A novel angiogenesis-associated risk score predicts prognosis and characterizes the tumor microenvironment in colon cancer
Highlight box
Key findings
• This study has identified two distinct molecular subtypes of angiogenesis in colon cancer (CC) patients, which differ significantly in their genomic backgrounds, expression profiles, prognostic outcomes, and tumor microenvironment characteristics. Furthermore, the study established an angiogenesis-related genes score (AR score) that independently predicts survival in CC patients and correlates with tumor mutation burden, cancer stem cell score, and drug sensitivity.
What is known and what is new?
• Angiogenesis, the formation of new blood vessels, plays a critical role in tumor growth and metastasis. Previous studies have investigated the role of angiogenesis in CC, but there is a lack of comprehensive bioinformatics analysis to clarify its molecular characteristics and prognostic values.
• The study established an AR score, which can be a novel biomarker for clinicians to guide the personalized management of CC patients.
What is the implication, and what should change now?
• The findings of this study suggest that angiogenesis characteristics play a crucial role in CC prognosis and treatment response.
• The AR score could potentially be used as a prognostic biomarker to guide individualized treatment strategies for CC patients.
• The identification of distinct molecular subtypes of angiogenesis opens up new opportunities for targeted therapies and immunotherapy development in CC.
Introduction
Colon cancer (CC) is one of the most common digestive malignancies and seriously threatens human health, with over 1.5 million new cases and nearly a million cancer-related deaths worldwide in 2020 (1). Well-performed surgery comprises the majority of curative treatments for CC and can achieve long-term survival in certain patients (2). Unfortunately, 70% (3) of colon and rectal cancer patients will develop metastatic disease, and approximately 20% of CC patients have unresectable distant metastases (4) at initial diagnosis, which indicates a poor prognosis. In recent years, immunotherapy has broadened the therapeutic spectrum for numerous tumor types. Immune checkpoint inhibitors (ICIs), represented by programmed death receptor 1 (PD-1) inhibitors, are a popular choice for cancer patients and can exert remarkable efficacy in a variety of solid malignancies, including colon and rectal cancer (5-7). However, not all cancer patients can benefit from immunotherapy, and further follow-up data have shown that many of those who can benefit will eventually become resistant to immunotherapy (8). Thus, it is an important clinical issue to predict a patient’s response to immunotherapy and ameliorate resistance to immunotherapy.
The immunosuppressive effect of the tumor microenvironment (TME) is ascribed as the main reason for cancer patients’ resistance to immunotherapies. Being perceived as a dynamic tumor ecosystem, the TME includes cancer cells, blood and lymphatic vessels, immune cells, stromal cells, and extracellular matrix (9). The abnormal structure of blood vessels and dysfunctional angiogenesis in the TME can promote the proliferation and metastases of tumor cells by providing a hypoxic, low pH, and immunosuppressive environment with high interstitial pressure (10). Recently, antiangiogenic agents aimed at normalizing aberrant blood vessels have gained much attention as a novel cancer therapy (10), and they can significantly improve the efficacy of immunotherapy in CC, as a preclinical study has demonstrated (11).
A previous study has demonstrated the role of individual angiogenesis-associated genes (AAGs) in the progression and metastasis of CC (12). However, the expression and prognostic role of AAGs have not been examined in CC patients. Thus, holistic analysis of the association between AAGs and CC can contribute to guiding the management of CC patients. The current study aimed to perform a comprehensive elucidation of the correlation between AAGs and CC for the purpose of improving the clinical management of CC. We present this article in accordance with the TRIPOD reporting checklist (available at https://tcr.amegroups.com/article/view/10.21037/tcr-23-2048/rc).
Methods
Data collection
In this study, we downloaded the RNA-sequencing data, somatic mutations, copy number variation (CNV) files, and clinical data of CC from The Cancer Genome Atlas (TCGA) database (https://portal.gdc.cancer.gov/). And we retrieved a Gene Expression Omnibus (GEO) dataset, GSE39582 (https://www.ncbi.nlm.nih.gov/), which contained the clinical information and normalized gene expression data, via GEO repository. After screening, samples without significant clinical data or with overall survival (OS) less than 90 days (13) were filtered out from further analysis. Moreover, AAGs were sourced from the MSigDB Team (http://www.broad.mit.edu/gsea/msigdb/) and pertinent literature (14-16), and are displayed in Table S1. Leveraging RNA-seq data from TCGA-colon adenocarcinoma (COAD) specimens accessed via UCSC Xena, all AAGs were discerned through the application of the limma package [P<0.05, |log2 fold change (FC)| >1]. Subsequently, pivotal genes were pinpointed utilizing a univariate Cox regression model. The study was conducted in accordance with the Declaration of Helsinki (as revised in 2013).
