A novel lactylation-related gene signature for effectively distinguishing and predicting the prognosis of ovarian cancer
Highlight box
Key findings
• Subtypes of ovarian cancer (OC) were associated with 14 key lactylation-related genes, and an 8-lactylation-related gene prognostic model predicted the prognosis of patients with OC.
What is known and what is new?
• Key lactylation-related genes are critical in the emergence of OC.
• We identified the lactylation-related subtypes of OC and the lactylation-related biomarkers.
What is the implication, and what should change now?
• Lactylation-related biomarkers are strongly associated with the prognosis of patients with OC.
Introduction
Ovarian cancer (OC) is one of the most common malignant tumors of the female reproductive system, with its incidence being only second to that of cervical and uterine body cancer (1). Since effective screening tools are lacking and early diagnosis is difficult, 80% of patients with OC are already at an advanced stage when they are diagnosed (2,3). It is estimated that 50–70% of patients with OC will relapse within 2 years of treatment, and only 30% will survive 5 years after treatment (1-3). At present, the treatment of OC mainly includes surgical treatment and platinum-based chemotherapy. Although treatment has improved recently, the 5-year survival rates have only slightly increased (3). It is essential to identify new therapeutic targets for OC given the limitations of existing treatments, and thus there is an urgent need to develop reliable new prognostic models to make targeted therapy more feasible.
Lactic acid, which is a metabolic waste product of glycolysis, has been long overlooked as a purportedly poor prognostic marker in relation to tumor occurrence and development (4-7). There is growing evidence however that lactic acid plays a crucial role in tumor growth, immune escape, invasion, and metastasis, as well as metabolic regulation (5-7). Lactic acid, for example, is secreted by cancer cells and promotes cancer growth (5). Lactate efflux through proton-coupled pathways can influence tumor growth by regulating the tumor microenvironment (8). The presence of extracellular acidosis impairs T-cell-mediated immunity, while the neutralization of tumor acidity may improve immunotherapy’s antitumor effects (9). Lactate metabolism-related genes (LRGs) in OC, however, remain unexplored bioinformatically.
It is generally accepted that lactic acid plays a critical role in tumor development and antitumor processes. Despite this, little is known about its specific function in OC. As a result, we conducted a systematic study to determine LRG expression levels in OC, investigated the correlation between lactate and the tumor immune microenvironment, and determined its prognostic value. We present this article in accordance with the TRIPOD reporting checklist (available at https://tcr.amegroups.com/article/view/10.21037/tcr-24-319/rc).
Methods
Data acquisition from The Cancer Genome Atlas (TCGA)-OC cohort
We downloaded 376 messenger RNA (mRNA) transcriptome profiles and the corresponding clinical data of TCGA-OC patients from TCGA database (https://portal.gdc.cancer.gov/repository). The filters used to select the samples as followed: a, primary site; b, Grade 3; c, stage of II–IV. TCGA data is downloaded from TCGA counts data, converted to TPM format based on the counts data, and then normalized to log2(TPM+1). There are a total of 332 LRGs listed in this study, as based on a published study (10). The study was conducted in accordance with the Declaration of Helsinki (as revised in 2013).
Identification of prognostic LRGs in OC
We performed a univariate Cox analysis to identify the prognostic genes with a P value of less than 0.05 in patients with OC. A Venn diagram was generated using the R package “venn” (v. 1.11; The R Foundation for Statistical Computing). The expression heatmap was then constructed with the R package “pheatmap” (v. 1.0.12). We conducted Kyoto Encyclopedia of Genes and Genomes (KEGG) pathway enrichment analyses with “clusterProfiler” package in R to identify signaling pathways of prognostic genes in OC. The Search Tool for the Retrieval of Interacting Genes/Proteins (STRING) website and the “clusterProfiler” package were then used to construct a protein-protein interaction (PPI) network based on the PRGs in OC. The PPI network was visualized using Cytoscape. The R packages “corrplot” (v. 0.92) and “circlize” (v. 0.4.15) were used to analyze the correlation networks. The absolute value of the correlation coefficient represents different correlation strengths within different ranges: below 0.3, indicates no correlation; 0.3 to 0.5, indicates low correlation; >0.5 to 0.9, indicates significant correlation; >0.9 to 1.0, indicates extremely high correlation.
