RPN1: a pan-cancer biomarker and disulfidptosis regulator
Highlight box
Key findings
• This research illuminates the pivotal role of ribophorin I (RPN1) in promoting disulfidptosis by triggering cell skeleton breakdown. It underscores its broad significance as a biomarker across diverse cancer types and its correlation with the efficacy of immune therapy targeting PD-L1, particularly in urothelial carcinoma.
What is known and what is new?
• Through genome-wide CRISPR/Cas9 screening, key genes contributing to disulfidptosis were identified, including RPN1. However, the precise mechanism by which RPN1 influences disulfidptosis remains unclear. It is known that RPN1 plays a crucial role in innate immunity in Arabidopsis, yet whether RPN1 serves as a novel cancer biomarker and its association with the efficacy of immunotherapy remain elusive.
What is the implication, and what should change now?
• The findings of this study underscore the mechanism by which RPN1 promotes disulfidptosis through inducing cell skeleton protein breakdown, emphasizing its impact on diagnosis, prognosis, and immune checkpoint inhibitor therapy in the context of pan-cancer scenarios. We demonstrate the inhibition of disulfidptosis upon RPN1 gene knockout in cell lines not previously validated, irrespective of SLC7A11 expression levels, providing additional evidence for RPN1 as a potential universal target for cancer therapy. Future research should explore whether other metabolic stress conditions can lower intracellular NADPH levels, thus inducing disulfidptosis. Additionally, our study highlights the potential of RPN1 as an immune therapy marker, necessitating further validation of its role in cohorts.
Introduction
In the tumor microenvironment, cells face challenges like oxidative stress, metabolic dysregulation, and rapid proliferation (1). Glutathione, crucial for protection, is intricately regulated by cysteine (1). SLC7A11 facilitates cysteine supply to tumor cells. Blocking cysteine uptake induces ferroptosis, linked to oxidative stress (1). Recent studies revealed cell death from reductive stress (2). Excess cysteine, boosted by SLC7A11 and glucose deprivation, led to disulfidptosis discovery, marked by abnormal disulfide bond formation in actin, causing F-actin contraction and cell membrane detachment (3). Key genes like SLC7A11, SLC3A2, ribophorin I (RPN1), and NCKAP1 were identified via CRISPR/Cas9 screening (3). Interestingly, even under glucose-deficient conditions, supplementing excess cysteine in the culture medium of renal cell carcinoma (RCC) cells (786-O) with low SLC7A11 expression induced disulfidptosis (3). It’s unclear if disulfidptosis is widespread in other tumor cell lines. Deleting RPN1 rendered urothelial carcinoma cells (UMRC6) resistant to disulfidptosis (3), but RPN1’s precise role remains unclear.
Cellular oxidative stress triggers typical immune responses (4), paralleled by reactions akin to reductive stress, such as NLRP1 and CARD8-mediated cell pyroptosis (5). Glucose deficiency and reduced ATP levels activate NLRP1 inflammasomes (6), disrupting cytoskeletal function and leading to disulfidptosis (3). Inflammasomes, pivotal in innate immunity, inflammation, and cell death, imply a link between disulfidptosis and immune activation, critical in cancer (7). RPN1’s significant role in Arabidopsis innate immunity underscores the interest in exploring its mechanisms and immune implications in disulfidptosis within the tumor microenvironment.
Several studies have investigated the relationship between RPN1 and cancer. For example, it may promote the progression of breast cancer (8) and be associated with poor prognosis in hepatocellular carcinoma (HCC) (9). However, the biomarker value of RPN1 across pan-cancers has yet to be investigated.
In this study, we propose that glucose deficiency can induce RPN1-dependent disulfidptosis. We observed RPN1-dependent disulfidptosis in breast cancer (MDA-MB-231) and lung cancer (A549) cell lines without additional cysteine supplementation. We hypothesize that RPN1 expression could predict patient outcomes, immune microenvironment, and response to immunotherapy. Through bioinformatics analysis across 33 tumor types, we explore RPN1’s clinical relevance, mechanisms in tumor biology, and implications for immunotherapy. RPN1 gene knockout inhibits disulfidptosis in various cell lines, regardless of SLC7A11 expression, suggesting RPN1 as a potential universal cancer therapy target. We present this article in accordance with the MDAR reporting checklist (available at https://tcr.amegroups.com/article/view/10.21037/tcr-24-581/rc).
Methods
Figure 1 depicts the workflow employed in this study. The study was conducted in accordance with the Declaration of Helsinki (as revised in 2013).