Consensus clustering analysis of AAGs
Consensus clustering was conducted to distinguish angiogenesis-associated patterns relating to the expression of angiogenesis regulators via the k-means method. The consensus clustering algorithm obtained from “ConsensuClusterPlus” package (17) was applied to determine the numbers and the stability of the clusters. One thousand times repetitions were employed to the make sure the stability of the classification. And the gene set variation analysis (GSVA) of the “GSVA” packages (18) was performed with the “c2.cp.kegg.v7.4” from the MSigDB database to identify the differences of biological function in AAGs.
Relationship of molecular patterns with the clinical characteristics, prognosis and TME of CC
The clinical value of the clusters identified by consensus clustering was assessed via the correlation among molecular patterns, survival results and clinical characteristics (age, gender, T-stage, N-stage, and M-stage). The Kaplan–Meier analysis obtained from “survival” and “survminer” packages (19) was performed to estimate the differences of OS among the clusters and to validate the results, the principal component analysis (PCA) was performed. The ESTIMATE algorithm (20) was conducted to estimate the immune and stromal scores of CC patients and then the CIBERSORT algorithm (21) was performed to assess the infiltration of 22 immune cell subtypes of every patient. And the single-sample gene set enrichment analysis (ssGSEA) algorithm (22) was applied to calculate the proportions of more specific components. Additionally, the expression of ICIs [PD-1, programmed death ligand-1 (PD-L1), and cytotoxic T lymphocyte-associated antigen-4 (CTLA-4)] was compared among distinct clusters.
Identification of differentially expressed genes (DEGs) and functional enrichment analysis
The DEGs (|log2FC| ≥1 and P<0.05) of distinct angiogenesis subgroups were identified using the limma package (23) and the correlation between DEGs and OS (P<0.05) was confirmed by univariable Cox regression. Using the “clusterProfiler” package, Gene Ontology (GO) and Kyoto Encyclopedia of Genes and Genomes (KEGG) analyses were conducted based on the identified DEGs.
Construction and clinicopathological features analysis of angiogenesis-associated genes score
To quantitatively estimate the level of angiogenesis characteristics in every CC patient, we established the angiogenesis-related genes score (AR score). We standardized the expression profile of DEGs from different angiogenesis characteristics clusters across CC samples and selected the intersecting genes. There were 234 DEGs between the two angiogenesis characteristics clusters as presented by the differential estimation, and univariate Cox regression (uniCox) analysis for DEGs was also performed. We employed LASSO regression and multivariate Cox regression analysis to screen for prognostic DEGs associated with angiogenesis clusters, and subsequently constructed a prognostic model. Through this approach, we identified eight key genes (STC1, CYP1B1, CD36, MMP12, HOXC6, HSPA1A, CXCL11, KLK10) and calculated an individual risk score (AR score) for each patient. Risk score = (0.2044 × expression of STC1) + (0.1718 × expression of CYP1B1) + (0.1738 × expression of CD36) + (−0.1556 × expression of MMP12) + (0.2139 × expression of HOXC6) + (0.1585 × expression of HSPA1A) + (−0.1907 × expression of CXCL11) + (0.1312 × expression of KLK10). Then, we separated CC patients into high-risk and low-risk groups according to the median AR score. To assess the prognostic ability of the risk model, receiver operating characteristic (ROC) and Kaplan-Meier curves were employed. We explored the correlation between the AR score and clinical factors. Then, the reliability of the AR score’s predictive role was examined in distinct subsets on the basis of various clinical characteristics. We compared the infiltration of immune cells and immune checkpoints (ICP) in the distinct AR score subgroups. In addition, we obtained the RNAss file titled “StemnessScores_RNAexp_20170127.2.tsv” and extracted tumor stem cell attributes from the transcriptome and epigenetic profiles of the specimens. These features were subsequently utilized to assess the stem cell-like properties of the tumors. Furthermore, we conducted a correlation analysis to explore the relevance of the AR score to the tumor mutation burden (TMB) score and cancer stem cell (CSC) score.
Development of a prognostic nomogram
Both the uniCox and multivariate Cox regression (multiCox) analyses in all sets were employed to elucidate whether the AR score exhibited independent prognostic value. In this study, we constructed a prognostic nomogram including age, sex, T stage, N stage, M stage and AR score to predict the 1-, 3-, and 5-year OS of CC patients more accurately. Then, calibration curve analysis and decision curve analysis (DCA) were performed to determine whether the established nomogram was reliable.
Mutation and drug sensitivity analysis
The mutation annotation format (MAF) from the TCGA database was computed using the maftools package (24), and we compared the mutational profiles between CC patients with different AR scores. Additionally, the tumor immune dysfunction and exclusion (TIDE) and immunophenotype score (IPS) were estimated for CC patients with different AR scores. The semi-inhibitory concentration (IC50) values of the drugs were assessed via the pRRophetic package (25) to explore the clinical utility of the AR score to predict the patient’s response to common chemotherapy agents. We compared the differences in the IC50 between the low-risk and high-risk groups by the Wilcoxon signed-rank test, and we used the box drawings obtained using the pRRophetic and ggplot2 packages to exhibit the results.
