Expression of copper metabolism-related genes is associated with the tumor immune microenvironment and predicts the prognosis of hepatocellular carcinoma
Highlight box
Key findings
• Comprehensive analysis of copper metabolism-related genes (CMRGs) involved in hepatocellular carcinoma (HCC) progression and prognosis can guide clinical decision-making.
• CMRGs signature effectively classify patients with HCC into two distinct categories based on the degree of risk, emphasizing important biological pathways, immunological features, and checkpoint molecules.
What is known and what is new?
• Abnormal copper metabolism can trigger the development and progression of many tumors, and few studies have explored the impact of CMRGs on the prognosis of HCC patients.
• In this study, we first analyzed the CMRGs related to HCC prognosis, and established and validated a prognostic prediction model for HCC based on five gene signatures, and explored the connections between CMRGs and the tumor immune microenvironment.
What is the implication, and what should change now?
• Characterization based on CMRGs provides an effective approach for prognostic prediction and personalized treatment of HCC. Follow-up studies should further explore the potential therapeutic targets of the identified genes.
Introduction
Hepatocellular carcinoma (HCC) is a highly heterogeneous type of tumor (1). Current data show that it has a high incidence rate and a worldwide mortality rate of up to 8.3% (2). Early detection of cancer can improve the overall survival (OS) of patients, but most patients are already in the advanced stage when diagnosed (3), making HCC difficult to cure. The main treatment method for HCC is still surgical treatment, including liver transplantation (4,5) and liver resection (6,7). Despite the progress made in treatment methods, the prognostic effect for patients is still not ideal. Therefore, it is necessary to screen for reliable prognostic markers to improve the accuracy of cancer diagnosis and treatment effectiveness, as well as to improve patient prognosis.
Copper is an essential trace element in the human body that plays a crucial role in various biological processes, including cellular respiration and antioxidant defense (8). As a regulatory factor in mitochondria, copper is a key component in normal cellular energy metabolism (9), providing the high energy demands required for tumor growth. Copper homeostasis is fundamental to maintaining normal cell growth. However, when certain proteins misfold, aggregate, or malfunction, copper may accumulate excessively or be improperly transported, leading to oxidative stress and cellular toxicity (10,11). Such abnormal copper metabolism can disrupt copper homeostasis and has been implicated in various cancers, including HCC (12,13), colorectal cancer (14), breast cancer (15), and gastric cancer (16). Numerous reports have highlighted the significant role of copper in these cancers. Moreover, intact mitochondrial metabolism regulates the anti-tumor effect of the immune system (17). Disturbance of copper metabolism affects mitochondrial metabolism and leads to a reduction in the immune response of tumor cells. Therefore, the characteristics of copper metabolism-related genes (CMRGs) may serve as promising indicators for assessing the prognosis of HCC and for determining the effectiveness of immunotherapy.
The tumor immune microenvironment (TIME) has been shown to play a critical role in cancer development (18-20). The TIME is composed of stromal cells, immune cells, and the extracellular matrix (21). Disruption of the TIME can lead to abnormal cell metabolism, thereby promoting tumor proliferation and metastasis. Targeting the TIME has thus become a promising cancer treatment strategy in recent years (22). However, few studies have analyzed the interplay between copper metabolism and the TIME in the context of HCC progression, which serves as the background for the present study.
Analyzing the impact of CMRGs on the prognosis of HCC patients is of great value. Therefore, this study explores the close relationship between CMRG expression levels and HCC, as well as the TIME. We constructed a risk model based on the differential expression of five CMRGs using the The Cancer Genome Atlas (TCGA) database, and the results were further validated using the International Cancer Genome Consortium (ICGC). In summary, our study elucidates the role of CMRGs in HCC and develops an effective HCC prognostic model that can improve patient outcomes and guide immunotherapy. We present this article in accordance with the TRIPOD reporting checklist (available at https://tcr.amegroups.com/article/view/10.21037/tcr-23-1890/rc).
