Tumour mutation burden and infiltrating immune cell subtypes influenced the breast cancer prognosis
Highlight box
Key findings
• We explored the immunogenicity of breast cancer by investigating the tumour mutation burden (TMB). There are seven prognostic immune-related genes between different TMB subgroups in breast cancer patients, and these genes deeply participate in the immune response of the body. And there is a correlation between the level of TMB and certain clinical and pathological features. TMB-related immunological infiltration characteristics showed meaningful value for prognosis prediction for breast cancer patients.
What is known and what is new?
• A higher TMB is thought to lead to an increase in tumour-specific epitopes, thereby facilitating immune recognition of tumour cells and resulting in better therapeutic outcomes. It has been shown that TMB can affect the immune microenvironment of tumours; that the prognostic importance of TMB and the relationship between TMB and infiltrating immune cells both vary by cancer type; and that high TMB is associated with prolonged survival and greater clinical benefit in patients who are treated with immune checkpoint inhibitors.
• Compared with low-TMB groups, the survival period of high-TMB group was relatively longer. In our study, we identified a total of 337 differentially expressed genes and seven were predictive of prognosis.
What is the implication, and what should change now?
• We suggest that infiltrating immune cells may be major players in anti-tumour immunity.
• The TMB-related immunization characteristics of this study may provide new biomarkers and potential treatment options for breast cancer.
Introduction
Breast cancer is the leading cause of cancer deaths in women (1-4). According to the 2020 Global Epidemiological Survey, breast cancer has overtaken lung cancer as the most common cancer among women, accounting for about 30% of all cancers in female patients, with a mortality-to-incidence ratio of 15% (3,5). The prognosis of breast cancer varies according to the complexity of the underlying molecular mechanisms, the degree of tumour heterogeneity, the degree of malignancy of the particular pathological type, and the stage of progression (6). Therefore, there is a need to develop reliable tools for assessing the prognosis and treatment outcomes of breast cancer patients to lay the foundation for future advances in precision medicine and personalised treatment.
The tumour immune microenvironment is composed of tumour-associated immune cells, fibroblasts, endothelial cells, and blood-derived cells that appear to be enriched during tumour progression. All of these cells play a role in tumourigenesis and tumour progression (7-9), and their mechanisms of action have become a research hotspot in recent years. Immunotherapies for cancer have now achieved remarkable success, with immune checkpoint blockade (ICB) therapy showing favorable clinical results in tumour patients with poor prognosis (10). Study has shown that a high tumour mutation burden (TMB) is among the factors most strongly associated with a good response to ICB (11). A higher TMB is thought to lead to an increase in tumour-specific epitopes (also known as neoantigens), thereby facilitating immune recognition of tumour cells and resulting in better therapeutic outcomes (11-13). It has been shown that TMB affects the immune microenvironment of tumours; that the prognostic importance of TMB and the relationship between TMB and infiltrating immune cells both vary by cancer type (14,15); and that high TMB is associated with prolonged survival and greater clinical benefit in patients with metastatic melanoma, non-small cell lung cancer, and colon cancer who are treated with immune checkpoint inhibitors (ICIs) (12,16-18). With the recent increase in biomarker-based immunotherapies, TMB has received increasing attention as a quantitative marker of tumour antigens. The present study sought to determine whether evaluating TMB can assist in the clinical treatment selection of breast cancer patients and ultimately improve clinical prognosis and survival.
In this study, we obtained data on mutations and transcriptional expression in breast cancer from The Cancer Genome Atlas (TCGA) database and used them to analyse the relationship between TMB and immune response, to explore the effects of the differential expression of immune-related genes (between high-TMB and low-TMB tumours) on patient survival, and to explore the relationship between TMB and infiltrating immune cells in the tumour microenvironment. We present this article in accordance with the TRIPOD reporting checklist (available at https://tcr.amegroups.com/article/view/10.21037/tcr-23-2195/rc).
Methods
Data selection and analysis
Data on somatic mutations in TCGA-BRCA (breast cancer susceptibility genes) cohort, consisting of 986 patients with breast cancer from 2013 to 2022, were downloaded from the Genomic Data Commons database (https://portal.gdc.cancer.gov). Gene expression profiles and corresponding clinicopathological data for 1,109 tumour samples and 113 samples of normal tissue were extracted from TCGA database (https://portal.gdc.cancer.gov/repository). The somatic mutation data were processed using VarScan software and characterised and visualised using the maftools package in R software (version 4.1.1). If there were missing expression profiles and clinical pathological data, the data were excluded. The study was conducted in accordance with the Declaration of Helsinki (as revised in 2013).
