Construction of a prognostic score model for breast cancer based on multi-omics analysis of study on bone metastasis
Highlight box
Key findings
• A prognostic prediction model was constructed based on 28 key differentially expressed genes (DEGs) identified through multi-omics analysis of studies on bone metastasis in breast cancer (BRCA).
What is known and what is new?
• BRCA is the most common type of cancer and the second leading cause of cancer-related death in women all over the world. Many prognostic models for BRCA have been developed, but only a few have been extensively validated in various settings. Furthermore, their performance is suboptimal in independent populations, particularly in patients with high risk and in young and elderly patients.
• Bone is the most common site of metastasis for BRCA, with the 5-year overall survival rate being about 20%. Therefore, bone metastasis is an indicator of poor prognosis in BRCA patients. We developed a prognostic score model for prognostic prediction in BRCA based on the genes associated with bone metastasis of BRCA and externally validated the reproducibility and generalizability of this prognostic model.
What is the implication, and what should change now?
• The prognostic prediction model of BRCA in this study can provide a promising method for clinical helping on clinical decision making and prognosis prediction for BRCA patients. Meanwhile, screening for prognostic biomarker genes and pathways in BRCA may provide potential therapeutic targets for future treatment.
Introduction
Breast cancer (BRCA) is the most common type of cancer, with an estimated 2.26 million cases recorded in 2020. The global age standardized incidence rate in females is estimated to be 48/100,000 and has been rising in the past four decades (1). During the most recent data years [2010–2019], the rate increased by 0.5% annually. At present, BRCA is the second leading cause of cancer-related death among women overall, second to lung cancer, posing a serious threat to women’s health (2,3).
It is a heterogeneous disease with significant differences between patients and even within each tumor (4). Based on cancer statistics, in the United States, the average 5-year and 10-year survival rates for women with non-metastatic invasive BRCA are 90% and 84%, respectively (5). Although the median survival and 5-year relative survival for de novo metastatic BRCA increased over the years, especially in younger women, the 5-year relative survival was not more than 40% (6). Moreover, a large percentage of cancer survivors who were initially diagnosed with early-stage (I–III) cancer later experienced a recurrence or progression to metastatic disease according to the analysis of US mortality data from the National Center for Health Statistics and US Census Bureau populations (3). Approximately 4% of women with a history of BRCA have metastatic disease, more than half of whom were initially diagnosed with early-stage BRCA (3,6). The common sites of metastasis include bone, lung, liver, and brain. Among the above metastatic sites, bone metastasis is most common, accounting for approximately 65–75% of metastasis cases, with the 5-year overall survival (OS) rate being about 20% (7-9). Therefore, bone metastasis is an indicator of poor prognosis in BRCA patients. Current treatments for bone metastasis include systemic therapies such as chemotherapy and endocrine therapy to slow the proliferation of cancer cells; bone-targeted treatments such as potent bisphosphonates or denosumab to inhibit the excessive bone destruction associated with cancer; and the use of bone-seeking radionucleotides (10). However, even these treatments for many patients result in a major reduction in skeletal complications, reduced bone pain and improved quality of life, they are only palliative (10). New treatment markers and targets are needed to achieve better prognosis.
Although BRCA subtypes partly indicate the preferential site of metastasis and help to guide clinical therapeutics, the development of more effective treatments against BRCA metastases depends on a systematic and deepened understanding of the molecular mechanism of metastatic heterogeneity (7). Bone metastasis of BRCA is associated with activation of several pathways, including epithelial-mesenchymal transition (EMT), angiogenesis, and interleukin 1 beta (IL-1β) pathway (11-13). However, the genetic and molecular mechanism of bone metastasis of BRCA remains unclear. With the development of gene expression profiling, such as RNA-seq and single-cell RNA-seq, researchers have identified gene expression signatures that are associated with bone metastasis or survival outcomes of BRCA (14,15). Recently, public database bioinformatics analysis has been widely used to investigate prognostic biomarkers in disease progression, and predictive models can also be used to assess prognosis in BRCA patients (16-18). As a result, identifying reliable signature genes and clinical factors will serve as a guide for clinical decision-making. In this study, we comprehensively analyzed the RNA-seq data from a BRCA cohort to construct a BRCA prognostic model based on differentially expressed genes (DEGs) in bone metastasis, and it can help identify patients at high risk of bone metastasis for early intervention. We present this article in accordance with the TRIPOD reporting checklist (available at https://tcr.amegroups.com/article/view/10.21037/tcr-23-1881/rc).
Methods
Data and patient samples extraction
This study was conducted in accordance with the Declaration of Helsinki (as revised in 2013). We downloaded the raw mRNA expression and clinical data of BRCA patients from The Cancer Genome Atlas (TCGA) database including normal group (n=113), tumor group (n=1,109). Limma package of R software was used to identify DEGs, with a significance threshold of P<0.01. Additionally, we downloaded the Series Matrix File data files of GSE20685 (annotation platform was GPL570), GSE2034 (annotation platform was GPL96), GSE124647 (annotation platform was GPL96), and GSE39494 (annotation platform was GPL6840) from the NCBI Gene Expression Omnibus (GEO) database. In GSE20685, a total of 327 BRCA patients with complete expression profiles and survival information were collected. GSE2034 was comprised of 286 samples, including 217 patients without bone metastasis and 69 patients with bone metastasis. GSE124647 featured 30 samples, consisting of 19 patients without bone metastasis and 11 patients with bone metastasis. Lastly, GSE39494 encompassed expression profile data of 10 samples, including 5 patients without bone metastasis and 5 patients with bone metastasis.
