Propofol regulates the progression of hepatocellular carcinoma via the POLR2L/TGF-β signaling pathway
Highlight box
Key findings
• Propofol can regulate hepatocellular carcinoma (HCC) progression through the POLR2L/TGF-β signaling pathway.
What is known and what is new?
• Propofol had an effect on HCC tumor progression.
• Propofol downregulated POLR2L expression and inhibited cell proliferation and growth. As well as control the development of HCC by affecting the POLR2L/TGF-β signaling loop.
What is the implication, and what should change now?
• This study provided new insights into the relationship between isoproterenol and the development of HCC, which is regulated through the POLR2L/TGF-β signaling pathway. However, further systematic studies in the clinical setting are needed for future directions.
Introduction
Hepatocellular carcinoma (HCC) accounts for over 75% of primary liver malignancies, which rank as the sixth most frequent cancer worldwide (1,2). The limited therapeutic options for HCC can be attributed to challenges such as late-stage diagnosis, aggressive tumor progression, and the propensity for early postoperative recurrence specific to this malignancy (3). While a combination of standard treatments, including partial liver resection, chemotherapy, radiofrequency ablation, and molecularly targeted therapy, are routinely employed for HCC management, their efficacy diminishes considerably in the context of advanced-stage HCC (4,5). Moreover, post-resection recurrence is alarmingly high in HCC patients, with over 70% experiencing a recurrence. This significant rate of recurrence is a primary contributor to the bleak prognosis associated with HCC (6,7). Given the incomplete knowledge of the underlying processes of HCC that exist today, it is imperative to explore its molecular basis. Such research not only aids in the early identification of potential biomarkers but also paves the way for innovative therapeutic strategies for HCC patients.
Propofol (2,6-diisopropyl phenol) stands as a gold standard for intravenous anesthesia, predominantly used during surgical procedures and intensive care sedation (8). Its unique pharmacological profile and implications in various therapeutic scenarios have garnered substantial attention in medical research (9,10). Beyond its primary role as an anesthetic agent, propofol showcases several unique characteristics. While its anesthetic benefits, such as rapid onset and short duration of action, are well-recognized, propofol has also been linked to some non-anesthetic effects. Notably, its potential tumor-suppressive activity in multiple cancers has emerged in recent literature. This suggests that propofol might be a more favorable choice over other anesthetics, especially in the context of cancer surgery (11-13). On the molecular front, POLR2L, a subunit of the RNA polymerase II complex, has attracted attention in the context of tumorigenesis. This gene plays a pivotal role in RNA transcription and, consequently, in the regulation of numerous cellular processes (14).
Mutations or dysregulation of POLR2L have been implicated in a variety of malignancies, emphasizing its potential role as both a biomarker and therapeutic target (15). The current study focused on the connection between POLR2L and HCC, particularly in the presence of anesthetics like propofol. Given the high recurrence and metastasis rates of HCC, there is an urgent need for novel clinical treatments. Propofol, a well-known anesthetic with potential tumor-suppressive properties, presented an intriguing avenue for exploration. Additionally, POLR2L’s crucial role in RNA transcription and its link to tumors underscored the importance of further research. Therefore, the study aimed to clarify the relationship between propofol and POLR2L in the context of HCC, potentially uncovering new therapeutic avenues and prognostic markers. We present this article in accordance with the MDAR reporting checklist (available at https://tcr.amegroups.com/article/view/10.21037/tcr-23-2066/rc).
Methods
Downloading of GSE101724 dataset
The propofol-related GSE101724 dataset was downloaded from the Gene Expression Omnibus (GEO; http://www.ncbi.nlm.nih.gov/geo). The data included six groups of samples, four of which were human induced pluripotent stem cell (hiPSC) samples treated with different concentrations of propofol and were regarded as the case group. The other two groups were untreated hiPSC-like and were regarded as control groups. The subsequent analysis was meticulously conducted utilizing the GPL10558 platform, using the information taken from the Illumina HumanHT-12 V4.0 expression beadchip. The study was conducted in accordance with the Declaration of Helsinki (as revised in 2013).
Weighted gene co-expression network analysis (WGCNA) of GSE101724 dataset
To delve deeper into the complex gene interactions and identify significant modules related to propofol treatment in HCC, we employed the WGCNA methodology. This robust approach enables the detection of clusters (or modules) of highly correlated genes, facilitating the identification of potential molecular mechanisms and pathways pertinent to our study (16). For our analysis, the entire gene expression dataset from GSE101724, comprising both treated and untreated hiPSCs samples, was utilized. The dataset underwent necessary preprocessing to ensure quality, including normalization, transformation, and outlier detection. A soft-thresholding power that was chosen by the scale-free topology requirement was used to generate the adjacency matrix. To assess the network connection of genes, the adjacency matrix was later converted into a topological overlap matrix (TOM). Dissimilarity measure (1-TOM)-based hierarchical clustering, was then applied to identify modules of co-expressed genes. Finally, the key module was further identified based on the connectivity among the identified modules and GSE101724 dataset samples.