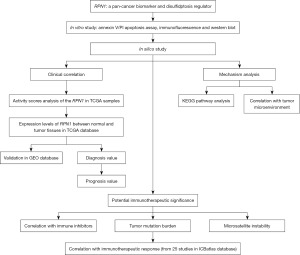
Cell lines and culture
All cell lines used in this study were originally obtained from the American Type Culture Collection (ATCC; Manassas, VA, USA) as follows: HEK-293T, A549, and MDA-MB-231. All cells were incubated in humidified air at 37 ℃ with 5% CO2. The cell lines were cultured in Dulbecco’s modified Eagle medium (DMEM; Gibco, Waltham, MA, USA) with 10% fetal bovine serum (FBS), 100 U/mL of penicillin and 100 µg/mL of streptomycin. All cell lines used in this study were regularly authenticated by the short tandem repeat (STR) method and had not been in culture for more than 2 months.
Gene knockout in cell lines
Plasmid
The single guide RNA (sgRNA) expression constructs were cloned into the LentiCrispr-V2-puro backbone. The sequences of the sgRNAs used in this study were as follows: RPN1-sg1 (5'-TGTAGGCAACAATCACAGGG-3'), RPN1-sg2 (5'-TGAGGACGTGAAGCGCACAG-3').
Lentivirus package
The 293T cell line was seeded into a 6-well plate and cultured until a confluency of 70% was achieved. For the transfection procedure, tube A contained a mixture of the target gene plasmid, psPAX2, and pMD2.G, suspended in 150 µL of Opti-MEM medium at a ratio of 3 µg:2 µg:1 µg. Concurrently, tube B was prepared with 24 µL of polyethylenimine (PEI) added to an equal volume of Opti-MEM medium. After a 30-minute incubation period to allow for complex formation, the mixture was added to the cells. The culture medium was replaced with complete medium (with 10% FBS, 100 U/mL of penicillin and 100 µg/mL of streptomycin). At 48 hours post-transfection, the supernatant was collected and cleared of cellular debris using a 0.45-µm filter membrane. The lentivirus present in the supernatant was then concentrated using the Lenti-Pac Lentivirus Concentration Solution (GeneCopoeia, LT007, Rockville, MD, USA), in preparation for subsequent gene knockout experiments.
Lentiviral infection
Cell lines designated for infection were plated in 6-well plates and allowed to reach 70% confluency prior to the initiation of the lentiviral infection assay. Prepared lentiviral solution was then added to the culture dishes. After 24 hours, the medium was replaced, and at 72 hours post-infection, selection with puromycin commenced. Following 2 weeks of selection, stable transfectants were successfully established.
Apoptosis assay
A549 and MDA-MB-231 cells stably knocking down RPN1 were collected and rinsed twice with phosphate-buffered saline (PBS), and stained with propidium iodide (PI; KGA-108; KeyGen, Changchun, China) for 30 minutes at room temperature. Stained cells were then examined by flow cytometry and results were analyzed with FlowJo software [Becton, Dickinson and Co. (BD), Franklin Lakes, NJ, USA]. The apoptosis assay was repeated a total of three times, including both technical and biological replicates.
Immunofluorescence
Cells were seeded into glass-bottomed culture dishes (801002; NEST Biotechnology, Wuxi, China) 1 day before experiments. Cells were fixed with 4% paraformaldehyde for 15 minutes without permeabilization and blocked with 3% bovine serum albumin for 30 minutes at room temperature, and rinsed twice with PBS between the interval step. Next, the cells were incubated with the phalloidin (PHDH1; Cytoskeleton, Denver, CO, USA) for 2 hours at room temperature or overnight at 4 ℃. After rinsing three times with PBS, the cells were incubated for 2 hours at room temperature with the following secondary antibodies: anti-mouse Alexa Fluor-594. Nuclei were stained with Hoechst 33342 for 5 minutes (Molecular Probes, Invitrogen, Carlsbad, CA, USA). The cells were imaged using laser scanning confocal microscopes (LSM880, ZEN2.6, 63× oil lens; ZEISS, Oberkochen, Germany).
Western blotting
Cells were harvested and lysed in radioimmunoprecipitation assay (RIPA) buffer [50 mM Tris-HCl pH 7.5, 150 mM NaCl, 1 mM ethylenediaminetetraacetic acid (EDTA), 1% NP40] containing Protease Inhibitors Cocktails set I and Phosphatase Inhibitor Cocktails set II (Sigma-Aldrich, Darmstadt, Germany), and centrifuged at 12,000 rpm/min for 20 minutes at 4 ℃. The cell lysates were then boiled in gel loading buffer for 10 minutes and resolved by 10% sodium dodecyl sulfate polyacrylamide gel electrophoresis (SDS-PAGE). The proteins were transferred to polyvinylidene fluoride (PVDF) membranes (Millipore, Burlington, MA, USA), which were then blocked in PBS with 5% non-fat milk and 0.1% Tween-20 and immunoblotted with primary antibodies overnight at 4 ℃. Horseradish peroxidase-conjugated secondary antibodies were used, and high-signal enhanced chemiluminescence (ECL) substrate (Tanon, Shanghai, China) was used for detection. Primary antibodies were used: RPN1 (12894-1-AP, 1:1,000; Proteintech, Rosemont, IL, USA), FLNA [4762S, 1:1,000; Cell Signaling Technology (CST), Danvers, MA, USA], FLNB (12979S, 1:2,000; CST), myosin IIa (MYH9; 3403S, 1:1,000; CST), TLN1 (4021S, 1:1,000; CST), Drebrin (10260-1-AP, 1:2,000; Proteintech), and actin (MA5-11869, 1:500; Thermo Fisher, Waltham, MA, USA).