Gene Ontology (GO) and Kyoto Encyclopedia of Genes and Genomes (KEGG) pathway enrichment analysis
Enrichment analyses of DEGs were performed using Metascape (www.metascape.org). We conducted biological pathway analysis of specific genes through GO terminology and KEGG pathways. A Min overlap ≥3 & P≤0.01 was considered statistically significant.
Construction of a prognosis-predicting model based on the bone metastasis-related DEGs in BRCA
The DEGs associated with bone metastases were carefully selected, and a prognostic-predicting model was constructed using the least absolute shrinkage and selection operator (LASSO) Cox regression. In the LASSO regression analysis, a risk score for each patient was calculated for each patient by incorporating the expression values of specific genes and weighting them with the corresponding estimated regression coefficients. Based on their risk scores, the patients were categorized into low- and high-risk groups, using the median risk score as the cut-off point. The survival differences between the two groups were evaluated using Kaplan-Meier and compared using log-rank statistical methods. Moreover, the predictive capability of the risk score model for patient prognosis was examined using LASSO regression analysis and stratified analysis. Additionally, to assess the prediction accuracy of the model, the receiver operating characteristic (ROC) curve was plotted.
Drug sensitivity analysis
Based on the Genomics of Drug Sensitivity in Cancer (GDSC) databases (https://www.cancerrxgene.org/), we used pRRophetic to predict the chemosensitivity of each tumor sample. Cox regression was employed to acquire estimated half maximal inhibitory concentration (IC50) values for individual chemotherapy drug treatment, and 10-fold cross-validations were conducted with the GDSC training dataset to evaluate the accuracy of regression and prediction.
Infiltrating immune cells analysis
The CIBERSORT algorithm was employed to estimate the relative proportions of 22 types of infiltrating immune cells using normalized gene expression data obtained from various subgroups of BRCA patients. P<0.05 was considered statistically different.
Gene set variation analysis (GSVA)
GSVA was used to estimate variation of gene set enrichment through the samples in an unsupervised manner. Gene sets were downloaded from the Molecular signatures database (v7.0). GSVA algorithm was used to score each gene set to evaluate the potential biological function changes of different samples.
Gene set enrichment analysis (GSEA)
GSEA (http://www.broadinstitute.org/gsea) of BRCA patients’ expression profiles was conducted on the TCGA database to identify genes showing differential expression between patients in the low- and high-risk groups. Gene sets were filtered using the maximum and minimum gene set sizes of 500 and 15 genes, respectively. After performing 100 alignments, enriched gene sets were obtained based on a P value <0.05 and a false discovery rate (FDR) value of 0.25.
Random survival forest (RSF)
The gene selection process was executed using the randomForestSRC. We applied the RSF algorithm to prioritize the significance of prognosis-related genes (nrep=1000, which indicated a number of 1,000 iterations in the Monte Carlo simulation).
Statistical analysis
Survival curves were generated by the Kaplan-Meier method and subsequently compared using the log-rank test. The Cox proportional hazards model was employed to conduct multivariate analysis. All statistical analyses were performed in R (version 3.6). All statistical tests were two-sided, and P<0.05 was statistically significant.
Results
DEGs for bone metastasis in BRCA
We downloaded GSE2034, GSE124647, and GSE39494 BRCA-related databases from the GEO database and included expression profile data from 326 patients, with 241 having no metastasis and 85 having bone metastasis. Combat-seq was employed to mitigate for batch effects within databases (Figure 1A,1B). A total of 677 DEGs were identified using Limma (P<0.01), comprising 379 upregulated genes and 298 downregulated genes. Volcano plots revealed the expression patterns of these differentially expressed genes between bone metastasis and non-metastasis BRCA (Figure 1C).
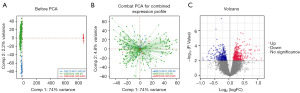
Construction of prognostic model for BRCA
Univariate Cox regression and LASSO regression were used to identify key DEGs in BRCA with bone metastasis based on clinical data from BRCA patients. Univariate Cox analysis (P<0.05) identified 77 genes significantly associated with bone metastasis BRCA. These genes are tabulated in Table 1. The functional enrichment analysis performed using Metascape revealed a significant enrichment of the DEGs in processes such as peptide metabolic process, regulation of B cell activation, intestinal immune network for IgA production (Figure 2A). Then protein-protein interaction (PPI) network was conducted using Cytoscape (Figure 2B).