Acquisition of overlapping genes
Utilizing the GEO2R tool, we identified differentially expressed genes (DEGs) from the GSE121724 dataset. For upregulated genes, we decided to use a significance level of P<0.05 in conjunction with selection criteria of a fold change (FC) larger than 1.3. In contrast, downregulated DEGs were filtered based on an FC less than 0.77 and a significance level of P<0.05. Subsequently, we employed the “VennDiagram” package in the R software environment to perform an intersection analysis. This analytical step aimed to find the overlapping genes among the upregulated DEGs, downregulated DEGs, and genes from the turquoise module.
Identification of key overlapping genes from the TCGA-LIHC dataset and 14 overlapping genes
For a comprehensive understanding of the genetic alterations in liver hepatocellular carcinoma (LIHC), we sourced RNAseq data along with corresponding clinical information for 371 LIHC and 50 adjacent non-tumorous samples from The Cancer Genome Atlas (TCGA; https://tcga-data.nci.nih.gov/tcga) dataset, accessible via the Genomic Data Commons (GDC) portal (https://portal.gdc.cancer.gov). The primary aim was to discern DEGs within these samples, which would potentially offer insights into the molecular dynamics of HCC. The “limma” package in the R software environment was employed to identify these DEGs. To ensure rigorous selection, genes exhibiting an FC greater than 1.3 (upregulated DEGs) or less than 0.77 (downregulated DEGs) with a P value threshold of less than 0.05 were designated as differentially expressed. After this DEGs determination, an intersection analysis was performed between the DEGs derived from the TCGA-LIHC dataset and the previously identified overlapping genes from the GSE101724 dataset. This analytical step was pivotal in narrowing down to a set of key overlapping genes.
Construction and validation of prognostic nomogram in HCC
We conducted univariate and multivariate Cox regression analyses on eleven major overlapping genes and clinical characteristics [grade, pT-stage, pTNM (tumor, node, metastasis)-stage, and age]. Variables that could be used as nomograms were identified by univariate and multivariate analyses. If the gene and prognosis were significantly different in both univariate and multivariate factors, it can be demonstrated that the gene is an element unrelated to other clinical variables. Based on all of the independent prognostic factors assessed by the previously described research, the probability of 1-, 3-, and 5-year overall survival (OS) was predicted, and a composite nomogram was made using the “rms” package of the R programming language. We then used calibration curves to compare the expected results of the nomograms.
Analysis of the prognostic relevance and clinical relevance of POLR2L in LIHC
To elucidate the prognostic implications and clinical correlations of the POLR2L in LIHC, we embarked on a multifaceted analysis. Initially, the Kaplan-Meier plotter online platform (https://kmplot.com) was employed to investigate the differential expression of POLR2L and its potential impact on various survival outcomes in LIHC patients. Specifically, we focused on OS, relapse-free survival (RFS), and disease-specific survival (DSS). Relevant P values were calculated using the log-rank test to determine significance. Afterward, analyzing POLR2L expression in various clinical features of LIHC was done using the University of ALabama at Birmingham CANcer (UALCAN) database, including sample type, patient gender, patient age, tumor grade, lymph node metastasis status, and histological subtype. Finally, the expression of POLR2L in different samples of the GSE101724 dataset was also examined.
Cell culture
The cell bank of the Chinese Academy of Sciences contributed to the human HCC cell lines SNU-387, MHCC-97H, HCC-LM3, and normal cell LO2. The cells were then cultivated at 37 ℃ in Dulbecco’s modified Eagle’s medium (DMEM) with 5% CO2 and 10% fetal bovine serum (FBS) and 1% penicillin/streptomycin. For specific experiments, cells were treated with propofol sourced from Zeneca Pharmaceuticals, following the manufacturer’s recommended concentrations and protocols.
Quantitative reverse transcription-polymerase chain reaction (qRT-PCR) assay
Total RNA was isolated from cells using the Trizol reagent. mRNA was found using an ABI7500 real-time PCR machine and the One Step SYBR PrimeScript PLUS RT-RNA PCR Kit, as directed. The TransScript Green MiRNA Two-Step qRT-PCR SuperMix Kit was also utilized to measure the expression of TGF-β1 and POLR2L. The relative expression levels were examined using the 2−ΔΔCt method. The internal control employed was β-actin. Primers were as follows: POLR2L forward: 5'-TGCAGGCCGAGTACACCGAGG-3', POLR2L reverse: 5'-GGTTTCAGCGTGGTCACTTCTC-3'; TGF-β1 forward: 5'-GGCCAGATCCTGTCCAAzGC-3', TGF-β1 reverse: 5'-GTGGGTTTCCACCATTAGCAC-3'; β-actin forward: 5'-TGTTACCAACTGGGACGACA-3', β-actin reverse: 5'-GGGGTGTTGAAGGTCTCAAA-3'.