Statistical analysis
All the analyses in our study were conducted using R software (version 4.1.2) and the relevant packages. A two-sided P<0.05 was defined as statistically significant.
Results
Genetic mutation landscape and generation subgroups of AAGs in CC
We described the details of our study in the flowchart in Figure S1. A total of 956 CC patients from COAD-TCGA and GSE39582 were included in our study. We displayed all the detailed information of CC patients in https://cdn.amegroups.cn/static/public/tcr-23-2048-1.xlsx. The expression of the AAGs in tumor samples and normal samples from the COAD-TCGA program was evaluated separately. In total, there was a significant difference in the background genome and expression profiles of AAGs between tumor samples and normal samples in CC, and the difference might be explained by the potential role of AAGs in the development of CC. Detailed information on the genetic background and expression profiles of the AAGs is presented in Figure S2.
For all genes in AAGs, the uniCox and Kaplan-Meier analyses were conducted to assure their predictive utility in CC (Table S2). Then, we demonstrated the correlation network of AAG interactions, regulator association, and their survival importance in CC patients (Figure S3A & https://cdn.amegroups.cn/static/public/tcr-23-2048-2.xlsx). A consensus clustering analysis relating to the expression levels of AAGs was employed, and the optimal number of clusters was identified as 2 (Figure S3B). Then, CC patients were finely divided into Cluster A (n=451) and Cluster B (n=505). The prognosis and clinical parameters between the two clusters are depicted in Figure S3C-S3E.
Characteristics of the TME in different subgroups
As indicated by the results of GSVA, Cluster A was abundant in oncogenic pathways (multiple cancers, such as small cell lung cancer, renal cell carcinoma, prostate cancer, and melanoma), metastasis-associated pathways (regulation of cell adhesion molecules, extracellular matrix (ECM) receptor interaction, and focal adhesion) and innate immune-associated pathways [Toll-like receptor (TLR) signalling pathway and nucleotide-binding oligomerization domain (NOD)-like receptor signalling pathway] (Figure 1A and https://cdn.amegroups.cn/static/public/tcr-23-2048-3.xlsx). With the CIBERSORT algorithm, the infiltrating levels of 23 human immune cell subsets between the two clusters were compared (https://cdn.amegroups.cn/static/public/tcr-23-2048-4.xlsx). There was a substantial enrichment difference in most immune cells between Clusters A and B (Figure 1B). The enrichment levels of the following cell types were notably higher in Cluster A compared to Cluster B: activated B cells, activated CD4 T cells, activated CD8 T cells, activated DC cells, CD56bright NK cells, CD56dim NK cells, eosinophils, gd T cells, immature B cells, immature DC cells, MDSCs, macrophages, mast cells, NK T cells, NK cells, plasmacytoid DC cells, regulatory T cells, T follicular helper cells, type 1 T helper cells and type 1 T helper cells. However, the opposite performance of Type.17.T.helper.cellna was observed. Moreover, Cluster A had significantly higher expressions of both PD-1, PD-L1, and CTLA-4 than Cluster B (Figure 1C-1E). Furthermore, the ESTIMATE algorithm revealed that higher TME scores including immune scores, stromal score and estimate score occurred in Cluster A than in Cluster B (Figure 1F-1H).
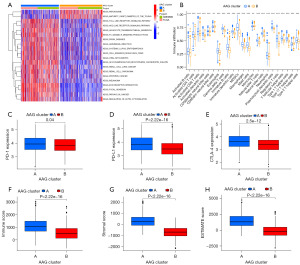
Identification of gene subgroups based on DEGs
Eight hundred eighty-four DEGs were obtained to elucidate the biological activity of different angiogenesis characteristics subgroups, and GO enrichment analysis and KEGG enrichment analysis were performed (https://cdn.amegroups.cn/static/public/tcr-23-2048-5.xlsx). Most of these DEGs were enriched in cancer- and metastasis-associated biological processes (Figure 2A,2B). A total of 432 genes with survival significance (P<0.05) were retrieved by uniCox analysis (https://cdn.amegroups.cn/static/public/tcr-23-2048-6.xlsx). Next, a consensus clustering method was performed to classify CC patients into different gene clusters (Clusters 1 and 2) based on the prognostic genes (Figure S4). As displayed in Figure 2C, CC patients in Cluster A had a longer OS than those in Cluster B. In addition, angiogenesis gene Cluster B patterns were relevant to advanced T stage, N stage and M stage (Figure 2D). A substantial difference in AAG expression was also observed in the angiogenesis gene clusters (Figure 2E).
