APOA1 mRNA and serum APOA1 protein as diagnostic and prognostic biomarkers in gastric cancer
Highlight box
Key findings
• The study identified apolipoprotein A-I (APOA1) as a potential prognostic biomarker for gastric cancer (GC). APOA1 was found to be downregulated in GC tissues and associated with poor prognosis.
• The serum APOA1 levels were significantly lower in GC patients, and these lower levels were associated with poor prognosis and clinical characteristics. The receiver operating characteristic analysis demonstrated the diagnostic ability of serum APOA1.
What is known and what is new?
• GC is a significant global health concern with poor survival rates, necessitating the search for effective diagnostic and prognostic markers.
• This study utilizes bioinformatics and clinical data to identify and validate lipid metabolism-related genes APOA1 as potential diagnostic and prognostic biomarkers for GC.
What is the implication, and what should change now?
• The protein level of APOA1 demonstrated excellent diagnostic capability.
• Elucidate the role of APOA1 in GC would be highly valuable.
Introduction
Gastric cancer (GC) is the fifth most common malignant tumor worldwide, with over one million new cases estimated annually. In this article, the term ‘gastric cancer’ specifically refers to its most prevalent histological type—adenocarcinoma. The diagnosis of GC is often made at an advanced stage, resulting in high mortality rates and making it the third leading cause of cancer-related deaths. The 5-year survival rate for advanced GC patients is as low as 18% (1,2). Thus, prognosis significantly impacts the survival and quality of life for patients. Due to the insidious onset and rapid progression of GC, most patients are already in advanced stages at the time of diagnosis. For confirmed advanced GC, chemotherapy is the standard treatment, but the median overall survival (OS) with conventional chemotherapy is only around eight months (3). Therefore, early diagnosis and treatment of GC are crucial for improving prognosis. Currently, early detection of GC primarily relies on endoscopic examinations. However, limitations exist regarding the coverage of endoscopy in different countries, and early GC may lack characteristic features under endoscopy, leading to the possibility of missed diagnoses even by experienced physicians. Therefore, the search for simple and feasible GC-specific or prognostic biomarkers is of great importance (4,5).
With the help of bioinformatics, we have identified a series of genes that can serve as diagnostic or prognostic biomarkers and therapeutic targets for GC (6,7). However, most of these biomarkers have not been extensively validated or are associated with complex and costly detection methods, which limits their clinical applicability. Therefore, it is crucial to identify novel markers that are readily accessible in clinical settings and can accurately predict prognosis for GC patients.
In recent years, the role of lipid metabolism in cancer has been extensively explored. Tumor cells alter lipid metabolism to meet their needs for growth and metastasis (8). Specifically, alterations in lipid metabolism can promote tumor cell growth, invasion, and metastasis, and regulate the characteristics of the tumor microenvironment through intracellular signaling pathways (9,10). Changes in lipid metabolism have been confirmed to be associated with tumor growth and treatment response in various cancers, including colorectal cancer, pancreatic cancer, and prostate cancer (11-13). However, the alterations in lipid metabolism in GC and whether these changes can serve as prognostic biomarkers have not been fully investigated. In this study, we aim to analyze differentially expressed lipid metabolism-related genes between GC and normal patients by using bioinformatics analysis of The Cancer Genome Atlas (TCGA) database. We analyze the pathways to elucidate the reprogramming of lipid metabolism pathways in GC. Subsequently, we screen prognostic lipid metabolism-related genes by intersecting significantly upregulated genes and prognosis-related genes. By integrating bioinformatics analysis and clinical data collection, we validate the significance of the selected biomarkers in diagnosing and predicting prognosis in GC. Furthermore, by collecting clinical data from GC patients, we further validate the diagnostic and prognostic predictive power of the selected biomarkers. Through the integration of bioinformatics and clinical data, our study aims to provide a deeper understanding for early diagnosis and prognosis prediction in GC. We present this article in accordance with the REMARK reporting checklist (available at https://tcr.amegroups.com/article/view/10.21037/tcr-23-1966/rc).
Methods
Data collection and processing
TCGA (https://portal.gdc.cancer.gov/) is currently the largest cancer genomics database (14). We downloaded transcriptomic and corresponding clinical data from 375 GC patients and 32 healthy controls from the TCGA database.