Identification of lactylation-related tumor subtypes in OC
The relationship between LRGs and OC subtypes was investigated. Consistent cluster analysis was conducted using the “ConsensusClusterPlus” package (version 1.54.0) on TCGA-OC cohort. Clustering variable (k) was set between 2 and 6. The “Pheatmap” (version 1.0.12) package was used to generate the heatmap. Based on Kaplan-Meier analysis, we compared the survival times among the different subgroups.
Identification of lactylation-related differentially expressed genes (DEGs) in OC
The “DEseq2” package in R was used to identify the DEGs between OC clusters. The cutoff criteria included a P value <0.05 and |log2 fold change| >0.75. In addition, the DEGs were visualized using the “ggplot2” R package. Based on the R package “pheatmap” (version 1.0.12), the DEG heatmap was created. The “clusterProfiler” package was then used to conduct Gene Ontology (GO) and KEGG analyses.
The immune activity of two lactylation-related clusters in OC
“Immunoeconomics” was used to examine the immune activity of LRGs. The immune activity of two clusters related to lactylation was compared using eight immune checkpoint genes: CD274, PDCD1, PDCD1LG2, CTLA4, LAG3, HAVCR2, TIGIT, and SIGLEC15. We used the R packages “pheatmap” and “ggplot2” to generate the heatmap and box plot, respectively. We compared the degree of immune-cell infiltration and activated immune pathways between the two groups via the Wilcoxon test. Statistical significance was set as a P<0.05. The data is sourced from TIMER’s website (https://cistrome.shinyapps.io/timer/), which uses deconvolution algorithms to infer the abundance of tumor infiltrating immune cells (TIICs) from gene expression profiles.
Two lactylation-related clusters of drug susceptibility
The pRRophetic algorithm was used to assess the drug sensitivity between two lactylation-related clusters in OC. Pharmacogenomics databases (https://www.cancerrxgene.org/) were used to evaluate each patient’s chemotherapy response. Predictions were made using the R package “pRRophetic”. An estimation of the IC50 was completed using the ridge regression method. Statistical significance was set at a P value of ≤0.05.
The development of a prognostic model based on LRGs
With the “glmnet” R package, Cox regression analysis was also applied to assess the prognostic value of the LRGs in the TCGA-OC cohort. Based on the minimum criteria, the λ condition was determined for variables with nonzero coefficients. The risk score was calculated using the following formula: risk score = sum (expression level of each gene × corresponding coefficient). On the basis of median risk scores, TCGA-OC patients were divided into low- and high-risk subgroups. Using the R package “survival”, we compared OS times among the different subgroups. Cox proportional hazard was conducted to calculate hazard ratios (HRs) with 95% confidence intervals (CIs).
Statistical analysis
Perform statistical analysis using R language (version 3.6.3). Survival analysis was used to determine independent prognostic factors for HCC, with a significance level of P<0.05. Bilateral P values <0.05 are considered statistically significant.
Results
Identification of key LRGs in OC
Univariate Cox analysis was conducted to identify the prognostic genes in TCGA-OCC patients and to clarify their roles. This analysis yielded 707 prognostic genes for OC (Figure 1A and Table S1). The prognostic LRGs were screened out using a Venn diagram (Figure 1A and Table S1). We eventually identified eight lactylation-related prognostic genes: SNRPA1, MPHOSPH6, POLDIP3, RB1, AHNAK, MAGOHB, HDAC1, and CALM1 (Figure 1A and Table S1).