Data collection
To comprehensively analyze tumor samples, we obtained genomic, somatic mutation-related, and clinicopathological information from multiple databases. The Cancer Genome Atlas (TCGA) database (10), accessed through the University of California Santa Cruz Xena browser (http://xena.ucsc.edu/), provided a large dataset of 15,776 samples from 33 cancer types (the tumor types and their abbreviations are listed in Table S1) and normal tissues, enabling robust statistical analyses and meaningful conclusions about various types of cancer. To validate our results, we searched for 12 additional datasets from the Gene Expression Omnibus (GEO) database (https://www.ncbi.nlm.nih.gov/geo/) (11), providing diverse samples and confirming reproducibility across different cohorts. The process of selecting datasets begins with identifying cancer types that match those in TCGA, such as “squamous cell carcinoma of the lung”, rather than broadly categorizing them as “lung cancer”. Next, we choose the largest available dataset for this specific cancer type. The reason we only validated in 12 microarray datasets is because validation was not successful in the other four types of cancer, possibly due to insufficient available datasets for these four types of cancer. Table S2 includes detailed information on the GEO datasets used in this study. The integration of multiple databases and datasets ensures a comprehensive analysis of tumor samples and strengthens the validity of our findings.
Comparing the expression and activity levels of RPN1 between normal and tumor tissues across the TCGA and GEO databases
To investigate the biological function of a gene, it is essential to have a certain level of baseline expression. Therefore, we first calculated the median expression level of RPN1 in each cancer type using the “limma” package in R and compared its expression difference between normal and cancer tissues. The gene expression levels were normalized using the fragments per kilobase per million (FPKM) algorithm.
To ensure adequate gene activity for proper biological function, we assessed RPN1 activity in pan-cancer using the “GSVA” package (12). The package identified 100 genes co-expressed with RPN1 using the Pearson correlation test. Then, these gene sets were matched to a predefined gene set using single-sample gene set enrichment analysis (GSEA), and their expression statistics were aggregated into activity scores using the Gaussian algorithm. Similarly, RPN1 activity was also compared between tumor and normal tissues.
Clinical correlation of RPN1
We conducted a stratified analysis using age, gender, and disease stage to investigate the correlation between RPN1 mRNA expression and clinical parameters. Receiver operating characteristic (ROC) curves and areas under the curve (AUCs) were used to evaluate the diagnostic value of RPN1 in pan-cancer, utilizing the “pROC” package in R software, through the online tool Xiantao Academic (https://www.xiantao.love/). The overall survival (OS) of the TCGA pan-cancer cohort were assessed using univariate Cox regression with hazard ratios (HRs) and Kaplan-Meier (KM) method with log-rank test, using the “survival” package.
Kyoto Encyclopedia of Genes and Genomes (KEGG) pathway analysis
To explore the signaling pathways related to RPN1, we retrieved the different pathways between low and high expression groups for the RPN1 gene in pan-cancer from the KEGG database and performed GSEA. To determine statistical significance, we used corrected false discovery rates (FDRs) of <0.05 and absolute log2 fold changes of ≥1. The c2.cp.kegg.v7.4.symbols were utilized for pathway analysis as the annotation reference sets. The top 5 signaling pathways with the highest normalized enrichment scores (NESs) were visualized using the “enrichplot” package. This particular part of the enrichment analysis was executed utilizing the “org.Hs.eg.db” package.
Correlation between RPN1 and tumor microenvironment
The Estimation of Stromal and Immune cells in Malignant Tumors using Expression data (ESTIMATE) package (13), a specialized tool for evaluating the extent of stromal and immune cell infiltration in the tumor microenvironment, was employed to analyze the tumor microenvironment and its association with RPN1 expression levels. Utilizing this package, stromal and immune scores were computed for each individual sample, serving as indicators of cell infiltration within the tumor microenvironment. Higher scores indicated greater levels of cell infiltration in the corresponding samples. To assess the relationship between RPN1 expression levels and the stromal or immune scores, correlation coefficients and P values were employed as metrics.