Methods
Data acquisition
RNA-sequencing (RNA-seq) data from 370 patients with HCC and 50 normal liver tissues were obtained from TCGA database (https://portal.gdc.cancer.gov/) for statistical analysis. Additionally, RNA-seq data and clinical information from 240 HCC patients were retrieved from the ICGC database to serve as a validation cohort. Samples without information on status, OS, and tumor-node-metastasis (TNM) staging were excluded. CMRGs were downloaded from the MsigDB. This study was conducted in accordance with the Declaration of Helsinki (as revised in 2013).
Differentially expressed CMRG
The ‘limma’ package was employed to identify differentially expressed CMRGs between HCC and normal tissues (23). The identification threshold was set to false discovery rate (FDR) of <0.05 and log2|fold change| of >1.5.
Identification of CMRG subgroups by consensus clustering
The single-factor Cox regression analysis identified 24 CMRGs that were associated with the prognosis of HCC, with a P value of less than 0.05 indicating statistical significance. ConsensusClusterPlus was employed to perform consensus clustering based on the expression levels of the 24 genes. The CIBERSORT algorithm (24) was used to calculate the abundance of immune cell infiltration of 22 immune cell types in the clustered subgroups.
Development and validation of a prognostic risk model for genes related to copper metabolism
The least absolute shrinkage and selection operator (LASSO) regression algorithm was utilized to screen previously filtered prognostic genes and eliminate overfitting with the ‘glmnet’ package. A multi-factor Cox regression was employed to determine the prognostic risk model for CMRGs. The risk score for each HCC sample was calculated using the following formula: risk score for each patient = β1 × Exp1 + β2 × Exp2 + β3 × Exp3 + β4 × Exp4 + β5 × Exp5, where Expi represents the expression of CMRGs in HCC, and βi represents their coefficients. Patients were classified into high-risk and low-risk groups based on this risk score. To determine the prognostic value of the model, we compared the difference in OS between the two risk groups using the Kaplan-Meier survival curve, and evaluated the efficacy of the established model using the time-dependent receiver operating characteristic (ROC) curve. Additionally, we associated the risk scores with conventional clinical features, such as gender, age, and clinical stage, and created a column chart to determine the effectiveness and accuracy of the model from multiple perspectives.
Risk group functional enrichment and tumor mutation load analysis
We performed gene set enrichment analysis (GSEA) (25) to identify potential molecular mechanisms or functional pathways between the high- and low-risk groups. GSEA was conducted on the Kyoto Encyclopedia of Genes and Genomes (KEGG) dataset c2.cp.kegg.v2023.1.Hs.symbols.gmt using the javaGSEA v.4.1.0 software. The threshold was set to normalized enrichment score (|NES|>1) and an FDR <0.05. In addition, we obtained the somatic mutation spectrum of HCC samples from the VarScan platform in the TCGA database and analyzed the differences in mutations between the different risk groups using the “Maftools” package in R.
Immuno-infiltration analysis
To explore the potential relationship between the CMRG-based prognostic model and immune cell infiltration in HCC, we used the online database Tumor Immune Estimation Resource (TIMER) (26) to estimate the infiltration levels of six immune cell types: B cells, macrophages, dendritic cells, CD4 T cells, CD8 T cells, and neutrophils, in the two risk groups. Pearson method was used to evaluate the correlation between the risk score and immune infiltration scores. Additionally, we analyzed the expression differences of key immune checkpoints between the two risk groups to explore the impact of CMRGs on the immune therapy response.
Statistical analysis
Statistical analysis was conducted using R (v.4.1.3) software and relevant packages. The chi-square test was used to analyze the differences in characteristics such as age and sex between the two groups. A P value <0.05 was considered statistically significant.