Lipid metabolism-related gene acquisition
We obtained a dataset containing lipid metabolism-related genes from the Gene Set Enrichment Analysis (GSEA) website (15) (https://www.gsea-msigdb.org/). The datasets downloaded included HALLMARK_FATTY_ACID_METABOLISM, REACTOME_METABOLISM_OF_LIPIDS, and Kyoto Encyclopedia of Genes and Genomes (KEGG) GLYCEROPHOSPHOLIPID_META-BOLISM. By taking the intersection of these datasets, a total of 861 lipid metabolism-related genes were collected.
Differential gene analysis
We performed differential gene expression analysis using the R language and the “limma” package. This analysis helped us identify statistically significant differentially expressed genes.
Gene functional enrichment analysis
We imported the differentially expressed genes into the Metascape database (https://metascape.org/) for gene enrichment analysis, including molecular functions (MF), biological processes (BP), cellular components (CC), and KEGG pathway analysis (16,17).
Immune infiltration analysis
We evaluated the immune infiltration status in GC based on the immune infiltration data available in the TCGA database. We utilized the CIBERSORT tool (18) to analyze the immune infiltration status. Specifically, we compared and analyzed the differences in immune cell infiltration between the APOA1 low-expression group and the high-expression group.
Protein expression analysis
We utilized the Human Protein Atlas (HPA) database (https://www.proteinatlas.org/), an open-source database containing information on human proteins discovered in normal and cancer tissues (19). In our study, we employed the protein expression module of HPA to examine the protein levels of APOA1 in normal gastric tissue and GC tissue. Specifically, we assessed and compared the expression levels of APOA1 protein in both types of tissues.
Clinical data collection
We collected clinical data from 2,741 GC patients treated at The Second Hospital of Dalian Medical University between 2012 and 2023. Additionally, clinical data from 813 patients with chronic gastritis were also included. Under the criteria that included: patients diagnosed with GC or chronic gastritis, with no restrictions on age or gender, who had not undergone chemotherapy or radiotherapy, had no history of other tumors, had complete clinical records. The study was conducted in accordance with the Declaration of Helsinki (as revised in 2013). The study was approved by the Ethics Committee of The Second Hospital of Dalian Medical University (No. 2023-045). Informed consent was taken from all the patients. Patients were excluded if they had a history of chemotherapy or radiotherapy, were diagnosed with fatty liver or hyperlipidemia, had severe cardiovascular diseases, cirrhosis, or other malignancies, or if their clinical data were incomplete. The data were obtained from electronic medical records and included information such as gender, age at diagnosis, tumor grade, tumor location, and lipid profile results. Additionally, the data encompassed the TNM classification of malignant tumors, where “T” stands for the size and extent of the main tumor, “N” represents the number of nearby lymph nodes that have cancer, and “M” indicates whether cancer has metastasized. This classification system, known as the TNM staging system, provides a detailed overview of cancer progression.
APOA1 testing
On the morning after admission, fasting venous blood samples of 10 mL were collected from the patients and transferred into anticoagulant tubes. The blood lipid profile, including triglycerides, cholesterol, high-density lipoprotein (HDL), low-density lipoprotein (LDL), apolipoprotein A-I (APOA1), and apolipoprotein B (APOB), was measured. The blood lipid analysis was performed using the SIEMENS ADVIA2400 instrument (Siemens Healthcare, Erlangen, Germany).
Statistical analysis
Independent sample t-tests were conducted to analyze quantitative variables. The receiver operating characteristic (ROC) curve analysis and Kaplan-Meier survival analysis were used to assess the effectiveness of R software in predicting survival outcomes. Cox proportional hazards models were employed to examine the associations among prognostic classifications, survival outcomes, and other clinical parameters. Results with a P value less than 0.05 were considered statistically significant.
Results
Analysis of lipid metabolism-related gene expression and functional enrichment in GC and control groups
In this study, we extracted data from 375 GC patients and 32 healthy control individuals from the TCGA database. By referring to the GSEA database, we identified 861 lipid metabolism-related genes expressed in patients with stomach adenocarcinoma (STAD) as recorded in TCGA. Using the limma package in R, we analyzed the differential expression of lipid metabolism genes between GC and control patients. Compared to the control group, we identified 357 upregulated lipid metabolism genes and 185 downregulated lipid metabolism genes (P<0.05) (Figure 1A,1B). Subsequently, we performed functional enrichment analysis of the differentially expressed genes using the Metascape website. The enrichment analysis revealed that the upregulated genes were associated with glycerophospholipid metabolism, oxidoreductase activity acting on the carbon-hydrogen (CH-CH) double bond group of donors, and sphingolipid metabolic process (Figure 1C-1E), while the downregulated genes were associated with lipid catabolic process and lipid modification (Figure 1F-1H).