Cell transfection
GeneChem Co., Ltd. (Shanghai, China) designed and produced the small interfering RNA (siRNA) targeting POLR2L (si-POLR2L #1 and si-POLR2L #2) and a nontargeting control siRNA (si-NC). The night before transfection, cells were seeded in 6-well plates, and siRNA was transfected into them using Lipofectamine 2000 (Thermo Fisher Scientific, Shanghai, China). The siRNAs had the following sequences: si-POLR2L #1: 5'-GCUGUCUAGUCACGAGCUUTT-3'; si-POLR2L #2: 5'-GCAGUAUCCUGGUCGUGUUTT-3'; negative control (si-NC): 5'-UUCUCCGAACGUGUCACGUTT-3'.
Cell Counting Kit-8 (CCK-8) assay
The CCK-8 kit was used to measure the rates of proliferation of the SNU-387 and MHCC-97H cell lines. After adding 10 µL of CCK-8 reagent to each well containing 3.0×103 cells in 100 µL 96-well plates that had been treated with propofol or not, the cells were incubated at 37 ℃ for 2 hours. The experimental data was then collected on days 0, 1, 2, 3, and 4 after the optical density (OD) at 450 nm was found.
Western blotting (WB) assay
SNU-387 and MHCC-97H cells were gathered and extracted using lysis buffer (100 mM Tris-HCl, 2% SDS, 1 mM Mercaptoethanol, and 25% Glycerol). After being boiled in a loading buffer, a comparable volume of cell extracts was separated on 15% sodium dodecyl sulfate-polyacrylamide gel electrophoresis (SDS-PAGE) gels. Distinct protein bands were transferred into polyvinylidene fluoride (PVDF) membranes. Primary antibodies for TGF-β1, vimentin, N-cadherin and E-cadherin (1:1,000); p-Smad2, p-Smad3, Smad2 and Smad3 (1:500); and POLR2L (1:1,000) were used for Western blots (Abcam, Cambridge, MA, USA). Secondary antibodies that were coupled to horseradish peroxidase were added, diluted 1:10,000 and left to sit at room temperature for an hour. The membranes were washed three times with phosphate-buffered saline (PBS) before the immunoreactive bands were found using ECL-PLUS/Kit (GE Healthcare, Piscataway, NJ, USA).
Transwell assay
The invasion and migration abilities of SNU-387 and MHCC-97H cell lines were detected by Transwell assays using 24-well Transwell inserts. After resuspended in serum-free RPMI-1640 medium, 1×105 cells were seeded into the upper chamber of a 24-well plate. Ten percent FBS-supplemented RPMI-1640 medium was added to the bottom chamber. At 37 ℃ and 5% CO2, the cells were incubated for 16 hours before the residual cells were scraped out with cotton swabs. The cells that were migrating were subsequently preserved for half an hour in a 4% methanol solution. Notably, Matrigel was required on the upper chamber for the cell invasion assay. Images were taken under a light microscope after 30-min staining with 4',6-diamidino-2-phenylindole (DAPI) at room temperature.
Flow cytometry analysis
Flow cytometry was used to evaluate apoptosis and the distribution of the cell cycle. Following two cold PBS washes, cells were taken when they were 70–80% confluent, and they were then frozen in 70% ethanol at −20 ℃ for an overnight period. After that, cells were exposed to RNase A and stained with propidium iodide (PI) for half an hour at room temperature in the dark in preparation for cell cycle analysis. After that, a flow cytometer was used to examine the DNA content. Cells were stained by the manufacturer’s instructions for apoptosis analysis using the Annexin V-fluorescein isothiocyanate (FITC)/PI Apoptosis Detection Kit. In summary, cells were resuspended in a binding buffer and then incubated for 15 minutes at room temperature in the dark with Annexin V-FITC and PI. Using a flow cytometer, the cell cycle distribution and apoptotic rate were measured, and the related software was used for analysis. Every experiment was conducted thrice, and the results were displayed as the mean ± standard deviation (SD).