Table 1
Gene expression level | Genes |
---|---|
Upregulated | CPT1A, RPL27, TM9SF3, TFF1, RPA3, GPN3, GGCX, AATK, CACYBP, SEC24A, BLVRA, KANSL2, SLC1A1, CRIP1, NDUFB1, GAPVD1, SNW1, SLC22A18, TOR1B, GSTO1, GSTK1, METTL17, ATP7B, SLC19A2, SLC30A5, WDR41, RACGAP1, ATG12 |
Downregulated | VDAC1, BRD4, ZFP36L2, TNFRSF17, NONO, GSTM4, IGLL5, AARS, SLC25A28, MED17, CIR1, UGP2, CCNC, RPL29, N4BP2L1, UBXN7, MED15, CD79A, GSTK1, TNFRSF13B, APC2, SOCS3, PRKG2, HLA-DMA, RDX, UBTF, MZB1, LCP1, TNFAIP2, CXCL13, OSBPL1A, APOBEC3C, FRS3, CD74, KRT5, HYAL2, MAP3K6, CD27, GUSBP11, SGPP1, RABIF, IL27RA, KRT15, WAC, HLA-J, PIGR, RASAL2, MPST, MTPAP |
DEGs, differentially expressed genes; BRCA, breast cancer.
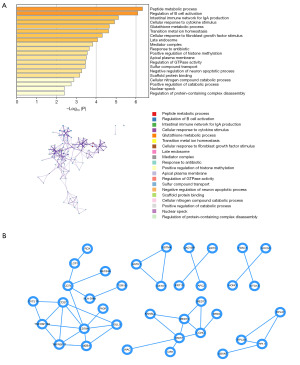
The partial likelihood deviance of validation included lower and upper standard deviations (SDs) as a function of log (lambda) for the dataset of the BRCA patients (Figure 3A). LASSO-Cox regression analysis revealed that the model built with 28 key DEGs performed better in both the training and test sets, and the OS was significantly lower in the high-risk group than in the low-risk group (Figure 3B). In LASSO regression analysis, a risk score for each patient was created by incorporating the expression values of each specific gene and weighting them with the corresponding estimated regression coefficients [risk score = SGPP1 × (−0.101225800921312) + CXCL13 × (−0.0990602304262338) + TFF1 × (−0.0761355688305833) + GSTM4 × (−0.0701245664074805) + OSBPL1A × (−0.0697959194240498) + SOCS3× (−0.0697408751094871) + SLC1A1 × (−0.0626539083827484) + SNW1 × (−0.0613959366359668) + UBTF × (−0.0532038393937342) + SLC19A2 × (−0.0522506821431928) + IGLL5 × (−0.050589292470364) + KRT15 × (−0.0275896594668161) + RPL3 × (−0.0221797643103188) + ATP7B × (−0.0191263079888773) + LCP1 × (−0.0182193631834509) + MZB1 × (−0.0180883496044173) + IL27RA × (−0.0111516113130218) + TNFAIP2 × (−0.0066362926318615) + CIR1 × (−0.00522630755396113) + PRKG2 × 0.00628130837936622 + GGCX × 0.00672477812884809 + UGP2 × 0.00901642206667127 + APC2 × 0.0502830638605627 + CPT1A × 0.0755668823957223 + VDAC1 × 0.0804574886170706 + RPA3 × 0.0929797695524507 + TOR1B × 0.125112100662822 + MED17 × 0.132621345321979] (Figure 3C). A prognostic score model for BRCA was developed based on the above 28 genes. The median risk score in the clinical cohort is firstly determined based on the model. Subsequently, enrolled patients are grouped into high-risk group (≥ the median risk score) and low-risk group (< the median risk score).
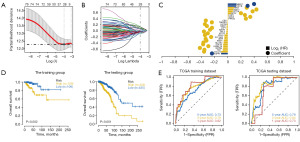
We randomly divided BRCA patients from TGCA database into training and testing sets in the ratio of 4:1. Patients were divided into high-risk and low-risk groups according to the median risk score (−0.0320772653171833, −0.00532802039832265, 0.0019647229505629 for TCGA training, TCGA testing, and GEO1 validating and GEO2 validating, respectively) and analyzed using Kaplan-Meier curves. OS was significantly lower in the high-risk group than in the low-risk group in both the training and testing sets (Figure 3D). The ROC curve showed that the area under the curve (AUC) values obtained in the training and testing sets for periods of 1, 3, and 5 years were greater than 0.70, indicating that the model had high training/testing accuracy (Figure 3E). Therefore, the model had strong predictive efficacy for the prognosis in BRCA patient.
Multi-omics analysis exploring clinical predictive value of the prognostic model for BRCA
The tumor microenvironment (TME), mainly composed of tumor-associated fibroblasts, immune cells, extracellular matrix, various growth factors, inflammatory factors, specific physicochemical characteristics, and cancer cells, significantly affects the diagnosis, survival outcome and clinical treatment sensitivity of tumors (19). We analyzed the relationship between risk score and tumor-infiltrating immune to further explore the molecular mechanisms of risk score affecting BRCA progression. Our result showed that risk score was significantly positively correlated with macrophages (M0, M1 and M2) and neutrophils; while was negatively correlated with dendritic cells, T cells, B cells, plasm cells, and monocytes (Figure 4A).
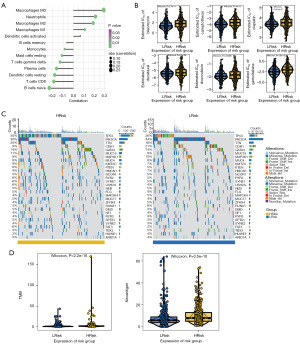
In clinical practice, for early-stage BRCA, surgery combined with chemotherapy is effective. Based on drug sensitivity data from the GDSC database and the comparison of the IC50 values to get a better comprehensive analysis of chemotherapy response, we predicted chemotherapy sensitivity for each tumor sample and explored the correlation with risk score and sensitivity of common chemotherapy drugs for BRCA patients. Pearson correlation between the risk score and IC50 of different chemical compounds was conducted, and six chemical compounds (bleomycin, camptothecin, cisplatin, docetaxel, doxorubicin, gemcitabine) were identified as having a negative correlation with the risk score, indicating that BRCA patients in high-risk group had a lower drug sensitivity (Figure 4B).