Construction and validation of TMB-related immune variables
TMB is defined as the total number of gene coding errors (base substitutions, insertions or deletions) per million bases detected in a cell (19). First, the TMB value in mutations/Mb was calculated for each sample as the total number of somatic mutations divided by the size of the sequenced region. The median TMB value was then used as the cut-off to divide breast cancer patients into low-TMB and high-TMB groups. Survival curves were plotted using the Kaplan-Meier method based on the clinical characteristics of each case, and the relationship between TMB and overall survival (OS) was analysed using the log-rank test. The Wilcoxon rank-sum test was applied to analyse the relationship between TMB and clinicopathological parameters, such as gender, age, Tumour-Node-Metastasis (TNM) stage, and immunohistochemical expression levels, in breast cancer patients.
Data on genes involved in immune activity were downloaded from the ImmPort database (https://www.immport.org/) and used together with the limma package in R to identify differentially expressed genes (DEGs) involved in immune function between high-TMB and low-TMB groups. Genes were identified as TMB-related DEGs if the absolute logarithmic fold change (|log FC|) was >1. Univariate and multivariate survival analyses based on DEGs were performed using Kaplan-Meier and Cox regression models. Finally, Tumour Immune Estimation Resource 2.0 (TIMER 2.0) and the Gene Expression Profiling Interactive Analysis (GEPIA) database were used to validate statistically significant relationships between immune-related DEGs and OS.
Gene set enrichment analysis
The gene transcription data were compared between high-TMB and low-TMB groups, as per the median TMB. Gene set enrichment analysis was performed using Kyoto Encyclopedia of Genes and Genomes (KEGG) and Gene Ontology (GO) analyses, with 1,000 random combinations. Enriched gene sets were considered clinically significant when the normalised enrichment score was >1, the normalised significance level (nominal P value) was <0.05, and multiple hypothesis testing returned a q value [P value adjusted for false discovery rate (FDR)] <0.25. The R packages clusterProfiler, org.Hs.eg.db, plot, and ggplot2 were used to analyse the molecular functions (MFs) of the DEGs (20).
Relationship between TMB and infiltrating immune cells
RNA sequencing transcriptomic data from breast cancer cases in TCGA database were analysed with the CIBERSORT package in R to illuminate the relationship between molecular expression and the abundance of the infiltrating immune cells. This algorithm employs inverse convolution to calculate the abundance of 22 types of tumour-infiltrating immune cells (21); its accuracy was validated by flow cytometry experiments. The difference in the expression of immune cells was considered statistically significant. The population of immune cells in each breast cancer patient was visualised using the pheatmap package in R. The Wilcoxon rank-sum test was applied to analyse the difference in the proportion of infiltrating immune cells between the high-TMB and low-TMB groups, and the data were visualised using the vioplot package (21). The abundance of CD8+ T cells, CD4+ T cells, regulatory T cells, macrophages, and natural killer cells was compared between the two groups.
Statistical analysis
SPSS 25.0 and Graphpad 8.0.1 software were used to generate Kaplan-Meier survival curves for the interactions of high and low TMB with survival status and duration, and the log-rank test was used to compare the curves. One-way analysis of variance (one-way ANOVA), Tukey’s multiple comparison test and the unpaired two-tailed t-test were used to analyse correlations between clinicopathological characteristics and TMB. Univariate and multivariate Cox regression analyses were performed to determine the effects of immune-related DEGs on prognosis. The unpaired t-test was used to assess differences in the populations of infiltrating immune cells between the high-TMB and low-TMB groups. The specific criteria for identifying clinical significance were q (FDR-adjusted P value) <0.01 and |log FC| >1, with P<0.05 considered statistically significant.
Results
Somatic mutations in breast cancer cases
Of the 986 TCGA breast cancer cases, mutations were present in 899 (91.18%) (Figure 1A), with the number ranging from 0 to 4,962 (median 39). The commonest mutation types were missense mutations, nonsense mutations, and single nucleotide polymorphisms (SNPs), with the most frequent single nucleotide variant (SNV) class being C>T (Figure 1A-1F). According to the waterfall plot, the top 10 genes with the highest mutation rates were TP53, PIK3CA, TTN, CDH1, GATA3, MUC16, KMT2C, MAP3K1, RYR2 and HMCN1 (Figure 1G).
