oncol. 2012;23:2638-42. [Crossref] [PubMed]
- Webb A, Cunningham D, Scarffe JH, et al. Randomized trial comparing epirubicin, cisplatin, and fluorouracil versus fluorouracil, doxorubicin, and methotrexate in advanced esophagogastric cancer. J Clin Oncol 1997;15:261-7. [Crossref] [PubMed]
- Ross P, Nicolson M, Cunningham D, et al. Prospective randomized trial comparing mitomycin, cisplatin, and protracted venous-infusion fluorouracil (PVI 5-FU) with epirubicin, cisplatin, and PVI 5-FU in advanced esophagogastric cancer. J Clin Oncol 2002;20:1996-2004. [Crossref] [PubMed]
- van Rossum PS, van Lier AL, van Vulpen M, et al. Diffusion-weighted magnetic resonance imaging for the prediction of pathologic response to neoadjuvant chemoradiotherapy in esophageal cancer. Radiother Oncol 2015;115:163-70. [Crossref] [PubMed]
- Westerterp M, van Westreenen HL, Reitsma JB, et al. Esophageal cancer: CT, endoscopic US, and FDG PET for assessment of response to neoadjuvant therapy--systematic review. Radiology 2005;236:841-51. [Crossref] [PubMed]
- Yanagawa M, Tatsumi M, Miyata H, et al. Evaluation of Response to Neoadjuvant Chemotherapy for Esophageal Cancer: PET Response Criteria in Solid Tumors Versus Response Evaluation Criteria in Solid Tumors. J Nucl Med 2012;53:872-80. [Crossref] [PubMed]
- van Rossum PS, Goense L, Meziani J, et al. Endoscopic biopsy and EUS for the detection of pathologic complete response after neoadjuvant chemoradiotherapy in esophageal cancer: a systematic review and meta-analysis. Gastrointest Endosc 2016;83:866-79. [Crossref] [PubMed]
- Toxopeus EL, Nieboer D, Shapiro J, et al. Nomogram for predicting pathologically complete response after neoadjuvant chemoradiotherapy for oesophageal cancer. Radiother Oncol 2015;115:392-8. [Crossref] [PubMed]
- Cheedella NK, Suzuki A, Xiao L, et al. Association between clinical complete response and pathological complete response after preoperative chemoradiation in patients with gastroesophageal cancer: analysis in a large cohort. Ann Oncol 2013;24:1262-6. [Crossref] [PubMed]
- Tan S, Zhang H, Zhang Y, et al. Predicting pathologic tumor response to chemoradiotherapy with histogram distances characterizing longitudinal changes in 18F-FDG uptake patterns. Med Phys. 2013;40:101707 [Crossref] [PubMed]
- Mandard AM, Dalibard F, Mandard JC, et al. Pathologic assessment of tumor regression after preoperative chemoradiotherapy of esophageal carcinoma. Clinicopathologic correlations. Cancer 1994;73:2680-6. [Crossref] [PubMed]
- Suzuki A, Xiao L, Hayashi Y, et al. Nomograms for prognostication of outcome in patients with esophageal and gastroesophageal carcinoma undergoing definitive chemoradiotherapy. Oncology 2012;82:108-13. [Crossref] [PubMed]
- Lin SH, Wang J, Allen PK, et al. A nomogram that predicts pathologic complete response to neoadjuvant chemoradiation also predicts survival outcomes after definitive chemoradiation for esophageal cancer. J Gastrointest Oncol 2015;6:45-52. [PubMed]
- Verhage RJ, Zandvoort HJ, ten Kate FJ, et al. How to define a positive circumferential resection margin in T3 adenocarcinoma of the esophagus. Am J Surg Pathol 2011;35:919-26. [Crossref] [PubMed]
- Lagarde SM, Reitsma JB, de Castro SM, et al. Prognostic nomogram for patients undergoing oesophagectomy for adenocarcinoma of the oesophagus or gastro-oesophageal junction. Br J Surg 2007;94:1361-8. [Crossref] [PubMed]
- Lagarde SM, Reitsma JB, Ten Kate FJ, et al. Predicting individual survival after potentially curative esophagectomy for adenocarcinoma of the esophagus or gastroesophageal junction. Ann Surg 2008;248:1006-13. [Crossref] [PubMed]
- Nafteux PR, Lerut AM, Moons J, et al. International Multicenter Study on the Impact of Extracapsular Lymph Node Involvement in Primary Surgery Adenocarcinoma of the Esophagus on Overall Survival and Staging Systems. Ann Surg 2015;262:809-15; discussion 815-6. [Crossref] [PubMed]
- Shapiro J, van Lanschot JJ, Hulshof MC, et al. Neoadjuvant chemoradiotherapy plus surgery versus surgery alone for oesophageal or junctional cancer (CROSS): long-term results of a randomised controlled trial. Lancet Oncol 2015;16:1090-8. [Crossref] [PubMed]