Furthermore, the Cell-type Identification by Estimating Relative Subsets of RNA Transcripts (CIBERSORT) package (14) was utilized to estimate the composition of the 22 distinct immune cell types infiltrating the tumor. This estimation was based on a deconvolution algorithm enabling the quantification of immune cell proportions within the tumor microenvironment. The correlation between the presence of these immune cell types and RPN1 expression levels was examined. A correlation coefficient threshold of ≥0.3 and a P value threshold of <0.001 were applied to ascertain the statistical significance of the correlations during this two-stage analysis.
Potential immunotherapeutic implication of RPN1
The association between immune treatment response and various indicators, such as immune inhibitors, tumor mutation burden (TMB), and microsatellite instability (MSI), has been well established. In our study, we aimed to investigate the correlations between the expression levels of RPN1 and these indicators, with the objective of better understanding their potential role in immune response. To explore the relationship between RPN1 and immune inhibitors, we utilized the Tumor-Immune System Interaction Database (TISIDB) online database (http://cis.hku.hk/TISIDB/index.php). TMB, defined as the total number of somatic gene coding errors, base substitutions, insertions, or deletions detected per million bases, was quantified for each case by dividing the total number of mutations by the exome size (38 Mb). MSI scores were obtained from previously published studies for TCGA cancer cases (15). To visualize the results, we employed the “fmsb” package. Additionally, we evaluated the differential expression of RPN1 between the responder and non-responder groups, encompassing a total of 25 clinical studies on immune therapy. These studies specifically investigated the effectiveness of anti-programmed cell death 1 (PD-1), anti-programmed cell death ligand 1 (PD-L1), and anti-CTLA4 immunotherapy. Our objective was to assess whether RPN1 could serve as a predictive marker for immunotherapy efficacy. Detailed information about these clinical studies can be found in Table S3. To perform this analysis, we utilized the online database ICBatlas (http://bioinfo.life.hust.edu.cn/ICBatlas/) and visualized the results using the online tool Xiantao Academic (https://www.xiantao.love/).
Statistical analysis
All statistical analyses in this study were performed using R software (version 4.2.2; r Foundation for Statistical Computing, Vienna, Austria). Either the Student’s t-test or the Wilcoxon rank-sum test was employed to assess continuous variables, depending on data distribution. In cases where the data did not follow a Gaussian distribution, the Mann-Whitney test was utilized. Categorical clinicopathological characteristics were compared using the Chi-square test or Fisher’s exact test. Pearson correlation analysis was conducted for correlation analysis. Statistical significance was determined by P values of <0.05 or <0.01.
Results
RPN1 induced cell death under glucose-free condition in disulfidptosis
In Figure 2, the experimental results indicate that under glucose deprivation conditions, RPN1 induces disulfidptosis. Initially, we employed flow cytometry to analyze cell death in wild-type (WT) and RPN1-knockout (KO)1/KO2 MDA-MB-231 as well as A549 cells (Figure S1) cultured in glucose-containing (+Glc) or glucose-deficient (−Glc) media (Figure 2A). This analysis revealed the impact of glucose availability on cell survival. In glucose deficiency, cell death increased, a phenomenon mitigated by RPN1 knockout. Hence, the conclusion is drawn that RPN1 serves as a promoter of cell death. To further elucidate cellular changes, we utilized immunofluorescence labeling of F-actin with fluorescent phalloidin in +Glc or −Glc media to examine WT and RPN1-KO MDA-MB-231 as well as A549 cells (Figure 2B). This enabled visualization of alterations in the actin cytoskeleton under different glucose conditions. In glucose deprivation, cell death and cytoskeletal breakdown were observed. Upon RPN1 knockout, this cytoskeletal breakdown was suppressed, indicating that RPN1 mediates cell death through the degradation of cytoskeletal proteins. Protein extraction and western blot analysis using non-reducing and reducing methods were conducted to study the expression of indicative cytoskeletal proteins in normal control (NC) and RPN1-KO MDA-MB-231 as well as A549 cells cultured in +Glc or −Glc media (Figure 2C). Under glucose deprivation, the absence of RPN1 contributed to increased stability of cytoskeletal proteins (FLNA, FLNB, MYH9, TLN1, Drebrin, and Actin, Figure 2C). This demonstrates that RPN1 loss contributes to the maintenance of cytoskeletal integrity. These findings collectively underscore the role of RPN1 in mediating disulfidptosis, possibly through mechanisms involving the induction of cytoskeletal protein degradation.