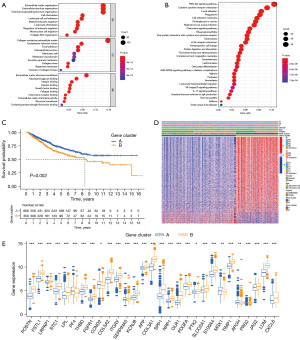
Development and validation of the prognostic AR score
The CC patients were randomly distributed into a training set (n=478) or a test set (n=478) at a ratio of 1:1. To construct an optimal prognostic model, we performed LASSO and multivariate Cox (multiCox) analyses for 432 angiogenesis cluster-associated prognostic DEGs. Next, eight genes (STC1, CYP1B1, CD36, MMP12, HOXC6, HSPA1A, CXCL11, KLK10) remained in the model, and the AR score was estimated as follows: Risk score = (0.2044 × expression of STC1) + (0.1718 × expression of CYP1B1) + (0.1738 × expression of CD36) + (−0.1556 × expression of MMP12) + (0.2139 × expression of HOXC6) + (0.1585 × expression of HSPA1A) + (−0.1907 × expression of CXCL11) + (0.1312 × expression of KLK10) (Figure S5). The distribution of patients in the two angiogenesis characteristics clusters, two gene clusters, and two AR score groups is presented in Figure 3A. A lower AR score was observed in AAG Cluster B (Figure 3B) and the Cluster A gene (Figure 3C). In the Kaplan-Meier analysis, CC patients with low AR score had a better OS than those with high AR score in the training cohort (Figure 3D), and the AUCs of 1-, 3-, and 5-year OS were 0.788, 0.743, and 0.721, respectively (Figure 3E). The significant distribution between the two risk groups was confirmed by PCA (Figure 3F). As displayed in the risk plot of AR score, when the AR score increased, the OS time decreased, and death increased (Figure 3G-3I). In Figure 3I, we present a heatmap of selected genes. The AR score of the test cohort and entire cohort were also evaluated to confirm its prognostic ability (Figures S6,S7). The CC patients were also divided into low- and high-risk subgroups according to the median score of the training cohort. The results of survival analysis were consistent with the results in the training cohort. It was also confirmed that the AR score had good AUC values for 1-, 3-, and 5-year OS in the test cohort and entire cohort. The correlation between AR score and multiple clinical variables (age, sex, T stage, N stage, M stage, and survival status) was explored. In general, the AR score was an independent risk factor for CC patients in the training cohort and the entire cohort, and detailed information is displayed in Figures S8-S10.
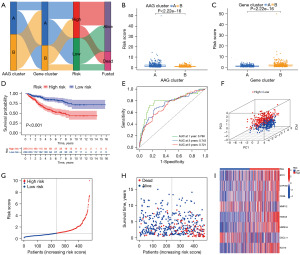
Construction of a nomogram to predict patients’ prognosis
A nomogram was constructed based on the risk scores and clinical variables. The survival outcomes, including 1-, 3-, and 5-year OS, were evaluated by the nomogram (Figure 4A). In Figure 4B, the nomogram’s calibration curves exhibited excellent accuracy between actual observations and predicted values. The AUC values of the nomogram in all cohorts exhibited great prognostic value for predicting the 1-, 3-, and 5-year OS rates (Figure 4C-4E). The predictive nomogram generated more net benefits for predicting survival in the DCA (Figure 4F-4H).
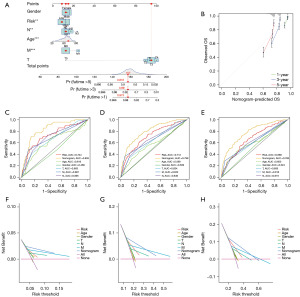
Assessment of TME and checkpoints in distinct groups
The association between the AR score and immune cell infiltration was evaluated with the CIBERSORT algorithm. The results indicated that the AR score was positively correlated with the infiltrating status of M0 macrophages, M2 macrophages, and neutrophils, while the AR score was negatively correlated with the infiltrating status of resting dendritic cells, M1 macrophages, plasma cells, resting NK cells, activated dendritic cells, follicular helper T cells, CD8+ T cells, activated memory CD4+ T cells, and neutrophils (Figure 5A). The AR score was positively linked to the stromal score (Figure 5B). As depicted in Figure 5C, most of the immune cells were closely related to the selected genes. Moreover, as the correlation between ICPs and this predictive feature revealed, 25 ICPs were inconsistently depicted in the two risk subgroups (Figure 5D).