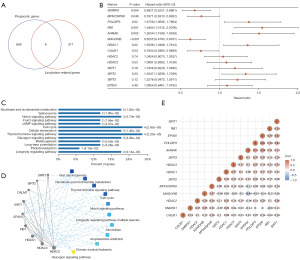
A study indicated lactase and delactylases to be the most important regulatory genes (10), one lactase and six delactylases were included as key LRGs: EP300, HDAC1, HDAC2, HDAC3, SIRT1, SIRT2, and SIRT3. Finally, we identified 14 key LRGs SNRPA1, MPHOSPH6, POLDIP3, RB1, AHNAK, MAGOHB, CALM1, EP300, HDAC1, HDAC2, HDAC3, SIRT1, SIRT2, and SIRT3 (Figure 1B). KEGG enrichment indicated that these pathways may play a crucial role in OC via nicotinate and nicotinamide metabolism, spliceosome, thyroid hormone signaling pathway, cell cycle, and glucagon signaling pathway (Figure 1C). Additionally, these pathways were confirmed to be associated with these genes through PPI network analysis (Figure 1D).
The results of the gene correlation analysis are summarized in Figure 1E. At the transcriptome level, the follow genes exhibited strong positive correlations: EP300, POLDIP3, SIRT1, and AHNAK; POLDIP3 and AHNAK; and HDAC1 and HDAC3 (Figure 1E and Table S1).
OC subtypes based on the LRGs
Despite lactylation’s close association with tumor development (4-6), the role of LRGs in OC remains to be investigated in detail. Via consensus clustering analysis, we stratified TCGA-OC patients into different subtypes based on the 14 LRGs (Figure 2A-2C and table available at https://cdn.amegroups.cn/static/public/tcr-24-319-1.xlsx). A consistency cluster and principal component analysis (PCA) showed that TCGA-OC patients were well stratified into two clusters when clustering variable (k) was 2 (Figure 2A-2D and table available at https://cdn.amegroups.cn/static/public/tcr-24-319-1.xlsx). Similar results have also been obtained in other study (7). A heatmap of LRGs in TCGA-OC patients showed good separation between the two groups (Figure 2E). We hope to monitor patients for a longer survival time. Overall survival was statistically significantly longer in the low-risk group 1 than in high-risk group 2 (HR: 0.622, 95% CI: 0.460–0.841; P=0.002; Figure 2F and table available at https://cdn.amegroups.cn/static/public/tcr-24-319-1.xlsx).
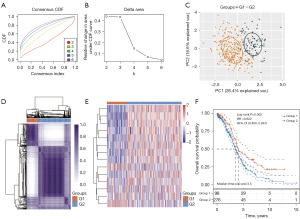
Identification of underlying mechanisms between the two OC groups
A volcano plot was used to identify 3918 DEGs between the G1 and G2 groups to (P value <0.05; |log2 fold change| >0.5850; Figure 3A and table available at https://cdn.amegroups.cn/static/public/tcr-24-319-2.xlsx). The DEGs included 104 upregulated and 3,814 downregulated genes in G1 compared with G2. There was a difference in the expression of the top 50 DEGs between the two groups visualized in the heatmap (Figure 3B and table available at https://cdn.amegroups.cn/static/public/tcr-24-319-2.xlsx).
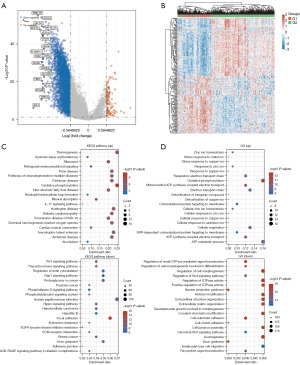
KEGG and GO enrichment analyses were conducted to clarify the biological roles of the 3,918 DEGs. In the KEGG analysis, 104 upregulated genes were found to be primarily involved in thermogenesis, oxidative phosphorylation, neutrophil extracellular trap formation, and IL-17 signaling pathway, while 3,814 downregulated genes were primarily involved in proteoglycans in cancer, focal adhesion, Wnt signaling pathway, ECM-receptor interaction, and the adherens junction (Figure 3C and table available at https://cdn.amegroups.cn/static/public/tcr-24-319-2.xlsx). According to the GO analysis of the biological process results, most of the upregulated genes were involved in oxidative phosphorylation, respiratory electron transport chain, adenosine triphosphate metabolic process, and response to zinc ion, while the downregulated genes were involved in ECM organization, regulation of GTPase activity, cell-substrate adhesion, and axonogenesis (Figure 3D and table available at https://cdn.amegroups.cn/static/public/tcr-24-319-2.xlsx). Studies have shown that proteoglycans in cancer, focal adhesion, ECM-receptor interaction, and adherens junction are tumor markers (10-12). According to the results above, G2 OC subtypes might be more capable of migrating and dividing than might be the G1 OC subtypes.