We further explored the mutation profiles of patients in the high- and low-risk groups, and the results showed that the proportion of mutations in genes such as TP53 was significantly higher in the high-risk group than in the low-risk group (Figure 4C). Further, the tumor mutational burden (TMB) and neoantigen in high-risk group were significantly higher than low risk groups (Figure 4D). Taken together, this model had a good clinical predictive value.
Robust analysis of the prognostic model for BRCA
The data used for validating the prognostic model were processed data of BRCA patients with survival data in the GEO database (GSE20685 and GSE12093). Based on the model, clinical staging of BRCA patients in the GEO database was predicted and survival differences between the high- and low-risk groups were assessed using Kaplan-Meier analysis. The OS of the high-risk group in two data sets was significantly lower than that of the low-risk group (Figure 5A). ROC curve analysis was performed to verify the predictive accuracy of the model. The results showed the AUC values of 1-, 3-, and 5-year in GSE20685 data set were not less than 0.70, and the AUC values of 1-, 3-, and 5-year in GSE12093 data set were not less than 0.65, indicating that the model had strong predictive efficacy for patient prognosis (Figure 5B).
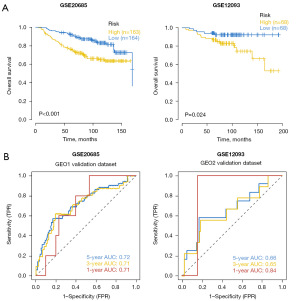
Analysis of independent prognostic factor
To analyze the independent prognostic factor for BRCA patients, we built the multivariable logistic regression prediction model based on integrating clinical information and the risk scores of patients in the high and low-risk groups and used the nomogram to visualize the model (Figure 6A). The total points were summated by adding each point of age, gender, stage, TMN classification, and risk score, respectively, and we found that the clinical indicators and risk score values all contributed to the total point. The calibration curves of 5- and 7-year survival demonstrated favorable prediction performance of nomogram (Figure 6B). Univariate Cox regression analysis and multivariate Cox regression analysis results demonstrated that age and risk score were the independent prognostic factors for BRCA patients (Figure 6C,6D).
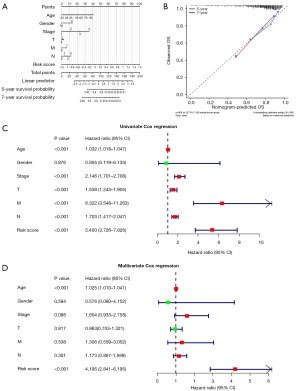
Relationship between risk score and clinical parameters
To further understand the relationship between the risk score and other clinical data, we grouped the risk score values of all samples by different clinical parameters, and found that the risk score was significantly associated with age (Figure 7A), fustat (Figure 7B), clinical-stage (Figure 7C) and the extent of the tumor (T, Figure 7D) (all P<0.001), but not with gender (Figure 7E) , the extent of spread to the lymph nodes (N, Figure 7F) classification and the presence of metastasis (M, Figure 7G) classification.
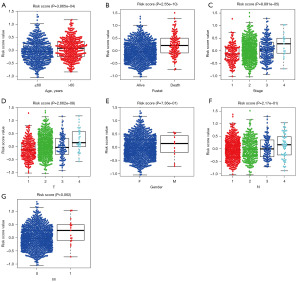
Identifying potential biological pathways associated with BRCA
We then used GSVA to reveal the pathways associated with the progression of BRCA based on the TGCA database. The data showed that signaling pathways converging at various biological processes were significantly different between low-risk and high-risk groups (Figure 8). The high-risk group was more likely to be related to KRAS signaling, IL2-STAT5 signaling, inflammatory response, Notch signaling, complement, hedgehog signaling, interferon-γ (IFN-γ) response, coagulation, EMT, interferon-a (IFN-a) response, angiogenesis, allograft rejection, hypoxia; while the low-risk was preferentially related to P13K-AKT-mTOR signaling, DNA repair, and Wnt signaling, indicating that disruption of these signaling pathways in BRCA patients would affect their prognosis.
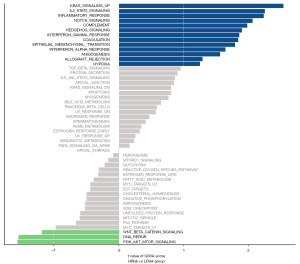
GSEA analysis was conducted to identify significant enrichment patterns using the TGCA database. GO pathway enrichment analysis revealed that the 677 DEGs were enriched in female meiotic nuclei division, meiotic I cell cycle, meiotic cell cycle, neuroinflammatory response, and regulation of chemotaxis (Figure 9A). KEGG pathway enrichment results revealed these genes were enriched in aminoacyl tRNA biosynthesis (ARS), cell cycle, cytokine-cytokine receptor interactions, hematopoietic cell lineage, and RNA degradation (Figure 9B).