Colony formation assay
Five hundred cells per well were used to seed 6-well plates, and the cells were left to attach for a full day. After that, they received 14 days of treatment at 37 ℃ with varying propofol doses. Following the incubation period, cells were stained with Alkali nitro tetrazolium blue chloride and preserved with 4% paraformaldehyde. Colonies containing over 50 cells were tallied (observed under a magnification factor of ×10), and the ability to proliferate following propofol therapy was contrasted with that of untreated controls.
Statistical analysis
The means ± SDs were used to show all the data. Student’s t-test was used to assess differences between the two groups, and one-way analysis of variance (ANOVA) and Tukey’s post-hoc test were employed to compare data across numerous groups. The Chi-squared test was used to compare categorical data. The Pearson correlation coefficient was used to measure how closely two variables were correlated. The Kaplan-Meier method was used to plot survival curves, and the Log-rank test was used to compare them. All in vitro experiments were repeated three times. The program SPSS 25.0 (IBM Corp., Armonk, NY, USA) was used for all statistical analyses. A statistically significant P value was defined as one less than 0.05.
Results
Identification of overlapping genes in the turquoise module and GSE101724-DEGs
After analysis of the propofol-associated GSE101724 dataset, a gene co-expression network was established. It was found that β =18 was the ideal soft-thresholding power, where the curve first reached an R2 value of 0.85 (Figure 1A). The sample dendrogram, combined with the trait heatmap, provided insights into the clustering of samples and their interrelationships, which are illustrated in Figure 1B. Using the dynamic tree-cutting approach, distinct modules were identified, and the inter-modular relationships were subsequently analyzed (Figure 1C,1D). The module-trait relationship heatmap revealed that the turquoise module exhibited the strongest association with the case group, as depicted in Figure 1E. Consequently, the essential module was identified as the turquoise module. Gene expression clustering heatmap of genes in propofol samples and control samples (Figure 1F). From the GSE101724 dataset, 19 upregulated DEGs and 16 downregulated DEGs were filtered out. Intersection analysis identified 14 overlapping genes from the downregulated DEGs and the turquoise module, as shown in Figure 1G.
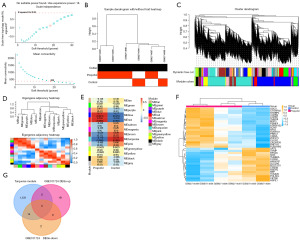
Screening of key prognostic genes in TCGA-LIHC samples
From the LIHC samples in the TCGA dataset, we identified 9,078 upregulated DEGs and 1,084 downregulated DEGs (Figure 2A). As shown in Figure 2B, intersection analysis of these DEGs with 14 overlapping genes revealed 11 key overlapping genes. These 11 genes were subjected to univariate and multivariate Cox proportional hazards regression analyses. Notably, only HSPE1 and POLR2L showed significant prognostic significance (Figure 2C,2D). The predictive significance of these two genes was then assessed by creating a nomogram. The findings demonstrated the strong prognostic predictive significance of HSPE1 and POLR2L for the 1-, 3-, and 5-year survival rates of the patients, indicating that these two genes have potential prognostic relevance in LIHC (Figure 2E,2F).
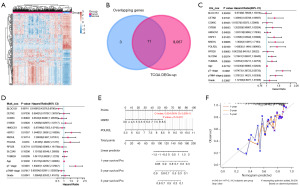
Impact of POLR2L differential expression on the prognostic in LIHC patients
We investigated the influence of POLR2L differential expression on various survival rates in LIHC patients. POLR2L is an important prognostic gene for LIHC, as demonstrated by our results, which showed that increased expression of POLR2L was linked to a general deterioration in OS, RFS, and DSS (Figure 3A-3C). Further validation using the UALCAN database disclosed significant discrepancies in POLR2L expression across different clinical features of LIHC samples (Figure 3D-3I). When compared to normal liver tissues, primary tumor liver samples exhibited markedly increased levels of POLR2L. However, no discernible differences in POLR2L expression were observed between male and female patients. Additionally, POLR2L expression was found to correlate with patient age, tumor grading, and histological subtypes. No significant variation in POLR2L expression was noted concerning nodal metastasis status.
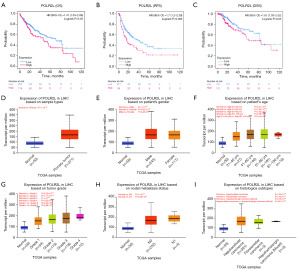
Knockdown of POLR2L inhibits proliferation, invasion and migration of HCC cell lines
We evaluated POLR2L levels in HCC cell lines compared to normal cells, observing a significant elevation in HCC cell lines (Figure 4A). For knockdown experiments, we employed si-POLR2L #1 and si-POLR2L #2. Notably, si-POLR2L #1 demonstrated superior knockdown efficiency (Figure 4B). SNU-387 and MHCC-97H cell proliferation, invasion, and migration were significantly reduced by POLR2L knockdown, according to further research employing CCK-8 and Transwell assays (Figure 4C-4I).