Results
Consensus clustering identified two copper metabolism-related differential gene subgroups in HCC
The expression of CMRGs in HCC samples and normal samples was first explored (Figure 1). The specific structure of this study is shown in Figure 1A. Using the limma algorithm for preliminary screening, we identified 5,642 differentially expressed genes (DEGs; 912 downregulated and 4,730 upregulated) in 370 tumor samples and 50 normal samples from the TCGA database (Figure 1C). Previous studies have reported that CMRGs are involved in the occurrence and development of HCC (27-29). Therefore, we explored whether any of the DEGs associated with OS (P<0.05) were related to CMRGs. A Venn diagram showed that 24 CMRGs were among the 3,038 DEGs generated by univariate Cox regression analysis (Figure 1B), and there is a difference in its expression between tumor and normal samples (Figure 1D). This suggests that CMRGs may have a role in the biological behavior of HCC. Through the consensus clustering algorithm, we divided HCC patients from the TCGA training cohort into subgroups based on the 24 CMRGs (Figure 2A). The subgroup with the highest clustering stability was found when K=2, with 113 patients assigned to cluster 1 and 257 patients assigned to cluster 2 (Figure 2B-2D). Significant differences were observed in the expression levels of CMRGs between the two subtypes, with patients in cluster 2 exhibiting better OS than those in cluster 1 (Figure 2E).
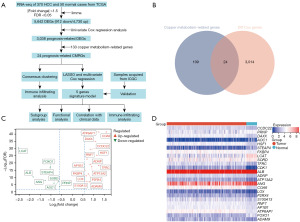
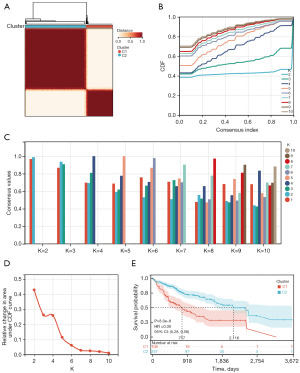
Copper metabolism genes are associated with immune cell infiltration
To explore the immune differences between the two subtypes, we used the CIBERSORT algorithm to calculate the degree of immune cell infiltration between them. The results showed that except for macrophages M0, naive and memory B cells, resting CD4 memory T cells, resting natural killer (NK) cells, monocytes, macrophages M1, macrophages M2, resting mast cells, and Neutrophils, all other immune cells had higher levels in cluster 2 (Figure 3). These results indicate significant differences in the tumor microenvironment and immune status between the two molecular subtypes, suggesting a close relationship between CMRGs and immune infiltration in HCC.
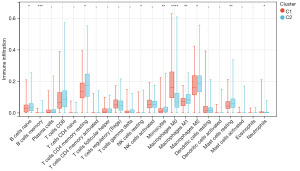
Risk scoring model based on genes related to copper metabolism has a good prognostic value
LASSO regression analysis was used to construct a risk feature model to evaluate the prognostic value of CMRGs in HCC (Figure 4). Five prognostic-related CMRGs [lecithin-cholesterol acyltransferase (LCAT), cyclin-dependent kinase 1 (CDK1), ATPase cation transporting 13A2 (ATP13A2), ring finger protein 7 (RNF7), and ADAM metallopeptidase domain 9 (ADAM9)] were identified (Figure 4A), the coefficients for each gene are shown in Figure 4C, and the risk score was calculated using the following formula: risk score = −0.0848 × Exp(LCAT) + 0.1050 × Exp(CDK1) + 0.0971 × Exp(ATP13A2) + 0.0018 × Exp(RNF7) + 0.0208 × Exp(ADAM9). Additionally, ROC analysis revealed that the constructed risk model exhibited precise predictive ability within 5 years, with the area under curve (AUC) values of 0.74, 0.72, and 0.71 for 1, 3, and 5 years, respectively (Figure 4B). The model successfully stratified TCGA patients into a high-risk group (n=183) and a low-risk group (n=182). The Kaplan-Meier curve indicated that the OS of the high-risk group was lower than that of the low-risk group (Figure 5A). A scatter plot demonstrated a significant difference in survival rates between the high-risk and low-risk groups, and the expression levels of the five genes were all associated with poor prognosis in HCC patients, and the heatmap clearly displayed significantly different expression levels of the five prognostic CMRGs in the two risk groups (Figure 5B). Figure 5C,5D show the predictive power of the ICGC-validated centralized risk model, and the five CMRG expressions, respectively.