The immune activity of two lactylation-related groups in OC
Research suggests there to be a close relationship between lactylation and immune activity in many cancers (13,14). We compared the immunity between two lactylation-related clusters of patients with OC. The resulting box plots revealed a clear difference in immune cells, with the abundance of CD4+ T cells and endothelial cells being lower in the G1 than in the G2 OC samples (Figure 4A and table available at https://cdn.amegroups.cn/static/public/tcr-24-319-3.xlsx). Moreover, the box plots indicated that the expressions six of the eight immune checkpoint inhibitor (ICI) related genes (i.e., CD274, HAVCR2, PDCD1LG2, and SIGLEC15) were lower in the G1 OC samples compared to the G2 samples (Figure 4B and table available at https://cdn.amegroups.cn/static/public/tcr-24-319-3.xlsx). The findings suggested that lactylation is closely related to immune activity.
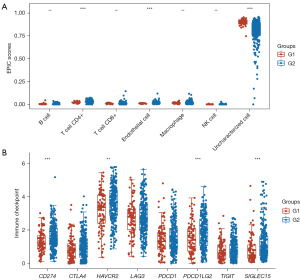
A comparison of the drug susceptibilities of the two OC groups
The two OC groups were then tested using the pRRophetic algorithms for drug sensitivity. The drug sensitivity analysis in one cluster of OC was conducted using gemcitabine, bleomycin, doxorubicin, cisplatin, and paclitaxel.
Gemcitabine, bleomycin, and doxorubicin had lower IC50 values in the high-risk G2 patients compared to the low-risk G1 patients (Figure 5A-5C and table available at https://cdn.amegroups.cn/static/public/tcr-24-319-4.xlsx). Compared to the G1 patients with OC, the G2 patients had higher IC50 values for cisplatin and paclitaxel (Figure 5D,5E and table available at https://cdn.amegroups.cn/static/public/tcr-24-319-4.xlsx). Based on these results, the chemotherapy drugs gemcitabine, bleomycin, and doxorubicin might be more effective for low-risk G1 patients, while cisplatin and paclitaxel might be more effective for high-risk G2 patients. The results further suggested that lactylation-related classification might be a useful predictor of chemotherapy response.
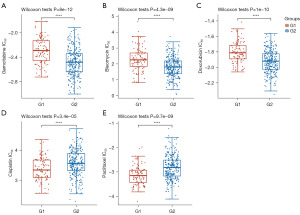
The development of a prognostic model based on LRGs
The 14 LRGs in OC were further selected via least absolute shrinkage and selection operator (LASSO) and Cox regression analyses (Figure 6A and table available at https://cdn.amegroups.cn/static/public/tcr-24-319-5.xlsx). We developed an eight-gene signature using the optimal λ value (Figure 6B and table available at https://cdn.amegroups.cn/static/public/tcr-24-319-5.xlsx). The risk score was calculated as follows: Risk score = SNRPA1 expression × (–0.1835) + MPHOSPH6 expression × (–0.1804) + POLDIP3 expression × 0.0807 + RB1 expression × 0.1033 + HDAC1 expression ×0.1565 + CALM1 expression × (–0.1174) + HDAC2 expression×0.0651 + SIRT2 expression × 0.1234.