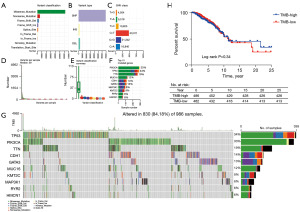
Survival prognosis and clinical characteristics of different TMB subgroups of breast cancer patients
TMB values were calculated for each breast cancer case of the downloaded data, and cases were divided into high-TMB (n=495) and low-TMB groups (n=491) based on the median TMB (1.34 mutations/Mb; range, 0.03–158.39 mutations/Mb). Eighteen cases were excluded from analysis due to a lack of corresponding data on clinical characteristics and survival, leaving 968 cases for the final analysis. Kaplan-Meier survival curves were plotted to determine whether TMB predicted survival, with the result that OS was longer among high-TMB patients than that of the low-TMB patients, although the difference was not statistically significant (χ2=0.926, P=0.34) (Figure 1H). At all follow-up points, the survival rate was lower in the low-TMB group than in the high-TMB group (92.12% vs. 95.47% after 3 years; 89.63% vs. 93.00% after 5 years; 86.38% vs. 88.27% after 10 years; 85.89% vs. 88.07% after 15 years). The longer survival observed in the high-TMB group suggests that immunotherapy may be more effective for breast cancer patients with a comparatively higher TMB.
After removing confounding variables, clinical characteristics were also compared between the high-TMB and low-TMB groups. There were statistically significant differences in age (above 50 vs. below 50) and tumour stage (T and N) (Figure 2A-2C), but there was no significant correlation with tumour proliferation index. Further analysis showed that high TMB positively correlated with older age and more advanced T stage (P<0.001, r=0.261 and 0.359), there is a weak correlation between the expression level of TMB and the older the age and the higher T stage of tumour. But negatively correlated with N stage (the number of lymph node metastases) (P=0.004, r=0.367), and it also shows a weak correlation. With the in-depth understanding of the molecular mechanism of breast cancer, the diagnosis and treatment of breast cancer has entered the era of molecular typing and precise treatment. The expression levels of estrogen receptor (ER), progesterone receptor (PR), human epidermal growth factor receptor 2 (HER2) and Ki-67 index in breast cancer are crucial for treatment. The results of this study show that TMB was also correlated with ER status and molecular subtype, with high-TMB cases generally being ER-negative and low-TMB cases tending to be luminal-type (Figure 2D,2E). In contrast, TMB were not significantly correlated with HER2 status, clinical stage and Ki-67 index (Figure 2F-2H).
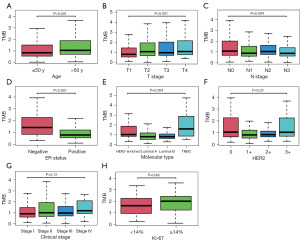
Analysis of the immune-related DEGs in TMB subgroups and construction of the prognosis signature
DEGs between the high-TMB and low-TMB groups were analysed using the limma package in R and visualised with the pheatmap package. There were a total of 337 DEGs between the TMB groups, of which 193 were highly expressed in the high-TMB group and 144 were highly expressed in the low-TMB group (Figure 3A). All DEGs were checked against a dataset of 2,483 immune-related genes from the ImmPort database, and 54 of the DEGs were identified as immune-related (Figure 3B). Next, patient survival was analysed according to the differential expression of these immune-related genes. Univariate survival analysis revealed seven immune-related DEGs that were strongly associated with prognosis. Further multivariate Cox regression analysis revealed that S100A8, CXCL5, TMSB15A, and PTGER3 all independently affected OS (Table 1). High expression of TMSB15A was associated with a shorter survival duration, whereas high expression of the other six genes (FABP7, S100A8, CXCL5, PTGER3, CXCL14 and PGR) was associated with longer survival (Figure 3C-3I). The predictive utility of these genes was validated using TIMER 2.0 and the GEPIA database (Figure 4), confirming that high expression of FABP7, CXCL14 and PTGER3 and low expression of TMSB15A were correlated with longer OS, with all differences being statistically significant.