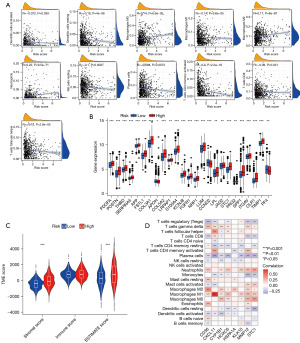
Association of AR score with TMB score, CSC score and drug sensitivity analysis
A higher TMB occurred in the high-risk groups than in the low-risk groups in our study (Figure S11A), and there was a positive correlation between AR score and TMB by Spearman correlation analysis (Figure S11B). In the consequent Kaplan-Meier analysis, CC patients with low TMB scores tended to have improved survival outcomes (Figure S11C). Then, the TMB and AR score were merged for the following survival analysis of CC patients, and the results revealed that patients with low AR score and low TMB score had the best survival outcomes, and patients with high AR score and high TMB score had the worst survival outcomes (Figure S11D). There appears to be a substantial correlation between the AR score and the classification of microsatellite instability (MSI) status (Figure S11E-S11G). In addition, there was a negative correlation between AR score and CSC score.
Drug sensitivity analysis
The TIDE scores and IPS scores were calculated to predict the response ability of CC patients, and patients with low risk had a lower TIDE score and a higher IPS score than those with high risk (Figure S12A-S12E). This finding could be ascribed to the reason that low-risk patients were more sensitive to immunotherapy (26). Moreover, the IC50 values of 138 drugs in COAD-TCGA subsets were evaluated to determine whether the AR score could be a biomarker for the therapeutic efficacy prediction for CC patients. In total, AAGs were associated with drug sensitivity, and detailed information on sensitive drugs related to the AR score is displayed in Figure S12F-S12P and Figure S13.
Discussion
Aberrant angiogenesis in tumors is characterized as disordered, immature, impermeable and dysfunctional (27). The concordant results indicated by a previous study (28) revealed that the onset of the angiogenic switch was necessary for the development from an in situ tumor to an aggressive cancer, especially in solid tumors. In addition to the hypoxia and acidic characteristics of the TME caused by angiogenesis, the overexpression of VEGF could also lead to an immune-suppressive TME (10). Moreover, poor perfusion due to angiogenesis could also prevent the diffusion of chemotherapeutic drugs (27,28). Recently, in addition to the independent antitumor effect of anti-angiogenesis drugs, the synergistic effect of anti-angiogenesis and immune response (29) or chemotherapy (30) has also been detected; thus, anti-angiogenesis in tumors was a promising target for drug investigation.
Notably, there is a lack of bioinformatics analysis to comprehensively clarify the molecular characteristics, immune interaction characteristics and predictive values of angiogenesis characteristics in CC. In the present study, the mutations and expression levels of AAG transcription profiles in the COAD-TCGA cohort were first assessed. The results revealed that the majority of AAGs were overexpressed in tumor samples, suggesting the prognostic ability of AAGs in CC patients. Due to the highly heterogeneous characteristics of CC, it is necessary to accurately distinguish the molecular subtypes to achieve individual target management of patients. In the current study, patients were classified into angiogenesis-mediated clusters (Clusters A and B), between which the clinical parameters, immune infiltrations, and functions were significantly different.
This study was the first to establish an angiogenesis-associated risk model to predict prognosis, discover the immune infiltration of the TME, distinguish the response to immunotherapy and explore potential chemotherapeutic drugs in CC. We established the AR score via LASSO Cox regression to quantitatively represent angiogenesis characteristics in CC patients. In our study, the AUC values of the AR score for 1-, 3-, and 5-year OS in the training cohort presented great accuracy and specificity (AUCs >0.7). MultiCox analysis was conducted to eliminate the bias of confounding variables, and the AR score remained independently associated with survival outcomes in all CC patients. Combined with the net benefits that could be generated from the AR score in the DCA, we concluded that this novel risk score model provides a practical method of great efficacy for clinicians to predict survival for CC patients.
In addition, eight genes in this risk model have been reported in a previous study of cancer. Stanniocalcin-1 (STC-1) could promote the progression of gastric cancer by enhancing angiogenesis via the ability to upregulate the expression of VEGF (31). A previous study concluded that cytochrome P450 family 1B1 (CYP1B1) is an angiogenesis-associated enzyme that is usually overexpressed in various cancers (32). CD36 is a scavenger receptor and has been studied as a target for suppressing in vivo angiogenesis in many tumor models (33,34). Interestingly, although matrix metalloproteinase 12 (MMP12) was overexpressed (35) in CC, it was reported that MMP12 could reduce the growth of CC cells and was associated with favourable OS in CC patients (36), which was concordant with the negative corresponding coefficient of MMP12 in the AR score calculation in our study. Human Hox genes (Homeobox) can regulate the processes of angiogenesis (37), and homeobox C6 (HOXC6) was reported to have the ability to promote the development of CC cells by inducing epithelial-mesenchymal transition (EMT) through the Wnt/β-catenin pathway (38). HSPA1A is a heat shock protein (HSP). Previous studies suggested that HSPA1A could promote the proliferation, metastasis, and invasion of cancer by exempting cancer cells from stress such as oxidative stress (39) and avoiding apoptosis. The expression of the chemokine ligand C-X-C motif chemokine ligand 11 (CXCL11) was upregulated in tumor samples of CC compared with normal samples, and the increased expression was correlated with improved OS in CC patients (40), confirming the negative correlation coefficient of CXCL11 in our AR score algorithm. Kallikrein-related peptidase 10 (KLK10) is a member of the kallikrein family, and a previous study has indicated that KLK10 could be a predictive biomarker and possible target of treatment (41).