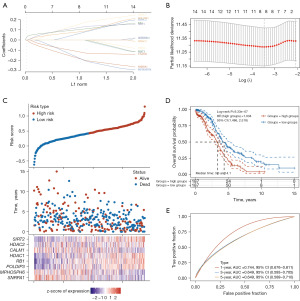
A low-risk and a high-risk group were established for TCGA-OC patients based on this gene signature (Figure 6C). Patients with a low-risk score had a higher overall survival rate than did those with a high-risk score (HR: 1.934, 95% CI: 1.486–2.518; P=9.33e–07; Figure 6D and table available at https://cdn.amegroups.cn/static/public/tcr-24-319-5.xlsx). Prognostic models were tested using the area under the receiver operating characteristic (ROC) curve (AUC). The AUCs for 1, 3, and 5 years were 0.744, 0.649, and 0.649, respectively (Figure 6E and table available at https://cdn.amegroups.cn/static/public/tcr-24-319-5.xlsx), indicating that this model was a good prognosticator.
A comparison of the tumor stemness of the two OC groups
There is a low survival rate after immune checkpoint blockade (ICB) treatment when the tumor immune dysfunction and exclusion (TIDE) score is high. TIDE scores of G2 TCGA-OCC patients were higher than those of G1 patients, suggesting an inadequate response to ICB therapy and a poor prognosis (Figure 7A and table available at https://cdn.amegroups.cn/static/public/tcr-24-319-6.xlsx).
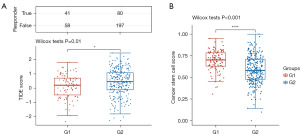
Cancer stem cells (CSCs) play an important role in tumor development, relapse, metastasis, and chemotherapy resistance. A significant difference was observed between G2 and G1 TCGA-OC patients in terms of CSC scores (Figure 7B and table available at https://cdn.amegroups.cn/static/public/tcr-24-319-6.xlsx). According to these results, lactylation-related classification might be a good indicator of ICB therapy response.
Discussion
A total of 707 prognostic genes and 14 key LRGs (SNRPA1, MPHOSPH6, POLDIP3, RB1, AHNAK, MAGOHB, CALM1, EP300, HDAC1, HDAC2, HDAC3, SIRT1, SIRT2, and SIRT3) were identified in TCGA-OC patients. Based on the 14 genes involved in lactylation, TCGA-OC patients were divided into low-risk and high-risk groups. Downregulated DEGs in the low-risk G1 group were associated with thermogenesis, oxidative phosphorylation, neutrophil extracellular trap formation, and IL-17 signaling pathway, whereas upregulated DEGs were associated with proteoglycans in cancer, focal adhesion, Wnt signaling pathway, ECM-receptor interaction, and adherens junction. The immune activity of the low-risk G1 group was lower than that of high-risk G2 group; and gemcitabine, bleomycin, and doxorubicin had lower IC50 values in the high-risk G2 OC patients, while cisplatin and paclitaxel had higher IC50 values in the low-risk G1 patients. The prognosis of patients with OC was also predicted with the help of an eight-gene prognostic model that included SNRPA1, MPHOSPH6, POLDIP3, RB1, HDAC1, CALM1, HDAC2, and SIRT2.
According to a series of studies, lactylate plays a significant role in inflammation, fibrosis, and oncogenic processes (5,6). For example, lactylate accumulates in the periphery of tumor tissues, causing vascular endothelial growth factor (VEGF) to be released, promoting angiogenesis and boosting cancer cell motility (5). Extracellular acidosis impairs T-cell-mediated immunity, and neutralizing tumor acidity may increase immunotherapy’s antitumor effectiveness (9). However, the relationship between lactylation and OC, remains largely unexplored.