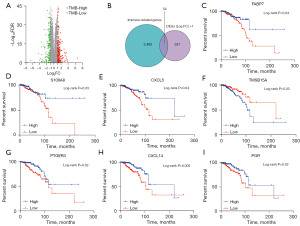
Table 1
Gene | Univariate analysis | Multivariate analysis | |||
---|---|---|---|---|---|
χ2 | P | HR (95% CI) | P | ||
FABP7 | 4.343 | 0.04* | 0.475 (0.125–1.808) | 0.28 | |
S100A8 | 4.654 | 0.03* | 0.07 (0.013–0.382) | 0.002* | |
CXCL5 | 4.348 | 0.04* | 0.138 (0.038–0.495) | 0.002* | |
TMSB15A | 4.852 | 0.03* | 5.599 (1.549–20.236) | 0.009* | |
PTGER3 | 5.942 | 0.02* | 0.238 (0.069–0.824) | 0.02* | |
CXCL14 | 7.961 | 0.005* | 0.445 (0.102–1.938) | 0.28 | |
PGR | 5.600 | 0.02* | 0.549 (0.107–2.831) | 0.47 |
*, P<0.05. DEGs, differentially expressed genes; HR, hazard ratio; CI, confidence interval.
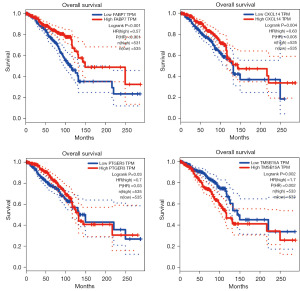
The Spearman’s correlation analysis indicated significant correlations between all the seven genes by using bc-GenExMiner database (P<0.001) (22). In addition, a significant moderate correlation was observed between PTGER3 and PGR (Figure 5A). By using GeneMANIA database, we observed strong association between CXCL5/14 and the most prominent weight of CXCL3, CXCL1 and CCL20 (Figure 5B). The molecular interaction network was then constructed for all the seven genes and other most frequently changed neighbor genes by using Integrated Interactions database (IID). We observed strong association between CXCL5 and CXCL14, but TMSB15A may not interact with the other six genes (Figure 5C).
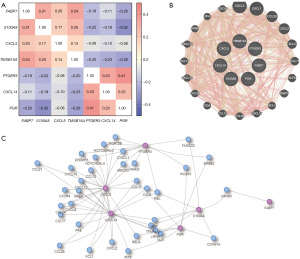
The limma package was further used to conduct gene set enrichment analysis of the identified DEGs. With respect to the GO terms, the main biological process (BP) terms identified were ‘cell chemotaxis’, ‘granulocyte migration’, and ‘humoral immune response’; the main cellular component (CC) terms included ‘secretory granule lumen’, ‘collagen-containing extracellular matrix’, and ‘vesicle lumen’; and the main MF terms were ‘receptor ligand activity’ and ‘G protein-coupled receptor binding’ (Figure 6A,6B). KEGG enrichment analysis showed that the most enriched pathways involving the DEGs included ‘cytokine-cytokine receptor interaction’, ‘IL-17 signaling’, and ‘viral protein interaction with cytokine and cytokine receptor’ (Figure 6C,6D). These results confirmed that the identified DEGs were deeply involved in the immune response.
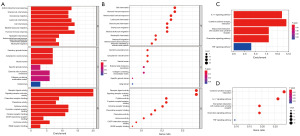
Relationship between TMB and infiltrating immune cells
The process of tumour mutation often results in the formation of more neoantigens, making the tumour more immunogenic. GO enrichment analysis suggested that the identified DEGs were involved in immune processes in the tumour microenvironment; however, numerous types of immune cells play a role in this environment. Hence, we used the CIBERSORT algorithm to compare the abundance of multiple immune cell types between the two TMB groups. First, we determined the overall abundance of 22 types of immune cells in breast cancer patients; the most abundant were T cells CD8+, T cells CD4+ naive, macrophages, plasma cells, and mast cells (Figure 7A). Next, we analysed the population of immune cells in 495 samples from the low-TMB group and 491 samples from the high-TMB group. Levels of activated CD4+ memory T cells, follicular helper T cells, activated dendritic cells and macrophages were higher in high-TMB tumours than in normal breast tissue, whereas proportions of memory B cells, resting dendritic cells and inactivated mast cells were higher in the low-TMB group; all differences were statistically significant (Figure 7B). However, no statistically significant correlations were observed between the numbers of these eight immune cell types and OS.