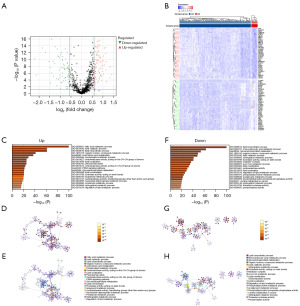
Identification of prognosis-associated lipid metabolism genes
By performing univariate Cox regression analysis, a total of 58 lipid metabolism genes were identified as prognostic markers for GC (Figure 2A). Intersection analysis with significantly differentially expressed lipid metabolism genes [|log fold change| >2, P<0.05] led to the selection of one significantly prognostic lipid metabolism gene, APOA1 (Figure 2B). Validation of APOA1 gene expression levels in GC and control patients was performed using the GEPIA database, which showed a downregulation of APOA1 gene expression in GC samples compared to normal samples (P<0.05, Figure 2C). Analysis of APOA1 protein expression in GC and normal tissues was conducted using the HPA website, revealing weak positive staining of APOA1 in normal tissues (HPA046715 antibody) and negative staining in GC tissues (Figure 2D,2E). Subsequently, TCGA-GC patients were divided into low-expression and high-expression groups based on APOA1 expression levels, using the optimal cutoff value. Kaplan-Meier analysis consistently demonstrated worse prognosis in the APOA1 high-expression group (Figure 2F). Furthermore, the predictive ability for 1-year survival was assessed using ROC analysis. The model showed a good area under the curve (AUC) value of 0.64 (P<0.001) for 1-year survival based on TCGA data (Figure 2G). These findings indicate that APOA1 serves as a prognostic lipid metabolism gene marker and can be used to predict the occurrence and prognosis of GC.
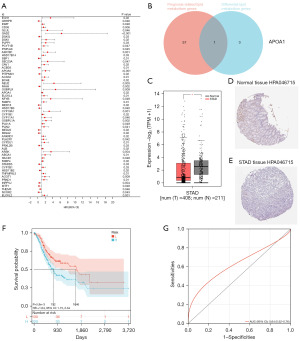
Expression and prognostic value of APOA1 in pan-cancer
Figure 3 illustrates the expression pattern of APOA1 gene in pan-cancer tissues. APOA1 gene exhibits decreased expression in lung adenocarcinoma (LUAD), lung squamous cell carcinoma (LUSC), STAD, testicular germ cell tumors (TGCT), and thyroid carcinoma (THCA), while it shows increased expression in ovarian serous cystadenocarcinoma (OV). These findings suggest that APOA1 has strong tumor specificity and can serve as a highly specific tumor biomarker.
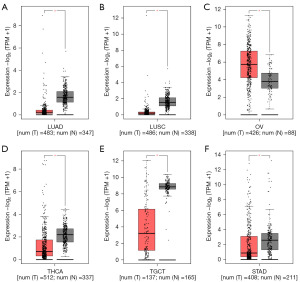
Correlation of APOA1 expression with clinical features
Our study results showed that the expression of APOA1 mRNA did not differ across different age groups (Figure 4A), genders (Figure 4B), and TNM stages (Figure 4C-4E). However, in terms of tumor grade, patients with G2 grade exhibited higher APOA1 expression compared to those with G3 grade (Figure 4F, P<0.01). This suggests a possible correlation between APOA1 expression and the malignancy grade of GC.
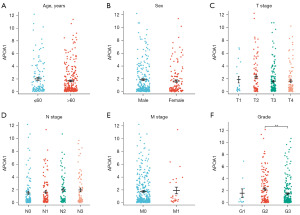
Correlation between APOA1 expression and immune infiltration levels in GC
The impact of APOA1 on immune infiltration in GC was further evaluated. As shown in Figure 5A, the APOA1 high-expression group exhibited higher expression of type II interferon (IFN) response, while the APOA1 low-expression group showed higher expression of antigen-presenting cell (APC) co-inhibition, APC co-stimulation, cytolytic activity, major histocompatibility complex (MHC) class I, and T cell co-inhibition. Additionally, we investigated the mRNA expression levels of APOA1 in 375 cancer tissues using the CIBERSORT database. The APOA1 high-expression group demonstrated higher expression in B cells naive, plasma cells, T cells CD4 memory resting, monocytes, and mast cells resting, while the APOA1 low-expression group exhibited higher expression in T cells CD4 memory activated and natural killer (NK) cells resting (Figure 5B).