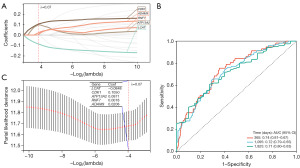
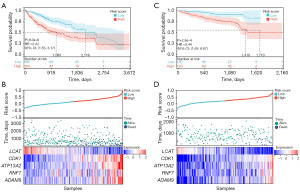
Risk scores were significantly correlated with clinical characteristics
We collected complete clinical information on patients in the TCGA cohort, including age, sex, clinical stage, and tumor grade. The results demonstrated a significant correlation between the risk score calculated by the model and age, T stage, grade, and stage, but not with sex (Figure 6A-6F). To explore the probability of the model predicting the survival rate of patients in the TCGA cohort, we established a nomogram (Figure 6G). The calibration curve revealed that the predicted results were consistent with the actual results (Figure 6H). These findings suggest that the risk model, which is based on CMRGs, is independent and plays a crucial role in predicting the clinical survival of HCC patients based on the calculated risk score.
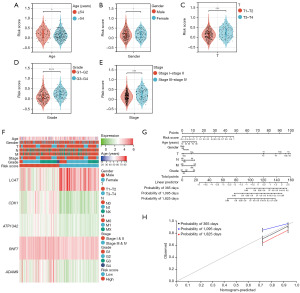
Differences in biological function between the two risk groups with tumor mutation burden (TMB)
We conducted GSEA on high-risk and low-risk groups to investigate the molecular mechanisms of HCC patients based on five CMRGs. Additionally, we analyzed the TMB of each patient in the TCGA cohort to calculate the difference in mutation spectrum between the two risk groups. KEGG enrichment analysis revealed that the high-risk group was mainly associated with DNA replication, cell cycle, and homologous recombination (Figure 7A-7C), while the low-risk group was primarily associated with multiple metabolic pathways (Figure 7D-7F). Notably, the gene mutation frequency in the high-risk group was higher than that in the low-risk group (Figure 7G), with TP53 being the most common mutation type, while TNN mutations were more frequent in the low-risk group. These findings provide distinct research directions for exploring the occurrence of HCC.
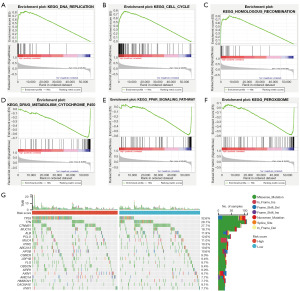
Significant difference in the level of immune infiltration between high- and low-risk groups
To assess the association between the risk model of CMRGs and the immune microenvironment of HCC, we used the TIMER algorithm to investigate the correlation between risk scores and levels of immune cell infiltration. The results showed that, except for a weak correlation with CD8 T cells, the risk score had a significant correlation with CD4 T cells, B cells, dendritic cells, macrophages, and neutrophils (Figure 8A-8F). Additionally, we observed differences in the expression levels of immune checkpoint genes between the high- and low-risk groups, with higher expression levels of PD-L1, CTLA4, HAVCR2, IDO1, and LAG3 in the high-risk group (Figure 9A-9E). Therefore, we conclude that the risk model based on the five CMRGs is strongly correlated with the TIME of HCC, and the CMRGs may act in synergy with immune features as potential prognostic characteristics of HCC.
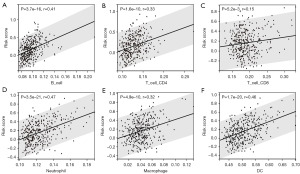
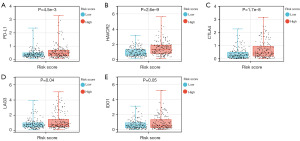
Effects of expression levels of five copper metabolism genes on the immune microenvironment of HCC
We analyzed the expression levels of the five CMRGs in HCC and their impact on prognosis. Consistent with previous studies, patients with LCAT overexpression and low expression of CDK1, ATP13A2, RNF7, and ADAM9 had a better prognosis (Figure 10A-10E). Finally, we explored the relationship between the expression levels of these five CMRGs and immune cell infiltration. It can be observed that LCAT was negatively correlated with seven types of immune cells in the TIME (P<0.01), whereas CDK1, ATP13A2, RNF7, and ADAM9 were positively correlated (P<0.01) and strongly correlated, which suggests that the five types of CMRGs play important roles in the TIME, and can be used in the future in terms of immunotherapeutic targets (Figure 10F).