LRGs were used to group divide TCGA-OC patients into two groups (high risk and low risk). The low-risk G2 subgroup had higher levels of immune activity than did the high-risk G1 subgroup. Compared to G1 OC patients, G2 OC patients had higher IC50 values for vinorelbine, paclitaxel, and cisplatin. These results suggest that OC tumors can be classified into two subgroups based on LRGs.
The expression of LRGs had a positive correlation with prognosis in patients with OC, and a prognostic model consisting of eight LRGs (SNRPA1, MPHOSPH6, POLDIP3, RB1, HDAC1, CALM1, HDAC2, and SIRT2) was developed for patients with OC.
U1 small nuclear ribonucleoprotein A (SNRPA1) has been shown to abrogate RMRP regulation of p53 and tumor cell growth (15). M-phase phosphoprotein 6 (MPHOSPH6) has been reported to be associated with a risk of hepatocellular carcinoma (16). POLDIP3 protein (POLDIP3) has been found to promote the progression of hepatocellular carcinoma (17). Retinoblastoma-associated protein (RB1) was demonstrated to regulate cell cycle progression and the downstream cyclin-dependent kinase in cancers (18). In one study, silencing histone deacetylase 1 (HDAC1) enhanced the chemotherapy response for OC (19). Calmodulin-1 (CALM1) was shown to promote the progression and impaired chemosensitivity to epidermal growth factor receptor (EGFR) inhibitor in esophageal squamous cell carcinoma (20). Histone deacetylase 1 (HDAC2) is considered to be a target for anticancer drugs (21). Finally, NAD-dependent protein deacetylase sirtuin-2 (SIRT2) has been associated with high risk across numerous cancers (22).
Some limitations to this study should be mentioned. It is still necessary to confirm these findings in vivo and in vitro by examining these eight LRGs. Furthermore, future research should also consider the related molecular mechanisms.
Conclusions
In conclusion, LRGs are closely related to tumor classification and immunity in patients with OC. The lactylation-related signature demonstrated good prognostic predictive performance for OC. These findings may offer new insights into the diagnosis and treatment of OC.
Acknowledgments
Funding: None.
Footnote
Reporting Checklist: The authors have completed the TRIPOD reporting checklist. Available at https://tcr.amegroups.com/article/view/10.21037/tcr-24-319/rc
Peer Review File: Available at https://tcr.amegroups.com/article/view/10.21037/tcr-24-319/prf
Conflicts of Interest: All authors have completed the ICMJE uniform disclosure form (available at https://tcr.amegroups.com/article/view/10.21037/tcr-24-319/coif). The authors have no conflicts of interest to declare.
Ethical Statement: The authors are accountable for all aspects of the work in ensuring that questions related to the accuracy or integrity of any part of the work are appropriately investigated and resolved. The study was conducted in accordance with the Declaration of Helsinki (as revised in 2013).
Open Access Statement: This is an Open Access article distributed in accordance with the Creative Commons Attribution-NonCommercial-NoDerivs 4.0 International License (CC BY-NC-ND 4.0), which permits the non-commercial replication and distribution of the article with the strict proviso that no changes or edits are made and the original work is properly cited (including links to both the formal publication through the relevant DOI and the license). See: https://creativecommons.org/licenses/by-nc-nd/4.0/.