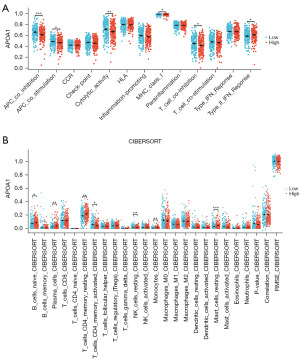
Study of serum APOA1 protein levels in GC patients
We collected clinical data from 2,741 GC patients and 813 gastritis control patients at The Second Hospital of Dalian Medical University to investigate the expression and prognostic significance of serum APOA1 protein levels in GC patients. The study results revealed that compared to gastritis patients, GC patients had significantly decreased levels of serum APOA1 protein (1.38 vs. 1.26, P<0.05) (Figure 6A), and it showed diagnostic potential for predicting GC occurrence [AUC: 0.63, 95% confidence interval (CI): 0.61–0.65] (Figure 6B). Furthermore, Kaplan-Meier analysis demonstrated that among the 2,741 GC patients, those with high serum APOA1 expression had a worse prognosis [hazard ratio (HR) =1.50, P<0.001] (Figure 6C). Additionally, ROC analysis of serum APOA1 protein levels for predicting 1-, 3-, and 5-year survival rates in GC patients showed good diagnostic performance: 1-year (AUC: 0.59, 95% CI: 0.56–0.63), 3-year (AUC: 0.59, 95% CI: 0.56–0.62), and 5-year (AUC: 0.60, 95% CI: 0.57–0.63) (Figure 6D). Furthermore, serum APOA1 protein levels showed a significant association with various clinical features (Figure 7). The older age group (>60 years), female patients, T4 stage, N3 stage, M1 stage, and G3 grade exhibited lower APOA1 expression (P<0.05). We further validated the impact of GC treatment on serum APOA1 expression, and the results indicated a significant increase in serum APOA1 expression after treatment compared to the pre-treatment expression, further highlighting the close association between serum APOA1 expression and GC disease itself (Figure 8).
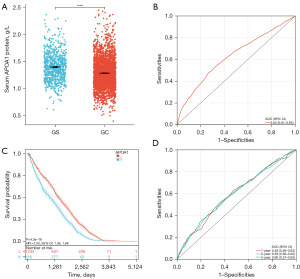
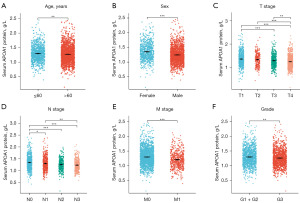
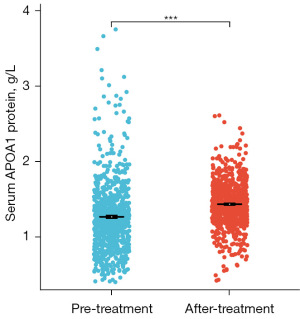
Discussion
Lipids are essential for energy storage, membrane proliferation, and the generation of signaling molecules. Currently, alterations in lipid metabolism in cancer cells are receiving increasing attention and recognition. There is mounting evidence that cancer cells often exhibit characteristic changes in lipid metabolism, including uptake, synthesis, storage, and breakdown of lipids (20,21). Our study revealed 357 upregulated and 185 downregulated lipid metabolism genes in GC patients compared to normal individuals, indicating lipid metabolism reprogramming in GC. Subsequent enrichment analysis of differentially expressed genes revealed that upregulated genes were associated with phospholipid metabolism, glycerophospholipid metabolism, and lipid biosynthesis, while downregulated genes were associated with lipid degradation and lipid modification. The dysregulation of the lipid metabolism pathway may constitute a significant contributing factor in the development and progression of GC. Similar findings have been observed in studies on other tumors, where FA oxidation-related pathways, such as phospholipids and glycerolipids, showed significant upregulation, while FA β-oxidation and FA metabolism exhibited significant downregulation in tumor tissues (21). This is consistent with our research findings. Chi et al. found that GC patients had upregulated expression of FA synthase (FASN), downregulation of lipid acidification and β-oxidation-related enzymes, leading to increased lipid synthesis and accumulation of lipid droplets in GC cells. These changes were shown to promote proliferation and metastasis of GC cells (22).