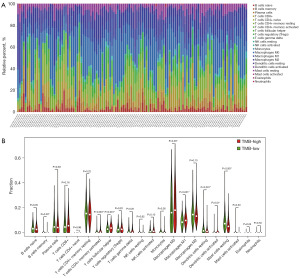
Discussion
In recent years, tumour treatment strategies have undergone a transformation due to breakthroughs in immunotherapy, with a shift from cellular to genetic biomarkers as prognostic tools. TMB is now recognised as an independent predictor of the efficacy of ICIs in various types of solid tumours (23), as well as having prognostic value for many cancer types, including metastatic melanoma, non-small cell lung cancer, colon cancer, bladder cancer, and ovarian cancer (16,17,24-26). With these advances in research, TMB and infiltrating immune cells have emerged as potential prognostic biomarkers for tumour treatment selection. Previous studies have shown that ICIs are effective in some triple-negative breast cancer patients, who typically present with high TMB and specific immune cell infiltration (27-29). It is therefore essential to explore the role played by TMB in all types of breast cancer and its relation to clinical prognosis.
In this study, we furthermore explored the immunogenicity of breast cancer by investigating the differences between high-TMB and low-TMB cases with respect to immune-related genes, infiltrating immune cells, key molecular pathways, and prognosis. We divided the cases from the TCGA database into high-TMB and low-TMB groups using the median TMB as the cut-off value. Although we can obtain more information when using TMB as a continuous variable for statistical analysis, there is currently no consensus on how to standardize the measurement of TMB and how to define TMB subgroups (15,30,31). As most relevant studies currently use TMB as a dichotomized variable for statistical analysis and TMB is a biomarker under study, therefore, this study still divides TMB into high-TMB and low-TMB groups based on the median (19,32,33). The results show that the 5-year survival rate was significantly higher in the high-TMB group than in the low-TMB group, and survival was better in the high-TMB group even without immunotherapy, which is consistent with the results of previous studies, although in our study the overall difference between groups was not statistically significant (28,34). The high-TMB group had a higher trend than the low-TMB group, which could provide more information for clinical treatment. Our study likewise demonstrates the potential of TMB as a prognostic marker for breast cancer patients. Various clinical studies have shown that high-TMB patients treated with ICIs have longer survival and higher response rates than low-TMB patients (23,24).
In this study, we analysed mutation data from 986 cases of breast cancer and identified the ten genes with the highest mutation rates (TP53, PIK3CA, TTN, CDH1, GATA3, MUC16, KMT2C, MAP3K1, RYR2, HMCN1); TP53 was the most commonly mutated gene in the cohort, which is consistent with data from previous study (35). TP53 mutations are uniquely associated with a strong anti-tumour immune response in several different tumour types. This is because they tend to result from a combination of changes in the degree of tumour aneuploidy leading to an increase in TMB. As breast cancer patients with TP53 mutations have a good immunotherapeutic response profile, these mutations may be suitable as prognostic biomarkers during therapy selection (36).
We also analysed the relationship between TMB and clinicopathological characteristics in breast cancer and found that higher TMB values were correlated with age, tumour T stage, and ER status. Patients with higher TMB expression tended to be older, with a high degree of tumour infiltration and fewer lymph node metastases, and were generally ER-negative. These results suggest that older patients with more advanced disease may benefit from ICIs. Further, the relationships between TMB and clinicopathological characteristics have rarely been reported in previous studies. Hence, these results may help clinicians predict the response of breast cancer patients to immunotherapy, pending in-depth investigation in future studies.
Previous study has reported that tumour expression of immune-related genes is closely associated with prognosis in cancer patients (37). This study identified a total of 337 DEGs between high-TMB and low-TMB cases of breast cancer (193 with high expression in the high-TMB group and 144 with high expression in the low-TMB group), of which 54 were related to immune function, including seven that were of prognosis predictive value. The predictive utility of these seven genes was validated against TIMER 2.0 and the GEPIA database, confirming that FABP7, PTGER3, CXCL14, and TMSB15A were all associated with higher or lower OS, consistent with previous studies (38-41). To investigate the MFs of these immunity-associated DEGs, we performed functional enrichment analysis of GO terms and KEGG pathways, which showed that they mainly played roles in cellular chemotaxis, granulocyte migration and humoral immunity, extracellular matrix and receptor ligand activity, and binding to G protein-coupled receptors; these results are consistent with previous reports (42).