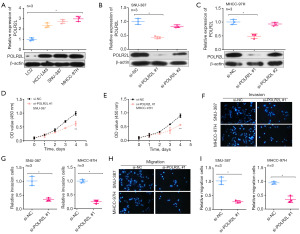
POLR2L knockdown can promote the apoptosis of HCC cell line and block the cell cycle in G1 phase
Through flow cytometry, we were able to identify notably higher levels of apoptosis in POLR2L knockdown liver cancer cell lines as compared to the control group (Figure 5A,5B). At the same time, Bax and caspase-3 mRNA expression levels were found to be considerably higher by qRT-PCR, but Bcl-2 mRNA expression was found to be significantly lower (Figure 5C,5D). Protein levels and mRNA trends were consistent (Figure 5E). Compared to the group under control, we detected by flow cytometry that POLR2L knockdown HCC cell lines had cell cycle arrest in G1 phase (Figure 5F-5I).
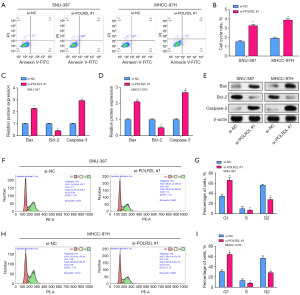
Propofol can regulate cell apoptosis and proliferation by down-regulating POLR2L expression
POLR2L was downregulated in the case group, according to the analysis of the GSE101724 dataset (Figure 6A). The expression level of POLR2L in SNU-387 and MHCC-97H cells was significantly decreased after propofol treatment (Figure 6B,6C). Subsequent CCK-8 assays revealed that propofol considerably inhibited cell proliferation (Figure 6D,6E) and suppressed cell growth (Figure 6F-6I) respectively. Meanwhile, in propofol treated SNU-387 and MHCC-97H HCC cell lines, POLR2L knockdown resulted in increased apoptosis (Figure 6J,6K).
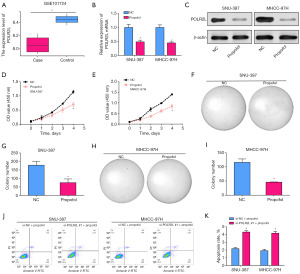
Propofol regulates HCC progression by POLR2L/TGF-β signaling pathway
Epithelial-mesenchymal transition (EMT)-related protein expression was seen in SNU-387 and MHCC-97H cell lines following POLR2L knockdown. The WB test findings indicated that there was a down-regulation of N-cadherin and vimentin expression, as well as a down-regulation of E-cadherin expression (Figure 7A). Simultaneously, WB demonstrated that TGF-β1 expression was downregulated, Smad2 and Smad3 expression remained unchanged, but phosphorylated p-Smad2 and p-Smad3 expression levels were markedly reduced (Figure 7B). Following the knockdown of POLR2L and the addition of propofol, the expression of TGF-β1 was further decreased in HCC cell lines (Figure 7C-7E).
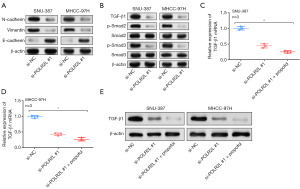
Discussion
HCC frequently coexists with chronic liver conditions like cirrhosis, which can exacerbate the progression of HCC. This concomitance underscores the complex nature of HCC’s pathogenesis and highlights the importance of early intervention and comprehensive management of underlying liver conditions to mitigate the severity of HCC (17,18). Currently, the intricate mechanisms underpinning HCC are not fully understood. This knowledge gap underscores the critical need to elucidate its molecular underpinnings to enhance diagnostic accuracy, devise preventive measures, and develop targeted therapeutic interventions (19). Anesthesia is an integral part of surgical interventions for HCC. Among various agents, propofol stands out as a widely used intravenous anesthetic. Intriguingly, emerging evidence suggests that propofol possesses potential anti-cancer properties, adding another dimension to its clinical significance (20,21).