Recent findings underscore the crucial role of APOA1 in lipid metabolism and its significant impact on GC progression. Exploiting drugs to boost APOA1 synthesis emerges as a strategic approach to positively adjust lipid profiles, potentially modulating the tumor microenvironment to slow or halt cancer progression. This approach exemplifies the synergy between pharmacological advances and dietary interventions, aiming to enhance the effectiveness of GC treatments. This strategy not only addresses individual patient needs but also paves the way for more personalized and effective therapies (23,24).
Prognostic biomarkers in GC have been extensively studied, and previous study has identified genes such as c-MET, VEGF, PIK3CA, PTEN, and HER2 as potential GC markers (25). However, the diagnostic and prognostic role of lipid metabolism-related genes in GC has not been investigated. In our study, we selected significantly differentially expressed genes and prognostic-related genes in GC and identified one significantly differentially expressed prognostic-related lipid metabolism gene, APOA1, through the intersection of these gene sets. We found that both the APOA1 gene and protein were downregulated in GC. Kaplan-Meier analysis revealed that patients with high APOA1 expression had worse OS compared to those with low expression. Furthermore, we assessed the 1-year predictive ability based on the ROC curve, and the AUC value calculated from TCGA data for 1-year survival was 0.64. These results suggest that APOA1 can serve as a prognostic lipid metabolism-related gene biomarker for predicting the occurrence and prognosis of GC.
APOA1 is a commonly used lipid indicator and possesses various biological activities, including anti-inflammatory, antioxidant, and anticoagulant effects, playing important roles in numerous BP (26). Previous studies (27,28) found that mice with GC exhibited lower plasma levels of APOA1, suggesting that the growth of malignant tumors in the stomach can lead to a decrease in plasma APOA1 protein levels in mice. The deficiency or reduction of APOA1 can result in the accumulation of cholesterol in tissues, and cancer cells often show increased cholesterol synthesis, possibly due to the high cellular demand for cholesterol to support rapid proliferation and cell membrane synthesis, thereby promoting tumor growth. Gumilas et al. (29) highlighted the potential mechanistic role of APOA1 in liver cirrhosis and hepatocellular carcinoma (HCC), stating that the primary biological function of APOA1 is to facilitate reverse cholesterol transport, transporting excess cholesterol from peripheral tissues to the liver for metabolism. Additionally, APOA1 possesses various biological properties such as anti-inflammatory, antioxidant, and immune-modulatory effects. Therefore, the authors proposed that a decrease in APOA1 levels could lead to chronic inflammation and metabolic abnormalities, thereby promoting tumorigenesis and tumor development. Furthermore, APOA1 can influence tumor development through the inhibition of inflammatory responses and regulation of cellular signaling pathways. This may involve multiple mechanisms, including the modulation of cell proliferation, apoptosis, angiogenesis, and the tumor microenvironment. APOA1 has also been shown to be associated with tumor prognosis in other cancers such as lung cancer, colorectal cancer, prostate cancer, and kidney cancer (30-33).
Immune response plays a significant role in the development and prognosis of GC. Previous study has indicated that the infiltration of CD8+ T cells, B cells, macrophages, and dendritic cells is closely associated with the prognosis of GC patients. The infiltration of CD8+ T cells and macrophages is positively correlated with patient survival, while the infiltration of B cells and dendritic cells is negatively correlated with patient survival (34). Previous research has also demonstrated that APOA1 can inhibit monocyte recruitment and macrophage chemotaxis in renal cancer (35). Elevated levels of APOA1 may inhibit tumor growth and metastasis by suppressing inflammation and promoting immune function. Peng et al. (36) investigated the role of APOA1 in pancreatic cancer in mice. The study found that APOA1 improves the tumor microenvironment by inhibiting inflammation and reducing oxidative stress, thereby suppressing tumor growth and metastasis. Additionally, other mechanisms of APOA1 have been mentioned in the literature. For example, APOA1 can enhance the phagocytic activity of macrophages, increasing the efficiency of tumor cell clearance. APOA1 can also inhibit tumor cell proliferation and induce tumor cell apoptosis, thereby suppressing tumor growth and metastasis. In order to explore whether APOA1 can affect immune infiltration in GC, we evaluated the immune infiltration in GC patients with high and low APOA1 expression. The results showed that patients with high APOA1 expression had higher expression of genes related to type II IFN response, while genes related to APC co-inhibition, APO co-stimulation, cytolytic activity, MHC class I, and T cell co-inhibition had lower expression. Type II IFN response is an immune response primarily regulated by IFN-γ. IFN-γ is a cytokine secreted by various immune cells such as T cells, natural killer cells, and macrophages, and it regulates multiple aspects of immune cell proliferation, differentiation, secretion, and activity. On the other hand, overexpression of genes related to APC co-inhibition and T cell co-inhibition can lead to T cell functional suppression. Cytolytic activity is involved in recognizing and killing infected cells and tumor cells. These results suggest that APOA1 may affect the prognosis of GC patients through the infiltration of negative immune cells.