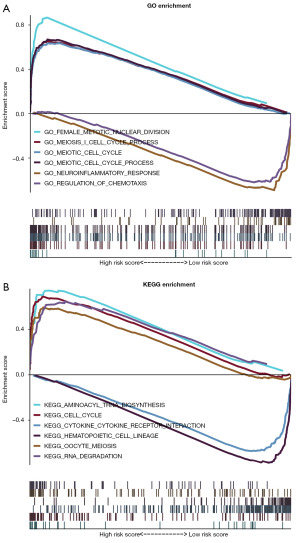
Predicting mRNA-miRNA-lncRNA interaction
miRNAs and lncRNAs play critical roles in the progression of BRCA (20-22). Therefore, we conducted a competitive endogenous RNA (ceRNA) network to visualize the mRNA-miRNA-lncRNA interaction. The miRWalk database and the ENCORI database were used to predict miRNA-binding sites and lincRNA-binding sites of these 28 genes. With the miRWalk database, 347 mRNA-miRNA pairs (accessibility <0.001 and TargetScan of 1 or miRDB of 1) were identified, including 21 mRNAs and 168 miRNAs. Next, these miRNAs were used to predict reciprocal lncRNAs using ENCORI database, and a total of 13,766 miRNA-lncRNA pairs were predicted (including 34 miRNAs and 4,040 lncRNAs). The CeRNA network was constructed by Cytoscape (v3.7) (Figure 10).
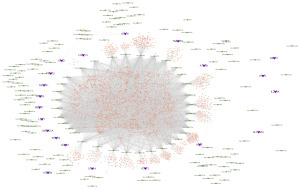
Identification of key genes via RSF analysis
Sensitivity analyses were conducted by the tree number in the RSF model and the derived variable importance ranking (Figure 11A). In our study, genes with relative importance greater than 0.8 were considered as the ultimate marker genes (Figure 11B). The key genes that were associated with prognosis of BRCA included voltage-dependent anion-selective channel 1 (VDAC-1), immunoglobulin lambda like polypeptide 5 (IGLL5), upstream binding transcription factor (UBTF), mediator complex subunit 17 (MED17), and marginal zone B and B1 cell specific protein (MZB1).
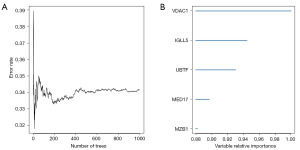
Discussion
BRCA is the most commonly occurring cancer in women and the second most common cancer overall (2). Even though women with non-metastatic invasive BRCA have a 5-year survival rate of up to 90%, women with distant metastatic invasive BRCA have a 5-year survival rate of only 30–40% (5,6). Most of the patients still have poor prognoses after metastasis. Bone is the most common site of metastasis for BRCA (7-9). A prognostic model based on multiple predictors can be used to predict the risks of a specific endpoint for individual patients. Many prognostic models for BRCA have been developed, but only a few have been extensively validated in various settings (23). Furthermore, their performance is suboptimal in independent populations, particularly in patients with high risk and in young and elderly patients (23). Therefore, we developed a prognostic score model for prognostic prediction in BRCA based on the genes associated with bone metastasis of BRCA and externally validated the reproducibility and generalizability of this prognostic model.
Based on the analysis of expression profile data of 326 patients from GSE2034, GSE124647, and GSE39494 BRCA-related databases, a total of 677 DEGs (379 upregulated genes and 298 downregulated genes) were preliminarily screened by Combat-seq correction, and 77 DEGs were identified by univariate Cox regression, with P value of <0.01 between BRCA with metastasis and without metastasis. A total of 28 of these genes (such as SGPP1, CXCL13, and TFF1) ultimately performed better in the LASSO Cox regression model for variable compression and were used to establish an optimal prognostic score model. A recent study showed a potential role of sphingosine-1-phosphate phosphatase 1 (SGPP1) in driving the metastasis of BRCAs into the bone microenvironment through the IL22R1-S1PR1 axis (24). Chemokine C-X-C motif ligand 13 (CXCL13) can target BRCA cells to promote proliferation, migration, and invasion (25,26). Furthermore, upregulation of trefoil factor-1 (TFF1) in estrogen-receptor (ER) positive BRCA is associated with a higher risk of bone metastasis (27). The risk score value for each sample by LASSO regression analysis based on these genes was obtained, and we divided these BRCA patients into two groups based on a median risk score. The high-risk group showed a significantly worse OS compared to the low-risk group. ROC curve analysis revealed that the model had high training/testing accuracy.