Recent research by Siddiqui et al. had unveiled another promising facet of propofol. Their investigation revealed that propofol can curb the proliferation of breast cancer cells (22). This discovery opened up intriguing avenues of exploration, especially considering the dual role of propofol as an anesthetic and a potential anti-cancer agent. Such findings emphasized the need for comprehensive studies to determine the mechanistic insights behind propofol’s anti-cancer effects, not only in breast cancer but also in other malignancies like HCC, as discussed earlier. Zhang et al.’s findings further bolstered the potential therapeutic attributes of propofol in the context of cancer treatment (23). Their study illustrated that propofol is not limited to its anesthetic effects; it also actively promotes apoptosis in liver cancer cells. Apoptosis is a natural cellular process that eliminates damaged cells, and its induction in cancer cells could serve as a valuable therapeutic strategy. Furthermore, Zhang et al. noted that propofol had a specific inhibitory impact on HCC cell metastasis, an important finding as metastasis was the primary cause of cancer-related mortality (23). In light of these discoveries, there was mounting proof that propofol functions as more than merely an anesthetic. Its role in hindering cell growth, promoting apoptosis, and inhibiting metastasis makes it an attractive subject for in-depth study, which may lead to innovative ways to treat cancer. Research by Miao et al. had demonstrated how propofol inhibits colon cancer cells’ ability to invade (24). The aforementioned findings underscored the potential efficacy of propofol as an anesthetic in oncological surgeries. Building upon this premise, the present study leveraged both bioinformatics analysis and functional assays to elucidated the intricate relationship between propofol and HCC.
In this study, by analyzing the propofol-related GSE101724 dataset and TCGA-LIHC samples using bioinformatics, we identified two pivotal prognostic genes, HSPE1 and POLR2L, with the latter being highlighted as the central prognostic gene for this investigation. POLR2L encoded a component of RNA polymerase II, which is responsible for messenger RNA synthesis in eukaryotes, and its product boasts a distinct zinc-binding domain along with four conserved cysteines (25,26). Previous findings by Yao et al. had elucidated that elevated levels of POLR2L were associated with advanced tumor grades and late metastatic stages1 (27). Moreover, research by Zhou et al. substantiated that POLR2L is an upregulated gene in drug-resistant gastric cancer and it interacts with miR-604, pointing towards its potential role in the resistance mechanism and pathogenesis of gastric cancer (28). Complementing this, Yao et al.’s literature also showed that POLR2L was one of the phosphorylation-related genes (PRGs) and protein kinases that have been found to express highly in HCC cancer stem cells (27). This gene played a key role in cell cycle-related pathways that drive the evolution of HCC and was closely associated with a poor prognosis in patients with HCC. In our exploration, a heightened expression of POLR2L translated to an adverse prognosis in HCC, with this gene exhibiting significant differential expression across varied clinical-pathological stages of LIHC. Furthermore, our in vitro experiments revealed that knocking down the expression of POLR2L considerably impeded cell growth, migration, and invasion in HCC. Collectively, these findings underscored the paramount importance of POLR2L in the tumorigenesis and progression of HCC, suggesting its potential as a therapeutic target for this malignancy.
In our investigation, it was evident that propofol modulates both cell apoptosis and proliferation by down-regulating POLR2L expression in HCC. The GSE101724 dataset and our experiments on SNU-387 and MHCC-97H cell lines collectively corroborated this regulatory effect of propofol on POLR2L. A crucial biological mechanism essential to the development of many malignancies is the EMT (29,30). Because EMT gave epithelial cells the ability to become migratory and invasive, it was essential for the spread of cancer (31). Upon delving into the EMT process in our study, following the knockdown of POLR2L, a change in the expression of signature EMT proteins was noticed. Interestingly, N-cadherin and vimentin expressions were downregulated, while E-cadherin expression was attenuated. These findings signified the suppressive role of POLR2L on EMT, reinforcing its influence on HCC progression. Furthermore, one of the most important mechanisms in the development of tumors and the advancement of cancer was the TGF-β signaling system (32). This pathway intricately regulated cell growth, differentiation, and apoptosis. Within this signaling cascade, TGF-β1, together with Smad2 and Smad3, held a paramount position. Their synergistic interactions enabled a cascade of cellular responses critical for cancer development (33). In our experimental paradigm, upon POLR2L knockdown, a significant reduction in TGF-β1 expression was observed. Interestingly, while the levels of Smad2 and Smad3 remained constant, their phosphorylated counterparts, p-Smad2 and p-Smad3, witnessed a substantial reduction, implying the suppressed activation of this signaling pathway. In HCC cell lines, the combined impact of POLR2L knockdown and propofol therapy further decreased the production of TGF-β1. In summary, our research outlined the complex function of POLR2L in the development of HCC and how propofol regulated it. The intertwined relationships between POLR2L, EMT processes, and the TGF-β signaling pathway underscored potential therapeutic avenues for HCC intervention.
Conclusions
In conclusion, by the GSE101724 dataset and public datasets, the key gene POLR2L is proven to be involved in propofol mechanism in HCC, and it could be a useful prognostic biomarker in HCC patients. A poor prognosis is associated with increased expression of the proto-oncogene POLR2L, which is seen in HCC. More importantly, we demonstrated that propofol treatment can inhibit POLR2L expression and the expression of TGF-β1, thus it is concluded that propofol regulates HCC progression via POLR2L/TGF-β signaling pathway. The study offers fresh insights into the connection between propofol and the development of HCC as well as novel therapeutic approaches for the management and prognosis of HCC.