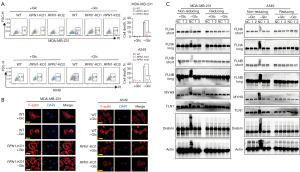
Differential expressions and activity of RPN1 were found in cancerous and normal tissues in 12 cancer types
Figure 3A depicts the expression pattern of RPN1 in 33 cancer tissues from TCGA databases, demonstrating robust mRNA expression levels (>5 FPKM normalized) in most solid tumors. Moreover, significant differences were observed between tumor and adjacent non-tumor tissues in 16 specific cancer types. Notably, BLCA, BRCA, CESC, CHOL, COAD, ESCA, GBM, HNSC, KIRP, LIHC, LUAD, LUSC, PRAD, READ, STAD, and UCEC exhibited higher expression levels in tumor tissues compared to adjacent non-tumor tissues (Figure 3B). In terms of gene activity, RPN1 exhibited robust activity in all 33 tumor tissues (Figure 3C). In comparison to adjacent non-tumor tissues, as illustrated in Figure 3D, RPN1 activity was generally higher in 19 tumor types, including BLCA, BRCA, CESC, CHOL, COAD, ESCA, GBM, HNSC, KIRP, LIHC, LUAD, LUSC, PAAD, PRAD, READ, STAD, THCA, THYM, and UCEC. This was corroborated by the differential expression levels of genes in the two tissue types, providing mutual validation of the results.
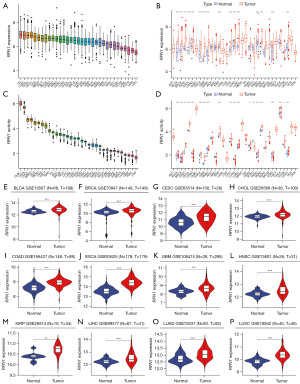
To validate the differential mRNA expression of RPN1 observed in the 12 tumor types with significant differences between tumor and adjacent non-tumor tissues, we utilized the GEO database for validation. As illustrated in Figure 3E-3P, the mRNA expression trends of RPN1 in 12 tumor types (including BLCA, BRCA, CESC, CHOL, COAD, ESCA, GBM, HNSC, KIRP, LIHC, LUAD, and LUSC) were consistent with the findings from the TCGA database across multiple validation datasets. Due to limited data availability in GEO, further validation of expression in PRAD, READ, STAD, and UCEC was not feasible.
RPN1 has moderate diagnostic and prognostic value
Using normal tissue samples from the TCGA database as a reference, we assessed the diagnostic potential of RPN1 (Figure 4A-4J). Our analysis revealed significant diagnostic value (AUC >0.8) for RPN1 in BLCA, BRCA, CESC, CHOL, COAD, ESCA, GBM, HNSC, LIHC, and LUSC. Notably, in the case of GBM (Figure 4G), the AUC reached an impressive value of 0.978, potentially influenced by the relatively small sample size in this dataset, comprising only five normal tissues and 174 tumor tissues.
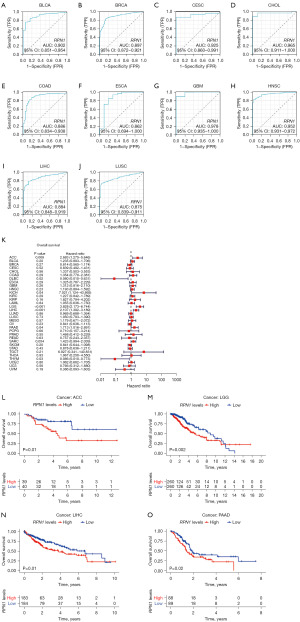
Additionally, the univariate Cox regression model indicated that high RPN1 expression in tumor tissues was associated with improved OS in DLBC [HR =0.080, 95% confidence interval (CI): 0.010–0.631, P=0.02, Figure 4K], and THYM (HR =0.086, 95% CI: 0.010–0.773, P=0.03, Figure 4K). However, it was linked to poorer prognosis in ACC (HR =2.683, 95% CI: 1.275−5.646, P=0.009), KICH (HR =7.021, 95% CI: 1.124−43.865, P=0.04), LGG (HR =3.828, 95% CI: 2.173−6.744, P<0.001), LIHC (HR =2.107, 95% CI: 1.392−3.189, P<0.001), and PAAD (HR =1.713, 95% CI: 1.016−2.891, P=0.04). These findings were partially corroborated by KM survival curves (Figure 4L-4O). Intriguingly, RPN1 exhibited favorable attributes in terms of expression profiling, diagnostic value, and prognostic significance across multiple cancer types, emphasizing its potential as a highly promising biomarker in this tumor context.
KEGG pathway and tumor microenvironment analyses show strong correlations between RPN1 expressions and immune response
To investigate potential mechanisms, we performed KEGG pathway enrichment analysis on different expression groups of RPN1 in eight validated cancer types (Figure 5, Table S4). Interestingly, regardless of the expression pattern, RPN1 was found to be enriched in immune-related pathways across multiple cancers. For example, it showed enrichment in the antigen processing and presentation pathway (NES =1.891, P=0.02, FDR q-value =0.405 in CESC; NES =1.728, P=0.013, FDR q-value =0.38 in LUSC), graft-versus-host disease (NES =1.735, P=0.026, FDR q-value =0.67 in KIRP; NES =1.787, P=0.038, FDR q-value =0.599 in BRCA), and RIG-I-like receptor signaling pathway (NES =1.921, P=0.017, FDR q-value =0.405 in CESC; NES =−1.422, P=0.01, FDR q-value =0.67 in COAD; NES =−1.775, P=0.013, FDR q-value =0.342 in LUAD; and NES =1.703, P=0.013, FDR q-value =0.380 in LUSC).