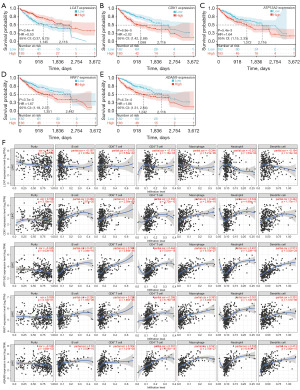
Discussion
Copper is an essential trace element for maintaining normal cellular function in living organisms. Disorders in copper metabolism can lead to abnormal cellular activity, increasing the incidence of cancer. Currently, research has also reported on therapeutic strategies for copper metabolism (30). This study aims to explore the important role of CMRGs in HCC.
In this study, we analyzed RNA-seq data of HCC from the TCGA database and identified prognosis-related copper metabolism genes based on DEGs. We found that CMRGs were differentially expressed in HCC tissues compared to normal tissues. Subsequently, we used consensus clustering to divide the differentially expressed copper metabolism genes into two subgroups and calculated the abundance of immune cell infiltration using the CIBERSORT algorithm. Our results showed that patients with poor prognosis had relatively lower immune cell infiltration status compared to those with better prognosis. In summary, our findings suggest that differentially expressed CMRGs are closely related to immune cell infiltration in HCC.
To further investigate the effect of CMRG disorders on the immune microenvironment of HCC, we constructed a prognostic risk model based on five CMRGs (LCAT, CDK1, ATP13A2, RNF7, and ADAM9) and validated it in the ICGC cohort, obtaining consistent results. LCAT has been previously associated with colorectal cancer (31) and epithelial ovarian cancer (32). Additionally, studies have reported that recombinant LCAT is a potential therapeutic strategy for patients with atherosclerosis (33-35). Consistent with previous reports, LCAT levels are lower in HCC patients (36), and insufficient LCAT expression may lead to decreased cellular cholesterol metabolism and increased risk of fatty liver. CDK1 is a critical kinase that promotes the process of mitosis and plays a vital role in cell growth. It binds to AKT and regulates its phosphorylation, thereby promoting the progression of advanced gastrointestinal stromal tumors (GISTs) (37). In liver cancer research, the CDK1 inhibitor RO1 can enhance the therapeutic effect of sorafenib by blocking the CDK1/PDK1/β-catenin signaling pathway (38). ATP13A2 is a lysosomal transporter protein whose insufficient expression disrupts mitochondrial function and induces toxicity and cell death (39). Additionally, ATP13A2 is significantly associated with HCC prognosis (40). RNF7 plays a positive regulatory role in the progression of multiple malignant tumors (41), and it is associated with the fibrotic progression of HCC (42). ADAM9, the fifth CMRG identified in the study, is a membrane-anchored protein whose overexpression is correlated with tumor invasiveness and poor prognosis (43). Modulating ADAM9 expression using sorafenib may be a promising therapeutic strategy for enhancing anti-tumor immunity in HCC patients (44). The analysis of the expression levels of the five genes in this study also confirmed a significant correlation between CMRGs and HCC patients’ prognosis and immune cell infiltration. In summary, the model established in this study has significant prognostic value, and the identified five CMRGs are key factors in cancer development.
Based on the risk score, HCC patients were divided into high- and low-risk groups, with significant differences in clinical characteristics between the two groups. To facilitate clinical application, we established a bar chart to provide personalized scoring. GSEA showed potential molecular functional differences between different risk groups. The difference in TMB between high and low-risk groups may be a direction for exploring HCC treatment targets. More importantly, there is a strong correlation between risk score and immune cell infiltration. Through analysis of the differential expression of multiple immune checkpoints, it was found that the low-risk group had significant expression of multiple immune checkpoints, which proves that the activation of TIME enables the low-risk group to exhibit a better prognosis and immune therapy response. The five CMRGs screened in this study can provide a reference for the diagnosis of HCC prognosis, and future research related to target therapy can be conducted. These results demonstrate that CMRGs can effectively guide immune therapy for HCC patients.