Acknowledgments
We were indebted to the investigators who participated in the conceptualization, design, and implementation of this study. Throughout the study, we sincerely thank the Department of Anesthesiology, Oriental Hepatobiliary Surgery Hospital, The Third Affiliated Hospital of Naval Military Medical University, Shanghai, China, and the Department of Anesthesiology, Shanghai First Women’s and Infants’ Hospital, School of Medicine, Tongji University, as well as the School of Life Sciences, Fudan University, Shanghai, China, for providing the necessary facilities and support.
Funding: None.
Footnote
Reporting Checklist: The authors have completed the MDAR reporting checklist. Available at https://tcr.amegroups.com/article/view/10.21037/tcr-23-2066/rc
Data Sharing Statement: Available at https://tcr.amegroups.com/article/view/10.21037/tcr-23-2066/dss
Peer Review File: Available at https://tcr.amegroups.com/article/view/10.21037/tcr-23-2066/prf
Conflicts of Interest: All authors have completed the ICMJE uniform disclosure form (available at https://tcr.amegroups.com/article/view/10.21037/tcr-23-2066/coif). The authors have no conflicts of interest to declare.
Ethical Statement: The authors are accountable for all aspects of the work in ensuring that questions related to the accuracy or integrity of any part of the work are appropriately investigated and resolved. The study was conducted in accordance with the Declaration of Helsinki (as revised in 2013).
Open Access Statement: This is an Open Access article distributed in accordance with the Creative Commons Attribution-NonCommercial-NoDerivs 4.0 International License (CC BY-NC-ND 4.0), which permits the non-commercial replication and distribution of the article with the strict proviso that no changes or edits are made and the original work is properly cited (including links to both the formal publication through the relevant DOI and the license). See: https://creativecommons.org/licenses/by-nc-nd/4.0/.
References
- Desai A, Sandhu S, Lai JP, et al. Hepatocellular carcinoma in non-cirrhotic liver: A comprehensive review. World J Hepatol 2019;11:1-18. [Crossref] [PubMed]
- Li Y, Li P, Wang N. Effect of let-7c on the PI3K/Akt/FoxO signaling pathway in hepatocellular carcinoma. Oncol Lett 2021;21:96. [Crossref] [PubMed]
- Zhu R, Guo W, Xu XJ, et al. An Integrating Immune-Related Signature to Improve Prognosis of Hepatocellular Carcinoma. Comput Math Methods Med 2020;2020:8872329. [Crossref] [PubMed]
- Xu XB, Zhang HY, Liu CL, et al. Liver transplantation for recurrence of primary liver carcinoma after resection and therapy of recurrent hepatocellular carcinoma at the liver graft(a long-term surviving case report and related literature review). Aviation Medicine of Air Force 2010;26:70-5.
- Feng GS, Hanley KL, Liang Y, et al. Improving the Efficacy of Liver Cancer Immunotherapy: The Power of Combined Preclinical and Clinical Studies. Hepatology 2021;73:104-14. [Crossref] [PubMed]
- Yamashita R, Long J, Saleem A, et al. Deep learning predicts postsurgical recurrence of hepatocellular carcinoma from digital histopathologic images. Sci Rep 2021;11:2047. [Crossref] [PubMed]
- Zheng L, Gu X, Zheng G, et al. Prediction of early recurrence and response to adjuvant Sorafenib for hepatocellular carcinoma after resection. PeerJ 2021;9:e12554. [Crossref] [PubMed]
- Nyssen P, Maho A, Malempré R, et al. Propofol inhibits the myeloperoxidase activity by acting as substrate through a redox process. Biochim Biophys Acta Gen Subj 2022;1866:130100. [Crossref] [PubMed]
- Mammoto T, Mukai M, Mammoto A, et al. Intravenous anesthetic, propofol inhibits invasion of cancer cells. Cancer Lett 2002;184:165-70. [Crossref] [PubMed]
- Kotani Y, Shimazawa M, Yoshimura S, et al. The experimental and clinical pharmacology of propofol, an anesthetic agent with neuroprotective properties. CNS Neurosci Ther 2008;14:95-106. [Crossref] [PubMed]