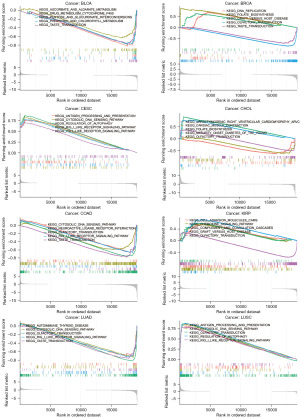
These findings prompted us to further investigate the relationship between RPN1 expression and the tumor microenvironment (Figure 6). Firstly, the ESTIMATE scoring system demonstrated a positive correlation between RPN1 expression and immune scores in LGG and SARC tumors (Figure 6A,6F), while it showed a negative correlation with PAAD (Figure 6D). Additionally, the CIBERSORT algorithm revealed correlations between RPN1 expression and the infiltration levels of various immune cells in multiple tumor tissues (Figure 6G-6O), although the distribution of this correlation appeared to be scattered and lacked a clear pattern. In summary, the preliminary mechanistic analysis results indicate a correlation between RPN1 and immune responses in tumor tissues, suggesting the need for further exploration of potential immune therapeutic responses.
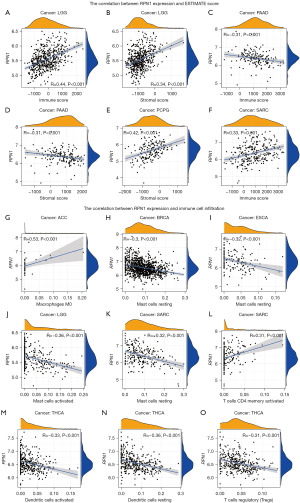
RPN1 expression potentially predicts immunotherapy efficacy for non-small cell lung cancer (NSCLC), RCC, and SKCM
After utilizing the TISIDB database, we identified notable correlations involving RPN1 expression in specific cancer types. In GBM, RPN1 expression displayed a positive correlation with the immune checkpoint IL10RB expression, whereas it exhibited a negative correlation with TGFBR1 expression (Figure 7A). Moreover, RPN1 expression showed significant correlations with TMB in THCA, STAD, SKCM, SARC, PAAD, LUSC, LUAD, LIHC, LGG, LAML, HNSC, DLBC, COAD, BRCA, and BLCA (Figure 7B). Similarly, RPN1 expression exhibited correlations with MSI scores in UCS, UCEC, STAD, LUSC, LUAD, LGG, HNSC, and BLCA (Figure 7C).
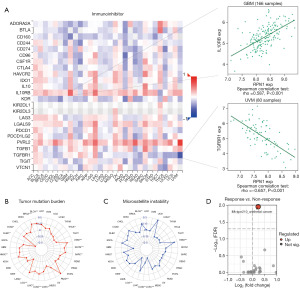
Furthermore, Figure 7D demonstrates the differential expressions of RPN1 in responder and non-responder groups across an anti-PD-L1 cohort. Specifically, responders with urothelial carcinoma (IMvigor210 study) exhibited up-regulated RPN1 expression.
Discussion
The RPN1 gene encodes a type I integral membrane protein found exclusively in the rough endoplasmic reticulum (16). It plays a crucial role as part of an N-oligosaccharyl transferase complex, which links high mannose oligosaccharides to asparagine residues within the Asn-X-Ser/Thr consensus motif of nascent polypeptide chains (16). This process is essential for protein N-glycosylation. The UMRC6 cells’ increased resistance to disulfidptosis upon RPN1 knockdown suggests that RPN1 may be involved in regulating cell survival pathways (3). However, the precise underlying mechanism remains unclear. In this study, we elucidated the previously unreported mechanistic role of RPN1 in inducing disulfidptosis. Through an investigation into cell death under glucose starvation in breast and lung cancer cell lines, we clarified the role of RPN1 in this process, primarily by inducing cell skeleton breakdown to promote disulfidptosis. To our knowledge, this study represents the first exploration of RPN1’s specific mechanism in disulfidptosis.