Not only in cancer tissues but also in the serum of patients, a decrease in APOA1 protein levels has been observed, such as in patients with renal cell carcinoma (35). A large meta-analysis also demonstrated a specific negative correlation between serum APOA1 levels and survival rates in breast cancer, lung cancer, colorectal cancer, ovarian cancer, nasopharyngeal carcinoma, and renal cell carcinoma (37). In this study, we analyzed the differential expression of serum APOA1 between GC patients and healthy individuals, as well as its impact on the prognosis of GC patients. We observed that serum APOA1 protein levels were significantly lower in GC patients compared to the chronic gastritis group (P<0.001). Kaplan-Meier analysis showed that patients with high serum APOA1 expression had a worse prognosis among the 2,741 GC patients (HR =1.50, P<0.001). ROC analysis of serum APOA1 protein levels for predicting 1-, 3-, and 5-year survival rates also demonstrated good diagnostic ability. Serum APOA1 expression was closely associated with clinical characteristics of the patients, such as age over 60, female gender, T4 stage, N3 stage, M1 stage, and G3 grade group, showing lower expression levels. We further investigated the impact of GC treatment on serum APOA1 expression and found that post-treatment serum APOA1 expression significantly increased compared to its expression before treatment, providing further evidence for the close association between serum APOA1 expression and GC occurrence. Based on our findings, we speculate that APOA1 may function as a potential tumor suppressor gene in GC. Given that APOA1 protein levels can be readily measured in plasma, changes in its expression could serve as a relevant biomarker for the diagnosis and prognosis of GC patients. A study has reported that lipid metabolism plays a significant role in GC, primarily associated with high-fat diet consumption and excessive lipid intake. Consuming a high-fat diet can lead to alterations in the gut microbiota, thereby affecting lipid metabolism. Additionally, cholesterol accumulation promotes tumor proliferation and invasion, while increased FA metabolism leads to enhanced FA synthesis and reduced FA oxidation, thereby impacting tumor growth and metastatic potential (38).
Our study has certain limitations that should be acknowledged. Firstly, we were unable to validate the clinical and prognostic significance of APOA1 mRNA in an independent dataset due to technical constraints. Secondly, our study primarily relied on bioinformatics analysis and clinical data, without conducting biomedical experiments to investigate the underlying mechanisms of APOA1 in GC progression and metastasis. Therefore, future investigations incorporating functional experiments to elucidate the role of APOA1 in GC would be highly valuable.
Conclusions
Our comprehensive study investigated the expression and prognostic significance of APOA1 mRNA and protein levels in GC. The protein level of APOA1 demonstrated excellent diagnostic capability. This finding contributes to improving the accuracy of prognosis prediction in GC patients and provides new insights for personalized treatment approaches.
Acknowledgments
Funding: This work received support from
Footnote
Reporting Checklist: The authors have completed the REMARK reporting checklist. Available at https://tcr.amegroups.com/article/view/10.21037/tcr-23-1966/rc
Peer Review File: Available at https://tcr.amegroups.com/article/view/10.21037/tcr-23-1966/prf
Conflicts of Interest: All authors have completed the ICMJE uniform disclosure form (available at https://tcr.amegroups.com/article/view/10.21037/tcr-23-1966/coif). The authors have no conflicts of interest to declare.
Ethical Statement: The authors are accountable for all aspects of the work in ensuring that questions related to the accuracy or integrity of any part of the work are appropriately investigated and resolved. This study was conducted in accordance with the Declaration of Helsinki (as revised in 2013). The study was approved by the Ethics Committee of The Second Hospital of Dalian Medical University (No. 2023-045). Informed consent was taken from all the patients.