Except for the different prognosis, an obvious difference between patients with low or high AR score was observed in genomic alteration in our study. A high TMB score was more relevant in the high AR score groups than in the low AR score groups, but samples with a higher TMB were not significantly associated with poor patient prognosis. As a previous study concluded, it remains controversial whether higher TMB is associated with improved prognosis, especially in non-ICI-treated patients (42). Interestingly, CC patients with low AR score and low TMB score had the best survival outcomes among all the patients in our study, suggesting that AR score could improve the predictive efficacy of TMB scores.
Intriguingly, we identified a negative correlation between AR score and the infiltration of M1 macrophages and a positive correlation between AR score and the infiltration of M2 macrophages, which indicated the polarization of macrophages from M1 to M2 subtypes, while the AR score increased. Therefore, it was reasonable to postulate that angiogenesis may be associated with the formation of the tumor-favourable TME. In fact, previous research suggested that by regulating clonal selection (10), an immunosuppressive TME could promote angiogenesis in cancer patients. The vicious circle between angiogenesis and the immunosuppressive TME needs to be explored in the future.
To improve the drug management of CC patients, we explored potential sensitive drugs based on the AR score. Therefore, clinicians could use these results as a reference to avoid drug resistance and improve the efficacy of treatments for individual patients. The AR score was indicated to be associated with patients’ sensitivity to immunotherapy by the analysis of TIDE and IPS signatures. Combining the results that decreased infiltration of multiple immune cells occurred in patients with high AR score, we concluded that the AR score might be used as a novel biomarker to predict the responsiveness to immunotherapy. The genes identified in this study that are associated with CC angiogenesis hold promise as new gene targets for subsequent foundational research on CC angiogenesis. Specifically, future investigations can start from immunohistochemical analyses to elucidate the expression patterns of these key genes, and then delve into their mechanisms of action in CC angiogenesis using in vitro and in vivo experimental approaches. These experimental methods include but are not limited to xenograft model construction, angiogenesis assays, chick chorioallantoic membrane angiogenesis analysis, lumen formation assays, mouse Matrigel injection models, and rabbit corneal angiogenesis assays. Through these systematic studies, we aim to comprehensively reveal the functions and regulatory networks of these key genes in CC angiogenesis.
There are several limitations in our study. First, our efforts might be limited by the retrospective nature of data from public databases and the inevitable bias of selection. Second, the lack of other clinical parameters could interfere with the clinical value of the AR score. The TCGA-COAD dataset exhibits a deficiency in chemotherapy and immunotherapy patient data, which may have introduced potential biases into our findings, thereby representing a notable limitation of our study. In order to alleviate this concern, we aim to conduct additional validation utilizing more extensive CC cohorts that comprehensively encompass these pertinent treatment details.
Conclusions
In conclusion, we have comprehensively examined the correlation between AAGs and clinical parameters, TME, therapeutic response, and survival outcomes in CC patients. The AR score can be a novel biomarker for clinicians to guide the personalized management of CC patients.
Acknowledgments
Funding: None.
Footnote
Reporting Checklist: The authors have completed the TRIPOD reporting checklist. Available at https://tcr.amegroups.com/article/view/10.21037/tcr-23-2048/rc
Peer Review File: Available at https://tcr.amegroups.com/article/view/10.21037/tcr-23-2048/prf
Conflicts of Interest: All authors have completed the ICMJE uniform disclosure form (available at https://tcr.amegroups.com/article/view/10.21037/tcr-23-2048/coif). The authors have no conflicts of interest to declare.
Ethical Statement: The authors are accountable for all aspects of the work in ensuring that questions related to the accuracy or integrity of any part of the work are appropriately investigated and resolved. The study was conducted in accordance with the Declaration of Helsinki (as revised in 2013).
Open Access Statement: This is an Open Access article distributed in accordance with the Creative Commons Attribution-NonCommercial-NoDerivs 4.0 International License (CC BY-NC-ND 4.0), which permits the non-commercial replication and distribution of the article with the strict proviso that no changes or edits are made and the original work is properly cited (including links to both the formal publication through the relevant DOI and the license). See: https://creativecommons.org/licenses/by-nc-nd/4.0/.