However, there are some potential limitations in this study. Firstly, the risk model was built based on public databases, which requires further validation with additional data. Secondly, it is necessary to further experimentally verify the specific roles of the identified CMRGs in HCC, as well as their impact on the efficacy of immune therapy for HCC.
Conclusions
We conducted a systematic analysis of the function and prognostic value of CMRGs in HCC. The risk model identified five CMEGs that are closely related to the immune microenvironment and show promising potential as prognostic markers. Our study reveals that CMRGs can serve as useful guides in predicting HCC prognosis and in developing effective immune therapy strategies.
Acknowledgments
Funding: This study was supported by
Footnote
Reporting Checklist: The authors have completed the TRIPOD reporting checklist. Available at https://tcr.amegroups.com/article/view/10.21037/tcr-23-1890/rc
Peer Review File: Available at https://tcr.amegroups.com/article/view/10.21037/tcr-23-1890/prf
Conflicts of Interest: All authors have completed the ICMJE uniform disclosure form (available at https://tcr.amegroups.com/article/view/10.21037/tcr-23-1890/coif). The authors have no conflicts of interest to declare.
Ethical Statement: The authors are accountable for all aspects of the work in ensuring that questions related to the accuracy or integrity of any part of the work are appropriately investigated and resolved. This study was conducted in accordance with the Declaration of Helsinki (as revised in 2013).
Open Access Statement: This is an Open Access article distributed in accordance with the Creative Commons Attribution-NonCommercial-NoDerivs 4.0 International License (CC BY-NC-ND 4.0), which permits the non-commercial replication and distribution of the article with the strict proviso that no changes or edits are made and the original work is properly cited (including links to both the formal publication through the relevant DOI and the license). See: https://creativecommons.org/licenses/by-nc-nd/4.0/.
References
- Wang YF, Yuan SX, Jiang H, et al. Spatial maps of hepatocellular carcinoma transcriptomes reveal spatial expression patterns in tumor immune microenvironment. Theranostics 2022;12:4163-80. [Crossref] [PubMed]
- Sung H, Ferlay J, Siegel RL, et al. Global Cancer Statistics 2020: GLOBOCAN Estimates of Incidence and Mortality Worldwide for 36 Cancers in 185 Countries. CA Cancer J Clin 2021;71:209-49. [Crossref] [PubMed]
- Wang W, Wei C. Advances in the early diagnosis of hepatocellular carcinoma. Genes Dis 2020;7:308-19. [Crossref] [PubMed]
- Mehta N, Bhangui P, Yao FY, et al. Liver Transplantation for Hepatocellular Carcinoma. Working Group Report from the ILTS Transplant Oncology Consensus Conference. Transplantation 2020;104:1136-42. [Crossref] [PubMed]
- Xu X. State of the art and perspectives in liver transplantation. Hepatobiliary Pancreat Dis Int 2023;22:1-3. [Crossref] [PubMed]
- Lin WD, Ye LN, Song ZS, et al. Wide surgical margins improve prognosis for HCC with microvascular invasion. Eur Rev Med Pharmacol Sci 2023;27:2052-9. [PubMed]
- Qian NS, Liao YH, Cai SW, et al. Comprehensive application of modern technologies in precise liver resection. Hepatobiliary Pancreat Dis Int 2013;12:244-50. [Crossref] [PubMed]