Open Access Statement: This is an Open Access article distributed in accordance with the Creative Commons Attribution-NonCommercial-NoDerivs 4.0 International License (CC BY-NC-ND 4.0), which permits the non-commercial replication and distribution of the article with the strict proviso that no changes or edits are made and the original work is properly cited (including links to both the formal publication through the relevant DOI and the license). See: https://creativecommons.org/licenses/by-nc-nd/4.0/.
References
- Smyth EC, Nilsson M, Grabsch HI, et al. Gastric cancer. Lancet 2020;396:635-48. [Crossref] [PubMed]
- Sexton RE, Al Hallak MN, Diab M, et al. Gastric cancer: a comprehensive review of current and future treatment strategies. Cancer Metastasis Rev 2020;39:1179-203. [Crossref] [PubMed]
- Li K, Zhang A, Li X, et al. Advances in clinical immunotherapy for gastric cancer. Biochim Biophys Acta Rev Cancer 2021;1876:188615. [Crossref] [PubMed]
- Joshi SS, Badgwell BD. Current treatment and recent progress in gastric cancer. CA Cancer J Clin 2021;71:264-79. [Crossref] [PubMed]
- Battista S, Ambrosio MR, Limarzi F, et al. Molecular Alterations in Gastric Preneoplastic Lesions and Early Gastric Cancer. Int J Mol Sci 2021;22:6652. [Crossref] [PubMed]
- Lei Z, Tan IB, Das K, et al. Identification of molecular subtypes of gastric cancer with different responses to PI3-kinase inhibitors and 5-fluorouracil. Gastroenterology 2013;145:554-65. [Crossref] [PubMed]
- Cristescu R, Lee J, Nebozhyn M, et al. Molecular analysis of gastric cancer identifies subtypes associated with distinct clinical outcomes. Nat Med 2015;21:449-56. [Crossref] [PubMed]
- Li S, Zeng H, Fan J, et al. Glutamine metabolism in breast cancer and possible therapeutic targets. Biochem Pharmacol 2023;210:115464. [Crossref] [PubMed]
- Zhang M, Wei T, Zhang X, et al. Targeting lipid metabolism reprogramming of immunocytes in response to the tumor microenvironment stressor: A potential approach for tumor therapy. Front Immunol 2022;13:937406. [Crossref] [PubMed]
- Mukherjee A, Bilecz AJ, Lengyel E. The adipocyte microenvironment and cancer. Cancer Metastasis Rev 2022;41:575-87. [Crossref] [PubMed]
- Baxter BA, Parker KD, Nosler MJ, et al. Metabolite profile comparisons between ascending and descending colon tissue in healthy adults. World J Gastroenterol 2020;26:335-52. [Crossref] [PubMed]
- Bai R, Rebelo A, Kleeff J, et al. Identification of prognostic lipid droplet-associated genes in pancreatic cancer patients via bioinformatics analysis. Lipids Health Dis 2021;20:58. [Crossref] [PubMed]
- Scheinberg T, Mak B, Butler L, et al. Targeting lipid metabolism in metastatic prostate cancer. Ther Adv Med Oncol 2023;15:17588359231152839. [Crossref] [PubMed]
- Jiang P, Sinha S, Aldape K, et al. Big data in basic and translational cancer research. Nat Rev Cancer 2022;22:625-39. [Crossref] [PubMed]
- Shang Y, Zhang Y, Liu J, et al. Decreased E2F2 Expression Correlates with Poor Prognosis and Immune Infiltrates in Patients with Colorectal Cancer. J Cancer 2022;13:653-68. [Crossref] [PubMed]
- Yue T, Chen S, Zhu J, et al. The aging-related risk signature in colorectal cancer. Aging (Albany NY) 2021;13:7330-49. [Crossref] [PubMed]
- Chen L, Zhang YH, Lu G, et al. Analysis of cancer-related lncRNAs using gene ontology and KEGG pathways. Artif Intell Med 2017;76:27-36. [Crossref] [PubMed]