References
- Sung H, Ferlay J, Siegel RL, et al. Global Cancer Statistics 2020: GLOBOCAN Estimates of Incidence and Mortality Worldwide for 36 Cancers in 185 Countries. CA Cancer J Clin 2021;71:209-49. [Crossref] [PubMed]
- Schmoll HJ, Van Cutsem E, Stein A, et al. ESMO Consensus Guidelines for management of patients with colon and rectal cancer. a personalized approach to clinical decision making. Ann Oncol 2012;23:2479-516. [Crossref] [PubMed]
- Wang J, Li S, Liu Y, et al. Metastatic patterns and survival outcomes in patients with stage IV colon cancer: A population-based analysis. Cancer Med 2020;9:361-73. [Crossref] [PubMed]
- Neumann J, Horst D, Kriegl L, et al. A simple immunohistochemical algorithm predicts the risk of distant metastases in right-sided colon cancer. Histopathology 2012;60:416-26. [Crossref] [PubMed]
- Robert C, Ribas A, Schachter J, et al. Pembrolizumab versus ipilimumab in advanced melanoma (KEYNOTE-006): post-hoc 5-year results from an open-label, multicentre, randomised, controlled, phase 3 study. Lancet Oncol 2019;20:1239-51. [Crossref] [PubMed]
- Garon EB, Rizvi NA, Hui R, et al. Pembrolizumab for the treatment of non-small-cell lung cancer. N Engl J Med 2015;372:2018-28. [Crossref] [PubMed]
- Meric-Bernstam F, Hurwitz H, Raghav KPS, et al. Pertuzumab plus trastuzumab for HER2-amplified metastatic colorectal cancer (MyPathway): an updated report from a multicentre, open-label, phase 2a, multiple basket study. Lancet Oncol 2019;20:518-30. [Crossref] [PubMed]
- Giannone G, Ghisoni E, Genta S, et al. Immuno-Metabolism and Microenvironment in Cancer: Key Players for Immunotherapy. Int J Mol Sci 2020;21:4414. [Crossref] [PubMed]
- Anderson NM, Simon MC. The tumor microenvironment. Curr Biol 2020;30:R921-5. [Crossref] [PubMed]
- Zhao Y, Yu X, Li J. Manipulation of immune-vascular crosstalk: new strategies towards cancer treatment. Acta Pharm Sin B 2020;10:2018-36. [Crossref] [PubMed]
- Unterleuthner D, Neuhold P, Schwarz K, et al. Cancer-associated fibroblast-derived WNT2 increases tumor angiogenesis in colon cancer. Angiogenesis 2020;23:159-77. [Crossref] [PubMed]
- Qiu P, Guo Q, Yao Q, et al. Characterization of Exosome-Related Gene Risk Model to Evaluate the Tumor Immune Microenvironment and Predict Prognosis in Triple-Negative Breast Cancer. Front Immunol 2021;12:736030. [Crossref] [PubMed]
- Sabah A, Tiun S, Sani NS, et al. Enhancing web search result clustering model based on multiview multirepresentation consensus cluster ensemble (mmcc) approach. PLoS One 2021;16:e0245264. [Crossref] [PubMed]
- Sun W, Xu Y, Zhao B, et al. The prognostic value and immunological role of angiogenesis-related patterns in colon adenocarcinoma. Front Oncol 2022;12:1003440. [Crossref] [PubMed]
- Zhen Z, Shen Z, Hu Y, et al. Screening and identification of angiogenesis-related genes as potential novel prognostic biomarkers of hepatocellular carcinoma through bioinformatics analysis. Aging (Albany NY) 2021;13:17707-33. [Crossref] [PubMed]
- Kang J, Xiang X, Chen X, et al. Angiogenesis-related gene signatures reveal the prognosis of cervical cancer based on single cell sequencing and co-expression network analysis. Front Cell Dev Biol 2023;10:1086835. [Crossref] [PubMed]
- Hänzelmann S, Castelo R, Guinney J. GSVA: gene set variation analysis for microarray and RNA-seq data. BMC Bioinformatics 2013;14:7. [Crossref] [PubMed]
- Rich JT, Neely JG, Paniello RC, et al. A practical guide to understanding Kaplan-Meier curves. Otolaryngol Head Neck Surg 2010;143:331-6. [Crossref] [PubMed]
- Meng Z, Ren D, Zhang K, et al. Using ESTIMATE algorithm to establish an 8-mRNA signature prognosis prediction system and identify immunocyte infiltration-related genes in Pancreatic adenocarcinoma. Aging (Albany NY) 2020;12:5048-70. [Crossref] [PubMed]
- Chen B, Khodadoust MS, Liu CL, et al. Profiling Tumor Infiltrating Immune Cells with CIBERSORT. Methods Mol Biol 2018;1711:243-59. [Crossref] [PubMed]