- Ge EJ, Bush AI, Casini A, et al. Connecting copper and cancer: from transition metal signalling to metalloplasia. Nat Rev Cancer 2022;22:102-13. [Crossref] [PubMed]
- Baker ZN, Cobine PA, Leary SC. The mitochondrion: a central architect of copper homeostasis. Metallomics 2017;9:1501-12. [Crossref] [PubMed]
- Scheiber I, Dringen R, Mercer JF. Copper: effects of deficiency and overload. Met Ions Life Sci 2013;13:359-87. [Crossref] [PubMed]
- Saporito-Magriñá CM, Musacco-Sebio RN, Andrieux G, et al. Copper-induced cell death and the protective role of glutathione: the implication of impaired protein folding rather than oxidative stress. Metallomics 2018;10:1743-54. [Crossref] [PubMed]
- Davis CI, Gu X, Kiefer RM, et al. Altered copper homeostasis underlies sensitivity of hepatocellular carcinoma to copper chelation. Metallomics 2020;12:1995-2008. [Crossref] [PubMed]
- Capriotti G, Piccardo A, Giovannelli E, et al. Targeting Copper in Cancer Imaging and Therapy: A New Theragnostic Agent. J Clin Med 2022;12:223. [Crossref] [PubMed]
- Gao W, Huang Z, Duan J, et al. Elesclomol induces copper-dependent ferroptosis in colorectal cancer cells via degradation of ATP7A. Mol Oncol 2021;15:3527-44. [Crossref] [PubMed]
- Blockhuys S, Wittung-Stafshede P. Roles of Copper-Binding Proteins in Breast Cancer. Int J Mol Sci 2017;18:871. [Crossref] [PubMed]
- Liu Y, Guan X, Wang M, et al. Disulfiram/Copper induces antitumor activity against gastric cancer via the ROS/MAPK and NPL4 pathways. Bioengineered 2022;13:6579-89. [Crossref] [PubMed]
- Porporato PE, Filigheddu N, Pedro JMB, et al. Mitochondrial metabolism and cancer. Cell Res 2018;28:265-80. [Crossref] [PubMed]
- Bagaev A, Kotlov N, Nomie K, et al. Conserved pan-cancer microenvironment subtypes predict response to immunotherapy. Cancer Cell 2021;39:845-865.e7. [Crossref] [PubMed]
- Zhu Y, Qin LX. Strategies for improving the efficacy of immunotherapy in hepatocellular carcinoma. Hepatobiliary Pancreat Dis Int 2022;21:420-9. [Crossref] [PubMed]
- Zhang Y, Li X, Li X, et al. Comprehensive analysis of cuproptosis-related long noncoding RNA immune infiltration and prediction of prognosis in patients with bladder cancer. Front Genet 2022;13:990326. [Crossref] [PubMed]
- Chen F, Zhuang X, Lin L, et al. New horizons in tumor microenvironment biology: challenges and opportunities. BMC Med 2015;13:45. [Crossref] [PubMed]
- Bejarano L, Jordāo MJC, Joyce JA. Therapeutic Targeting of the Tumor Microenvironment. Cancer Discov 2021;11:933-59. [Crossref] [PubMed]
- Ritchie ME, Phipson B, Wu D, et al. limma powers differential expression analyses for RNA-sequencing and microarray studies. Nucleic Acids Res 2015;43:e47. [Crossref] [PubMed]
- Newman AM, Liu CL, Green MR, et al. Robust enumeration of cell subsets from tissue expression profiles. Nat Methods 2015;12:453-7. [Crossref] [PubMed]
- Subramanian A, Tamayo P, Mootha VK, et al. Gene set enrichment analysis: a knowledge-based approach for interpreting genome-wide expression profiles. Proc Natl Acad Sci U S A 2005;102:15545-50. [Crossref] [PubMed]
- Li T, Fan J, Wang B, et al. TIMER: A Web Server for Comprehensive Analysis of Tumor-Infiltrating Immune Cells. Cancer Res 2017;77:e108-10. [Crossref] [PubMed]
- Antonucci L, Porcu C, Iannucci G, et al. Non-Alcoholic Fatty Liver Disease and Nutritional Implications: Special Focus on Copper. Nutrients 2017;9:1137. [Crossref] [PubMed]