The relationship between RPN1 and cancer has been partially elucidated. For instance, RPN1 promotes proliferation, migration, and invasion of breast cancer cells through the PI3K/AKT/mTOR signaling pathway (8), possibly by inhibiting apoptosis triggered by endoplasmic reticulum stress (17). In HCC, survival analysis shows that high RPN1 expression is associated with adverse OS in HCC patients (9). Zheng et al. found that the Circ-SNX27 sponging miR-375/RPN1 axis contributes to HCC progression (18). Disulfidptosis-associated genes containing RPN1 have been used in risk models related to prognosis and immune characteristics of brain glioma (19) and HCC (20) patients, serving as independent prognostic factors for glioma. However, the comprehensive expression profile of RPN1 across pan-cancers, its clinical relevance, and its relationship with the tumor microenvironment remain unclear. In this study, we further examined the differential expression and activity of RPN1 across various cancer types. The results revealed strong mRNA expression of RPN1 in 16 solid tumors, with significant differences between tumor tissues of specific cancer types and adjacent non-tumor tissues. Additionally, RPN1 demonstrated robust activity in all analyzed tumor tissues. Validation from the GEO database confirmed consistent mRNA expression trends across 12 cancer types. RPN1 exhibited significant diagnostic potential across 12 cancer types, particularly with high accuracy in GBM. Elevated RPN1 expression in tumor tissues correlated with improved OS in DLBC and THYM, whereas it was associated with poorer prognosis in ACC, KICH, LGG, LIHC, and PAAD. Moreover, RPN1 was linked to immune response, being enriched in immune-related pathways, correlating with immune scores in tumor tissues, and associated with various immune cells. Notably, in UCC, RPN1 showed potential in predicting the efficacy of anti-PD-L1 immune therapy. In summary, these findings emphasized RPN1’s significance in pan-cancer scenarios and its implications for diagnosis, prognosis, and immune checkpoint inhibitor therapy. To our knowledge, this is the first study of RPN1 in pan-cancers integrating data from multiple public gene databases.
Glucose deprivation is a common feature of the tumor microenvironment, which induces metabolic reprogramming in tumor cells to maintain redox balance (21). For instance, tumor cells acquire glutathione as a protective mechanism by upregulating SLC7A11 expression (22), or adopt alternative strategies like IDH1 and ME1 to support NADPH formation (23). As suggested by the authors (3), future investigations should explore whether other metabolic stress conditions that deplete intracellular NADPH levels can induce dual sulfur death. However, it is crucial to note that the heterogeneity in the protective mechanisms employed by different tumor cells to maintain redox balance, especially in the context of pan-cancer. Therefore, the universal significance of inducing dual sulfur death under glucose deprivation as a novel therapeutic target for cancer needs clarification. Considering the dependency of dual sulfur death on the RPN1 gene, we examined the mRNA and protein expression levels of RPN1 across various cancer types to identify tumor types with potential for inducing dual sulfur death therapy. Importantly, we demonstrated inhibition of dual sulfur death upon RPN1 knockout in cell lines not validated in previous studies, regardless of SLC7A11 expression levels. This provides additional evidence for RPN1 as a potential universal target for cancer therapy. Furthermore, our study highlights the potential of RPN1 as an immune therapy marker, although further validation in cohorts is required.
This study has several limitations. Firstly, we did not further validate the differential expression of RPN1 in tumor and adjacent tissues obtained from our own tissue samples, which were used to verify the bioinformatics analysis. Specifically, investigating the expression of RPN1 in cohorts undergoing anti-PD-L1 therapy to validate its biomarker value in the real world was not performed. Secondly, some tumor types did not receive consistent validation across multiple databases, which may be related to systematic sampling biases. Finally, the detailed mechanism by which RPN1 promotes disulfidptosis through inducing cell skeleton breakdown requires further elucidation.
Conclusions
Overall, these findings emphasize RPN1’s significance in disulfidptosis induction, its pan-cancer relevance, and implications for diagnosis, prognosis, and immune therapy.
Acknowledgments
Funding: None.
Footnote
Reporting Checklist: The authors have completed the MDAR reporting checklist. Available at https://tcr.amegroups.com/article/view/10.21037/tcr-24-581/rc
Data Sharing Statement: Available at https://tcr.amegroups.com/article/view/10.21037/tcr-24-581/dss
Peer Review File: Available at https://tcr.amegroups.com/article/view/10.21037/tcr-24-581/prf
Conflicts of Interest: All authors have completed the ICMJE uniform disclosure form (available at https://tcr.amegroups.com/article/view/10.21037/tcr-24-581/coif). The authors have no conflicts of interest to declare.
Ethical Statement: The authors are accountable for all aspects of the work in ensuring that questions related to the accuracy or integrity of any part of the work are appropriately investigated and resolved. The study was conducted in accordance with the Declaration of Helsinki (as revised in 2013).
Open Access Statement: This is an Open Access article distributed in accordance with the Creative Commons Attribution-NonCommercial-NoDerivs 4.0 International License (CC BY-NC-ND 4.0), which permits the non-commercial replication and distribution of the article with the strict proviso that no changes or edits are made and the original work is properly cited (including links to both the formal publication through the relevant DOI and the license). See: https://creativecommons.org/licenses/by-nc-nd/4.0/.