- Huang L, Wu C, Xu D, et al. Screening of Important Factors in the Early Sepsis Stage Based on the Evaluation of ssGSEA Algorithm and ceRNA Regulatory Network. Evol Bioinform Online 2021;17:11769343211058463. [Crossref] [PubMed]
- Ritchie ME, Phipson B, Wu D, et al. limma powers differential expression analyses for RNA-sequencing and microarray studies. Nucleic Acids Res 2015;43:e47. [Crossref] [PubMed]
- Mayakonda A, Lin DC, Assenov Y, et al. Maftools: efficient and comprehensive analysis of somatic variants in cancer. Genome Res 2018;28:1747-56. [Crossref] [PubMed]
- Geeleher P, Cox N, Huang RS. pRRophetic: an R package for prediction of clinical chemotherapeutic response from tumor gene expression levels. PLoS One 2014;9:e107468. [Crossref] [PubMed]
- Jiang P, Gu S, Pan D, et al. Signatures of T cell dysfunction and exclusion predict cancer immunotherapy response. Nat Med 2018;24:1550-8. [Crossref] [PubMed]
- Viallard C, Larrivée B. Tumor angiogenesis and vascular normalization: alternative therapeutic targets. Angiogenesis 2017;20:409-26. [Crossref] [PubMed]
- Ye W. The Complexity of Translating Anti-angiogenesis Therapy from Basic Science to the Clinic. Dev Cell 2016;37:114-25. [Crossref] [PubMed]
- Yi M, Jiao D, Qin S, et al. Synergistic effect of immune checkpoint blockade and anti-angiogenesis in cancer treatment. Mol Cancer 2019;18:60. [Crossref] [PubMed]
- Ohtsu A, Shah MA, Van Cutsem E, et al. Bevacizumab in combination with chemotherapy as first-line therapy in advanced gastric cancer: a randomized, double-blind, placebo-controlled phase III study. J Clin Oncol 2011;29:3968-76. [Crossref] [PubMed]
- He LF, Wang TT, Gao QY, et al. Stanniocalcin-1 promotes tumor angiogenesis through up-regulation of VEGF in gastric cancer cells. J Biomed Sci 2011;18:39. [Crossref] [PubMed]
- D'Uva G, Baci D, Albini A, et al. Cancer chemoprevention revisited: Cytochrome P450 family 1B1 as a target in the tumor and the microenvironment. Cancer Treat Rev 2018;63:1-18. [Crossref] [PubMed]
- Sp N, Kang DY, Kim DH, et al. Nobiletin Inhibits CD36-Dependent Tumor Angiogenesis, Migration, Invasion, and Sphere Formation Through the Cd36/Stat3/Nf-Kb Signaling Axis. Nutrients 2018;10:772. [Crossref] [PubMed]
- Kaur B, Cork SM, Sandberg EM, et al. Vasculostatin inhibits intracranial glioma growth and negatively regulates in vivo angiogenesis through a CD36-dependent mechanism. Cancer Res 2009;69:1212-20. [Crossref] [PubMed]
- Asano T, Tada M, Cheng S, et al. Prognostic values of matrix metalloproteinase family expression in human colorectal carcinoma. J Surg Res 2008;146:32-42. [Crossref] [PubMed]
- Yang W, Arii S, Gorrin-Rivas MJ, et al. Human macrophage metalloelastase gene expression in colorectal carcinoma and its clinicopathologic significance. Cancer 2001;91:1277-83. [Crossref] [PubMed]
- Abate-Shen C. Deregulated homeobox gene expression in cancer: cause or consequence? Nat Rev Cancer 2002;2:777-85. [Crossref] [PubMed]
- Qi L, Chen J, Zhou B, et al. HomeoboxC6 promotes metastasis by orchestrating the DKK1/Wnt/β-catenin axis in right-sided colon cancer. Cell Death Dis 2021;12:337. [Crossref] [PubMed]
- Garrido C, Brunet M, Didelot C, et al. Heat shock proteins 27 and 70: anti-apoptotic proteins with tumorigenic properties. Cell Cycle 2006;5:2592-601. [Crossref] [PubMed]
- Luan Y, Luan Y, Zhao Y, et al. Isorhamnetin in Tsoong blocks Hsp70 expression to promote apoptosis of colon cancer cells. Saudi J Biol Sci 2019;26:1011-22. [Crossref] [PubMed]
- Liu K, Lai M, Wang S, et al. Construction of a CXC Chemokine-Based Prediction Model for the Prognosis of Colon Cancer. Biomed Res Int 2020;2020:6107865. [Crossref] [PubMed]
- Alexopoulou DK, Papadopoulos IN, Scorilas A. Clinical significance of kallikrein-related peptidase (KLK10) mRNA expression in colorectal cancer. Clin Biochem 2013;46:1453-61. [Crossref] [PubMed]
- Valero C, Lee M, Hoen D, et al. The association between tumor mutational burden and prognosis is dependent on treatment context. Nat Genet 2021;53:11-5. [Crossref] [PubMed]