- Wang J, Cheng CS, Lu Y, et al. Novel Findings of Anti-cancer Property of Propofol. Anticancer Agents Med Chem 2018;18:156-65. [Crossref] [PubMed]
- Sun H, Wang Y, Zhang W. Propofol inhibits proliferation and metastasis by up-regulation of miR-495 in JEG-3 choriocarcinoma cells. Artif Cells Nanomed Biotechnol 2019;47:1738-45. [Crossref] [PubMed]
- Xu K, Tao W, Su Z. Propofol prevents IL-13-induced epithelial-mesenchymal transition in human colorectal cancer cells. Cell Biol Int 2018;42:985-93. [Crossref] [PubMed]
- Liu Y, Jia W, Li J, et al. Identification of Survival-Associated Alternative Splicing Signatures in Lung Squamous Cell Carcinoma. Front Oncol 2020;10:587343. [Crossref] [PubMed]
- Muste Sadurni M, Saponaro M. Deregulations of RNA Pol II Subunits in Cancer. Appl Biosci 2023;2:459-76. [Crossref]
- Liu Z, Ma C, Gu J, et al. Potential biomarkers of acute myocardial infarction based on weighted gene co-expression network analysis. Biomed Eng Online 2019;18:9. [Crossref] [PubMed]
- Dong Y, Cai Q, Fu L, et al. Study of the G Protein Nucleolar 2 Value in Liver Hepatocellular Carcinoma Treatment and Prognosis. Biomed Res Int 2021;2021:4873678. [Crossref] [PubMed]
- Fu Q, Yang F, Xiang T, et al. A novel microRNA signature predicts survival in liver hepatocellular carcinoma after hepatectomy. Sci Rep 2018;8:7933. [Crossref] [PubMed]
- Shi L, Shang X, Nie K, et al. Identification of potential crucial genes associated with the pathogenesis and prognosis of liver hepatocellular carcinoma. J Clin Pathol 2021;74:504-12. [Crossref] [PubMed]
- Li Y, Dong W, Yang H, et al. Propofol suppresses proliferation and metastasis of colorectal cancer cells by regulating miR-124-3p.1/AKT3. Biotechnol Lett 2020;42:493-504. [Crossref] [PubMed]
- Xu Y, Pan S, Jiang W, et al. Effects of propofol on the development of cancer in humans. Cell Prolif 2020;53:e12867. [Crossref] [PubMed]
- Siddiqui RA, Zerouga M, Wu M, et al. Anticancer properties of propofol-docosahexaenoate and propofol-eicosapentaenoate on breast cancer cells. Breast Cancer Res 2005;7:R645-54. [Crossref] [PubMed]
- Zhang J, Wu GQ, Zhang Y, et al. Propofol induces apoptosis of hepatocellular carcinoma cells by upregulation of microRNA-199a expression. Cell Biol Int 2013;37:227-32. [Crossref] [PubMed]
- Miao Y, Zhang Y, Wan H, et al. GABA-receptor agonist, propofol inhibits invasion of colon carcinoma cells. Biomed Pharmacother 2010;64:583-8. [Crossref] [PubMed]
Mamoor S. Over-expression of RNA polymerase II subunit L in human endometrial cancer. 2021 . Doi: .10.31219/osf.io/5nmr7 - Barba-Aliaga M, Alepuz P, Pérez-Ortín JE. Eukaryotic RNA Polymerases: The Many Ways to Transcribe a Gene. Front Mol Biosci 2021;8:663209. [Crossref] [PubMed]
- Yao F, Zhan Y, Li C, et al. Single-Cell RNA Sequencing Reveals the Role of Phosphorylation-Related Genes in Hepatocellular Carcinoma Stem Cells. Front Cell Dev Biol 2022;9:734287. [Crossref] [PubMed]
- Zhou D, Li X, Zhao H, et al. Combining multi-dimensional data to identify a key signature (gene and miRNA) of cisplatin-resistant gastric cancer. J Cell Biochem 2018;119:6997-7008. [Crossref] [PubMed]
- Chen T, You Y, Jiang H, et al. Epithelial-mesenchymal transition (EMT): A biological process in the development, stem cell differentiation, and tumorigenesis. J Cell Physiol 2017;232:3261-72. [Crossref] [PubMed]
- Ribatti D, Tamma R, Annese T. Epithelial-Mesenchymal Transition in Cancer: A Historical Overview. Transl Oncol 2020;13:100773. [Crossref] [PubMed]
- Pearson GW. Control of Invasion by Epithelial-to-Mesenchymal Transition Programs during Metastasis. J Clin Med 2019;8:646. [Crossref] [PubMed]
- Liu S, Chen S, Zeng J. TGF‑β signaling: A complex role in tumorigenesis Mol Med Rep 2018;17:699-704. (Review). [PubMed]
- Luo K. Signaling Cross Talk between TGF-β/Smad and Other Signaling Pathways. Cold Spring Harb Perspect Biol 2017;9:a022137. [Crossref] [PubMed]