Immune cells in the tumour immune microenvironment play an important role in tumour development, as they can antagonise or promote tumourigenesis (28). In tumour immunotherapy, enabling the body to build an effective immune barrier is the key to achieving the best therapeutic effect. Therefore, it is important to explore and understand the characteristics of the tumour immune microenvironment. In this study, we found a relationship between TMB and the abundance of different types of infiltrating immune cells. Analysis of levels of 22 types of immune cells in breast cancer showed that the most abundant types were CD8+ T cells, quiescent CD4+ T cells, naïve macrophages (M0), pro-inflammatory macrophages (M1), plasma cells, and mast cells. Compared with low-TMB patients, breast cancer patients with higher TMB had reduced levels of naïve B cells, memory B cells, plasma cells, quiescent CD4+ memory T cells, alternatively activated macrophages (M2), resting dendritic cells and quiescent mast cells. Our study suggests that infiltrating immune cells may be major players in anti-tumour immunity, resulting in greater sensitivity to immunotherapy and better therapeutic outcomes in breast cancer patients with certain infiltrating immune cell profiles.
On the whole, the relationship between TMB and clinical pathological characteristics has rarely reported in previous studies, and we have obtained immune-related genes from the ImmPort database, and analyzed the immune-related genes that differentiated between different TMB subgroups. These genes are correlated with the prognosis of patients, which may help clinicians predict the response of patients with breast cancer to immunotherapy, and we will verify these results in the future studies. However, our study still has some limitations. First, the results are based on TCGA dataset rather than laboratory experiments; future laboratory studies are needed to validate associations between prognostic factors and infiltrating immune cells in the tumour environment. Second, prospective data from a large clinical sample are needed to validate the prognostic role of immune-related DEGs in breast cancer patients. Finally, analysis of the function of immune-related DEGs in breast cancer progression can only indirectly address the potential relationship between infiltrating immune cells and immunotherapy resistance, and the immune-related genes identified as potential prognostic markers (particularly those involved in immune cell infiltration) require further experimental exploration to elucidate their function and mechanisms of action. Therefore, the results of this study should be further investigated in clinical trials with larger sample sizes.
Conclusions
TMB can cause significant changes in the ingredients of infiltrating immune cells in tumour microenvironments, and produce anti-tumour immune responses. In summary, TMB-related immunological infiltration characteristics in this study had a good predictive value in patients with breast cancer. There are immunological DEGs and varying degrees of immune cells infiltration between different TMB subgroups. They can provide important predictive information on the survival status of breast cancer patients, broad the idea of clinical diagnosis and treatment. Therefore, the TMB-related immunization characteristics of this study may provide new biomarkers and potential treatment options for breast cancer.
Acknowledgments
The authors thank the Department of Pathology, The Fourth Hospital of Hebei Medical University for its role in preparing the analysis tools and evaluating data in this study. The abstract of this article was presented at the 35th Annual European Congress of Pathology, Dublin, Ireland, 9–13 September, 2023.
We are grateful for the publicly available datasets that made this study possible. The data supporting our findings were generously provided by The Cancer Genome Atlas (TCGA) database, accessible at https://cancergenome.nih.gov/.
Funding: None.
Footnote
Reporting Checklist: The authors have completed the TRIPOD reporting checklist. Available at https://tcr.amegroups.com/article/view/10.21037/tcr-23-2195/rc
Peer Review File: Available at https://tcr.amegroups.com/article/view/10.21037/tcr-23-2195/prf
Conflicts of Interest: All authors have completed the ICMJE uniform disclosure form (available at https://tcr.amegroups.com/article/view/10.21037/tcr-23-2195/coif). The authors have no conflicts of interest to declare.
Ethical Statement: The authors are accountable for all aspects of the work in ensuring that questions related to the accuracy or integrity of any part of the work are appropriately investigated and resolved. The study was conducted in accordance with the Declaration of Helsinki (as revised in 2013).
Open Access Statement: This is an Open Access article distributed in accordance with the Creative Commons Attribution-NonCommercial-NoDerivs 4.0 International License (CC BY-NC-ND 4.0), which permits the non-commercial replication and distribution of the article with the strict proviso that no changes or edits are made and the original work is properly cited (including links to both the formal publication through the relevant DOI and the license). See: https://creativecommons.org/licenses/by-nc-nd/4.0/.