The clinical predictive value of the prognostic model for BRCA was further evaluated using multi-omics analysis. Although BRCA has been viewed as a relatively non-immunogenic cancer, the BRCA TME is rich in immune infiltrates with distinct functions and varies according to tumor subtypes (28,29). Recent advances in immuno-oncology have revealed prognostic and predictive values for tumor infiltrating lymphocytes (TILs) in BRCA, especially in patients with hormone receptor negative subtype. Higher tumor-infiltrating B cells, tumor-infiltrating T cells, and dendritic cells represent a favorable prognosis, while high tumor-associated macrophages (TAM) and neutrophil infiltration promote progression in BRCA (30-34). Similar to the above studies, after analysis of the relationship between risk score and tumor-infiltrating immune cells, we found that risk score was significantly positively correlated with TAM and neutrophils, and negatively correlated with B cells, T cells, and dendritic cells. Meanwhile, TME is of prime importance in clinical settings due to the development of drug resistance and recurrence of BRCA by inducing genes involved in self-renewal. Based on drug sensitivity data from the GDSC database, we found that high-risk group had a higher IC50 of Bleomycin, Camptothecin, Cisplatin, Docetaxel, Doxorubicin, and Gemcitabine, indicating that BRCA patients in high-risk group presented low sensitivity to these drugs. Moreover, TMB is associated with high neoantigen burden and helps predict responses to certain cancer immunotherapies. In addition, high TMB is associated with better response rates to immunotherapies in melanoma, non-small cell lung cancer (NSCLC) and urothelial cancer (35-38). In this study, we found that TMB and neoantigen in the high-risk group were significantly higher than in low-risk group, this may be because high TMB is more common in metastatic BRCA (39). All these data suggested that based on the predicting model, BRCA patients with high-risk score exhibited features of poor prognosis, indicating that this model had a good clinical predictive value. To validate the robustness of the prognostic model, we downloaded the processed data of BRCA patients with survival information. Based on this model, high-risk patients had a worse OS. Further, the ROC curve analysis revealed that this model had strong predictive efficacy for patient prognosis. Through multivariate Cox regression analysis, we were able to conclude that our prognostic model was efficient and independent of other clinical factors, such as age, gender, clinical stage, and TNM classification. The nomogram model incorporating these factors exhibited better discrimination ability for predicting prognosis in BRCA patients. Therefore, the model may provide a promising method for distinguishing the high-risk BRCA patients and help guide the stratified treatment of BRCA patients.
The prognostic biomarker pathways of BRCA were screened. The results of GSVA showed that different signal pathways were activated in different risk groups of BRCA. Being consistent with existing reports, our study indicates that KRAS signaling, IL2-STAT5 signaling, Notch signaling, and hedgehog signaling play important roles in BRCA metastasis (40-44). GSEA analysis showed significantly increased expression of genes in the high-risk group, mainly involving the meiotic nuclear division, meiosis I cell cycle, and meiotic cell cycle. The miRNAs and lncRNAs also play critical roles in the progression of BRCA through a variety of biological effects and mechanisms (20-22). Aco-expression network of the 28 genes-related mRNAs and lncRNAs was constructed in this study, and most nodes in the network were reported for the first time. Then, RSF revealed that the key genes that were associated with the prognosis of BRCA included VDAC-1, IGLL5, UBTF, MED17, and MZB1, with a relative importance >0.8. Among them, VDAC1, MED17, and MZB1 are unfavorable and associated with poor prognosis in BRCA, while IGLL5 was identified as the best predictor of relapse-free survival with >85% accuracy (45-49). Although experimental validation has not been conducted, the above results provide potential targets for future treatment of BCRA patients with bone metastasis.
Conclusions
In conclusion, 677 genes related to BRCA bone metastasis were screened by DEGs analysis based on public databases. Based on the univariate Cox regression and LASSO-Cox regression model analysis, we developed a prognostic model consisting of 28 prognostic DEGs, which can help distinguish the high-risk BRCA patients. The predictive efficacy of our prognostic model was further tested by external validation cohorts. Furthermore, the 28 signature genes-based predictive model and nomogram might be useful for prognostic prediction for BRCA patients. The prognostic prediction model of BRCA in this study can provide a promising method for clinical helping on clinical decision making and prognosis prediction for BRCA patients. Meanwhile, screening for prognostic biomarker genes and pathways in BRCA may provide potential therapeutic targets for future treatment.
Acknowledgments
Funding: This work was supported by
Footnote
Reporting Checklist: The authors have completed the TRIPOD reporting checklist. Available at https://tcr.amegroups.com/article/view/10.21037/tcr-23-1881/rc
Peer Review File: Available at https://tcr.amegroups.com/article/view/10.21037/tcr-23-1881/prf
Conflicts of Interest: All authors have completed the ICMJE uniform disclosure form (available at https://tcr.amegroups.com/article/view/10.21037/tcr-23-1881/coif). The authors have no conflicts of interest to declare.
Ethical Statement: The authors are accountable for all aspects of the work in ensuring that questions related to the accuracy or integrity of any part of the work are appropriately investigated and resolved. This study was conducted in accordance with the Declaration of Helsinki (as revised in 2013).
Open Access Statement: This is an Open Access article distributed in accordance with the Creative Commons Attribution-NonCommercial-NoDerivs 4.0 International License (CC BY-NC-ND 4.0), which permits the non-commercial replication and distribution of the article with the strict proviso that no changes or edits are made and the original work is properly cited (including links to both the formal publication through the relevant DOI and the license). See: https://creativecommons.org/licenses/by-nc-nd/4.0/.