References
- Bast RC Jr, Hennessy B, Mills GB. The biology of ovarian cancer: new opportunities for translation. Nat Rev Cancer 2009;9:415-28. [Crossref] [PubMed]
- Kuroki L, Guntupalli SR. Treatment of epithelial ovarian cancer. BMJ 2020;371:m3773. [Crossref] [PubMed]
- Lee JY, Kim S, Kim YT, et al. Changes in ovarian cancer survival during the 20 years before the era of targeted therapy. BMC Cancer 2018;18:601. [Crossref] [PubMed]
- Wang ZH, Peng WB, Zhang P, et al. Lactate in the tumour microenvironment: From immune modulation to therapy. EBioMedicine 2021;73:103627. [Crossref] [PubMed]
- Chen AN, Luo Y, Yang YH, et al. Lactylation, a Novel Metabolic Reprogramming Code: Current Status and Prospects. Front Immunol 2021;12:688910. [Crossref] [PubMed]
- Ying M, You D, Zhu X, et al. Lactate and glutamine support NADPH generation in cancer cells under glucose deprived conditions. Redox Biol 2021;46:102065. [Crossref] [PubMed]
- Zhou Y, Wei X, Li W, et al. Comprehensive analysis of mitophagy-related subtypes of breast cancer and the association with immune related characteristics. Heliyon 2023;9:e23267. [Crossref] [PubMed]
- Brown TP, Ganapathy V. Lactate/GPR81 signaling and proton motive force in cancer: Role in angiogenesis, immune escape, nutrition, and Warburg phenomenon. Pharmacol Ther 2020;206:107451. [Crossref] [PubMed]
- Erra Díaz F, Dantas E, Geffner J. Unravelling the Interplay between Extracellular Acidosis and Immune Cells. Mediators Inflamm 2018;2018:1218297. [Crossref] [PubMed]
- Cheng Z, Huang H, Li M, et al. Lactylation-Related Gene Signature Effectively Predicts Prognosis and Treatment Responsiveness in Hepatocellular Carcinoma. Pharmaceuticals (Basel) 2023;16:644. [Crossref] [PubMed]
- Senga SS, Grose RP. Hallmarks of cancer-the new testament. Open Biol 2021;11:200358. [Crossref] [PubMed]
- Pickup MW, Mouw JK, Weaver VM. The extracellular matrix modulates the hallmarks of cancer. EMBO Rep 2014;15:1243-53. [Crossref] [PubMed]
- Ye L, Jiang Y, Zhang M. Crosstalk between glucose metabolism, lactate production and immune response modulation. Cytokine Growth Factor Rev 2022;68:81-92. [Crossref] [PubMed]
- Chen L, Huang L, Gu Y, et al. Lactate-Lactylation Hands between Metabolic Reprogramming and Immunosuppression. Int J Mol Sci 2022;23:11943. [Crossref] [PubMed]
- Chen Y, Hao Q, Wang S, et al. Inactivation of the tumor suppressor p53 by long noncoding RNA RMRP. Proc Natl Acad Sci U S A 2021;118:e2026813118. [Crossref] [PubMed]
- Zhang Y, Wang S, Wen X, et al. Association of ACYP2 and MPHOSPH6 genetic polymorphisms with the risk of hepatocellular carcinoma in chronic hepatitis B virus carriers. Oncotarget 2017;8:86011-9. [Crossref] [PubMed]
- Liu XN, Yuan JH, Wang TT, et al. An alternative POLDIP3 transcript promotes hepatocellular carcinoma progression. Biomed Pharmacother 2017;89:276-83. [Crossref] [PubMed]
- Knudsen ES, Pruitt SC, Hershberger PA, et al. Cell Cycle and Beyond: Exploiting New RB1 Controlled Mechanisms for Cancer Therapy. Trends Cancer 2019;5:308-24. [Crossref] [PubMed]
- Liu X, Yu Y, Zhang J, et al. HDAC1 Silencing in Ovarian Cancer Enhances the Chemotherapy Response. Cell Physiol Biochem 2018;48:1505-18. [Crossref] [PubMed]
- Liu T, Han X, Zheng S, et al. CALM1 promotes progression and dampens chemosensitivity to EGFR inhibitor in esophageal squamous cell carcinoma. Cancer Cell Int 2021;21:121. [Crossref] [PubMed]
- Jo H, Shim K, Kim HU, et al. HDAC2 as a target for developing anti-cancer drugs. Comput Struct Biotechnol J 2023;21:2048-57. [Crossref] [PubMed]
- Wang T, Xu Z, Lu Y, et al. Recent Progress on the Discovery of Sirt2 Inhibitors for the Treatment of Various Cancers. Curr Top Med Chem 2019;19:1051-8. [Crossref] [PubMed]