References
- Afifi AM, Saad AM, Al-Husseini MJ, et al. Causes of death after breast cancer diagnosis: A US population-based analysis. Cancer 2020;126:1559-67. [Crossref] [PubMed]
- Bray F, Ferlay J, Soerjomataram I, et al. Global cancer statistics 2018: GLOBOCAN estimates of incidence and mortality worldwide for 36 cancers in 185 countries. CA Cancer J Clin 2018;68:394-424. [Crossref] [PubMed]
- Sung H, Ferlay J, Siegel RL, et al. Global Cancer Statistics 2020: GLOBOCAN Estimates of Incidence and Mortality Worldwide for 36 Cancers in 185 Countries. CA Cancer J Clin 2021;71:209-49. [Crossref] [PubMed]
- Dumas A, Vaz Luis I, Bovagnet T, et al. Impact of Breast Cancer Treatment on Employment: Results of a Multicenter Prospective Cohort Study (CANTO). J Clin Oncol 2020;38:734-43. [Crossref] [PubMed]
- Huo X, Shen G, Li J, et al. Identification of the GTPase IMAP family as an immune-related prognostic biomarker in the breast cancer tumor microenvironment. Gene 2022;812:146094. [Crossref] [PubMed]
- Fitzpatrick A, Tutt A. Controversial issues in the neoadjuvant treatment of triple-negative breast cancer. Ther Adv Med Oncol 2019;11:1758835919882581. [Crossref] [PubMed]
- Voena C, Chiarle R. Advances in cancer immunology and cancer immunotherapy. Discov Med 2016;21:125-33. [PubMed]
- Quail DF, Joyce JA. Microenvironmental regulation of tumor progression and metastasis. Nat Med 2013;19:1423-37. [Crossref] [PubMed]
- Balkwill FR, Capasso M, Hagemann T. The tumor microenvironment at a glance. J Cell Sci 2012;125:5591-6. [Crossref] [PubMed]
- Ribas A, Wolchok JD. Cancer immunotherapy using checkpoint blockade. Science 2018;359:1350-5. [Crossref] [PubMed]
- Salmon H, Remark R, Gnjatic S, et al. Host tissue determinants of tumour immunity. Nat Rev Cancer 2019;19:215-27. [PubMed]
- Kandoth C, McLellan MD, Vandin F, et al. Mutational landscape and significance across 12 major cancer types. Nature 2013;502:333-9. [Crossref] [PubMed]
- Thomas A, Routh ED, Pullikuth A, et al. Tumor mutational burden is a determinant of immune-mediated survival in breast cancer. Oncoimmunology 2018;7:e1490854. [Crossref] [PubMed]
- Feng R, Xu J, Huang J, et al. An immune-related prognostic gene ULBP2 is correlated with immunosuppressive tumor microenvironment and immunotherapy in breast cancer. Heliyon 2023;10:e23687. [Crossref] [PubMed]
- Zhang B, He L, Zhou C, et al. A pancancer analysis of the clinical and genomic characteristics of multiple primary cancers. Sci Rep 2024;14:2367. [Crossref] [PubMed]
- Chan TA, Wolchok JD, Snyder A. Genetic Basis for Clinical Response to CTLA-4 Blockade in Melanoma. N Engl J Med 2015;373:1984. [Crossref] [PubMed]
- Le DT, Uram JN, Wang H, et al. PD-1 Blockade in Tumors with Mismatch-Repair Deficiency. N Engl J Med 2015;372:2509-20. [Crossref] [PubMed]
- Goodman AM, Kato S, Bazhenova L, et al. Tumor Mutational Burden as an Independent Predictor of Response to Immunotherapy in Diverse Cancers. Mol Cancer Ther 2017;16:2598-608. [Crossref] [PubMed]
- Samstein RM, Lee CH, Shoushtari AN, et al. Tumor mutational load predicts survival after immunotherapy across multiple cancer types. Nat Genet 2019;51:202-6. [Crossref] [PubMed]
- Pathan M, Keerthikumar S, Ang CS, et al. FunRich: An open access standalone functional enrichment and interaction network analysis tool. Proteomics 2015;15:2597-601. [Crossref] [PubMed]
- Newman AM, Liu CL, Green MR, et al. Robust enumeration of cell subsets from tissue expression profiles. Nat Methods 2015;12:453-7. [Crossref] [PubMed]
- Jézéquel P, Frénel JS, Campion L, et al. bc-GenExMiner 3.0: new mining module computes breast cancer gene expression correlation analyses. Database (Oxford) 2013;2013:bas060. [Crossref] [PubMed]
- Klempner SJ, Fabrizio D, Bane S, et al. Tumor Mutational Burden as a Predictive Biomarker for Response to Immune Checkpoint Inhibitors: A Review of Current Evidence. Oncologist 2020;25:e147-59. [Crossref] [PubMed]