References
- Wilkinson L, Gathani T. Understanding breast cancer as a global health concern. Br J Radiol 2022;95:20211033. [Crossref] [PubMed]
- Coleman MP, Quaresma M, Berrino F, et al. Cancer survival in five continents: a worldwide population-based study (CONCORD). Lancet Oncol 2008;9:730-56. [Crossref] [PubMed]
- Giaquinto AN, Sung H, Miller KD, et al. Breast Cancer Statistics, 2022. CA Cancer J Clin 2022;72:524-41. [Crossref] [PubMed]
- Turashvili G, Brogi E. Tumor Heterogeneity in Breast Cancer. Front Med (Lausanne) 2017;4:227. [Crossref] [PubMed]
- Breast Cancer: Statistics. 2022; Available online: https://www.cancer.net/cancer-types/breast-cancer/statistics
- Mariotto AB, Etzioni R, Hurlbert M, et al. Estimation of the Number of Women Living with Metastatic Breast Cancer in the United States. Cancer Epidemiol Biomarkers Prev 2017;26:809-15. [Crossref] [PubMed]
- Liang Y, Zhang H, Song X, et al. Metastatic heterogeneity of breast cancer: Molecular mechanism and potential therapeutic targets. Semin Cancer Biol 2020;60:14-27. [Crossref] [PubMed]
- Tahara RK, Brewer TM, Theriault RL, et al. Bone Metastasis of Breast Cancer. Adv Exp Med Biol 2019;1152:105-29. [Crossref] [PubMed]
- Chen MT, Sun HF, Zhao Y, et al. Comparison of patterns and prognosis among distant metastatic breast cancer patients by age groups: a SEER population-based analysis. Sci Rep 2017;7:9254. [Crossref] [PubMed]
- Coleman R, Body JJ, Aapro M, et al. Bone health in cancer patients: ESMO Clinical Practice Guidelines. Ann Oncol 2014;25:iii124-37. [Crossref] [PubMed]
- Tulotta C, Ottewell P. The role of IL-1B in breast cancer bone metastasis. Endocr Relat Cancer 2018;25:R421-34. [Crossref] [PubMed]
- Scimeca M, Trivigno D, Bonfiglio R, et al. Breast cancer metastasis to bone: From epithelial to mesenchymal transition to breast osteoblast-like cells. Semin Cancer Biol 2021;72:155-64. [Crossref] [PubMed]
- Ferrer A, Roser CT, El-Far MH, et al. Hypoxia-mediated changes in bone marrow microenvironment in breast cancer dormancy. Cancer Lett 2020;488:9-17. [Crossref] [PubMed]
- Huang R, Li Z, Zhang J, et al. Construction of Bone Metastasis-Specific Regulation Network Based on Prognostic Stemness-Related Signatures in Breast Invasive Carcinoma. Front Oncol 2021;10:613333. [Crossref] [PubMed]
- Ren Q, Khoo WH, Corr AP, et al. Gene expression predicts dormant metastatic breast cancer cell phenotype. Breast Cancer Res 2022;24:10. [Crossref] [PubMed]
- Lv X, He M, Zhao Y, et al. Identification of potential key genes and pathways predicting pathogenesis and prognosis for triple-negative breast cancer. Cancer Cell Int 2019;19:172. [Crossref] [PubMed]
- Pan Y, Liu G, Yuan Y, et al. Analysis of differential gene expression profile identifies novel biomarkers for breast cancer. Oncotarget 2017;8:114613-25. [Crossref] [PubMed]
- Vishnubalaji R, Sasidharan Nair V, Ouararhni K, et al. Integrated Transcriptome and Pathway Analyses Revealed Multiple Activated Pathways in Breast Cancer. Front Oncol 2019;9:910. [Crossref] [PubMed]
- Baghban R, Roshangar L, Jahanban-Esfahlan R, et al. Tumor microenvironment complexity and therapeutic implications at a glance. Cell Commun Signal 2020;18:59. [Crossref] [PubMed]
- Zhang M, Bai X, Zeng X, et al. circRNA-miRNA-mRNA in breast cancer. Clin Chim Acta 2021;523:120-30. [Crossref] [PubMed]
- Cao XH, Yang K, Liang MX, et al. Variation of Long Non-Coding RNA And mRNA Profiles in Breast Cancer Cells With Influences of Adipocytes. Front Oncol 2021;11:631551. [Crossref] [PubMed]
- Liu L, Zhang Y, Lu J. The roles of long noncoding RNAs in breast cancer metastasis. Cell Death Dis 2020;11:749. [Crossref] [PubMed]
- Phung MT, Tin Tin S, Elwood JM. Prognostic models for breast cancer: a systematic review. BMC Cancer 2019;19:230. [Crossref] [PubMed]
- Kim EY, Choi B, Kim JE, et al. Interleukin-22 Mediates the Chemotactic Migration of Breast Cancer Cells and Macrophage Infiltration of the Bone Microenvironment by Potentiating S1P/SIPR Signaling. Cells 2020;9:131. [Crossref] [PubMed]
- Kazanietz MG, Durando M, Cooke M. CXCL13 and Its Receptor CXCR5 in Cancer: Inflammation, Immune Response, and Beyond. Front Endocrinol (Lausanne) 2019;10:471. [Crossref] [PubMed]
- Panse J, Friedrichs K, Marx A, et al. Chemokine CXCL13 is overexpressed in the tumour tissue and in the peripheral blood of breast cancer patients. Br J Cancer 2008;99:930-8. [Crossref] [PubMed]