References
- Machesky LM. Deadly actin collapse by disulfidptosis. Nat Cell Biol 2023;25:375-6. [Crossref] [PubMed]
- Zhang R, Kang R, Tang D. Reductive cell death: the other side of the coin. Cancer Gene Ther 2023;30:929-31. [Crossref] [PubMed]
- Liu X, Nie L, Zhang Y, et al. Actin cytoskeleton vulnerability to disulfide stress mediates disulfidptosis. Nat Cell Biol 2023;25:404-14. [Crossref] [PubMed]
- Qin Q, Li R, Li L, et al. Multi-target regulation of pro-inflammatory cytokine production by transcription factor Blimp-1. Inflamm Res 2023;72:217-20. [Crossref] [PubMed]
- Orth-He EL, Huang HC, Rao SD, et al. Protein folding stress potentiates NLRP1 and CARD8 inflammasome activation. Cell Rep 2023;42:111965. [Crossref] [PubMed]
- Liao KC, Mogridge J. Activation of the Nlrp1b inflammasome by reduction of cytosolic ATP. Infect Immun 2013;81:570-9. [Crossref] [PubMed]
- Lillo S, Saleh M. Inflammasomes in Cancer Progression and Anti-Tumor Immunity. Front Cell Dev Biol 2022;10:839041. [Crossref] [PubMed]
- Shen WJ, Zhang Y. RPN1 promotes the proliferation and invasion of breast cancer cells by activating the PI3K/AKT/mTOR signaling pathway. Discov Oncol 2024;15:25. [Crossref] [PubMed]
- Zheng W, Zheng Y, Bai X, et al. RPNs Levels Are Prognostic and Diagnostic Markers for Hepatocellular Carcinoma. J Oncol 2022;2022:7270541. [Crossref] [PubMed]
- Cancer Genome Atlas Research Network. The Cancer Genome Atlas Pan-Cancer analysis project. Nat Genet 2013;45:1113-20. [Crossref] [PubMed]
- Clough E, Barrett T, Wilhite SE, et al. NCBI GEO: archive for gene expression and epigenomics data sets: 23-year update. Nucleic Acids Res 2024;52:D138-44. [Crossref] [PubMed]
- Hänzelmann S, Castelo R, Guinney J. GSVA: gene set variation analysis for microarray and RNA-seq data. BMC Bioinformatics 2013;14:7. [Crossref] [PubMed]
- Yoshihara K, Shahmoradgoli M, Martínez E, et al. Inferring tumour purity and stromal and immune cell admixture from expression data. Nat Commun 2013;4:2612. [Crossref] [PubMed]
- Newman AM, Liu CL, Green MR, et al. Robust enumeration of cell subsets from tissue expression profiles. Nat Methods 2015;12:453-7. [Crossref] [PubMed]
- Bonneville R, Krook MA, Kautto EA, et al. Landscape of Microsatellite Instability Across 39 Cancer Types. JCO Precis Oncol 2017;2017:PO.17.00073.
- Ramírez AS, Kowal J, Locher KP. Cryo-electron microscopy structures of human oligosaccharyltransferase complexes OST-A and OST-B. Science 2019;366:1372-5. [Crossref] [PubMed]
- Ding J, Xu J, Deng Q, et al. Knockdown of Oligosaccharyltransferase Subunit Ribophorin 1 Induces Endoplasmic-Reticulum-Stress-Dependent Cell Apoptosis in Breast Cancer. Front Oncol 2021;11:722624. [Crossref] [PubMed]
- Zheng C, Liang J, Yu S, et al. Circ-SNX27 sponging miR-375/RPN1 axis contributes to hepatocellular carcinoma progression. Korean J Physiol Pharmacol 2023;27:333-44. [Crossref] [PubMed]
- Wang X, Yang J, Yang F, et al. The disulfidptosis-related signature predicts prognosis and immune features in glioma patients. Sci Rep 2023;13:17988. [Crossref] [PubMed]
- Wang Y, Yuan Z, Zhu Q, et al. Disulfidptosis-related genes of prognostic signature and immune infiltration features in hepatocellular carcinoma supported by bulk and single-cell RNA sequencing data. J Gastrointest Oncol 2024;15:377-96. [Crossref] [PubMed]
- Reinfeld BI, Madden MZ, Wolf MM, et al. Cell-programmed nutrient partitioning in the tumour microenvironment. Nature 2021;593:282-8. [Crossref] [PubMed]
- Lang X, Green MD, Wang W, et al. Radiotherapy and Immunotherapy Promote Tumoral Lipid Oxidation and Ferroptosis via Synergistic Repression of SLC7A11. Cancer Discov 2019;9:1673-85. [Crossref] [PubMed]
- Ying M, You D, Zhu X, et al. Lactate and glutamine support NADPH generation in cancer cells under glucose deprived conditions. Redox Biol 2021;46:102065. [Crossref] [PubMed]