- Rizvi NA, Hellmann MD, Snyder A, et al. Cancer immunology. Mutational landscape determines sensitivity to PD-1 blockade in non-small cell lung cancer. Science 2015;348:124-8. [Crossref] [PubMed]
- Zhang C, Shen L, Qi F, et al. Multi-omics analysis of tumor mutation burden combined with immune infiltrates in bladder urothelial carcinoma. J Cell Physiol 2020;235:3849-63. [Crossref] [PubMed]
- Bi F, Chen Y, Yang Q. Significance of tumor mutation burden combined with immune infiltrates in the progression and prognosis of ovarian cancer. Cancer Cell Int 2020;20:373. [Crossref] [PubMed]
- Keup C, Kimmig R, Kasimir-Bauer S. Liquid Biopsies to Evaluate Immunogenicity of Gynecological/Breast Tumors: On the Way to Blood-Based Biomarkers for Immunotherapies. Breast Care (Basel) 2020;15:470-80. [Crossref] [PubMed]
- Gao C, Li H, Liu C, et al. Tumor Mutation Burden and Immune Invasion Characteristics in Triple Negative Breast Cancer: Genome High-Throughput Data Analysis. Front Immunol 2021;12:650491. [Crossref] [PubMed]
- Karn T, Denkert C, Weber KE, et al. Tumor mutational burden and immune infiltration as independent predictors of response to neoadjuvant immune checkpoint inhibition in early TNBC in GeparNuevo. Ann Oncol 2020;31:1216-22. [Crossref] [PubMed]
- Ravaioli S, Limarzi F, Tumedei MM, et al. Are we ready to use TMB in breast cancer clinical practice? Cancer Immunol Immunother 2020;69:1943-5. [Crossref] [PubMed]
- de Melo Gagliato D, Buzaid AC, Perez-Garcia J, et al. Immunotherapy in Breast Cancer: Current Practice and Clinical Challenges. BioDrugs 2020;34:611-23. [Crossref] [PubMed]
- Marabelle A, Fakih M, Lopez J, et al. Association of tumour mutational burden with outcomes in patients with advanced solid tumours treated with pembrolizumab: prospective biomarker analysis of the multicohort, open-label, phase 2 KEYNOTE-158 study. Lancet Oncol 2020;21:1353-65. [Crossref] [PubMed]
- Park SE, Park K, Lee E, et al. Clinical implication of tumor mutational burden in patients with HER2-positive refractory metastatic breast cancer. Oncoimmunology 2018;7:e1466768. [Crossref] [PubMed]
- Wang F, Tang C, Gao X, et al. Identification of a six-gene signature associated with tumor mutation burden for predicting prognosis in patients with invasive breast carcinoma. Ann Transl Med 2020;8:453. [Crossref] [PubMed]
- Zhang M, Zhang J. PEG3 mutation is associated with elevated tumor mutation burden and poor prognosis in breast cancer. Biosci Rep 2020;40:BSR20201648. [Crossref] [PubMed]
- Li L, Li M, Wang X. Cancer type-dependent correlations between TP53 mutations and antitumor immunity. DNA Repair (Amst) 2020;88:102785. [Crossref] [PubMed]
- Zhao Z, He B, Cai Q, et al. Combination of tumor mutation burden and immune infiltrates for the prognosis of lung adenocarcinoma. Int Immunopharmacol 2021;98:107807. [Crossref] [PubMed]
- Yang K, Hu B, Zhu G, et al. Correlation of Reduced PTGER3 Expression with Prognosis and Immune Infiltration in Clear Cell Renal Carcinoma. Arch Esp Urol 2023;76:270-82. [Crossref] [PubMed]
- Sjöberg E, Augsten M, Bergh J, et al. Expression of the chemokine CXCL14 in the tumour stroma is an independent marker of survival in breast cancer. Br J Cancer 2016;114:1117-24. [Crossref] [PubMed]
- Xie Q, Xiao YS, Jia SC, et al. FABP7 is a potential biomarker to predict response to neoadjuvant chemotherapy for breast cancer. Cancer Cell Int 2020;20:562. [Crossref] [PubMed]
- Wang Y, Li J. Identification of Candidate Biomarker TMSB15A and Its Prognostic Value in Breast Cancer. Hans Journal of Data Mining 2022;12:1-7. [Crossref]
- Zhang Y, Di X, Chen G, et al. An immune-related signature that to improve prognosis prediction of breast cancer. Am J Cancer Res 2021;11:1267-85. [PubMed]