- Spadazzi C, Mercatali L, Esposito M, et al. Trefoil factor-1 upregulation in estrogen-receptor positive breast cancer correlates with an increased risk of bone metastasis. Bone 2021;144:115775. [Crossref] [PubMed]
- Burugu S, Asleh-Aburaya K, Nielsen TO. Immune infiltrates in the breast cancer microenvironment: detection, characterization and clinical implication. Breast Cancer 2017;24:3-15. [Crossref] [PubMed]
- Garcia-Recio S, Hinoue T, Wheeler GL, et al. Multiomics in primary and metastatic breast tumors from the AURORA US network finds microenvironment and epigenetic drivers of metastasis. Nat Cancer 2023;4:128-47. [PubMed]
- Qiu SQ, Waaijer SJH, Zwager MC, et al. Tumor-associated macrophages in breast cancer: Innocent bystander or important player? Cancer Treat Rev 2018;70:178-89. [Crossref] [PubMed]
- Wu L, Saxena S, Goel P, et al. Breast Cancer Cell-Neutrophil Interactions Enhance Neutrophil Survival and Pro-Tumorigenic Activities. Cancers (Basel) 2020;12:2884. [Crossref] [PubMed]
- Qin Y, Peng F, Ai L, et al. Tumor-infiltrating B cells as a favorable prognostic biomarker in breast cancer: a systematic review and meta-analysis. Cancer Cell Int 2021;21:310. [Crossref] [PubMed]
- Savas P, Virassamy B, Ye C, et al. Single-cell profiling of breast cancer T cells reveals a tissue-resident memory subset associated with improved prognosis. Nat Med 2018;24:986-93. [Crossref] [PubMed]
- Tian S, Yan L, Fu L, et al. A Comprehensive Investigation to Reveal the Relationship Between Plasmacytoid Dendritic Cells and Breast Cancer by Multiomics Data Analysis. Front Cell Dev Biol 2021;9:640476. [Crossref] [PubMed]
- Snyder A, Makarov V, Merghoub T, et al. Genetic basis for clinical response to CTLA-4 blockade in melanoma. N Engl J Med 2014;371:2189-99. [Crossref] [PubMed]
- Rizvi NA, Hellmann MD, Snyder A, et al. Cancer immunology. Mutational landscape determines sensitivity to PD-1 blockade in non-small cell lung cancer. Science 2015;348:124-8. [Crossref] [PubMed]
- Carbone DP, Reck M, Paz-Ares L, et al. First-Line Nivolumab in Stage IV or Recurrent Non-Small-Cell Lung Cancer. N Engl J Med 2017;376:2415-26. [Crossref] [PubMed]
- Rosenberg JE, Hoffman-Censits J, Powles T, et al. Atezolizumab in patients with locally advanced and metastatic urothelial carcinoma who have progressed following treatment with platinum-based chemotherapy: a single-arm, multicentre, phase 2 trial. Lancet 2016;387:1909-20. [Crossref] [PubMed]
- Barroso-Sousa R, Jain E, Cohen O, et al. Prevalence and mutational determinants of high tumor mutation burden in breast cancer. Ann Oncol 2020;31:387-94. [Crossref] [PubMed]
- Kumar A, Golani A, Kumar LD. EMT in breast cancer metastasis: an interplay of microRNAs, signaling pathways and circulating tumor cells. Front Biosci (Landmark Ed) 2020;25:979-1010. [Crossref] [PubMed]
- Kim RK, Suh Y, Yoo KC, et al. Activation of KRAS promotes the mesenchymal features of basal-type breast cancer. Exp Mol Med 2015;47:e137. [Crossref] [PubMed]
- Jesser EA, Brady NJ, Huggins DN, et al. STAT5 is activated in macrophages by breast cancer cell-derived factors and regulates macrophage function in the tumor microenvironment. Breast Cancer Res 2021;23:104. [Crossref] [PubMed]
- Krishna BM, Jana S, Singhal J, et al. Notch signaling in breast cancer: From pathway analysis to therapy. Cancer Lett 2019;461:123-31. [Crossref] [PubMed]
- Riobo-Del Galdo NA, Lara Montero Á, Wertheimer EV. Role of Hedgehog Signaling in Breast Cancer: Pathogenesis and Therapeutics. Cells 2019;8:375. [Crossref] [PubMed]
- Yang G, Zhou D, Li J, et al. VDAC1 is regulated by BRD4 and contributes to JQ1 resistance in breast cancer. Oncol Lett 2019;18:2340-7. [Crossref] [PubMed]
- Magrì A, Reina S, De Pinto V. VDAC1 as Pharmacological Target in Cancer and Neurodegeneration: Focus on Its Role in Apoptosis. Front Chem 2018;6:108. [Crossref] [PubMed]
- Zhang D, Yang S, Li Y, et al. Prediction of Overall Survival Among Female Patients With Breast Cancer Using a Prognostic Signature Based on 8 DNA Repair-Related Genes. JAMA Netw Open 2020;3:e2014622. [Crossref] [PubMed]
- Watanabe M, Shibata M, Inaishi T, et al. MZB1 expression indicates poor prognosis in estrogen receptor-positive breast cancer. Oncol Lett 2020;20:198. [Crossref] [PubMed]
- Ascierto ML, Kmieciak M, Idowu MO, et al. A signature of immune function genes associated with recurrence-free survival in breast cancer patients. Breast Cancer Res Treat 2012;131:871-80. [Crossref] [PubMed]