- Yang M, Wu X, Hu J, et al. COMMD10 inhibits HIF1α/CP loop to enhance ferroptosis and radiosensitivity by disrupting Cu-Fe balance in hepatocellular carcinoma. J Hepatol 2022;76:1138-50. [Crossref] [PubMed]
- Wachsmann J, Peng F. Molecular imaging and therapy targeting copper metabolism in hepatocellular carcinoma. World J Gastroenterol 2016;22:221-31. [Crossref] [PubMed]
- Tsymbal S, Li G, Agadzhanian N, et al. Recent Advances in Copper-Based Organic Complexes and Nanoparticles for Tumor Theranostics. Molecules 2022;27:7066. [Crossref] [PubMed]
- Mihajlovic M, Gojkovic T, Vladimirov S, et al. Changes in lecithin: cholesterol acyltransferase, cholesteryl ester transfer protein and paraoxonase-1 activities in patients with colorectal cancer. Clin Biochem 2019;63:32-8. [Crossref] [PubMed]
- Russell MR, Graham C, D'Amato A, et al. Diagnosis of epithelial ovarian cancer using a combined protein biomarker panel. Br J Cancer 2019;121:483-9. [Crossref] [PubMed]
- Bonaca MP, George RT, Morrow DA, et al. Recombinant human lecithin-cholesterol acyltransferase in patients with atherosclerosis: phase 2a primary results and phase 2b design. Eur Heart J Cardiovasc Pharmacother 2022;8:243-52. [Crossref] [PubMed]
- Ossoli A, Simonelli S, Vitali C, et al. Role of LCAT in Atherosclerosis. J Atheroscler Thromb 2016;23:119-27. [Crossref] [PubMed]
- Lin X, Zhang W, Yang C, et al. Depleting LCAT Aggravates Atherosclerosis in LDLR-deficient Hamster with Reduced LDL-Cholesterol Level. J Adv Res. 2023; Epub ahead of print. [Crossref] [PubMed]
- Tahara D, Nakanishi T, Akazawa S, et al. Lecithin-cholesterol acyltransferase and lipid transfer protein activities in liver disease. Metabolism 1993;42:19-23. [Crossref] [PubMed]
- Lu X, Pang Y, Cao H, et al. Integrated Screens Identify CDK1 as a Therapeutic Target in Advanced Gastrointestinal Stromal Tumors. Cancer Res 2021;81:2481-94. [Crossref] [PubMed]
- Wu CX, Wang XQ, Chok SH, et al. Blocking CDK1/PDK1/β-Catenin signaling by CDK1 inhibitor RO3306 increased the efficacy of sorafenib treatment by targeting cancer stem cells in a preclinical model of hepatocellular carcinoma. Theranostics 2018;8:3737-50. [Crossref] [PubMed]
- Vrijsen S, Besora-Casals L, van Veen S, et al. ATP13A2-mediated endo-lysosomal polyamine export counters mitochondrial oxidative stress. Proc Natl Acad Sci U S A 2020;117:31198-207. [Crossref] [PubMed]
- Huang J, Xu S, Yu Z, et al. ATP13A2 is a Prognostic Biomarker and Correlates with Immune Infiltrates in Hepatocellular Carcinoma. J Gastrointest Surg 2023;27:56-66. [Crossref] [PubMed]
- Hua H, Xie H, Zheng J, et al. RNF7 Facilitated the Tumorigenesis of Pancreatic Cancer by Activating PI3K/Akt Signaling Pathway. Oxid Med Cell Longev 2023;2023:1728463. [Crossref] [PubMed]
- Matsuura K, Tanaka Y. Host genetic variants influencing the clinical course of hepatitis C virus infection. J Med Virol 2016;88:185-95. [Crossref] [PubMed]
- Chou CW, Huang YK, Kuo TT, et al. An Overview of ADAM9: Structure, Activation, and Regulation in Human Diseases. Int J Mol Sci 2020;21:7790. [Crossref] [PubMed]
- Kohga K, Takehara T, Tatsumi T, et al. Sorafenib inhibits the shedding of major histocompatibility complex class I-related chain A on hepatocellular carcinoma cells by down-regulating a disintegrin and metalloproteinase 9. Hepatology 2010;51:1264-73. [Crossref] [PubMed]