- Wang H, Lu L, Liang X, et al. Identification of prognostic genes in the pancreatic adenocarcinoma immune microenvironment by integrated bioinformatics analysis. Cancer Immunol Immunother 2022;71:1757-69. [Crossref] [PubMed]
- Liang X, Li L, Fan Y. Diagnostic, Prognostic, and Immunological Roles of HELLS in Pan-Cancer: A Bioinformatics Analysis. Front Immunol 2022;13:870726. [Crossref] [PubMed]
- Yang K, Wang X, Song C, et al. The role of lipid metabolic reprogramming in tumor microenvironment. Theranostics 2023;13:1774-808. [Crossref] [PubMed]
- Xiong Z, Lin Y, Yu Y, et al. Exploration of Lipid Metabolism in Gastric Cancer: A Novel Prognostic Genes Expression Profile. Front Oncol 2021;11:712746. [Crossref] [PubMed]
- Chi Y, Wang H, Wang F, et al. PHTF2 regulates lipids metabolism in gastric cancer. Aging (Albany NY) 2020;12:6600-10. [Crossref] [PubMed]
- Georgila K, Vyrla D, Drakos E. Apolipoprotein A-I (ApoA-I), Immunity, Inflammation and Cancer. Cancers (Basel) 2019;11:1097. [Crossref] [PubMed]
- Delk SC, Chattopadhyay A, Escola-Gil JC, et al. Apolipoprotein mimetics in cancer. Semin Cancer Biol 2021;73:158-68. [Crossref] [PubMed]
- Matsuoka T, Yashiro M. Biomarkers of gastric cancer: Current topics and future perspective. World J Gastroenterol 2018;24:2818-32. [Crossref] [PubMed]
- Cochran BJ, Ong KL, Manandhar B, et al. APOA1: a Protein with Multiple Therapeutic Functions. Curr Atheroscler Rep 2021;23:11. [Crossref] [PubMed]
- Chong PK, Lee H, Zhou J, et al. Reduced plasma APOA1 level is associated with gastric tumor growth in MKN45 mouse xenograft model. J Proteomics 2010;73:1632-40. [Crossref] [PubMed]
- Huang B, Song BL, Xu C. Cholesterol metabolism in cancer: mechanisms and therapeutic opportunities. Nat Metab 2020;2:132-41. [Crossref] [PubMed]
- Gumilas NSA, Harini IM, Ernawati DA, et al. Potential of Apolipoprotein A1 (ApoA1) for Detecting Liver Cirrhosis and Hepatocellular Carcinoma. Asian Pac J Cancer Prev 2022;23:2001-8. [Crossref] [PubMed]
- Shi H, Huang H, Pu J, et al. Decreased pretherapy serum apolipoprotein A-I is associated with extent of metastasis and poor prognosis of non-small-cell lung cancer. Onco Targets Ther 2018;11:6995-7003. [Crossref] [PubMed]
- Guo SP, Chen C, Zeng ZF, et al. Serum Apolipoprotein A-I Predicts Response of Rectal Cancer to Neoadjuvant Chemoradiotherapy. Cancer Manag Res 2021;13:2623-31. [Crossref] [PubMed]
- Wang J, Xu LF, Liu C, et al. Identifying the role of apolipoprotein A-I in prostate cancer. Asian J Androl 2021;23:400-8. [Crossref] [PubMed]
- Liu Z, Xiao Y, Tang L, et al. Apolipoprotein A1 -75 G/A and +83 C/T polymorphisms and renal cancer risk. Lipids Health Dis 2015;14:143. [Crossref] [PubMed]
- Wang M, Li Z, Peng Y, et al. Identification of immune cells and mRNA associated with prognosis of gastric cancer. BMC Cancer 2020;20:206. [Crossref] [PubMed]
- Zeng W, Xiong G, Hua L, et al. APOA1 mRNA and protein in kidney renal clear cell carcinoma correlate with the disease outcome. Sci Rep 2022;12:12406. [Crossref] [PubMed]
- Peng M, Zhang Q, Liu Y, et al. Apolipoprotein A-I Mimetic Peptide L-4F Suppresses Granulocytic-Myeloid-Derived Suppressor Cells in Mouse Pancreatic Cancer. Front Pharmacol 2020;11:576. [Crossref] [PubMed]
- Wu J, Zhang C, Zhang G, et al. Association Between Pretreatment Serum Apolipoprotein A1 and Prognosis of Solid Tumors in Chinese Population: A Systematic Review and Meta-Analysis. Cell Physiol Biochem 2018;51:575-88. [Crossref] [PubMed]
- Cui MY, Yi X, Zhu DX, et al. The Role of Lipid Metabolism in Gastric Cancer. Front Oncol 2022;12:916661. [Crossref] [PubMed]